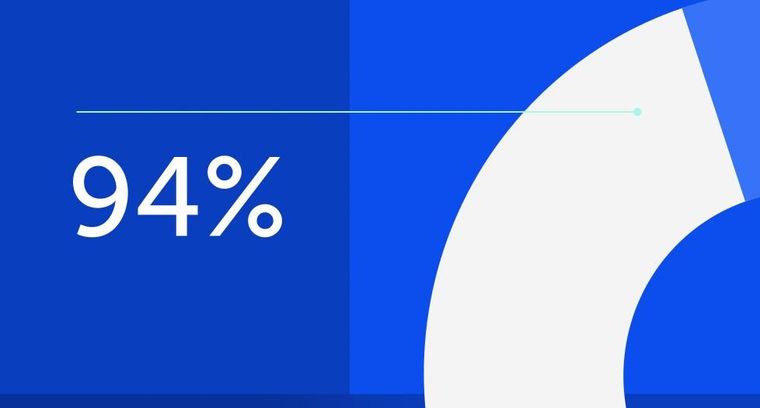
94% of researchers rate our articles as excellent or good
Learn more about the work of our research integrity team to safeguard the quality of each article we publish.
Find out more
ORIGINAL RESEARCH article
Front. Virol., 19 July 2024
Sec. Bioinformatic and Predictive Virology
Volume 4 - 2024 | https://doi.org/10.3389/fviro.2024.1419276
The emergence of SARS-CoV-2 lineages derived from Omicron, including BA.2.86 (nicknamed “Pirola”) and its relative, JN.1, has raised concerns about their potential impact on public and personal health due to numerous novel mutations. Despite this, predicting their implications based solely on mutation counts proves challenging. Empirical evidence of JN.1’s increased immune evasion capacity in relation to previous variants is mixed. To improve predictions beyond what is possible based solely on mutation counts, we conducted extensive in silico analyses on the binding affinity between the RBD of different SARS-CoV-2 variants (Wuhan-Hu-1, BA.1/B.1.1.529, BA.2, XBB.1.5, BA.2.86, and JN.1) and neutralizing antibodies from vaccinated or infected individuals, as well as the human angiotensin-converting enzyme 2 (ACE2) receptor. We observed no statistically significant difference in binding affinity between BA.2.86 or JN.1 and other variants. Therefore, we conclude that the new SARS-CoV-2 variants have no pronounced immune escape or infection capacity compared to previous variants. However, minor reductions in binding affinity for both the antibodies and ACE2 were noted for JN.1. Future research in this area will benefit from increased structural analyses of memory B-cell derived antibodies and should emphasize the importance of choosing appropriate samples for in silico studies to assess protection provided by vaccination and infection. Moreover, the fitness benefits of genomic variation outside of the RBD of BA.2.86 and JN.1 need to be investigated. This research contributes to understanding the BA.2.86 and JN.1 variants’ potential impact on public health.
The continual emergence of SARS-CoV-2 variants remains a challenge for global public health initiatives. Notably, BA.2.86, which shares common ancestry with Omicron (BA.1), was initially identified in late July 2023 in Israel and Denmark (1, 2). Since then, BA.2.86 spread globally (3–5). The initial worldwide spread of BA.2.86 sparked concerns regarding its potential impact on personal and public health. Acknowledging these concerns, the World Health Organization (WHO) designated BA.2.86 as a variant under monitoring on August 17th, 2023 (5).
The genetic makeup of BA.2.86 sets it apart from the original Omicron variant (B.1.1.529) and the XBB.1.5 variant (5), raising concerns due to the numerous mutations in BA.2.86. These concerns are fueled by the observation of the rapid rise of previous variants with a high mutation count, exemplified by the surge in percent case counts of Omicron in late 2021 and early 2022, and XBB.1.5 in early 2023 (6, 7). BA.2.86 is distinguished by 35 mutations from the XBB.1.5 variant (8). Notably, the Spike gene (S) of BA.2.86 exhibits a distinctive profile with 33 mutations in comparison to the original Omicron variant, with 14 of these mutations occurring in the receptor binding domain (RBD) (8). Since antibodies primarily target the viral S protein’s RBD (9), it is considered a region of particular interest in the viral genome. Moreover, mutations in this vital RBD region can affect the efficiency of crucial viral-cell binding events (10), impacting the virus’ ability to bind to the human angiotensin-converting enzyme 2 (ACE2) receptor. For instance, the emergence of novel SARS-CoV-2 variants featuring RBD mutations has been associated with an increased affinity for binding to the ACE2 receptor in XBB.1.5 (10). This heightened RBD and ACE2 affinity has been linked to the accelerated person-to-person transmissibility observed in viruses like Omicron B.1.1.529 and XBB.1.5, leading to their predominance within the population (11). RBD mutations can also facilitate cross-species infections and increase the virus’ zoonotic potential (12). Furthermore, RBD mutations can hinder the efficacy of vaccines that target that region (13, 14).
Despite original concerns about the number of mutations in BA.2.86, and in a departure from past experiences, this variant has exhibited a notably low prevalence in the USA as of February 2024 (15). The failure of BA.2.86 to ascend in variant prevalence indicates that relying solely on mutation counts does not suffice to gauge the severity of a new SARS-CoV-2 variant. More recently, the JN.1 variant, a relative of BA.2.86, emerged in 2023, once again sparking a concern owing to its increased mutation count. JN.1 is characterized by the Leu455Ser mutation in the RBD, which some authors have said makes it “one of the most immunologically evasive variants.” (16). Preliminary evidence indicates that the Leu455Ser mutation diminishes the binding affinity of the ACE2 receptor with JN.1’s RBD, potentially hindering viral entry into host cells while enhancing JN.1’s capacity to evade humoral immunity (17). Furthermore, Leu455Ser may confer greater evasion of JN.1 against the antibodies raised by a monovalent vaccine (18). In addition to JN.1’s mutation within the RBD, it bears three additional distinct mutations outside of the RBD that BA.2.86 lacks (19–21). Despite preliminary indications that RBD mutations would make BA.2.86 or JN.1 more capable than its preceding variants of escaping current vaccines, treatments, or antibodies produced by natural infection, conclusive empirical evidence is lacking and may not be immediately available. Furthermore, recent studies indicate that these new variants may not present pronounced differences in immune escape or infective capabilities when compared to previous variants despite their accumulated mutations. For example, Jeworowski et al. attested that JN.1’s serum neutralization escape did not increase over previously circulating strains despite its stronger increase in worldwide circulation when compared to BA.2.86 (16, 22).
We build upon in silico methodologies established in our previous work (7, 23) to conduct extensive comparative analysis of the binding affinity of JN.1, BA.2.86, and other previous SARS-CoV-2 variant RBDs (Wuhan-Hu-1, BA.1/B.1.1.529, BA.2, and XBB.1.5) to the human ACE2 receptor and neutralizing antibodies from infected individuals and patients vaccinated with different vaccines (including bivalent vaccines). Our findings hold significance for public health and contribute new methods to rapidly address the dynamic challenges posed by the evolving viral variants.
In short, we examined 31 different RBD structures (27 RBD structures were extracted from 26 PDB files. One RBD structure was taken from a previous publication. Three PDB structures were predicted from six different SARS-CoV-2 variant RBDs (Wuhan-Hu-1, BA.1/B.1.1.529, BA.2, XBB.1.5, BA.2.86, and JN.1). Whenever more than one RBD structure was available for a variant, a single representative was selected based the presence of the interfacing residues and the results of docking validation experiments against the corresponding antibody. We cluster together BA.1 and B.1.1.529 in this study as they have identical RBD sequences. We used In silico docking experiments to calculate the binding affinity metrics between the six selected RBD structures and 27 different ligands, including 17 neutralizing antibody structures and ten ACE2 structures, resulting in 162 docking experiments total. All the analyzed structures are available on GitHub (see “Data Availability Statement”). The complete methodology is detailed below.
Given the high infectiousness of the Omicron subvariants and their predominance in the past two years, we selected BA.1/B.1.1.529, BA.2, XBB.1.5, BA.2.86, and JN.1 variants’ RBDs, as well as the original Wuhan-Hu-1 strain (referred to here as “wild type” or WT) for docking. Table 1 summarizes the sources of different RBD structures, as detailed below.
We retrieved a complete genome sequence of BA.2 from the GISAID’s EpiCoV database (41). We annotated and translated the S gene following a similar method to Jacob Machado et al. (42). We used the B.1.1.529 RBD sequence from our group’s initial in silico antibody docking study as a template, following the methodology described in (23). Finally, we extracted a fragment of the corresponding RBD region from the BA.2 sequence (within amino acid residues 336 through 528 in relation to the Wuhan-Hu-1 reference) (43). We obtained the RBD sequences for JN.1 and BA.2.86 from Yang et al., 2023 (17). These amino acid sequences were input to AlphaFold2 (44) via ColabFold using default parameters (45) to predict RBD structures. Colabfold provided a subsequent unrelaxed protein structure, meaning that the side chains within the produced PDB file were in energetically unfavorable and unrealistic conformations. Therefore, we relaxed the produced PDB structures with the Amber relaxation procedure prior to docking (46).
We obtained the XBB.1.5 structure from our group’s previous in silico paper. The XBB.1.5 structure was generated using ColabFold prior to this study (7). We downloaded other available SARS-CoV-2 Spike RBD crystal structures from the Protein Data Bank (PDB) (47). We derived the WT and BA.1/B.1.1.529 RBD structures from the Protein Data Bank as they represent empirically derived structures from an RBD-Antibody or RBD-ACE2 complex (24–30, 37, 40, 48).
For every RBD-antibody and RBD-ACE2 complex we isolated on PDB, we extracted the RBD structure from that complex. We extracted the RBD structure from each complex and subsequently docked that structure against the corresponding Antibody or ACE2 structure to reproduce the initial complex for validation and ensure an accurate docking location.
We expanded the antibody selection compared to previous studies that focused on therapeutic antibodies (7, 23). In this study, we selected 17 antibodies from the PDB, including those that were derived from vaccinated patients, patients vaccinated with breakthrough SARS-CoV-2 infection, and patients who experienced SARS-CoV-2 infection without vaccination. We included two therapeutic antibodies. 13 of the 17 selected antibodies were derived from memory B-cells from human patients (25, 26, 34, 48, 49). The initial complexed RBD was docked to each antibody structure alongside the representative RBD structures we derived from PDB and ColabFold. Antibodies are listed in Table 2.
We used PDB’s ACE2 structures derived from studies analyzing the structure of the ACE2-RBD complex. The ACE2 structures were isolated for docking. We docked the initial complexed RBD to each ACE2 structure alongside the RBDs that we derived from PDB and ColabFold. We selected ACE2 structures with the PDB IDs 6M0J, 7A98, 7DF4, 7YJ3, 8SPI, 7WBl, 7WPB, 8DM6, 7ZF7, and 7X09 (24, 29, 30, 34–40). We chose ten different structures of ACE2 to have an increased sample size with a diversity of minor structural deviations that may affect docking.
To prepare the Fab structures for docking, we renumbered the amino acid residues according to HADDOCK’s (v2.4) requirements such that there were no overlapping residue IDs between the heavy and light chains (50, 51). We selected residues in the Fab structures’ complementarity-determining regions (CDRs) as “active residues”, for docking analysis to assess antibody neutralization. We selected residues in the ACE2 binding pocket forming polar contacts with the RBD in the crystallized structure as “active residues” for docking prediction and analysis of ACE2-RBD binding. We used the same active residues for each ACE2 and antibody structure for each dock to the six RBD structures. We selected residues in the S1 portion of the RBD as active residues. Each RBD has similar active residues when docking against an antibody. However, there are variations in active residue selection to account for differences in amino acid composition between variants. We docked each of the 17 antibody structures and 10 ACE2 structures against six RBD structures using HADDOCK, a biomolecular modeling software that provides docking predictions for provided protein structures (50, 51). Specifically, we used the HADDOCK Web Server (v2.4) for the docking simulations with the default parameters (50, 51). HADDOCK utilizes a linear scoring function to calculate HADDOCK score, which is composed of a weighted sum of multiple calculated variables such as van der Waals energy, electrostatic energy, desolvation energy, and restraints violation energy1, as shown in the equation below. We used the active residues identified in our structure preparation process as inputs into HADDOCK to guide molecular docking.
The HADDOCK software produces multiple output PDB files of docking results and their subsequent docking metrics. We placed the top-scoring PDB output file, after qualitative analysis, to ensure a realistic binding pose for each docking experiment with HADDOCK into the software PRODIGY (v2.1.3) for further analysis. PRODIGY is a web service collection focused on binding affinity predictions for biological complexes (52, 53). PRODIGY uses a model that calculates binding affinity from a weighted sum derived from the number and type of contacts formed between proteins in a complex as shown below.
This process resulted in 162 sets of docked structures. We selected the screened top predicted output structure, per HADDOCK, for each antibody-RBD or ACE2-RBD pair for quantitative analysis after assessing the binding pose. Statistical tests were conducted in R (54), implementing the Kruskall-Wallis and the paired Wilcoxon-Mann-Whitney test to compare the docking metrics produced by HADDOCK and the binding affinity prediction of PRODIGY between variants (55, 56). We also used the selected HADDOCK output structure to visually analyze the structural conformation of interfacing residues and docked proteins using PyMol (v2.5.5) (57). A visual representation of our general methodology is shown in Figure 1.
Figure 1 Generalization of our in silico methodology, illustrating three primary steps: structural generation, molecular docking, and statistical analysis.
We compared docking predictions of viral proteins to antibodies and ACE2 with Kruskall-Wallis and paired Wilcoxon-Mann-Whitney tests. The tested variables were HADDOCK score, van der Waals energy, electrostatic energy, desolvation energy, buried surface area, and PRODIGY’s ΔG predictions. These tests return values that are not statistically significant between JN.1/BA.2.86 and previous Omicron variants at a 95% confidence level for every displayed metric, with a few exceptions, in Figures 2, 3. Tabular representations of the metrics represented in Figures 2, 3 are shown in Supplementary Tables S3, S4 respectively. The difference in Desolvation Energy is statistically significant, via the paired Wilcoxon-Mann-Whitney test, between XBB.1.5 and JN.1 in the antibody comparison, with JN.1 having a lower Desolvation Energy. The difference in desolvation energy between JN.1 and BA.1/B.1.1.529 is statistically significant in the ACE2 comparisons, with BA.1/B.1.1.529 having a lower desolvation energy. In the ACE2 analysis, the Kruskal-Wallis test yields statistically significant differences between all groups.
Figure 2 Boxplots illustrating the comparative docking scores of ACE2 and RBD structures generated by HADDOCK and PRODIGY. Each boxplot highlights the distribution of docking scores for different variants. Pairwise comparisons were performed using the Wilcoxon-Mann-Whitney signed-rank test, indicated by the horizontal lines. The Kruskal-Wallis test was used to compare the independent samples’ medians, indicated in the bottom left.
The Wuhan or WT variant exhibited a higher binding affinity, which is statistically significant, to ACE2 than the BA.2.86 and JN.1 variants when assessing ΔG value in Figure 3. The WT variant also exhibited a lower desolvation energy score when binding to ACE2, which is statistically significant, than the BA.2.86 and JN.1 variants. The WT variant exhibited a higher, statistically significant, electrostatic energy score than BA.2.86.
The Kruskal-Wallis test, which assesses statistical significance for group comparisons as a whole, returns a statistically significant p value at a 95% confidence interval in the ACE2 comparisons for Desolvation Energy in Figure 3.
We use the PRODIGY ΔG score as the primary metric to measure binding affinity. Thus, we conclude that all the Omicron subvariants’ (including BA.2.86 and JN.1) performance for the ACE2 and antibody docking simulations were similar. Slight reductions in antibody-RBD and ACE2-RBD binding affinity were observed for JN.1 when compared to BA.2.86, but they are not statistically significant at a p-value of 0.81 and 0.22, respectively, for a 95% confidence interval.
Figure 2 illustrates various metrics produced by HADDOCK and PRODIGY estimations of the protein-to-protein binding affinities between our aggregate antibody arsenal and RBD structures. It includes 17 antibody structures and six variant RBD structures (102 experiments in total). The results of ACE2 to RBD docking experiments are shown in Figure 3, including 10 ACE2 and six RBD structures (60 docking experiments in total). Figures 2, 3 also show the non-significative p-values of the Kruskal-Wallis statistical test in the bottom left of each plot and the Wilcoxon-Mann-Whitney signed-rank test between each RBD, again with the exceptions mentioned above. These figures are derived from metrics obtained from the best PDB complex structure, determined by HADDOCK, for each experiment.
Figure 4 shows the structural analysis of the interfacing residues between the RBDs of JN.1 and BA.2.86 with antibodies. The only difference in the RBD in JN.1 and BA.2.86 is the Leu455Ser substitution in JN.1. The residue at position 455 does not form polar contacts in either the JN.1-RBD or BA.2.86-RBD complexes in Figures 4A, B, nor is the residue at position 455 near the docking interface for either complex. The Leucine at position 455, in the RBD of BA.2.86, may interact with residues within the RBD. The substitution of Leucine for Serine at position 455 may alter intramolecular interactions of the RBD, inherently affecting its tertiary structure. This disparity in structure may affect the binding pose of the RBD in complex with the antibody, which is highly dependent on the antibody mechanism. In Figure 4A, one can note that BA.2.86 generally forms polar contacts with residues that do not propagate steric hindrance. In contrast, Figure 4B shows that JN.1 forms two Histidine polar contacts, a side chain that introduces more steric hindrance and rigidity. Histidine contacts may reduce proximal residue interdigitation, providing a lower binding affinity score. Whereas a less sterically hindered side chain that maintains a polar contact, such as Lysine in its place, may increase interdigitation and binding. In Figure 4C, the Phenylalanine at position 489 of BA.2.86’s RBD interdigitates well with antibody residues, potentially increasing nonpolar interactions and decreasing the effect of the steric hindrance from the bulky Phenylalanine side chain. In Figure 4D, one can notice that residues appear less interdigitated than in Figure 4C. This may be due to the destabilizing effect of the Serine at position 455 in JN.1, in addition to the alteration of the RBD tertiary structure mentioned previously. Leucine455 is shown in yellow in Figure 4C, close to Isoleucine100 of the antibody. Both of these residues may form nonpolar interactions, strengthening binding. In Figure 4D, this Serine substitution has caused a lack of attraction between the two residues, potentially causing the segment within the RBD to retract back in docking and decrease binding affinity.
Figure 3 Boxplots illustrating the comparative docking scores of ACE2 and RBD structures generated by HADDOCK and PRODIGY. Each boxplot highlights the distribution of docking scores for different variants. Pairwise comparisons were performed using the Wilcoxon-Mann-Whitney signed-rank test, indicated by the horizontal lines. The Kruskal-Wallis test was used to compare the independent samples’ medians, indicated in the bottom left.
Figure 4 (A, B) display the output PDB files from HADDOCK of the docking jobs of antibody P2D9 to BA.2.86 and JN.1, respectively. (C, D) display the output PDB files from HADDOCK of the docking jobs of antibody 10-5B to BA.2.86 and JN.1, respectively. The antibody structure in each is shown on the right in green, and the RBD is shown on the left in magenta for BA.2.86 or greencyan for JN.1. Interacting RBD residues are labeled in yellow. The yellow dashed lines represent the polar contacts formed between residues within 3.0Å. Residues discussed in the results section on the antibody are labeled in blue. Important mutations referenced in the results section are highlighted in yellow and labeled in red.
Figure 5 shows the structural analysis of the interfacing residues between the RBDs of JN.1, BA.2.86, and XBB.1.5 with ACE2. When assessing Figures 5A, B, one can notice that XBB.1.5 and JN.1 adopt a markedly different binding pose to each other; the angle of binding has approximately a 90-degree disparity. However, they do maintain similar interfacing residues. First, it is to note that at position 445, XBB.1.5 has a Proline residue while JN.1 has a Histidine residue. Proline offers enhanced rigidity to that of Histidine, potentially adding to the disparity in tertiary structure between XBB.1.5 and JN.1, affecting binding. Second, there is a deletion in the JN.1 RBD at position 483, where there is a Valine in position 483 in XBB.1.5. This Valine does not interface with ACE2. However, this deletion may lead to an alteration in tertiary structure that causes a variation in binding pose. Lastly, there is a Serine at position 490 in XBB.1.5, while there is a Phenylalanine in the corresponding position of 489 in JN.1. When assessing XBB.1.5, this Serine may form contacts in slight alterations of the docking pose with the Threonine27, Lysine31, and Glutamate35 in ACE2. These contacts could potentially increase stability. A future mutation of the Serine to an Aspartate or Glutamate may provide a more stable interaction with ACE2. When assessing the corresponding structure of JN.1, the Phenylalanine is proximal to Histidine34 of ACE2. This proximity may increase pi-pi bonding. However, given the constraints of the Alpha Helix that Histidine34 is part of, there is limited flexibility, and steric hindrance between the two bulky side chains could cause detrimental effects on binding. When assessing Figures 5C, D, one can notice a minimal difference in binding pose between BA.2.86 and JN.1. The only difference between the sequence of BA.2.86 and JN.1 is the residue at position 455. In JN.1, the residue at position 455 is a Leucine. This Leucine is close in proximity to Isoleucine100 and Phenylalanine72 in ACE2. This proximity could add to nonpolar interactions, increasing binding affinity. Future mutation of this residue to a Phenylalanine may provide pi-pi and further van der Waals interaction to enhance ACE2 affinity. When assessing the Serine in position 455 in JN.1, it is positioned far away from the ACE2-RBD interface, leaving it unlikely to interact with any ACE2 residue to strengthen interaction significantly. There is a Lysine at position 68 in ACE2, which may form an interaction with the electronegative oxygen within Serine. However, Lysine68 is part of an alpha-helix of ACE2 and is angled away from Serine455 of the RBD, making the interaction less likely.
Figure 5 (A, B) display the output PDB files from HADDOCK of the docking jobs of the ACE2 structure from PDB 7XO9 and XBB.1.5 and JN.1, respectively. (C, D) display the output PDB files from HADDOCK of the docking jobs between the ACE2 structure from PDB 7WPB and BA.2.86 and JN.1, respectively. In each, the images show the ACE2 structure on the right in blue and the RBD on the left, colored in yellow for XBB.1.5, magenta for BA.2.86, and greencyan for JN.1. Interacting ACE2 residues, referenced in the results section, are labeled in blue. The red dashed lines represent the polar contacts formed between residues within 3.0Å. Important mutations referenced in the results section are labeled in red and highlighted in red.
The antibody P2D9, identified in Luo et al. (26), was obtained from the memory B cells of individuals vaccinated with the BNT162b2-vaccine (26). These individuals also had breakthrough BA.1 infection (26). P2D9 was found to neutralize all tested variants of concern and omicron sublineages (26). Luo et al. tested neutralization capabilities against variants such as the WT, Alpha, Delta, BA.1, and BA.2 variants (26). Pseudovirus neutralization profiles were determined for P2D9. Luo et al. found on the BA.1, BA.2. and the WT variant that P2D9 had IC50 values of 0.0117, 0.1381, and 0.0075 µg/mL, respectively (26). When assessing the values in Supplementary Table S5, one can notice similar values for Van der Waals energy and HADDOCK score between the three variants. However, the WT variant has a significantly lower Prodigy ΔG value, correlating with the empirical values. It is noted that BA.2 records a lower Prodigy ΔG value than the BA.1 Prodigy ΔG value. However, BA.1 Electrostatic Energy value is substantially lower than that of BA.2 potentially explaining the discrepancy between their IC50 values despite their Prodigy ΔG values. The WT variant has an Electrostatic Energy value between BA.1 and BA.2. The metrics in Supplementary Table S5 indicate P2D9 will perform similarly to previous Omicron variants on BA.2.86 and JN.1, maintaining its broadly neutralizing characteristic.
The antibody 6-2C, identified in Liu et al. (25), was obtained from the memory B-cells of individuals vaccinated with the BBIBP-CorV inactivated vaccine (25). Specifically, Liu et al. obtained samples from patients who had robust humoral immune responses after the second dose of the BBIBP-CorV vaccine (25). When assessing Supplementary Table S6, one can notice that the WT variant has lower values for Van der Waals energy, HADDOCK score, and Prodigy ΔG relative to the BA.1 and BA.2 variant. Electrostatic energy is slightly higher between 6-2C and the WT variant. Overall, these metrics indicate that 6-2C has a higher binding affinity to the WT variant relative to BA.1 and BA.2 variants. When assessing empirical data reported in Liu et al. (25), the minimum amount of antibody quantity to achieve 50% reduction in viral infectivity for the WT, BA.1, and BA.2 variants is 37, 1149, and 948 ng/mL respectively (25). One can note that 62-C is far more potent on the WT variant than BA.1 and BA.2 variants. The empirical values correlate with the obtained docking metrics and post-docking metrics. When assessing the data for BA.2.86 and JN.1 in Supplementary Table S6, the metrics indicate that 6-2C will maintain neutralization capabilities for BA.2.86 and JN.1.
The antibody CV2.1169, identified in Planchais et al. (31), was obtained from the memory B-cells of individuals infected with the WT strain. Planchais et al. specifically filtered for serum samples from individuals with high seroneutralization for single B-cell antibody cloning. Planchais et al. found that the antibody CV2.1169 potently neutralized SARS-CoV-2 variants of concern. Planchais et al. concluded that CV2.1169 was a prime candidate for the prevention and treatment of COVID-19. When analyzing the Planchais et al. (31) study, IC50 values for BA.1 and BA.2 are 850 and 756 pM, respectively, corresponding with the Prodigy ΔG values displayed in Supplementary Table S7, with BA.2 demonstrating a lower Prodigy ΔG, lower electrostatic energy score, and lower HADDOCK score. The data in Supplementary Table S7 indicates CV2.1169 will maintain its neutralizing capabilities as the various docking and affinity metrics for BA.2.86 and JN.1 are similar to BA.1 and BA.2.
The docking metrics we obtained tell a complex story when assessing them as a single component to form conclusions regarding the binding affinity of BA.2.86 and JN.1 to neutralizing antibodies and ACE2. Statistically significant Desolvation Energy differences are noted in the results. Desolvation Energy measures the energy change of protein atoms moving from interactions with a solvent to a non-solvent (58). Desolvation Energy can significantly impact binding affinity and is a component in the calculation of binding affinity (59). Taken alone, desolvation energy metrics may indicate lower or higher binding between two separate molecules within a solvent. However, we have the binding affinity metrics provided by PRODIGY, which do not indicate a statistically significant difference in binding affinity for neutralizing antibodies and ACE2 between JN.1 and BA.2.86 relative to previous Omicron variants. It is an interesting question for further research whether a small decrease in binding affinity to neutralizing antibodies for JN.1 may lead to significant evasion of antibodies for JN.1. In the context of our current docking metrics that compare JN.1 to XBB.1.5 and BA.1/B.1.1.529, we conclude that there is not significant immune evasion by JN.1 relative to those variants. Significant changes are not observed between JN.1 and BA.2.86 to neutralizing antibodies and ACE2. BA.2.86 appears to have no significant evasion to RBD targeting antibodies and does not appear to bind with a significantly higher affinity to ACE2 than previous variants. Relative to BA.2.86, JN.1 does demonstrate a 3.9% increase in the median ΔG value to the RBD-targeting antibody arsenal in our study, indicating slightly increased antibody evasion. At the same time, JN.1 exhibits a lower ACE2 binding affinity than BA.2.86, with a 4.9% increase for the median ΔG value for JN.1 relative to BA.2.86. JN.1’s observed increase in predominance over BA.2.86 does not appear to be dependent on enhancement in ACE2 affinity. Cell entry capability due to ACE2 affinity is predicted to be lower than that observed in our predictions for BA.2.86, likely due to the substitution of the Leucine at position 455 for Serine in JN.1.
The docking experiment metadata includes the number of clusters calculated by HADDOCK. The number of clusters is inversely correlated with the certainty and stability of docked complexes. Our HADDOCK docking process most often results in a low number of clusters. This indicates stability in the docking location and conformation. As shown in Supplementary Figure S5 below, 72% of the antibody docking experiments resulted in 2 or fewer clusters (73/102) and 80% of the ACE2 docking experiments resulted in 2 or fewer clusters (48/60). Within a given cluster, there were often a large proportion of the docked complexes (out of the 200 possible) that clustered together. For example, many of the experiments had clusters of 100+ complexes (out of the 200 possible from the water refinement step in HADDOCK) that clustered together in the first cluster, indicating a high stability and confidence in the docking.
Studies have been published regarding BA.2.86 and JN.1. In one study titled, “Sensitivity of BA.2.86 to prevailing neutralizing antibody responses,” patients had blood sera collected before and after XBB.1.5 predominance (60). Sheward et al. found that blood samples collected before and after XBB.1.5 prevalence had moderately lower geometric mean neutralizing titers for BA.2.86 relative to XBB.1.5. Sheward et al. concluded that BA.2.86 did not appear to have the same level of relative immune escape as when the Omicron variant initially emerged in the shadow of the Delta variant in late 2021. The in silico results for BA.2.86 correspond with Sheward et al. (60).
In an article published titled ‘SARS-CoV-2 Omicron subvariant BA.2.86: limited potential for global spread’, Wang et al. review numerous studies regarding BA.2.86 including Sheward et al. (60). Wang et al. analyze multiple studies that show that recent infection or vaccination increases neutralization of BA.2.86. Wang et al. generally conclude that BA.2.86 may not possess the transmissibility that previous Omicron strains possessed. Wang et al.’s analysis corresponds with the in silico results for BA.2.86. Wang et al. do note that their conclusion does not apply to the JN.1 variant as they did not examine it (61).
In an article titled ‘Humoral immune escape by current SARS-CoV-2 variants BA.2.86 and JN.1, December 2023’, Jeworowski et al. collected sera from a group of individuals who had received three to four vaccine doses in September 2023, a majority having prior breakthrough infection (22). These individuals were initially vaccinated using mRNA or vector-based vaccinations (22). Jeworowski et al. found that JN.1 did not exhibit a significant reduction in serum neutralization titers relative to BA.2.86 (22). It is to be noted that all of the individuals in the study reported a SARS-CoV-2 infection during the period of Omicron prevalence (22). Jeworowski et al. concluded that antibody evasion did not appear to cause the increased prevalence of JN.1. Jeworowski et al. (22)’s results correspond with the results of our study.
In another study on JN.1 titled “Fast evolution of SARS-CoV-2 BA.2.86 to JN.1 under heavy immune pressure’, Yang et al. studied the humoral immune evasion and ACE2 binding affinity of JN.1 (17). Yang et al. collected blood samples from patients who were reinfected with XBB post BA.5 or BA.7 breakthrough infection (17). Yang et al. also collected samples from individuals who had received three doses of inactivated vaccines and subsequently contracted XBB (17). Yang et al. used a blood serum neutralization titer method to assess antibody neutralization. Yang et al. found that JN.1 had significantly higher antibody evasion than BA.2.86 but had a notably lower ACE2 binding affinity than BA.2.86 (17). Yang et al.’s trends generally correspond with our results. However, the magnitude to which we observe antibody evasion is distinct (17). We do not note a significant increase, which Yang et al. note, in antibody evasion relative to previous strains, there is a noted decrease in ACE2 binding, as there is a reduction in binding affinity for JN.1 relative to BA.2.86.
We have correctly analyzed relative immune evasion for RBD targeting antibodies for the BA.1/B.1.1.529, XBB.1.5, and BA.2.86 variants with the in silico approach in the past (7, 23, 62, 63). When assessing the CDC Data Tracker, at the time of writing, JN.1 accounts for approximately 96.4% of current variants detected (15). Our approach, in which we focus on RBD-antibody and RBD-ACE2 interaction, does not explain the relative predominance of JN.1 to BA.2.86.
Immune evasion and transmissibility are not exclusively dictated by mutations in the RBD region. In the case of JN.1, several mutations of interest are present in nonstructural proteins that may play a role in immune evasion (13, 14, 64). For instance, mutations in nsp6 have been identified as capable of inhibiting the interferon type I (IFN-I) pathway of the host cell (64). This inhibition leads to the absence of IFNA and IFNB production, crucial components in the defense against viral infections (65, 66).
In our tabulation of mutations S8, JN.1 contains mutations, lacking in BA.2.86, in the proteins nsp3 and nsp6. JN.1 also contains a mutation, lacking in BA.2.86, within ORF7b, a gene whose function is not well studied. Nsp3 is associated with binding to host proteins and plays a role in viral replication (20, 21, 67).
This study, alongside our previous study involving XBB.1.5, demonstrates a decline in structural and electrostatic change within the RBD to evade vaccine, infection, and therapeutically derived antibodies (7). The predominant variants of the last two years maintain similar antibody evasion potential, marking a stark contrast to the structural and electrostatic divergence in the RBD that was introduced with the B.1.1.529 variant, which was highly evasive of existing antibodies, causing significant increases in hospitalizations and deaths (7, 15, 23). The results of our in silico analysis alongside traditional empirical analysis demonstrate that antibody evasion may not be the source of the relative increase in transmissibility demonstrated by JN.1 over BA.2.86 (22). Increased affinity to the ACE2 receptor is the direction of evolutionary pressure for the RBD of the Spike Protein. Antibody defenses derived from memory B-cells, via breakthrough infection and vaccination, have been studied to be increasingly more capable of binding the RBD of a broad amount of SARS-CoV-2 variants, which make their aggregate electrostatic and steric interactions increasingly similar to that of ACE2 (68). Therefore, since SARS-CoV-2 faces evolutionary pressure to evolve towards a highly conserved target, as ACE2 maintains conservation and a slow evolutionary rate, it is improbable that the RBD will evolve around increasingly strong antibody defenses without reducing ACE2 affinity, which would inherently reduce its transmissibility (69). We noted this very phenomenon with JN.1 relative to BA.2.86, as JN.1 has slightly increased antibody evasion, but it also has decreased ACE2 affinity. This substantial increase in predominance for JN.1 over BA.2.86, alongside our results and existing empirical results, implies that evolution outside of the RBD is enhancing relative SARS-CoV-2 variant transmissibility (22). Unfortunately, precise quantification of the impact of mutations outside the Spike protein contributing to JN.1’s increased immune escape compared to other variants remains challenging (17). An avenue for future study will be to assess disparities in transmissibility of point mutated non-RBD genes to quantify how mutations outside of the RBD can influence transmissibility. Future vaccine development would be wise to consider epitopes outside of the RBD. It will be important to assess areas of high homology between variants and build on previous work to develop multi-epitope vaccines that can be effective at neutralizing variants of the future (70). In addition to vaccine development, future work can build upon existing computational work to assess drug inhibition potential against conserved targets such as the main protease and how drug resistance may occur through point mutations outside of the RBD (71, 72).
We acknowledge that our in silico methodology obtains docking and binding affinity metrics from a single complex, and that may limit individual assessment of antibodies and slightly bias individual docking data. We exclude explicit molecular dynamics simulations, potentially biasing our data. This potential bias, however, may be limited as we do not derive our conclusions from the docking and binding metrics of one complex but the metrics of dozens of complexes to the studied variants. We acknowledge that we cannot, as of yet, assess the interaction of multiple antibodies with the spike protein in neutralization interactions. However, a significant benefit to this approach is the ability to study the neutralization capabilities of individual antibodies, not the aggregate capabilities of antibodies prevalent in the blood. The antibody selection significantly differs from the antibodies studied in existing serological studies, which may be biased by quantities of prevalent antibodies, not the quality of such antibodies. The studied antibody array primarily consists of memory B-cell derived antibodies that are studied to be broadly neutralizing against different variants (25, 26, 31, 34, 48, 49). Memory B-cell-derived antibodies created in response to an antigen are not as easily observable when using blood serum neutralization titers. The in silico results support the empirical results that the selected antibody array, primarily consisting of broadly neutralizing antibodies, maintains its efficacy across different variants (25, 26, 31, 34, 48, 49). The presence of such broadly neutralizing antibodies also may indicate a level of prevalence of memory B-cells within vaccinated or infected individuals that differentiate to produce such antibodies (25, 31, 34, 48, 49, 73, 74). Serum antibody neutralization titers may be accurate in predicting the prevention of initial infection but are not as accurate in regards to the prediction of the prevention of serious disease. This is because serum antibody neutralization titers only account for antibodies prevalent in the blood at the time of collection. The secondary immune response, via the production of antibodies derived from memory B-cells, cannot easily be measured using this method. Neutralization titers, using blood samples, is a biased measurement method as the proportions of antibodies within the bloodstream will reflect specific neutralizing capabilities against recently introduced antigens from infection or vaccination. For example, in Yang et al., 2023, the authors specifically assess sera collected after XBB infection, biasing the proportions of antibodies within the blood that have neutralizing capabilities towards epitopes of XBB and structurally similar variants. When an immune response occurs to an introduced SARS-CoV-2 antigen in an individual with memory B-cells that can respond to that antigen, the proportions of neutralizing antibodies can and will change as the memory B-cells will differentiate to produce plasma cells to create neutralizing antibodies (73). In contrast to the limitation of a bias in the proportion of neutralizing antibodies provided in serum neutralization studies, this in silico method primarily uses antibodies that have been studied to be produced upon memory B-cell stimulation and neutralize SARS-CoV-2 variants, which may be more indicative of protection induced by prior infection or vaccination. A larger and broader study analyzing the presence of memory B-cells that differentiate to eventually produce broadly neutralizing antibodies is needed to support the notion that the general population can produce such broadly neutralizing and effective antibodies against current and future variants. In addition to the study of the natural production of broadly neutralizing antibodies, future work into developing therapeutic antibodies based upon the highly capable antibodies produced by the memory B-cells used in the study could lead to improved treatment outcomes.
Our study indicates that ACE2 and antibody binding of the BA.2.86 and JN.1 variants is not significantly different from previous variants. Moreover, our study shows the ongoing efficacy of antibodies induced by various means in the global population to fight BA.2.86 and JN.1. Finally, the in silico results suggest that variation within the RBD has not led to the increased fitness JN.1 has demonstrated relative to BA.2.86. Mutations outside the RBD that may enhance the fitness of JN.1 must be studied to enhance our ability to respond to the ongoing SARS-CoV-2 pandemic. As we have shown that mutation counts in the RBD do not necessarily imply immune evasion, we conclude that the field of genomic epidemiology must open a new chapter with a functional perspective above and beyond the mission of sequencing novel variants.
The original contributions presented in the study are publicly available. This data can be found here: https://github.com/colbyford/SARS-CoV-2_BA.2.86_Spike-RBD_Predictions.
SY: Conceptualization, Data curation, Formal analysis, Investigation, Methodology, Software, Supervision, Validation, Visualization, Writing – original draft, Writing – review & editing. SG: Data curation, Formal analysis, Investigation, Methodology, Software, Validation, Visualization, Writing – original draft, Writing – review & editing. DM: Data curation, Formal analysis, Investigation, Project administration, Supervision, Validation, Visualization, Writing – original draft, Writing – review & editing. CF: Conceptualization, Data curation, Formal analysis, Investigation, Methodology, Project administration, Software, Supervision, Validation, Visualization, Writing – original draft, Writing – review & editing. DJ: Conceptualization, Funding acquisition, Investigation, Project administration, Resources, Software, Supervision, Writing – original draft, Writing – review & editing.
The author(s) declare that no financial support was received for the research, authorship, and/or publication of this article.
The FP7 WeNMR (project # 261572), H2020 West-Life (project # 675858), the EOSC-hub (project # 777536) and the EGI-ACE (project # 101017567) European e-Infrastructure projects are acknowledged for the use of their web portals, which make use of the EGI infrastructure with the dedicated support of CESNET-MCC, INFN-LNL-2, NCG-INGRID-PT, TW-NCHC, CESGA, IFCA-LCG2, UA-BITP, TR-FC-1ULAKBIM, CSTCLOUD-EGI, IN2P3-CPPM, CIRMMP, SURFsara and NIKHEF, and the additional support of the national GRID Initiatives of Belgium, France, Italy, Germany, the Netherlands, Poland, Portugal, Spain, UK, Taiwan and the US Open Science Grid. We acknowledge the Statens Serum Institut Bioinformatics and Microbial Genomics for the upload of the BA.2 genome sequence on GISAID. We acknowledge the following entities at the University of North Carolina at Charlotte: Academic Affairs, The Office of Research, The Center for Computational Intelligence to Predict Health and Environmental Risks (CIPHER), The Department of Bioinformatics and Genomics, The College of Computing and Informatics, and the University Research Computing group. We acknowledge the support of the Belk Family.
Author CTF is the owner of Tuple, LLC, a biotechnology consulting firm.
The remaining authors declare that the research was conducted in the absence of any commercial or financial relationships that could be construed as a potential conflict of interest.
All claims expressed in this article are solely those of the authors and do not necessarily represent those of their affiliated organizations, or those of the publisher, the editors and the reviewers. Any product that may be evaluated in this article, or claim that may be made by its manufacturer, is not guaranteed or endorsed by the publisher.
The Supplementary Material for this article can be found online at: https://www.frontiersin.org/articles/10.3389/fviro.2024.1419276/full#supplementary-material
Supplementary Table 3 | A tabular representation of the median binding prediction metrics for the studied SARS-CoV-2 variants against the studied neutralizing antibody arsenal.
Supplementary Table 4 | A tabular representation of the median binding prediction metrics for the studied SARS-CoV-2 variants against the ACE2 receptor.
Supplementary Table 5 | Individual docking metrics for the antibody P2D9 against the different variants used in the study.
Supplementary Table 6 | Individual docking metrics for the antibody 6-2C against the different variants used in the study.
Supplementary Table 7 | Individual docking metrics for the antibody CV2.1169 against the different variants used in the study.
Supplementary Table 8 | The table above displays the mutations for JN.1 and BA.2.86 relative to the B.1.1.529 variant. It shows the mutation location, the mutated amino acid present, the gene that the mutation is within, and the function of the gene. The mutation data is derived from the Outbreak.info lineage comparison tool and the UCSC Genome Browser (20, 21).
Supplementary Figure 5 | Barcharts representing the distribution of the quantities of HADDOCK clusters from the experiments in this study. Scatterplots show the number of protein complexes (out of 200 possible) that clustered in each quantity of clusters. Experiments with a large proportion of complexes that clustered into clusters 1 or 2 indicate stability in the docking predictions.
1. UK Health Security Agency. Risk assessment for SARS-CoV-2 variant V-23AUG-01 (BA.2.86)(2023). Available online at: https://www.gov.uk/government/publications/investigation-of-sars-cov-2-variants-of-concern-variant-risk-assessments/risk-assessment-for-sars-cov-2-variant-v-23aug-01-or-ba286 (Accessed August 23, 2023).
2. Centers for Disease Control and Prevention. Risk Assessment Summary for SARS CoV2 Sublineage BA.2.86(2023). Available online at: https://www.cdc.gov/respiratory-viruses/whats-new/covid-19-variant.html (Accessed November 1, 2023).
3. Kuchipudi SV. Why public health experts are concerned about BA.2.86, the latest COVID-19 variant(2023). Available online at: https://www.pbs.org/newshour/health/why-public-health-experts-are-concerned-about-ba-2-86-the-latest-covid-19-variant (Accessed November 2, 2023).
4. Centers for Disease Control and Prevention. Update on SARS CoV-2 Variant BA.2.86(2023). Available online at: https://www.cdc.gov/respiratory-viruses/whats-new/covid-19-variant-update-2023-08-30.html (Accessed August 10, 2023).
5. World Health Organization. Tracking SARS-CoV-2 variants(2023). Available online at: https://www.who.int/activities/tracking-SARS-CoV-2-variants/ (Accessed November 1, 2023).
6. Callaway E. Coronavirus variant XBB.1.5 rises in the United States—is it a global threat? Nature. (2023) 613:222–3. doi: 10.1038/d41586-023-00014-3
7. Ford CT, Yasa S, Machado DJ, White RA, Janies DA. Predicting changes in neutralizing antibody activity for SARS-CoV-2 XBB.1.5 using in silico protein modeling. Front Virol. (2023) 3:1172027. doi: 10.3389/fviro.2023.1172027
8. Yang S, Yu Y, Jian F, Song W, Yisimayi A, Chen X, et al. Antigenicity and infectivity characterisation of SARS-CoV-2 BA.2.86. Lancet Infect Dis. (2023) 23:639–44. doi: 10.1016/S1473-3099(23)00573-X
9. Park A. Will the New COVID-19 Vaccine Work Against the BA.2.86 Variant?(2023). Available online at: https://time.com/6308418/ba-2-86-covid-19-variant-vaccine/.
10. Devaux CA, Fantini J. ACE2 receptor polymorphism in humans and animals increases the risk of the emergence of SARS-CoV-2 variants during repeated intraand inter-species host-switching of the virus. Front Microbiol. (2023) 14. doi: 10.3389/fmicb.2023.1199561
11. Yue C, Song W, Wang L, Jian F, Chen X, Gao F, et al. ACE2 binding and antibody evasion in enhanced transmissibility of XBB.1.5. Lancet Infect Dis. (2023) 23. doi: 10.1016/S1473-3099(23)00010-5
12. Bate N, Savva CG, Moody PCE, Brown EA, Evans SE, Ball JK, et al. In vitro evolution predicts emerging SARS-CoV-2 mutations with high affinity for ACE2 and cross-species binding. Public Library Sci Pathog. (2022) 18. doi: 10.1371/journal.ppat.1010733
13. Dai L, Zheng T, Xu K, Han Y, Xu L, Huang E, et al. A universal design of betacoronavirus vaccines against covid-19, mers, and sars. Cell. (2020) 182:722–33. doi: 10.1016/j.cell.2020.06.035
14. Yang J, Wang W, Chen Z, Lu S, Yang F, Bi Z, et al. A vaccine targeting the rbd of the s protein of sars-cov-2 induces protective immunity. Nature. (2020) 586:572–7. doi: 10.1038/s41586-020-2599-8
15. Centers of Disease Control and Prevention. COVID data tracker(2023). Available online at: https://covid.cdc.gov/covid-data-tracker/variant-proportions (Accessed February 17, 2024).
16. Wang X, Lu L, Jiang S. SARS-CoV-2 evolution from the BA. 2.86 to JN. 1 variants: unexpected consequences. Trends Immunol. (2024) 45. doi: 10.1016/j.it.2024.01.003
17. Yang S, Yu Y, Xu Y, Jian F, Song W, Yisimayi A, et al. Fast evolution of SARS-COV-2 BA.2.86 to JN.1 under heavy immune pressure. Lancet Infect Dis. (2023) 24:E70–2. doi: 10.1016/S1473-3099(23)00744-2
18. Kaku Y, Okumura K, Padilla-Blanco M, Kosugi Y, Uriu K, Hinay AA, et al. Virological characteristics of the SARS-CoV-2 JN. 1 variant. Lancet Infect Dis. (2024) 24. doi: 10.1016/S1473-3099(23)00813-7
19. Fan H, Qin S, Cui Y. Emergence and characterization of the SARS-CoV-2 JN. 1 variant: global prevalence and implications for public health. Zoonoses. (2024) 4:994. doi: 10.15212/ZOONOSES-2023-0061
20. Kent WJ, Sugnet CW, Furey TS, Roskin KM, Pringle TH, Zahler AM, et al. The human genome browser at UCSC. Genome Res. (2002) 12:996–1006. doi: 10.1101/gr.229102
21. Gangavarapu K, Latif AA, Mullen JL, Alkuzweny M, Hufbauer E, Tsueng G, et al. Outbreak. info genomic reports: scalable and dynamic surveillance of SARS-CoV-2 variants and mutations. Nat Methods. (2023) 20:512–22. doi: 10.1038/s41592-023-01769-3
22. Jeworowski LM, Mühlemann B, Walper F, Schmidt ML, Jansen J, Krumbholz A, et al. Humoral immune escape by current SARS-CoV-2 variants BA.2.86 and JN.1, December 2023. Eurosurveillance. (2024) 29:2300740. doi: 10.2807/1560-7917.ES.2024.29.2.2300740
23. Ford CT, MaChado DJ, Janies DA. Predictions of the SARS-CoV-2 Omicron variant (B.1.1.529) spike protein receptor-binding domain structure and neutralizing antibody interactions. Front Virol. (2022) 2:830202. doi: 10.3389/fviro.2022.830202
24. Lan J, Ge J, Yu J, Shan S, Zhou H, Fan S, et al. Structure of the SARS-CoV-2 spike receptor-binding domain bound to the ACE2 receptor. Nature. (2020) 581:215–20. doi: 10.1038/s41586-020-2180-5
25. Liu Y, Wang Z, Zhuang X, Zhang S, Chen Z, Zou Y, et al. Inactivated vaccine-elicited potent antibodies can broadly neutralize SARS-CoV-2 circulating variants. Nat Commun. (2023) 14:2179. doi: 10.1038/s41467-023-37926-7
26. Luo M, Zhou B, Reddem ER, Tang B, Chen B, Zhou R, et al. Structural insights into broadly neutralizing antibodies elicited by hybrid immunity against SARS-CoV-2. Emerg Microbes Infect. (2023) 12:2146538. doi: 10.1080/22221751.2022.2146538
27. Barnes CO, Jette CA, Abernathy ME, Dam K-MA, Esswein SR, Gristick HB, et al. SARS-CoV-2 neutralizing antibody structures inform therapeutic strategies. Nature. (2020) 588:682–7. doi: 10.1038/s41586-020-2852-1
28. Barnes CO, West AP Jr., Huey-Tubman KE, Hoffmann MAG, Sharaf NG, Hoffman PR, et al. Structures of human antibodies bound to SARS-CoV-2 spike reveal common epitopes and recurrent features of antibodies. Cell. (2020) 182:828–842.e16. doi: 10.1016/j.cell.2020.06.025
29. Benton DJ, Wrobel AG, Xu P, Roustan C, Martin SR, Rosenthal PB, et al. Receptor binding and priming of the spike protein of SARS-CoV-2 for membrane fusion. Nature. (2020) 588:327–30. doi: 10.1038/s41586-020-2772-0
30. Xu C, Wang Y, Liu C, Zhang C, Han W, Hong X, et al. Conformational dynamics of SARS-CoV-2 trimeric spike glycoprotein in complex with receptor ACE2 revealed by cryo-EM. Sci Adv. (2021) 7:eabe5575. doi: 10.1126/sciadv.abe5575
31. Planchais C, Fernández I, Bruel T, Dias de Melo G, Prot M, Beretta M, et al. Potent human broadly SARS-CoV-2–neutralizing IgA and IgG antibodies effective against Omicron BA. 1 and BA. 2. J Exp Med. (2022) 219. doi: 10.1084/jem.20220638
32. Westendorf K, Žentelis S, Wang L, Foster D, Vaillancourt P, Wiggin M, et al. LY-CoV1404 (bebtelovimab) potently neutralizes SARS-CoV-2 variants. Cell Rep. (2022) 39. doi: 10.1016/j.celrep.2022.110812
33. Jones BE, Brown-Augsburger PL, Corbett KS, Westendorf K, Davies J, Cujec TP, et al. The neutralizing antibody, LY-CoV555, protects against SARS-CoV-2 infection in nonhuman primates. Sci Trans Med. (2021) 13:eabf1906. doi: 10.1126/scitranslmed.abf1906
34. Nutalai R, Zhou D, Tuekprakhon A, Ginn HM, Supasa P, Liu C, et al. Potent cross-reactive antibodies following omicron breakthrough in vaccinees. Cell. (2022) 185:2116–31. doi: 10.1016/j.cell.2022.05.014
35. Han P, Li L, Liu S, Wang Q, Zhang D, Xu Z, et al. Receptor binding and complex structures of human ACE2 to spike RBD from omicron and delta SARS-CoV-2. Cell. (2022) 185:630–40. doi: 10.1016/j.cell.2022.01.001
36. Yin W, Xu Y, Xu P, Cao X, Wu C, Gu C, et al. Structures of the Omicron spike trimer with ACE2 and an anti-Omicron antibody. Science. (2022) 375:1048–53. doi: 10.1126/science.abn8863
37. Zhao Z, Xie Y, Bai B, Luo C, Zhou J, Li W, et al. Structural basis for receptor binding and broader interspecies receptor recognition of currently circulating Omicron sub-variants. Nat Commun. (2023) 14:4405. doi: 10.1038/s41467-023-39942-z
38. Saville JW, Mannar D, Zhu X, Berezuk AM, Cholak S, Tuttle KS, et al. Structural analysis of receptor engagement and antigenic drift within the BA. 2 spike protein. Cell Rep. (2023) 42. doi: 10.1016/j.celrep.2022.111964
39. Xu Y, Wu C, Cao X, Gu C, Liu H, Jiang M, et al. Structural and biochemical mechanism for increased infectivity and immune evasion of Omicron BA. 2 variant compared to BA. 1 and their possible mouse origins. Cell Res. (2022) 32:609–20. doi: 10.1038/s41422-022-00672-4
40. Zhang W, Shi K, Geng Q, Herbst M, Wang M, Huang L, et al. Structural evolution of SARS-CoV-2 omicron in human receptor recognition. J Virol. (2023) 97:e00822–23. doi: 10.1128/jvi.00822-23
41. Khare S, Gurry C, Freitas L, Schultz MB, Bach G, Diallo A, et al. GISAID’s role in pandemic response. China CDC Weekly. (2021) 3:1049. doi: 10.46234/ccdcw2021.255
42. Machado DJ, Scott R, Guirales S, Janies DA. Fundamental evolution of all Orthocoronavirinae including three deadly lineages descendent from Chiroptera-hosted coronaviruses: SARS-CoV, MERS-CoV and SARS-CoV-2. Cladistics. (2021) 37:461–88. doi: 10.1111/cla.12454
43. Khare S, Gurry C, Freitas L, Schultz MB, Bach G, Diallo A, et al. Perspectives: GISAID’s role in pandemic response. China CDC Weekly. (2021) 3:1049–51. doi: 10.46234/ccdcw2021.255
44. Jumper J, Evans R, Pritzel A, Green T, Figurnov M, Ronneberger O, et al. Highly accurate protein structure prediction with AlphaFold. Nature. (2021) 596:583–9. doi: 10.1038/s41586-021-03819-2
45. Mirdita M, Schütze K, Moriwaki Y, Heo L, Ovchinnikov S, Steinegger M. ColabFold: making protein folding accessible to all. Nat Methods. (2022) 19:679–82. doi: 10.1038/s41592-022-01488-1
46. Case DA, Aktulga HM, Belfon K, Cerutti DS, Cisneros GA, Cruzeiro VWD, et al. AmberTools. J Chem Inf Model. (2023) 63:6183–91. doi: 10.1021/acs.jcim.3c01153
47. Berman HM, Westbrook J, Feng Z, Gilliland G, Bhat TN, Weissig H, et al. The protein data bank. Nucleic Acids Res. (2000) 28:235–42. doi: 10.1093/nar/28.1.235
48. Wang K, Jia Z, Bao L, Wang L, Cao L, Chi H, et al. Memory B cell repertoire from triple vaccinees against diverse SARS-CoV-2 variants. Nature. (2022) 603:919–25. doi: 10.1038/s41586-022-04466-x
49. Dejnirattisai W, Zhou D, Ginn HM, Duyvesteyn HME, Supasa P, Case JB, et al. The antigenic anatomy of SARS-CoV-2 receptor binding domain. Cell. (2021) 184:2183–200. doi: 10.1016/j.cell.2021.02.032
50. Dominguez C, Boelens R, Bonvin AMJJ. HADDOCK: a protein-protein docking approach based on biochemical or biophysical information. J Am Chem Soc. (2003) 125:1731–7. doi: 10.1021/ja026939x
51. van Zundert GCP, Rodrigues JPGLM, Trellet M, Schmitz C, Kastritis PL, Karaca E, et al. The HADDOCK2.2 web server: user-friendly integrative modeling of biomolecular complexes. J Mol Biol. (2016) 428:720–5. doi: 10.1016/j.jmb.2015.09.014
52. Vangone A, Bonvin AMJJ. Contacts-based prediction of binding affinity in protein–protein complexes. eLife. (2015) 4:e07454. doi: 10.7554/eLife.07454
53. Xue LC, Rodrigues JP, Kastritis PL, Bonvin AM, Vangone A. PRODIGY: a web server for predicting the binding affinity of protein–protein complexes. Bioinformatics. (2016) 32:3676–8. doi: 10.1093/bioinformatics/btw514
54. R Core Team. R: A Language and Environment for Statistical Computing. Vienna, Austria: R Foundation for Statistical Computing (2022).
55. Kruskal WH, Wallis WA. Use of ranks in one-criterion variance analysis. J Am Stat Assoc. (1952) 47:583–621. doi: 10.1080/01621459.1952.10483441
56. Wilcoxon F. Individual comparisons by ranking methods. Biometr Bull. (1945) 1:80. doi: 10.2307/3001968
57. Schrödinger LLC. The PyMOL molecular graphics system, version 1.8. (2015). Available at: https://pymol.org/.
58. Li H, Yan Y, Zhao X, Huang S-Y. Inclusion of desolvation energy into protein–protein docking through atomic contact potentials. J Chem Inf Model. (2022) 62:740–50. doi: 10.1021/acs.jcim.1c01483
59. Shoichet BK, Leach AR, Kuntz ID. Ligand solvation in molecular docking. Proteins: Struct Funct Bioinf. (1999) 34:4–16. doi: 10.1002/(ISSN)1097-0134
60. Sheward DJ, Yang Y, Westerberg M, Öling S, Muschiol S, Sato K, et al. Sensitivity of the sars-cov-2 ba. 2.86 variant to prevailing neutralising antibody responses. Lancet Infect Dis. (2023) 23:e462–3. doi: 10.1016/S1473-3099(23)00588-1
61. Wang X, Lu L, Jiang S. SARS-CoV-2 Omicron subvariant BA. 2.86: limited potential for global spread. Signal Transduct Target Ther. (2023) 8:439. doi: 10.1038/s41392-023-01712-0
62. VanBlargan LA, Errico JM, Halfmann PJ, Zost SJ, Crowe JE, Purcell LA, et al. An infectious sars-cov-2 b.1.1.529 omicron virus escapes neutralization by therapeutic monoclonal antibodies. Nat Med. (2022) 28:490–5. doi: 10.1038/s41591-021-01678-y
63. Tomezsko PJ, Ford CT, Meyer AE, Michaleas AM, Jaimes R. Human cytokine and coronavirus nucleocapsid protein interactivity using large-scale virtual screens. Front Bioinf. (2024) 4:1397968. doi: 10.3389/fbinf.2024.1397968
64. Xia H, Cao Z, Xie X, Zhang X, Chen JY-C, Wang H, et al. Evasion of type i interferon by sars-cov-2. Cell Rep. (2020) 33. doi: 10.1016/j.celrep.2020.108234
65. Tsugawa Y, Kato H, Fujita T, Shimotohno K, Hijikata M. Critical role of interferon-α constitutively produced in human hepatocytes in response to rna virus infection. PloS One. (2014) 9:e89869. doi: 10.1371/journal.pone.0089869
66. Sakuragi S, Liao H, Yajima K, Fujiwara S, Nakamura H. Rubella virus triggers type i interferon antiviral response in cultured human neural cells: involvement in the control of viral gene expression and infectious progeny production. Int J Mol Sci. (2022) 23:9799. doi: 10.3390/ijms23179799
67. Bai C, Zhong Q, Gao GF. Overview of SARS-CoV-2 genomeencoded proteins. Sci China Life Sci. (2022) 65:280–94. doi: 10.1007/s11427-021-1964-4
68. Chen S, Guan F, Candotti F, Benlagha K, Camara NOS, Herrada AA, et al. The role of B cells in COVID-19 infection and vaccination. Front Immunol. (2022) 13:988536. doi: 10.3389/fimmu.2022.988536
69. Badawi S, Ali BR. ACE2 Nascence, trafficking, and SARS-CoV-2 pathogenesis: the saga continues. Hum Genomics. (2021) 15:8. doi: 10.1186/s40246-021-00304-9
70. Khan MT, Islam MJ, Parihar A, Islam R, Jerin TJ, Dhote R, et al. Immunoinformatics and molecular modeling approach to design universal multi-epitope vaccine for SARS-CoV-2. Inf Med Unlocked. (2021) 24:100578. doi: 10.1016/j.imu.2021.100578
71. Parihar A, Sonia ZF, Akter F, Ali MA, Hakim FT, Hossain MS. Phytochemicals-based targeting RdRp and main protease of SARS-CoV-2 using docking and steered molecular dynamic simulation: A promising therapeutic approach for Tackling COVID-19. Comput Biol Med. (2022) 145:105468. doi: 10.1016/j.compbiomed.2022.105468
72. Parihar A, Ahmed SS, Sharma P, Choudhary NK, Akter F, Ali MA, et al. Plant-based bioactive molecules for targeting of endoribonuclease using steered molecular dynamic simulation approach: a highly conserved therapeutic target against variants of SARS-CoV-2. Mol Simulation. (2023) 49:1267–79. doi: 10.1080/08927022.2022.2113811
73. Quast I, Tarlinton D. B cell memory: understanding COVID-19. Immunity. (2021) 54. doi: 10.1016/j.immuni.2021.01.014
Keywords: SARS-CoV-2, coronavirus, antibodies, ACE2, protein docking
Citation: Yasa S, Guirales-Medrano S, Machado DJ, Ford CT and Janies D (2024) Predicting antibody and ACE2 affinity for SARS-CoV-2 BA.2.86 and JN.1 with in silico protein modeling and docking. Front. Virol. 4:1419276. doi: 10.3389/fviro.2024.1419276
Received: 18 April 2024; Accepted: 20 June 2024;
Published: 19 July 2024.
Edited by:
Akio Adachi, Tokushima University, JapanReviewed by:
Masaru Yokoyama, National Institute of Infectious Diseases (NIID), JapanCopyright © 2024 Yasa, Guirales-Medrano, Machado, Ford and Janies. This is an open-access article distributed under the terms of the Creative Commons Attribution License (CC BY). The use, distribution or reproduction in other forums is permitted, provided the original author(s) and the copyright owner(s) are credited and that the original publication in this journal is cited, in accordance with accepted academic practice. No use, distribution or reproduction is permitted which does not comply with these terms.
*Correspondence: Shirish Yasa, c3lhc2FAY2hhcmxvdHRlLmVkdQ==; Daniel Janies, ZGphbmllc0BjaGFybG90dGUuZWR1
Disclaimer: All claims expressed in this article are solely those of the authors and do not necessarily represent those of their affiliated organizations, or those of the publisher, the editors and the reviewers. Any product that may be evaluated in this article or claim that may be made by its manufacturer is not guaranteed or endorsed by the publisher.
Research integrity at Frontiers
Learn more about the work of our research integrity team to safeguard the quality of each article we publish.