- 1Department of Pharmacology, Faculty of Medicine, University of Malaya, Kuala Lumpur, Malaysia
- 2Department of Paediatrics, Faculty of Medicine, University of Malaya, Kuala Lumpur, Malaysia
- 3Centre for Population Health (CePH), Faculty of Medicine, University of Malaya, Kuala Lumpur, Malaysia
- 4Sports Medicine Unit, Faculty of Medicine, University of Malaya, Kuala Lumpur, Malaysia
- 5Department of Social and Preventive Medicine, Faculty of Medicine, University of Malaya, Kuala Lumpur, Malaysia
- 6Department of Nutrition, Harvard T.H. Chan School of Public Health, Boston, MA, United States
Background: Several non-synonymous single-nucleotide polymorphisms (nsSNPs) have been shown to be associated with obesity. Little is known about their associations and interactions with physical activity (PA) in relation to adiposity parameters among adolescents in Malaysia.
Methods: We examined whether (a) PA and (b) selected nsSNPs are associated with adiposity parameters and whether PA interacts with these nsSNPs on these outcomes in adolescents from the Malaysian Health and Adolescents Longitudinal Research Team study (n = 1,151). Body mass indices, waist–hip ratio, and percentage body fat (% BF) were obtained. PA was assessed using Physical Activity Questionnaire for Older Children (PAQ-C). Five nsSNPs were included: beta-3 adrenergic receptor (ADRB3) rs4994, FABP2 rs1799883, GHRL rs696217, MC3R rs3827103, and vitamin D receptor rs2228570, individually and as combined genetic risk score (GRS). Associations and interactions between nsSNPs and PAQ-C scores were examined using generalized linear model.
Results: PAQ-C scores were associated with % BF (β = −0.44 [95% confidence interval −0.72, −0.16], p = 0.002). The CC genotype of ADRB3 rs4994 (β = −0.16 [−0.28, −0.05], corrected p = 0.01) and AA genotype of MC3R rs3827103 (β = −0.06 [−0.12, −0.00], p = 0.02) were significantly associated with % BF compared to TT and GG genotypes, respectively. Significant interactions with PA were found between ADRB3 rs4994 (β = −0.05 [−0.10, −0.01], p = 0.02) and combined GRS (β = −0.03 [−0.04, −0.01], p = 0.01) for % BF.
Conclusion: Higher PA score was associated with reduced % BF in Malaysian adolescents. Of the nsSNPs, ADRB3 rs4994 and MC3R rs3827103 were associated with % BF. Significant interactions with PA were found for ADRB3 rs4994 and combined GRS on % BF but not on measurements of weight or circumferences. Targeting body fat represent prospects for molecular studies and lifestyle intervention in this population.
Introduction
Childhood obesity is a cause of much concern in Malaysia, a multiethnic country in South-East Asia, as the prevalence was estimated to be the highest in the region (22% in boys and 19% in girls) (1). An increased in health problems is foreseeable as the prevalence of overweight and obesity in Malaysian adults were already among the highest in the Asian region (1). Lifestyle factors such as eating behavior (2), sugary drink consumptions (3), lack of physical activity (PA) (4), and poor sleep quality (5) have been previously shown to be associated with increased risk of obesity in the Malaysian adolescents. Only a few studies have examined the genetic contribution to obesity in this population, one gene that had been studied being the neuropeptide-Y gene (6). Thus, further studies to examine possible associations of different single-nucleotide polymorphisms (SNPs) and obesity is timely.
Non-synonymous single-nucleotide polymorphisms (nsSNPs) have been widely studied in different diseases, while some were found to be associated with obesity in different populations. nsSNPs are SNPs with single amino acid substitution in a protein sequence that may lead to missense mutation or nonsense mutation. The change in protein sequent may subsequently affect protein structure and its protein interactions and exert possible functional effects (7). Among the nsSNP associated with adiposity is the fatty acid-binding protein-2 (FABP2) rs1799883 whereby the amino acid substitution from alanine to threonine was associated with increased sensitivity to carbohydrates (8). Another is the ghrelin (GHRL) rs696217 in which amino acid substitution from leucine to methionine may increase susceptibility to obesity and metabolic syndrome. The melanocortin-3-receptor (MC3R) missense mutation Val81Ile, rs3827103, was associated with increased susceptibility to obesity and may play a role in response to weight loss intervention (9–11). Other examples of nsSNPs reported to be associated with obesity included leptin (LEPR) rs1805094 (Lys656Asn) (12), beta-2 adrenergic receptor (ADRB2) rs1042714 (Glu27Gln) (13), beta-3 adrenergic receptor (ADRB3) rs4994 (Trp64Arg) (14), and vitamin D receptor (VDR) rs2228570 (Met1Thr) (15). Rarer nsSNPs such as the peroxisome proliferator-activated receptor gamma (PPARG) rs1800571 with gain-of-function mutation (Pro115Gln) has also been found to be possibly pathogenic for morbid obesity (16). These nsSNPs are thus relevant candidates to be studied in relation to obesity in our Malaysian adolescents.
Elsewhere, the interaction between genetic factors and the environment on obesity traits are increasingly being studied. Yet, studies on how genetic factors interact with environment factors are lacking in our unique population. A recent large meta-analysis examining genome-wide and PA interaction in obesity found that the effect of obesity risk loci of fat mass and obesity-associated (FTO) gene was attenuated by 30% in physically active individuals (17). There were increasing efforts to examine other possible candidate genes that may have similar effect as the FTO genes (18, 19). Less is known about the interaction of the above mentioned nsSNPs with PA in children. Studying the effect of gene and PA interaction using other adiposity parameters such as percentage body fat (% BF) from this region of the world may also potentially uncover different susceptible SNPs compared to when using body mass indices (BMI) in adolescents such as found in a study on children from New Zealand (20).
This study was aimed to examine possible associations between selected nsSNPs and adiposity parameters in the cohort of the Malaysian Health and Adolescents Longitudinal Research Team study (MyHeARTs) (21). MyHeARTs was the first longitudinal adolescent cohort study in Malaysia and among the few in South East Asia. This cohort provides a unique opportunity to study health and lifestyle factors in this region as well as genetic contribution to certain outcomes such as obesity. nsSNPs chosen were those previously shown to be associated with obesity and of possible functional significance. Different adiposity parameters such as BMI, waist–hip ratio (WHR), and % BF will be used. This study will also examine if there are interaction between these SNPs and self-reported PA in relation to adiposity for further planning of interventions of this growing obesity problem in adolescents.
Methodology
Study Population
This was a cross-sectional study from the second wave of the MyHeARTs in which 15-year-old participants were recruited from selected secondary schools between May 2014 and July 2014 (n = 1,179). Details of the MyHeARTs have been reported using a published protocol (21). The protocols were approved by the ethics committees of the University of Malaya Medical Center (MEC Ref. No: 896.34). In short, MyHeARTs was a longitudinal cohort study that recruited secondary school students from three different states in the Central and Northern Regions of Peninsular Malaysia, namely, Selangor, Federal Territory of Kuala Lumpur, and Perak. The first wave was recruited between March and May 2012 from the age of 13 years old (n = 1,361) (21).
Demographics and Puberty Status
Two self-administered questionnaires were used, a Parental Questionnaire and Student Questionnaire. These questionnaires were used to obtain demographic and lifestyle information. Puberty stages were self-reported based on Tanner staging system (22).
Assessment of PA
Self-reported PA was assessed using the validated Physical Activity Questionnaire for Older Children (PAQ-C) based on the Malay version, which has good internal consistency and acceptable validity (23, 24). The 10 items in the PAQ-C captured the levels of PA in the last 7 days including type and frequency of different selected activities, participation at separate times during the week, daily physical activities, and any unusual activities. Calculation of the PAQ-C score has been described elsewhere whereby the score ranges between 1 (low) and 5 (high) (25). Participants were classified into active (score ≥2.33) and inactive (score <2.33) (4).
Body Composition and Weight-Related Parameters
Measurements of these parameters were performed by trained personnel including body weight (kilograms), height, and waist and hip circumferences as per MyHeARTs study protocol (21). Height was measured without socks and shoes using a calibrated vertical Seca PorTable 217 Stadiometer (Seca, United Kingdom). Weight was measured with light clothing using a Seca 813 digital electronic weighing scale (Seca, United Kingdom). Both WC and HC were measured with a non-elastic Seca measuring tape (Seca 201, Seca, UK), to the nearest millimeter. A portable body composition analyzer (Tanita SC-240 MA, Body Composition Analyser, Tanita Europe B.V., The Netherlands) was used to measure % BF. BMI was calculated as baseline body weight divided by height squared (kilograms per square meter). WHR was calculated as WC divided by HC (WC/HC). BMI was classified according to the World Health Organization criteria (26). For this study, adiposity parameters examined were BMI, WHR as measure of central adiposity, and % BF.
SNP Selection and Genotyping
Genomic DNA from blood samples was extracted using GeneAll DNA Extraction Kits (Qiagen, Hilden, Germany). The quality of the extracted DNA was such that the absorbance ratios of at least 1.8 was attained for both 260/280 and 260/230 readings. All DNA samples and duplicates were diluted to 10 and 20 ng/µL, respectively, before being transferred to the respective wells. Quality controls included a blank and five duplicates.
Non-synonymous single-nucleotide polymorphisms that were reported to alter the amino acid sequence of protein candidate genes with multiple allele frequency (MAF) of more than 0.05 (except PPARG rs1800571) and have been previously associated with obesity were selected from the NCBI SNP database. A total of 11 nsSNPs within 10 candidate genes, namely, ADRB2 (rs1042714), ADRB3 (rs4994), FABP2 (rs1799883), GHRL (rs696217 and rs4684677), IGFBP1 (rs4619), LEPR (rs1805094), MC3R (rs3827103), PPARG (rs1800571), SHBG (rs6259), and VDR (rs2228570) were genotyped (see Table S1 in Supplementary Material for MAF and call rate). Genotyping was performed using a Sequenom MassARRAY platform with iPLEX GOLD chemistry (Sequenom, San Diego, CA, USA) following the manufacturer’s protocols. The MassARRAY system was created based on the technology of matrix-assisted laser desorption/ionization time-of-flight mass spectrometry.
Statistical Analysis
Power calculation was performed using QUANTO (version 1.2.4, University of Southern California, Los Angeles, CA, USA). An estimated sample size of 1,059 would provide 80% power at an alpha of 0.05. The sample from MyHeARTs were sufficient to carry out further statistical analysis as pooled participants. For interaction analysis, we included all participants (case-only approach) and an estimated sample of 1,116 could achieve 80% power to detect gene–environment interaction in a model whereby the relative efficiency = 1 and p = 0.05 (27).
Continuous variables were presented as means with SDs, while categorical variables were presented as frequency and percentages. Variables were tested for normal distribution using the Kolmogorov–Smirnov test and further assessed using the Q–Q plots. The spread of data was obtained from the data itself. BMI and % BF did not conform to normal distribution and hence log transformation was performed for regression analysis. Comparisons of categorical variables were performed using χ2 test while the Students’ T-test and Mann–Whitney U test were used to compare continuous variable between those who were physically active and those inactive.
For each SNP, missing values were not imputed for all analyses. Genotype distribution was assessed for Hardy–Weinberg equilibrium (HWE) by using the χ2 test. A p value of more than 0.05 signifies agreement with HWE. 4 nsSNPs (ADRB2 rs1042714, IGFBP1 rs4619, GHRL rs4684677, and PPARG rs1800571) deviated from the HWE and were excluded from the final analyses (Table S1 in Supplementary Material). SNPs with very low frequencies of homozygote variants (n < 10) were also excluded from further analysis: LEPR rs1805094 and SHBG rs6259 (Table S1 in Supplementary Material). Unweighted genetic risk score (GRS) were calculated using the method described by Reddon et al. whereby the risk alleles of the five chosen nsSNPs were summed (each risk allele was scored as 1 and the other allele as 0) (19). The GRS scores could range from 0 to 10.
Linear regression using the generalized linear model (GLM) was used to examine the associations between PA and adiposity parameters (log transformed BMI, WHR, and log transformed % BF) using univariate and multivariate analysis adjusting for gender, ethnicity, and pubertal status. The GLM was also used to examine the association between genotypes of selected nsSNPs (homozygous, heterozygous, and homozygous variant) and adiposity parameters adjusting for covariates above. For WHR, the model included BMI as additional covariates. GLM was used to examine the interactions between individual nsSNPs, measured as additive allele (0 = no risk allele, 1 = 1 risk allele, and 2 = 2 risk alleles) and PA (total PAQ-C score) on logBMI, WHR, and log % BF. Interactions were examined (1) as unadjusted model (nsSNP × PA) and (2) by including a product term (PAQ-C scores × nsSNPs) adjusted for gender, ethnicity, pubertal stages, PAQ-C scores, and nsSNP.
The association between combined GRS and adiposity parameters and its interaction with PA were also examined using the same GLM model as described above. Associations and interaction effect were presented as beta (β) with 95% confidence interval (CI). To correct for testing for multiple adiposity parameters and SNPs, the false discovery rate (FDR) was calculated using Benjamini–Hochberg procedure (28). The FDR chosen was 0.25 and a corrected p-value of less than 0.025 was considered as statistically significant. Statistical analysis was performed using SPSS 24.0 software (IBM SPSS Statistics, NY, USA).
Results
Characteristics of the MyHeARTs Participants
Of the 1,151 15-year-old adolescents, 61% were females, 79% were of Malay ethnicity, and 62% had reached puberty (Table 1). Based on their PAQ-C scores, 48% were considered physically active while more than half were inactive. It was found that 863 adolescents (76%) were thin or of normal weight while 277 (24%) were classified as overweight or obese with no significant differences found across PA levels. Those who were physically active have significantly lower WHR (p = 0.01) and % BF (p < 0.0001) compared to those who were inactive.
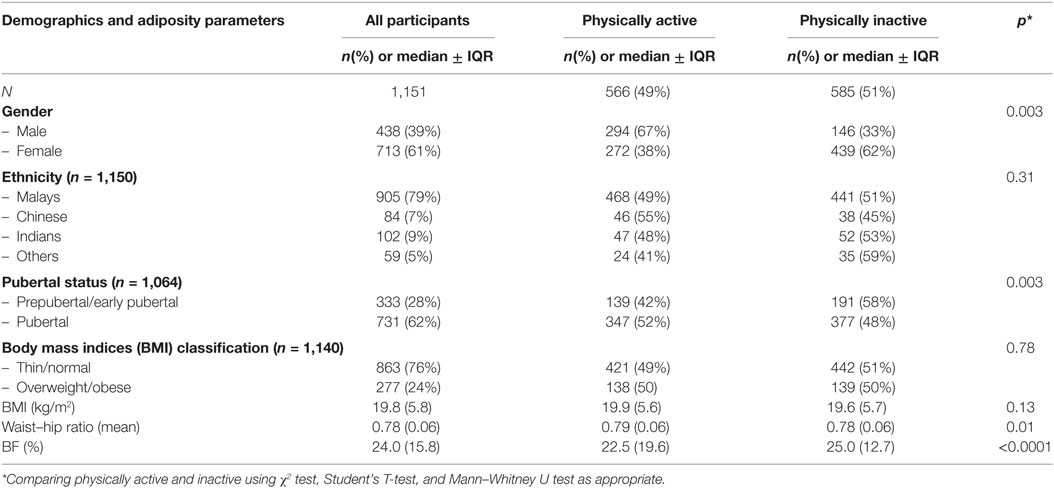
Table 1. Demographics and adiposity parameters of Malaysian adolescents from the Malaysian Health and Adolescents Longitudinal Research Team study comparing physically active and inactive participants.
Association Between PA (PAQ-C Scores) and Adiposity Parameters
In unadjusted regression model, those with higher total PAQ-C score were associated with significantly increased WHR and reduced % BF compared to those who with lower scores (Table 2). After adjustment, only % BF remained significantly associated with PAQ-C scores. Higher PAQ-C scores were associated with reduced % BF (β = −0.44 [95% CI −0.72, −0.16] p = 0.002).
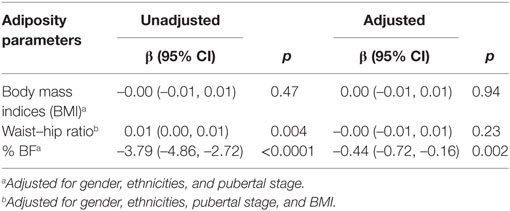
Table 2. Association of physical activity (Physical Activity Questionnaire for Older Children scores) and adiposity parameters presented as β with 95% confidence interval (CI) and p-value.
Association Between nsSNPs/GRS and Adiposity Parameters
The genotype distribution of selected nsSNPs and its association with adiposity parameters in the MyHeARTs cohort are presented in Table 3. In the unadjusted models, homozygote variants of ADRB3 rs4994 and MC3R rs3827103 were associated with reduced % BF, while FABP2 rs1799883 and GHRL rs696217 were associated with increased % BF. In the multivariate analysis and after considering correction for multiple testing, only the CC genotype of ADRB3 rs4994 (β = –0.16 [95% CI –0.28, –0.05], corrected p = 0.01) and AA genotype of MC3R rs3827103 (β = –0.06 [95% CI –0.12, –0.00], p = 0.02) were significantly associated with % BF compared to TT and GG genotypes, respectively. No other significant associations were found between nsSNPs and adiposity parameters in this population.
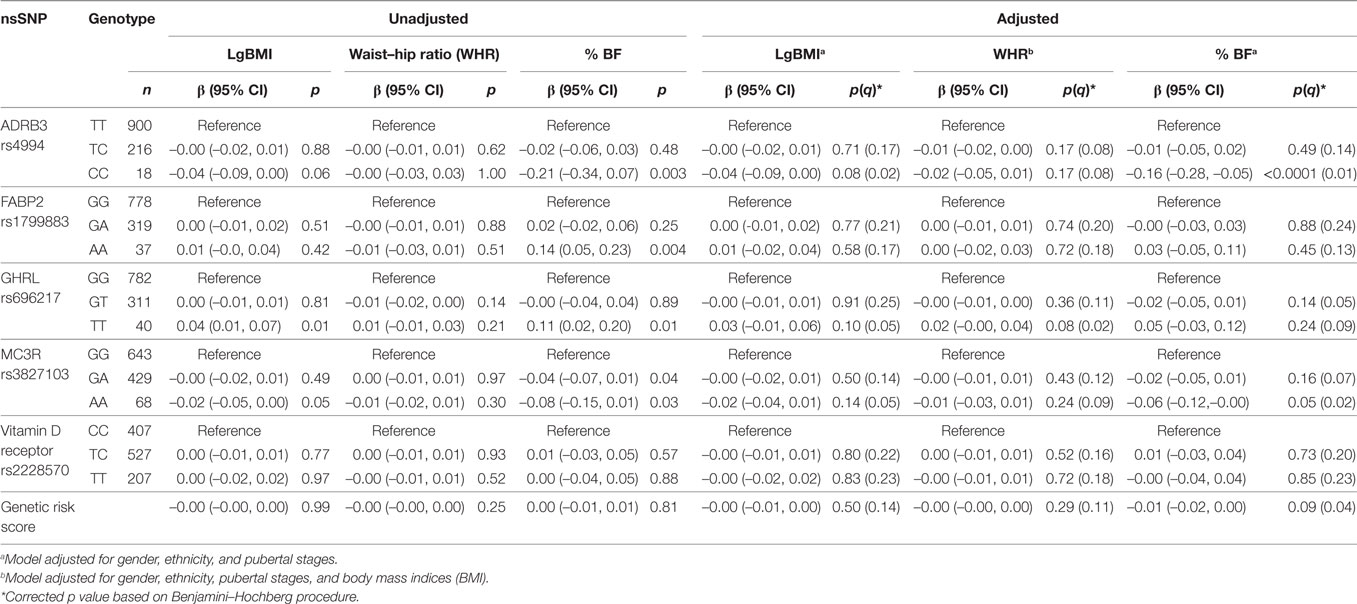
Table 3. Association between selected non-synonymous single-nucleotide polymorphisms (nsSNPs) and adiposity parameters in Malaysian Health and Adolescents Longitudinal Research Team study adolescents presented as β with 95% confidence interval (CI) and p values [uncorrected and corrected (q)].
Interaction Between nsSNPs and PA on Adiposity Parameters
In the unadjusted model, there were significant interactions found between ADRB3 rs4994, MC3R rs3827103, VDR rs2228570, and GRS and total PAQ-C scores on % BF (Table 4). However, in the adjusted model that included the main effects, significant interaction with PA on % BF were observed only with ADRB3 rs4994 (β = –0.05[–0.10,–0.01], p = 0.02) and combined GRS (β = –0.03[–0.04,–0.01], p = 0.01) with small effect size observed per unit increase.
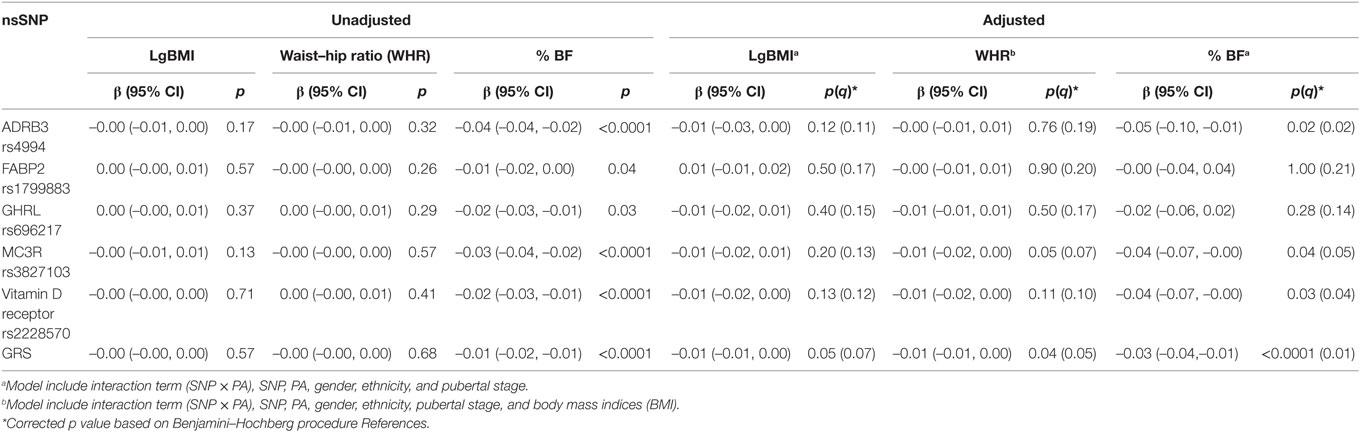
Table 4. Interaction between selected non-synonymous single-nucleotide polymorphisms (nsSNPs) (additive allele model) and their combined genetic risk score (GRS) and physical activity (Physical Activity Questionnaire for Older Children scores) in Malaysian Health and Adolescents Longitudinal Research Team study adolescents presented as β and 95% confidence interval (CI) and p values (uncorrected and corrected q).
Discussion
Of the MyHeARTs participants, almost 25% were considered overweight and obese and 48% were physically inactive. Higher PA scores were significantly associated with reduced % BF in our adolescents. % BF was shown to be more influenced by nsSNPs rather than measurement of BMI or circumferences with CC genotype of ADRB3 rs4994 and AA genotype of MC3R rs3827103 shown to be associated with small but significant protective effect on this adiposity measure. Significant interactions with PA on adiposity measures were demonstrated where ADRB3 rs4994 and combined GRS shown to interact with PA on % BF with overall protective effect.
Previous study on Malaysian adolescents from the first wave of MyHeARTs cohort demonstrated inverse correlation between PA scores and % BF, as well as waist circumference and BMI (4). In addition, it was shown that the levels of PA in adolescents who were obese were low, whereby less than a third were considered as physically active. The protective effect of PA on adiposity parameters represents an opportunity for early intervention in adolescents, in view of the high prevalence of obesity in this country (1). Of concern, significant downward trends of PA were shown in Malaysian girls in the 2-year follow-up of the same cohort accompanied by concurrent % BF increment (29). Thus, effective policies to promote PA in this age group are warranted with interventions to be planned at all levels of the community (30).
The protective effects of the MC3R rs3827103 on body fat was particularly evident in subjects with AA genotype. MC3R may have a role in inhibiting energy storage and lowering food efficiency—the body’s ability to convert food to adiposity (31). By contrast, a study among obese children in Thailand on this nsSNPs showed no significant association with adiposity (32). We observed that the ADRB3 rs4994 were associated with changes in % BF where CC homozygotes had protective effects. ADRB3 rs4994 has been reported to be associated with body fat distribution in Koreans (33). The ADRB3 rs4994 (Trp64Arg) polymorphism may exert functional effect on the β3-adrenergic receptor which are involved in regulating lipolysis in adipose tissues and have a role in energy expenditure (34). Kurokawa et al. in his meta-analysis demonstrated that the ADRB3 rs4994 were associated with obesity in East Asian rather than in Caucasians (14). However, studies on this SNPs yielded inconsistent results (35) and thus it has been suggested that this SNP may demonstrate ethnic heterogeneity (36).
We did not observe significant association between GHRL rs696217 polymorphisms and any adiposity markers. The GHRL rs696217 or leu72met was one of the most commonly studied SNPs of the preproghrelin gene and lies outside the region where mature ghrelin product is encoded (37), hence its functional significance remains uncertain. Nevertheless, as ghrelin is an orexigenic peptide, this mutation might produce an increased expression or gain in function of the peptide. This is thought to eventually influence ghrelin secretion and/or activity which is important in regulating satiety, food intake and energy balance in human (38). Studies in both children and adult populations demonstrated associations between this nsSNP and adiposity parameters such as BMI (39–41), visceral fat areas (42) and HC (43) while others could not demonstrate significant associations (44, 45). Furthermore, we did not observe any significant association between FABP2 rs1799883 and VDR rs2228570 polymorphisms and any adiposity markers. Their association with obesity-related parameters have been previously studied in different populations (14, 31–33, 46–50) with differing results. This could be possibly due to the genetic heterogeneity or differences between populations in terms of environmental factors that influence phenotypic expression of the gene variants.
ADRB3 rs4994 and combined GRS of these nsSNPs was shown to interact with PA on % BF while no significant interactions were found for other adiposity parameters. A study by Marti et al. suggested that ADRB3 rs4994 interacts with PA whereby it is a risk factor for obesity among inactive but not in physically active individuals (51). In contrast to our study, the Finnish Diabetes Prevention Study found that GHRL rs696217 modified the effect of moderate-to-vigorous PA on weight and WC in their overweight cohort (18). Studying the effect of gene and PA interaction using adiposity parameters such as % BF instead of the commonly used measures of BMI may potentially uncover novel susceptible SNPs compared to when using BMI in children and adolescent. In the Asia Pacific region, the SCOPE study on 6-year-old children New Zealander of Caucasian origin found that SNPs such as OLFM4-9568856, GNPDA2-rs10938397, CLOCK-rs4864548, and LEPR-1045895 showed genotype differences with PA when comparing % BF (20). This New Zealand study suggested that children who maybe genetically predisposed to obesity could benefit from higher levels of PA to improve their body fat profile. Although our study differs from the New Zealand in terms of ethnicities of children involved, the key message remains true. Targeting at-risk children and adolescents at time when their PA levels are still modifiable by interventions represents opportunity to reduce obesity in the general population. Yet, it is worth noting that meta-analysis of randomized controlled trial could not find the differential effects of the FTO SNPs, the most commonly studied SNPs and environment interaction, on weight loss interventions (52). This raises the question on the clinical significance of gene and environment interaction on interventions targeting obesity. Utilization of multiple gene models is necessary in further studies to consider exercise intervention as a preventive health strategy which is clinically relevant to predict the individual response.
To our knowledge, this is the first adolescent study on adiposity in Malaysia that has included both genetic and environment components using comprehensive anthropometric assessments. The MyHeARTs recruited adolescents from different states in Malaysia encompassing both urban and rural settings and different socioeconomic groups. This longitudinal cohort provides a wealth of information on adolescents’ health and lifestyle in this region of the world. As this study is part of a longitudinal cohort, there were limitations in terms of participants included. This cohort has low representative of the Chinese ethnic groups and therefore analyses were only performed in pooled participants. Thus, the sample is rather heterogeneous in terms of ethnicity. We included ethnicity as covariates in the association study, however, replication of this study using higher number of adolescents from these ethnicities of similar ancestries should be planned to allow stratification of genetic risk according to ethnicity. Besides twins and siblings, family relationship between participants were not able to be ascertained. Physical activities were self-reported by students rather than measured by devices and thus may still be subjected to recall bias. However, the PAQ-C is a validated tool that has good internal consistency and acceptable validity in Malaysia (4). We did not include dietary or nutritional information to account for the effect of these environmental factors in our models.
Selection of only nsSNPs to be studied has its own limitations. NsSNPs generally have lower frequency than synonymous SNP; hence, there lies the probability of false positive in a small sample of general population. Six out of 11 nsSNPs genotyped were not included in the final analysis due to deviation from HWE or low frequency of homozygous variants which may reflect differences in our population compared to others. The number of adolescents in the extreme phenotype (morbid obesity) was small and therefore was not studied separately. This group may represent true risk of genetic and environment interactions especially when considering non-synonymous mutation and hence future study using methods such as exome sequencing should be considered.
In conclusion, higher PA score was associated with reduced % BF in our Malaysian adolescents. Only ADRB3 rs4994 and MC3R rs3827103 were shown to be associated with reduced % BF, while the other nsSNPs were not found to be associated with adiposity measures in our population. Significant interactions with PA were found for ADRB3 rs4994 and combined GRS on % BF rather than measurements of weight or circumferences. These findings represent prospects for molecular studies and opportunity to incorporate genetic and lifestyle factors in elucidating the contributions to obesity problem and planning of appropriate intervention for Malaysian adolescents, especially targeting at the body fat. Further follow-up from this longitudinal cohort should examine changes in the interaction of PA and SNPs on % BF over time.
Ethics Statement
The genetic part of the study was carried out in accordance with the recommendations of Guidelines on the Ethical Practice in Human Genetics and Human Genomic Research, Universiti Sains Malaysia (USM) Human Research Ethics Committee with written informed consent from all subjects (53). All subjects gave written informed consent in accordance with the Declaration of Helsinki. The protocol was approved by the ethics committees of the University of Malaya Medical Centre (MEC Ref. No: 896.34).
Author Contributions
All authors are involved in the conception or design of the work. HM, NM, NZ, TS, MJ, ZM, and MM are involved in acquisition, analysis, and interpretation of data for the work; NZ and NM drafted the manuscript, and all authors were involved in revising the manuscript and final approval of the submitted manuscript.
Conflict of Interest Statement
The authors declare that the research was conducted in the absence of any commercial or financial relationships that could be construed as a potential conflict of interest.
Acknowledgments
The authors acknowledge the contributions of other co-investigators, postgraduate students, and research assistants involved in this longitudinal study including Dr Yamunah Appalasamy. The authors are grateful to all the students and their parents or guardians for their participation in making this study a success. They would also like to extend our gratitude to all the school teachers and colleagues for their support and assistance in this study.
Funding
This study is funded by the High Impact Research, Ministry of Higher Education Grant, Malaysia (HIR H00021-00-E000082), the University Malaya Research Grant (UMRP022A and C-14HTM), and the University Malaya Institute of Postgraduate Research Grant (PG317-2016A).
Supplementary Material
The Supplementary Material for this article can be found online at https://www.frontiersin.org/articles/10.3389/fendo.2018.00209/full#supplementary-material.
References
1. Ng M, Fleming T, Robinson M, Thomson B, Graetz N, Margono C, et al. Global, regional, and national prevalence of overweight and obesity in children and adults during 1980-2013: a systematic analysis for the global burden of disease study 2013. Lancet (2014) 384(9945):766–81. doi:10.1016/S0140-6736(14)60460-8
2. Loh DA, Moy FM, Zaharan NL, Mohamed Z. Eating behaviour among multi-ethnic adolescents in a middle-income country as measured by the self-reported children’s eating behaviour questionnaire. PLoS One (2013) 8(12):e82885. doi:10.1371/journal.pone.0082885
3. Loh DA, Moy FM, Zaharan NL, Jalaludin MY, Mohamed Z. Sugar-sweetened beverage intake and its associations with cardiometabolic risks among adolescents. Pediatr Obes (2017) 12(1):e1–5. doi:10.1111/ijpo.12108
4. Su TT, Sim PY, Nahar AM, Majid HA, Murray LJ, Cantwell MM, et al. Association between self-reported physical activity and indicators of body composition in Malaysian adolescents. Prev Med (2014) 67:100–5. doi:10.1016/j.ypmed.2014.07.001
5. Firouzi S, Poh BK, Ismail MN, Sadeghilar A. Sleep habits, food intake, and physical activity levels in normal and overweight and obese Malaysian children. Obes Res Clin Pract (2014) 8(1):e70–8. doi:10.1016/j.orcp.2012.12.001
6. Zain SM, Mohamed Z, Jalaludin MY, Fauzi F, Hamidi A, Zaharan NL. Comprehensive evaluation of the neuropeptide-Y gene variants in the risk of obesity: a case-control study and meta-analysis. Pharmacogenet Genomics (2015) 25(10):501–10. doi:10.1097/FPC.0000000000000164
7. Yates CM, Sternberg MJ. The effects of non-synonymous single nucleotide polymorphisms (nsSNPs) on protein-protein interactions. J Mol Biol (2013) 425(21):3949–63. doi:10.1016/j.jmb.2013.07.012
8. Imtiaz S, Nasir K, Dhrolia MF, Hussain M, Abbas HN, Ahmad A. Mortality trend among hemodialysis patients during the Islamic month of Ramadan: a 24 years retrospective study. J Coll Physicians Surg Pak (2015) 25(3):189–92.doi: 03.2015/jcpsp.189192
9. Fox CS, Heard-Costa NL, Vasan RS, Murabito JM, D’Agostino Sr RB, Atwood LD, et al. Genomewide linkage analysis of weight change in the Framingham heart study. J Clin Endocrinol Metab (2005) 90(6):3197–201. doi:10.1210/jc.2004-1752
10. Obregon AM, Diaz E, Santos JL. Effect of the melanocortin-3 receptor Thr6Lys and Val81Ile genetic variants on body composition and substrate oxidation in Chilean obese children. J Physiol Biochem (2012) 68(1):71–6. doi:10.1007/s13105-011-0120-4
11. Santos JL, De la Cruz R, Holst C, Grau K, Naranjo C, Maiz A, et al. Allelic variants of melanocortin 3 receptor gene (MC3R) and weight loss in obesity: a randomised trial of hypo-energetic high- versus low-fat diets. PLoS One (2011) 6(6):e19934. doi:10.1371/journal.pone.0019934
12. Ghalandari H, Hosseini-Esfahani F, Mirmiran P. The association of polymorphisms in leptin/leptin receptor genes and ghrelin/ghrelin receptor genes with overweight/obesity and the related metabolic disturbances: a review. Int J Endocrinol Metab (2015) 13(3):e19073. doi:10.5812/ijem.19073v2
13. Apalasamy Y, Ming M, Rampal S, Bulgiba A, Mohamed Z. Gender-dependent association of a β(2)-adrenergic gene variant with obesity parameters in Malaysian Malays. Asia Pac J Public Health (2015) 27(2):N154–65. doi:10.1177/1010539511430250
14. Kurokawa N, Young EH, Oka Y, Satoh H, Wareham NJ, Sandhu MS, et al. The ADRB3 Trp64Arg variant and BMI: a meta-analysis of 44 833 individuals. Int J Obes (2008) 32(8):1240–9. doi:10.1038/ijo.2008.90
15. Han F, Lv Y, Gong L, Liu H, Wan Z, Liu L. VDR gene variation and insulin resistance related diseases. Lipids Health Dis (2017) 16(1):157. doi:10.1186/s12944-017-0477-7
16. Ristow M, Müller-Wieland D, Pfeiffer A, Krone W, Kahn C. Obesity associated with a mutation in a genetic regulator of adipocyte differentiation. N Engl J Med (1998) 339(14):953–9. doi:10.1056/NEJM199810013391403
17. Graff M, Scott RA, Justice AE, Young KL, Feitosa MF, Barata L, et al. Genome-wide physical activity interactions in adiposity – a meta-analysis of 200,452 adults. PLoS Genet (2017) 13(4):e1006528. doi:10.1371/journal.pgen.1006528
18. Kilpelainen TO, Lakka TA, Laaksonen DE, Mager U, Salopuro T, Kubaszek A, et al. Interaction of single nucleotide polymorphisms in ADRB2, ADRB3, TNF, IL6, IGF1R, LIPC, LEPR, and GHRL with physical activity on the risk of type 2 diabetes mellitus and changes in characteristics of the metabolic syndrome: the Finnish Diabetes Prevention Study. Metabolism (2008) 57(3):428–36. doi:10.1016/j.metabol.2007.10.022
19. Reddon H, Gerstein HC, Engert JC, Mohan V, Bosch J, Desai D, et al. Physical activity and genetic predisposition to obesity in a multiethnic longitudinal study. Sci Rep (2016) 6:18672. doi:10.1038/srep18672
20. Krishnan M, Shelling AN, Wall CR, Mitchell EA, Murphy R, McCowan LME, et al. Gene-by-activity interactions on obesity traits of six year old New Zealand European children: a children of SCOPE study. Pediatr Exerc Sci (2017) 30(1):69–80. doi:10.1123/pes.2017-0077
21. Hazreen MA, Su TT, Jalaludin MY, Dahlui M, Chinna K, Ismail M, et al. An exploratory study on risk factors for chronic non-communicable diseases among adolescents in Malaysia: overview of the Malaysian Health and Adolescents Longitudinal Research Team study (The MyHeART study). BMC Public Health (2014) 14(Suppl 3):S6. doi:10.1186/1471-2458-14-S3-S6
22. Sun Y, Tao F, Su P; China Puberty Research Collaboration. Self-assessment of pubertal Tanner stage by realistic colour images in representative Chinese obese and non-obese children and adolescents. Acta Paediatr (2012) 101(4):e163–6. doi:10.1111/j.1651-2227.2011.02568.x
23. Kowalski KC, Crocker PR, Faulkner RA. Validation of the Physical Activity Questionnaire for Older Children. Pediatr Exerc Sci (1997) 9(2):174–86. doi:10.1123/pes.9.2.174
24. Dan S, Mohd N, Zalilah M. Determination of factors associated with physical activity levels among adolescents attending school in Kuantan, Malaysia. Malays J Nutr (2011) 17(2):175–817.
25. Crocker PR, Bailey DA, Faulkner RA, Kowalski KC, McGrath R. Measuring general levels of physical activity: preliminary evidence for the Physical Activity Questionnaire for Older Children. Med Sci Sports Exerc (1997) 29(10):1344–9. doi:10.1097/00005768-199710000-00011
26. Himes JH. Challenges of accurately measuring and using BMI and other indicators of obesity in children. Pediatrics (2009) 124(Suppl 1):S3–22. doi:10.1542/peds.2008-3586D
27. Murcray C, Lewinger J, Conti D, Thomas D, Gauderman W. Sample size requirements to detect gene-environment interactions in genome-wide association studies. Genet Epidemiol (2011) 35(3):201–10. doi:10.1002/gepi.20569
28. Benjamini Y, Hochberg Y. Controlling the false discovery rate – a practical and powerful approach to multiple testing. J R Stat Soc Ser B Methodol (1995) 57(1): 289–300.
29. Majid HA, Amiri M, Azmi NM, Su TT, Jalaludin MY, Al-Sadat N. Physical activity, body composition and lipids changes in adolescents: analysis from the MyHeART Study. Sci Rep (2016) 6:30544. doi:10.1038/srep30544
30. Van Sluijs EM, McMinn AM, Griffin SJ. Effectiveness of interventions to promote physical activity in children and adolescents: systematic review of controlled trials. BMJ (2007) 335(7622):703. doi:10.1136/bmj.39320.843947.BE
31. Chen AS, Marsh DJ, Trumbauer ME, Frazier EG, Guan XM, Yu H, et al. Inactivation of the mouse melanocortin-3 receptor results in increased fat mass and reduced lean body mass. Nat Genet (2000) 26(1):97–102. doi:10.1038/79254
32. Wannaiampikul S, Phonrat B, Tungtrongchitr A, Limwongse C, Chongviriyaphan N, Santiprabhob J, et al. Genetic variant screening of MC3R and MC4R genes in early-onset obese children and their relatives among a Thai population: family-based study. Genet Mol Res (2015) 14(4):18090–102. doi:10.4238/2015.December.22.35
33. Kim K, Lee S, Lee S, Lim K, Cheun W, Ahn N, et al. Comparison of body fat distribution and blood lipid profiles according to Trp64Arg polymorphism for the beta 3-adrenergic receptor gene in Korean middle-aged women. J Nutr Sci Vitaminol (2006) 52(4):281–6. doi:10.3177/jnsv.52.281
34. Umekawa T, Yoshida T, Sakane N, Kogure A, Kondo M, Honjyo H. Trp64Arg mutation of beta3-adrenoceptor gene deteriorates lipolysis induced by beta3-adrenoceptor agonist in human omental adipocytes. Diabetes (1999) 48(1):117–20. doi:10.2337/diabetes.48.1.117
35. Daghestani M, Daghestani M, Daghistani M, Eldali A, Hassan ZK, Elamin MH, et al. ADRB3 polymorphism rs4994 (Trp64Arg) associates significantly with bodyweight elevation and dyslipidaemias in Saudis but not rs1801253 (Arg389Gly) polymorphism in ARDB1. Lipids Health Dis (2018) 17(1):58. doi:10.1186/s12944-018-0679-7
36. Zhan S, Ho SC. Meta-analysis of the association of the Trp64Arg polymorphism in the β3 adrenergic receptor with insulin resistance. Obes Res (2005) 13(10):1709–19. doi:10.1038/oby.2005.209
37. Kojima M, Hosoda H, Date Y, Nakazato M, Matsuo H, Kangawa K. Ghrelin is a growth-hormone-releasing acylated peptide from stomach. Nature (1999) 402(6762):656–60. doi:10.1038/45230
38. Malik S, McGlone F, Bedrossian D, Dagher A. Ghrelin modulates brain activity in areas that control appetitive behavior. Cell Metab (2008) 7(5):400–9. doi:10.1016/j.cmet.2008.03.007
39. Imaizumi T, Ando M, Nakatochi M, Yasuda Y, Honda H, Kuwatsuka Y, et al. Effect of dietary energy and polymorphisms in BRAP and GHRL on obesity and metabolic traits. Obes Res Clin Pract (2016) 12(1):39–48. doi:10.1016/j.orcp.2016.05.004
40. Korbonits M, Gueorguiev M, O’Grady E, Lecoeur C, Swan DC, Mein CA, et al. A variation in the ghrelin gene increases weight and decreases insulin secretion in tall, obese children. J Clin Endocrinol Metab (2002) 87(8):4005–8. doi:10.1210/jcem.87.8.8881
41. Kuzuya M, Ando F, Iguchi A, Shimokata H. Preproghrelin Leu72Met variant contributes to overweight in middle-aged men of a Japanese large cohort. Int J Obes (2006) 30(11):1609–14. doi:10.1038/sj.ijo.0803296
42. Takezawa J, Yamada K, Morita A, Aiba N, Watanabe S. Preproghrelin gene polymorphisms in obese Japanese: association with diabetes mellitus in men and with metabolic syndrome parameters in women. Obes Res Clin Pract (2009) 3(4):179–91. doi:10.1016/j.orcp.2009.04.003
43. You Y, Yu Y, Wu Y, Rao W, Zhang Y, Liu Y, et al. Association study between ghrelin gene polymorphism and metabolic syndrome in a Han Chinese population. Clin Lab (2017) 63(1):175–81. doi:10.7754/Clin.Lab.2016.160715
44. Hinney A, Hoch A, Geller F, Schafer H, Siegfried W, Goldschmidt H, et al. Ghrelin gene: identification of missense variants and a frameshift mutation in extremely obese children and adolescents and healthy normal weight students. J Clin Endocrinol Metab (2002) 87(6):2716. doi:10.1210/jcem.87.6.8672
45. Jo DS, Kim SL, Kim SY, Hwang PH, Lee KH, Lee DY. Preproghrelin Leu72Met polymorphism in obese Korean children. J Pediatr Endocrinol Metab (2005) 18(11):1083–6. doi:10.1515/JPEM.2005.18.11.1083
46. Mirrakhimov AE, Kerimkulova AS, Lunegova OS, Moldokeeva CB, Zalesskaya YV, Abilova SS, et al. An association between TRP64ARG polymorphism of the B3 adrenoreceptor gene and some metabolic disturbances. Cardiovasc Diabetol (2011) 10:89. doi:10.1186/1475-2840-10-89
47. Brondani LA, Duarte GC, Canani LH, Crispim D. The presence of at least three alleles of the ADRB3 Trp64Arg (C/T) and UCP1 -3826A/G polymorphisms is associated with protection to overweight/obesity and with higher high-density lipoprotein cholesterol levels in Caucasian-Brazilian patients with type 2 diabetes. Metab Syndr Relat Disord (2014) 12(1):16–24. doi:10.1089/met.2013.0077
48. Cruz M, Valladares-Salgado A, Garcia-Mena J, Ross K, Edwards M, Angeles-Martinez J, et al. Candidate gene association study conditioning on individual ancestry in patients with type 2 diabetes and metabolic syndrome from Mexico City. Diabetes Metab Res Rev (2010) 26(4):261.s–70.s. doi:10.1002/dmrr.1082
49. Liu Y, Wu G, Han L, Zhao K, Qu Y, Xu A, et al. Association of the FABP2 Ala54Thr polymorphism with type 2 diabetes, obesity, and metabolic syndrome: a population-based case-control study and a systematic meta-analysis. Genet Mol Res (2015) 14(1):1155–68. doi:10.4238/2015.February.6.19
50. Vimaleswaran KS, Cavadino A, Berry DJ, Whittaker JC, Power C, Jarvelin MR, et al. Genetic association analysis of vitamin D pathway with obesity traits. Int J Obes (2013) 37(10):1399–406. doi:10.1038/ijo.2013.6
51. Marti A, Corbalan MS, Martinez-Gonzalez MA, Martinez JA. TRP64ARG polymorphism of the beta 3-adrenergic receptor gene and obesity risk: effect modification by a sedentary lifestyle. Diabetes Obes Metab (2002) 4(6):428–30. doi:10.1046/j.1463-1326.2002.00227.x
52. Livingstone KM, Celis-Morales C, Papandonatos GD, Erar B, Florez JC, Jablonski KA, et al. FTO genotype and weight loss: systematic review and meta-analysis of 9563 individual participant data from eight randomised controlled trials. BMJ (2016) 354:i4707. doi:10.1136/bmj.i4707
Keywords: adolescent, Malaysian Health and Adolescents Longitudinal Research Team study, obesity, non-synonymous single-nucleotide polymorphism, physical activity, body fat
Citation: Zaharan NL, Muhamad NH, Jalaludin MY, Su TT, Mohamed Z, Mohamed MNA and Majid HA (2018) Non-Synonymous Single-Nucleotide Polymorphisms and Physical Activity Interactions on Adiposity Parameters in Malaysian Adolescents. Front. Endocrinol. 9:209. doi: 10.3389/fendo.2018.00209
Received: 01 September 2017; Accepted: 13 April 2018;
Published: 27 April 2018
Edited by:
Tarunveer Singh Ahluwalia, Steno Diabetes Center Copenhagen (SDCC), DenmarkReviewed by:
Eusebio Chiefari, Università degli Studi Magna Graecia di Catanzaro, ItalyChristian Theil Have, University of Copenhagen, Denmark
Copyright: © 2018 Zaharan, Muhamad, Jalaludin, Su, Mohamed, Mohamed and Majid. This is an open-access article distributed under the terms of the Creative Commons Attribution License (CC BY). The use, distribution or reproduction in other forums is permitted, provided the original author(s) and the copyright owner are credited and that the original publication in this journal is cited, in accordance with accepted academic practice. No use, distribution or reproduction is permitted which does not comply with these terms.
*Correspondence: Nur Lisa Zaharan, bGlzYUB1bW1jLmVkdS5teQ==, bnVybGlzYXphaGFyYW5AdW0uZWR1Lm15