- 1Department of Microbial Ecology, Netherlands Institute of Ecology (NIOO-KNAW), Wageningen, Netherlands
- 2Laboratory of Virology, Wageningen University and Research, Wageningen, Netherlands
- 3Department of Plant Biology and the Ecology, Evolution and Behavior Program, Michigan State University, East Lansing, MI, United States
Many plant viruses have a multipartite organization, with multiple genome segments packaged into separate virus particles. The genome formula describes the relative frequencies of all viral genome segments, and previous work suggests rapid changes in these frequencies facilitate virus adaptation. Many studies have reported mixed viral infections in plants, often resulting in strong virus–virus interactions. Here, we tested whether mixed infections with tripartite alfalfa mosaic virus (AMV) and monopartite potato virus Y (PVY) affected the genome formula of the tripartite cucumber mosaic virus (CMV), our experimental model. We found that the CMV titer was reduced in mixed infections with its tripartite Bromoviridae relative AMV and in triple infections with both AMV and PVY, indicating notable virus–virus interactions. The variability of the CMV genome formula was significantly lower in mixed infections (CMV and AMV, CMV and PVY, and CMV and AMV and PVY) than in single infections (CMV only). These observations led to the surprising conclusion that mixed infections with two distinct viruses constrain the CMV genome formula. It remains unclear how common these effects are for different combinations of virus species and strains and what the underlying mechanisms are. We, therefore, extended a simulation model to consider three putative scenarios in which a second virus affected the genome formula. The simulation results also suggested that shifts in the genome formula occur, but may not be widespread due to the required conditions. One scenario modeled—co-infection exclusion through niche differentiation—was congruent with the experimental data, as this scenario led to reductions in genome formula variability and titer of the multipartite virus. Whereas previous studies highlighted host–species effects, our results indicate that the genome formula is also affected by mixed infections, suggesting that there is a broader set of environmental cues that affect the genome formula.
Introduction
Many plant viruses have a multipartite genome organization in which the segmented genome is packaged into separate virus particles (1). A multipartite genome organization is considered costly due to the constraints it places on transmission. For effective transmission of multipartite viruses, a high multiplicity of infection (MOI, the number of virus particles entering each cell) is required to ensure that no genome segments are lost (2). The substantial costs associated with multipartition raise the question of the potential benefits associated with multipartite genome organization. One hypothesis is that multipartition allows for flexible gene expression through differential accumulation of segments, as the accumulation of each genomic segment of a multipartite virus is not equal. This unequal ratio of segment accumulation is referred to as the “genome formula”. In both faba bean necrotic stunt virus (FBNSV) (3) and alfalfa mosaic virus (AMV) (4), variations in the genome formula have been observed in viral populations. The genome formula is not a fixed viral trait but rather depends on factors such as host species (4, 5) and the presence of viral satellites (6, 7). Through altering the genome formula, the gene copy number is flexible in multipartite viruses. This flexibility could allow for adaptation to heterogeneous environments by changing the gene expression without mutation (3, 8). By contrast, it has been suggested that the genome formula could stabilize the gene expression of DNA viruses by compensating for differences in transcription (9). Theory suggests that the optimum equilibrium value for the genome formula may be sensitive to the exact conditions under which the virus is replicating, varying with the multiplicity of infection and even the size of the host cell population (10).
Mixed viral infections are common in plants in both natural and agro-ecosystems (11, 12). Indeed, mixed infections are more common in nature than predicted by chance (13), partly due to viruses sharing competent vector species (14, 15). Virus–virus interactions in mixed infections can be characterized on a scale ranging from synergistic to antagonistic, and often result in a change in the viral titer of one or several of the virus species present. This change in titer can be a strong increase (16–21) or a decrease (22).
Interspecific virus competition increases as relatedness increases (23), in some cases resulting in superinfection exclusion where a primary virus infection prevents the onset of secondary infection by another virus. This phenomenon is also referred to as “cross-protection” in cases where infection with a mild strain protects against a more virulent strain (24). When multiple (related) viruses infect a host at the same time, they may spatially segregate and invade separate clusters of cells, interacting only at the borders of these clusters (25). This phenomenon is termed “mutual exclusion” (26, 27).
We expected mixed infections to affect the genome formula under many conditions, for three reasons. First, although the temporal dynamics of genome formula change have not been studied in detail, both theoretical and experimental work suggests that the genome formula can change rapidly. Models suggest that genome formula shifts occur within several viral generations, provided the MOI is low (10). One week of infection was sufficient time for the AMV genome formula to adapt to different hosts (4), suggesting that over the span of a single mixed infection event, there is enough time for the genome formula to change. Second, the host species has an effect on the genome formula for FBNSV and AMV (3, 4); as plant viruses reprogram host cells and alter plant physiology, we expected that mixed infections could likewise affect the genome formula. Third, theoretical work suggests that the genome formula equilibrium is highly sensitive to the exact conditions under which the virus replicates, including MOI and the host cell population size (10). Given the strong interactions that occur between many viruses in mixed infections and the demonstrable effect of mixed infections on titer, we expected the genome formula to be affected by co-infection with other viruses.
Here, we introduce three scenarios that illustrate mechanisms by which mixed infections can affect the genome formula. We introduce these scenarios to illustrate that multiple plausible mechanisms could explain how mixed infections affect the genome formula, inspired by the structure used in the presentation of seminal work (28). Later, we will develop simulation models of virus infection based on these three scenarios, capturing the mechanisms proposed, with the aim of exploring whether they can lead to changes in the genome formula. For simplicity, the scenarios are illustrated using two viruses, one monopartite and one multipartite, although the mechanisms may equally apply to multipartite–multipartite virus interactions.
Scenario 1: co-opting of viral gene products. If a co-infecting monopartite virus provides a function in trans (i.e., which can be used by another virus), the multipartite co-infecting virus may downregulate its segment containing the gene encoding an equivalent function. With this downregulation, the multipartite virus benefits from the monopartite virus’s gene expression and reduces its own costs. An example of a trait where this scenario may be applicable is within-host movement. In mixed infections of cucumber mosaic virus (CMV) and zucchini yellow mosaic virus (ZYMV, Potyviridae) in Cucurbita pepo, systemic movement of CMV is facilitated by ZYMV (29). In this scenario, we would predict the downregulation of CMV RNA3, which encodes the movement protein.
Scenario 2: co-infection exclusion. If a monopartite virus occupies the same niche as a multipartite virus, the genome formula of the multipartite virus may shift due to changing selection pressures (e.g., selection for rapid movement or upregulation of the replication machinery).
Scenario 3: co-infection exclusion through niche differentiation. If a monopartite virus partially occupies the same niche as a multipartite virus and outcompetes it in the overlapping niche portion, the observed overall genome formula of the multipartite virus may shift if different genome formulas are selected in each niche. As the monopartite outcompetes the multipartite, only the subpopulation in the exclusive niche space will be maintained. It will become the sole determinant of the overall genome formula of the multipartite virus across multiple niche spaces. This effect becomes visible only when the first niche space no longer contributes to virus accumulation. An example of niche competition and exclusion in a host–pathogen system has been observed in mixed infections with tapeworm species Hymenolepsis diminuta and acanthocephala species Moniliformis dubius, where M. dubius excluded H. diminuta from occupying the anterior region of the intestine, leading to distinct niches for both parasites in mixed infections, whereas in single infections their niches would overlap (30, 31). While we predicted that mixed infections may lead to virus–virus interactions and thereby result in genome formula change, empirical evidence is lacking. Thus far, the only data showing an effect of virus–virus interactions on the genome formula involve satellite viruses (6, 32).
In this paper, we tested empirically whether mixed infections can affect the genome formula and used simulation models to explore what the underlying mechanisms could be. We focused on experimentally measuring the effect of mixed viral infections on the genome formula and the viral titer. We inoculated Nicotiana tabacum plants with combinations of the tripartite +ssRNA CMV, tripartite +ssRNA AMV, a virus from the same Bromoviridae family as CMV, and the monopartite +ssRNA potato virus y (PVY) of the Potyviridae family, whose member species are commonly found in synergistic mixed infections with CMV in nature (12, 21). In our experiments, we found that mixed infections strongly affected both the CMV titer and the variability of the CMV genome formula, causing reductions in both. Our simulation models showed that the genome formula can be altered in all three hypothetical scenarios, while the results for Scenario 3 are most comparable to the data.
Results
The genome formula of cucumber mosaic virus is more variable in single infection
We measured the accumulation of CMV in single and mixed infections with AMV, PVY, or both viruses to determine the effect of co-infection on the CMV genome formula. For all plants for which we confirmed infection by all viruses present in the inoculum, we quantified the relative accumulation of CMV RNA1, RNA2, and RNA3 by RT-qPCR to determine the genome formula (Figure 1, Table 1). CMV’s RNA2 and RNA3 both express a second protein (i.e., 2b and CP, respectively) from a subgenomic RNA, but we designed our primers to anneal to a region present only in the full-length RNA (33). In single CMV infections, the genome formula was variable between plants, as shown by the spread between observations (Figure 1). In this treatment, the relative frequency of RNA1 ranged from 0.232 to 0.615, highlighting the considerable genome formula variation between plants. In all three mixed infections, the CMV genome formula appeared to be constrained to a smaller space, as evidenced in a ternary plot, and similar in all three scenarios (Figure 1). The smaller genome formula space occupied by the mixed infections overlapped a portion of the larger space occupied by the single CMV infections. The standard deviations of the GF data are ~2–5× times greater for CMV RNAs 1 and 3 in the single CMV infections than in the mixed infections (Table 1).
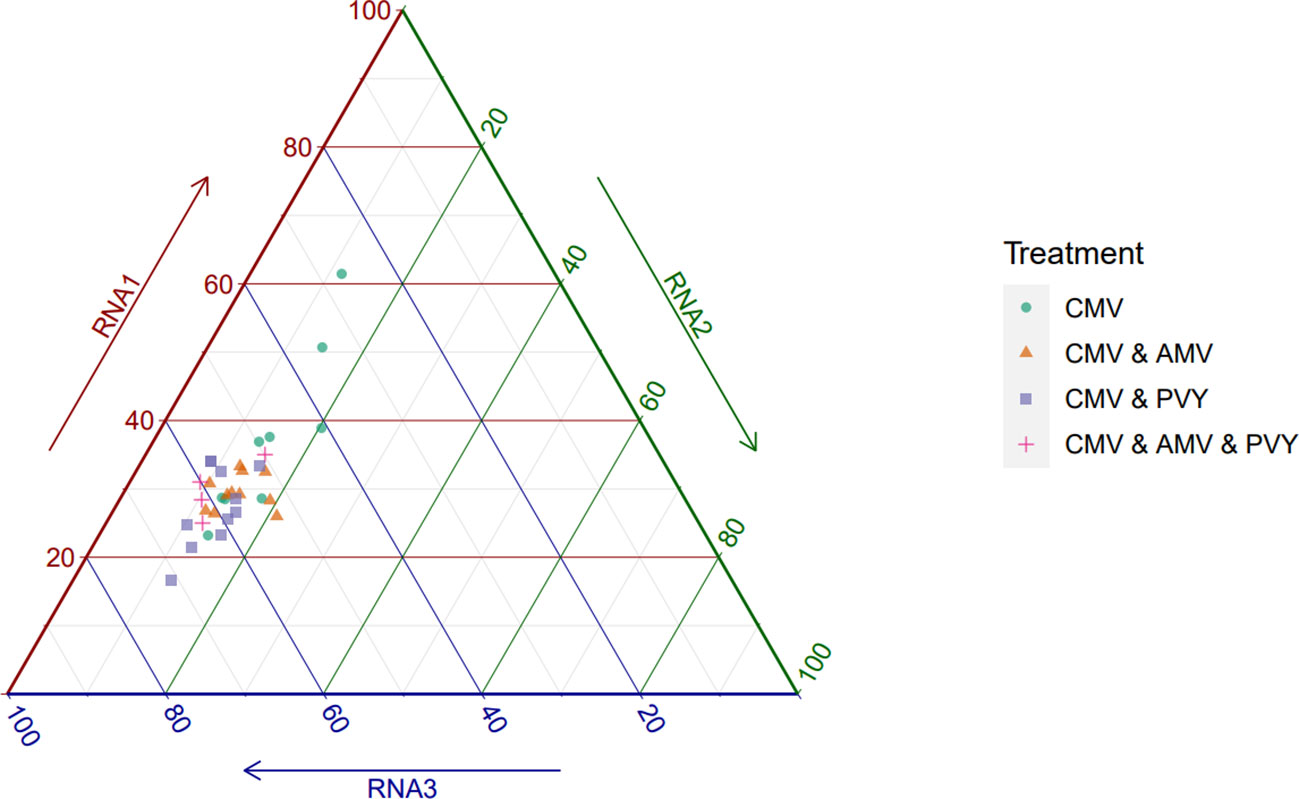
Figure 1 Cucumber mosaic virus genome formulae across mixed infection treatments. Relative segment frequencies for CMV RNA1, RNA2, and RNA3 are shown in a ternary plot. Relative frequencies are shown as percentages ranging from 0% to 100%. The genome formula for CMV is constrained to the lower left corner of the genome formula space under mixed infection conditions (low RNA 1, high RNA 3 frequency). For single infections, CMV can occupy a wider range of genome formula values (high RNA 1, low RNA 3). Across all samples, RNA 2 frequency is low.
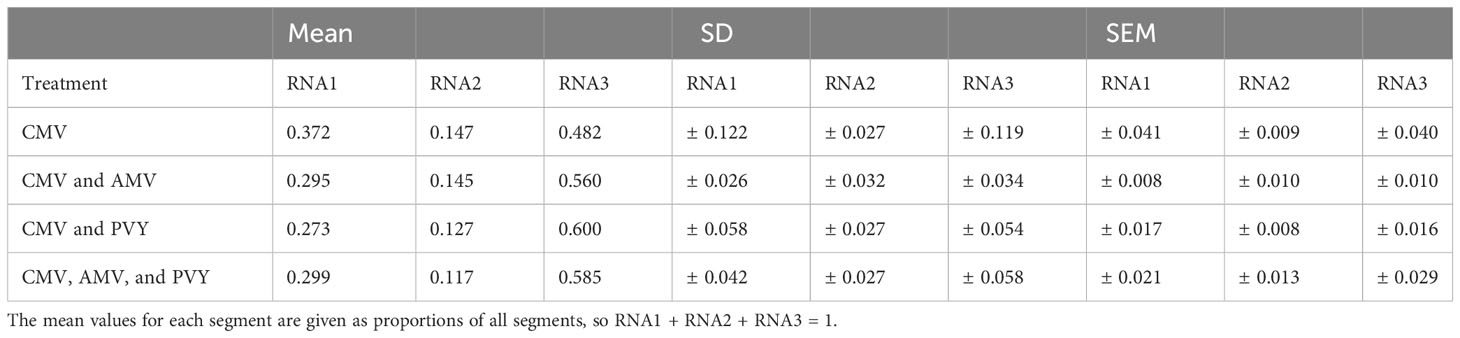
Table 1 Cucumber mosaic virus genome formulas in single and mixed infections in Nicotiana tabacum (SD, Standard deviation and SEM, standard error of the mean).
For the formal analysis of the genome formula data, we used an approach based on PERMANOVA, a permutation-based non-parametric analysis of variance (34). As the different CMV RNAs interact with each other during infection, we performed a univariate analysis on the distance in the three-dimensional genome formula space between observations (see Methods). Using this approach, we found that overall there were significant differences between treatments (F3,34 = 3.804, p = 0.012), where treatment accounted for 26.9% of the variation based on the r2 value. We then performed the PERMDISP2 procedure on the same genome formula distance metric to test specifically whether the genome formula spread was homogenous over treatments (35). We found significant differences in genome formula spread between treatments (F3,34 = 3.817, p = 0.017), although in pairwise post-hoc comparisons only the difference between CMV infections and CMV and AMV mixed infections was significant (corrected p-value = 0.043; see Table S1). A significant PERMANOVA result can indicate differences in centroid, spread, or both, whereas the PERMDISP2 procedure detects differences in spread only. As both procedures gave a significant result here, we can only conclude with certainty that mixed infections significantly affect the spread of the CMV genome formula. The formal analysis, therefore, supports the notion that mixed infections restrict CMV to a smaller region of genome formula space, as suggested by the visualization of the experimental data (Figure 1).
Mixed infection reduces the titer of cucumber mosaic virus in N. tabacum
The titer of CMV was measured on a subset of four randomly selected infected plants per treatment. We measured CMV titer as the sum of the accumulation of each RNA segment relative to the stably expressed transcripts of three N. tabacum genes (Table S2) (36) using RT-qPCR. CMV titer estimates were log-transformed prior to the ANOVA. In comparison with single infections, the CMV titer was significantly lower in the mixed infections tested (one-way ANOVA, F3,12 = 17.178, p = 1.22 × 10-4) (Figure 2). This significant effect was driven by AMV, as both the CMV and AMV double infection and the CMV, AMV, and PVY triple infection are significantly different from the CMV single infection, whereas the CMV and PVY double infection is not (Table 2). AMV showed a highly variable titer across replicates under mixed infection conditions (as illustrated by the SEM in Figure 2). In the CMV and AMV double infection, AMV titers ranged from 8-fold to 44,423-fold higher than host reference transcripts. In the triple infection, the range of AMV titers was 68-fold to 760,614-fold. Interestingly, there was no correlation between CMV titer and the titers of co-infecting viruses AMV (Pearson correlation on log-transformed accumulation; r = 0.05, n = 6, p = 0.90) or PVY (r = 0.28, n = 6, p = 0.51). Though large titer differences were observed for AMV, its genome formula was not reliably measurable using our current assay because of high AMV titers, which were nearly outside the dynamic range of the assay. The PVY titer in mixed infections was consistently low (Figure 2). Samples of PVY single infections were lacking due to the accidental destruction of samples during diagnostic assays. Thus, we could not determine whether PVY titer was low due to competition with co-infecting viruses or whether PVY accumulation decreased over the course of infection regardless of the presence of other viruses. In single infections, the AMV titer was similar to the high and variable levels observed in triple infections, and these results are included in the data deposition.
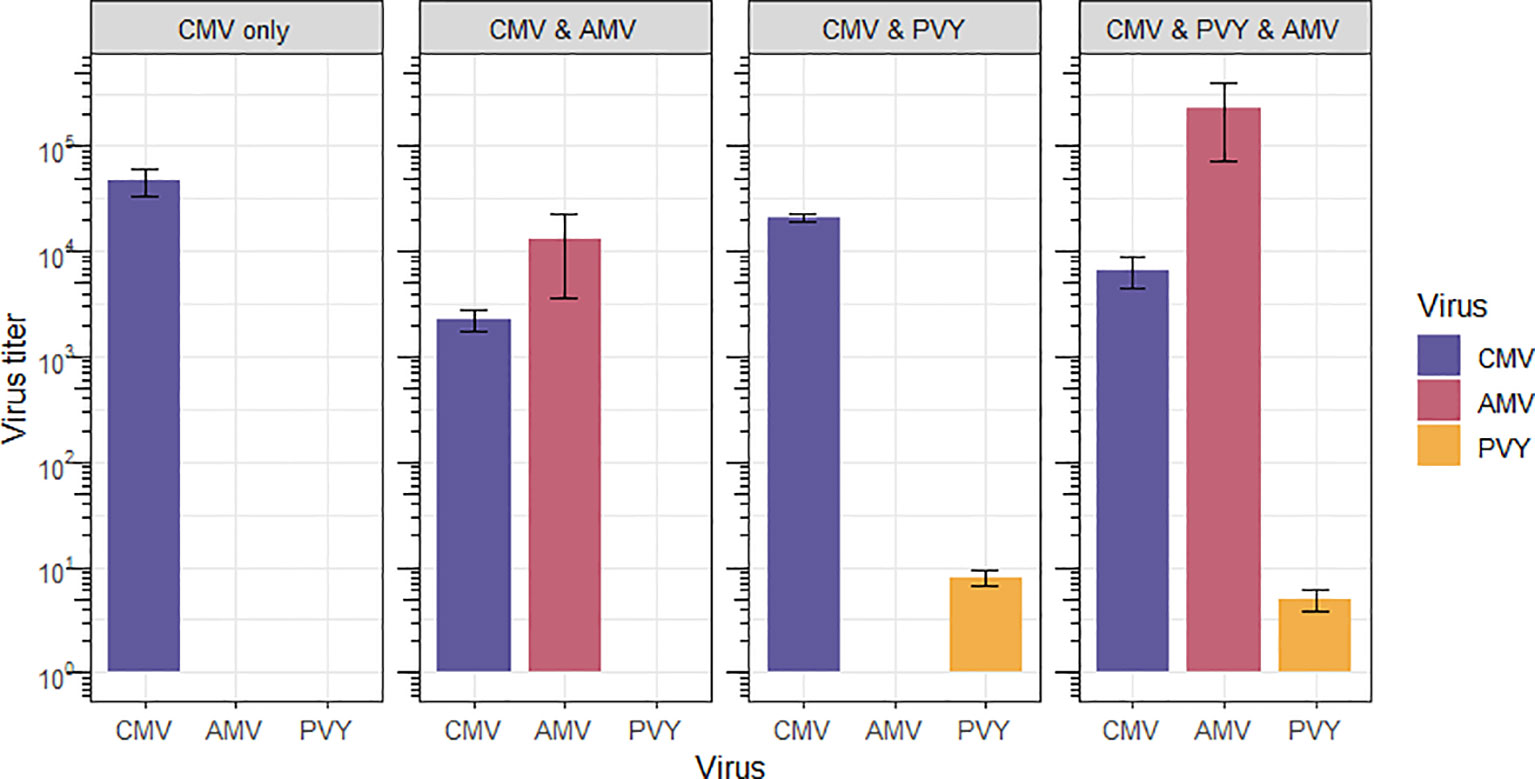
Figure 2 Virus titers in single, double, and triple co-infections. Titer is shown per mixed infection treatment (boxes, labeled on top) relative to the mean accumulation of stable host reference genes L25, β-tubulin, and Ntubc2 (data not shown). For multipartite viruses CMV and AMV, titer is shown as the sum of the accumulation of each segment. Error bars show the standard error of the mean (SEM) between biological replicates (n=4). The titer is plotted on a 10-log scale.

Table 2 Dunnett’s test for comparing the CMV titer in mixed infection treatments with a CMV single infection as the control treatment.
Simulations of virus evolution predict that mixed infections can affect the genome formula
Three scenarios under which mixed infections can affect the genome formula were described in the introduction: co-opting of viral gene products (Scenario 1), co-infection exclusion (Scenario 2), and co-infection exclusion through niche differentiation (Scenario 3). To explore whether these three scenarios are plausible, we adapted a previously developed simulation model of genome formula evolution in a bipartite virus (10). Our model predicted how the genome formula would evolve over several rounds of infection in a population of cells, incorporating stochastic variation in the number of virus particles infecting individual cells and a function linking the genome formula and virus particle yield. The MOI (a mean value) of both viruses was fixed over rounds of infection, which allowed us to study the effect of the second virus on the multipartite virus’s genome formula without having to choose conditions that favored the maintenance of both viruses at similar levels. The results for each scenario are described in detail below. Figure 3 shows an illustration of each scenario and the resulting modeled genome formula change over a range of MOIs. For a sub-selection of conditions explored, we show genome formula values over rounds of infection to demonstrate that equilibrium had been reached by the end of the simulation (Figures S1–S3).
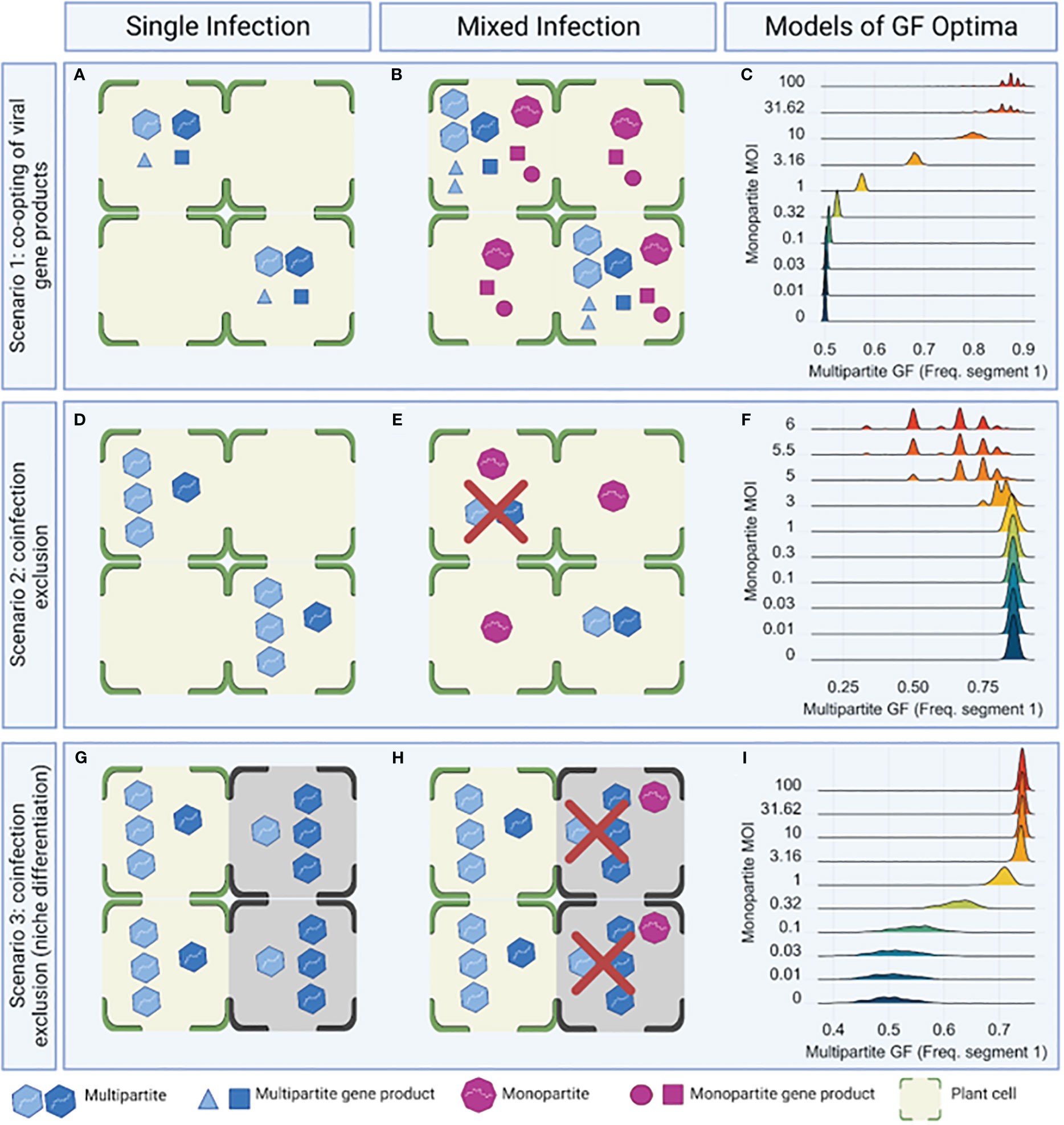
Figure 3 Overview of three simulation models of mixed infections and the effect on multipartite virus accumulation. For each modeled scenario, three panels are shown. The left and middle panels show a cartoon overview of the modeled mechanism under single (A, D, G) and mixed (B, E, H) infection conditions. The right panel shows the simulated optimal genome formula under model conditions (C, F, I). Scenario 1 (A–C) illustrates how the co-option of gene products from a second virus can impact the genome formula of a multipartite virus, in this case shifting the genome formula from a balanced value (f1 =0.5) to an unbalanced one (f1 > 0.5) as more cells become infected by the second virus (C). Scenario 2 (D–F) illustrates a shift in the genome formula caused by a reduction in the number of infected cells due to co-infection exclusion by a second virus, which forces the multipartite virus to optimize infectivity over virus particle yield. In this case, the genome formula imbalance becomes smaller as the number of cells infected by the second virus increases (F). Scenario 3 (G–I) illustrates the effects of having multiple niches (shown here in green and dark gray) that can be invaded by the multipartite virus while having a second, monopartite virus that can infect only one niche. If the second virus can restrict access of the multipartite virus to those cells it infects, and if the two niches have different optimal genome formula values, the genome formula is predicted to change (I). Model output (C, F, I) is colored along a range of high MOI (red) to low MOI (blue) of the monopartite virus. This figure was created using BioRender.com.
Scenario 1: co-opting of viral gene products. Scenario 1 allowed the multipartite virus to co-opt a gene product from the second, monopartite virus, which replaced its own second-segment gene product. As the MOI of the monopartite virus increased, the multipartite genome formula shifted away from a balanced equilibrium (i.e., a 1:1 ratio of segments) toward higher frequencies of segment 1 (Figures 3A–C).
Scenario 2: co-infection exclusion. Scenario 2 allowed co-infection exclusion of the multipartite virus by the second, monopartite virus; the monopartite virus blocked replication of the multipartite virus in all cells it infected. If there was an unbalanced genome formula equilibrium (i.e., not equimolar) in the absence of the monopartite virus, as the MOI of the second virus increased, the genome formula shifted away from its initial equilibrium to a more balanced equilibrium (Figures 3D–F). This shift occurred because the number of cells infected by the multipartite virus became smaller due to co-infection exclusion. The surviving multipartite virus populations shifted their genome formula to a balanced value (1:1) that optimized infectivity over virus particle yield (10). Under the conditions chosen, the highest virus–particle yield per cell was obtained when the intracellular frequency of virus segment 1 was ~0.91 (this follows because μ = 1; see the Methods section for a full explanation of parameter μ). Given that we assumed that the probability of infection is the same for all virus particles, equal frequencies of virus segments (i.e., frequency of segment 1 = frequency of segment 2 = 0.5) would lead to infection of most cells. As there would be a trade-off between virus–particle yield per infected cell and the probability of infecting a cell, the equilibrium genome formula would reflect a value that maximized virus–particle production over all cells. For the MOI value (2) used, in the absence of the second virus, the equilibrium frequency of segment 1 was approximately ~ 0.86 (Figure 3F), suggesting that the selection of the genome formula was driven by virus–particle yield. As the second virus infected more cells and the number of cells infected by the multipartite virus decreased, the frequency of segment 1 fell to ~ 0.5 (Figure 3F), suggesting that selection was driven by infecting as many cells as possible. This shift in the main driver of selection occurred because when the MOI is 2, the intracellular frequency of virus segment 1 is 0.5 in most infected cells, as most cells will be infected by a 1:1 ratio of the two segments regardless of the segment frequencies in the inoculum. Only in a small number of cells will high frequencies of segment 1 be reached, which would lead to strong increases in virus–particle yield per cell. As the total number of infected cells dropped due to the action of the second virus, infections with high frequencies in segment 1 became so scarce that they no longer affected the equilibrium genome formula.
Scenario 3: co-infection exclusion through niche differentiation. Scenario 3 expanded on Scenario 2, dividing the population of host cells into two subpopulations with different optimal values of the genome formula for virus yield. The monopartite virus infected and caused coinfection exclusion in only one of the cell subpopulations. As the MOI of the second virus increased, the multipartite virus populations shifted away from the balanced genome formula toward a higher frequency of segment 1 (Figures 3G–I).
These simulation results (shown in Figures 3C, F, I) show that it is plausible that co-infection affects the genome formula through different mechanisms. By contrast, co-infection clearly does not lead to universal changes in the multipartite genome formula. In all three scenarios considered here, at least a moderate level of cellular infection by the second monopartite virus was required for appreciable changes in the genome formula (monopartite MOI ≥ 0.3, meaning ≥ 25% of cells were infected by it). In addition, Scenario 1 required the second virus to produce gene products to replace functionally all of those from one multipartite virus segment. Scenario 2 required a trade-off between optimal genome formula values for the infection of cells and for the virus–particle yield, as explained in the results paragraph on this scenario above. Finally, Scenario 3 required different genome formula optima in the two niches to see a genome formula shift. The model results, therefore, illustrate that mixed infections can affect the genome formula, while we emphasize that this effect manifests only under select conditions and not universally.
Discussion
Genome formula variability of cucumber mosaic virus decreased under mixed infections
Through experimental co-infection with the multipartite virus CMV, we have shown that the virus titer and the variability of the genome formula of CMV are reduced in co-infections with multipartite relatives AMV and monopartite PVY and in a triple co-infection with both. Recent work has emphasized the variability of the genome formula (3, 4, 37). A key factor shown to influence the genome formula of multipartite viruses is the host species (3, 4, 37), but prior work on CMV showed that its genome formula is stable across hosts Nicotiana tabacum, Nicotiana benthamiana, and Chenopodium quinoa (33). The lack of genome formula variability at the between-host level suggests that CMV may have a more stable genome formula than other multipartite viruses studied to date, and that genome formula plasticity may not be universal in multipartite viruses. Strikingly, the reduced CMV genome formula variability we observed here appeared to be independent of (i) the titer of the co-infecting virus—AMV constrained the CMV genome formula to the same space at both high and low AMV titers—and (ii) the effect of the co-infecting virus on CMV titer—PVY constrained the genome formula space of CMV but did not affect CMV titer. The lack of effect of PVY on the CMV titer contradicts previous studies reporting either a synergistic increase in the titer of both viruses (21) or an increased CMV titer (38) in a CMV-Potyviridae mixed infection, suggesting that virus–virus interactions may depend on the specific viral strains used. CMV has an exceptionally large host range (~1,200 species) (39), which increases its chance of encountering other viruses in mixed infections more than specialist multipartite viruses such as FBNSV. We speculate that this may have led to a constrained optimal genome formula for CMV in mixed infections compared to the range of genome formulas shown in single infections. Of note is a recent study of bipartite begomoviruses, which frequently occur as co-infections, in which the genome formula was distinctly different in single and mixed infections in plants (40), highlighting that the changes in genome formula variability we have described for CMV might not apply to other multipartite viruses. The experimental work on the effects of mixed infections on the genome formula is therefore limited to a handful of virus strains and species under a limited set of conditions. It remains to be shown how common these effects are for different viruses across conditions and what the full scope of the effects on virus–virus interaction and the genome formula might be.
Integrating experimental data and modeling results
We expected highly regulated biological systems to exhibit low variability in a constant environment. Given that the presence of other viruses could disrupt viral replication and spread, we would have expected higher genome formula variability in mixed infections. However, in our experiments, we found that mixed infections reduced the variability of the CMV genome formula more than single infections. By contrast, in the three scenarios modeled, we saw different effects of mixed infections on genome formula variability. In Scenarios 1 and 2, genome formula variability increased concomitantly with the MOI of the second virus. In both cases, changes in genome formula variability were likely dependent on the exact model parameters chosen, so we do not think the observed patterns are very informative for predicting changes in genome formula variability. For Scenario 3, we saw a strong decrease in genome formula variability, driven by the restriction of the multipartite virus to a subpopulation of host cells. The proposed mechanism not only will lead to a shift in the genome formula but will also always lead to a reduction in genome formula variability: mixed infections limit the multipartite virus to a population of cells that are more homogeneous concerning the optimal genome formula. Interestingly, this scenario would also predict a reduction in the CMV titer in mixed infections due to intraspecific competition, as observed here in some treatments. Moreover, the restriction of the multipartite virus to a subpopulation of cells could be induced by different viruses. Cowpea chlorotic mottle virus (CCMV) has a strong antagonistic effect on the spatial distribution of CMV during mixed infections in some tissues (41), highlighting that these types of virus–virus interactions exist and that CMV is susceptible to such effects. As both AMV and PVY decrease CMV genome variability and limit the genome formula to a similar space, a parsimonious explanation for the experimental results will not depend on highly specific virus–virus interactions. The underlying mechanism for Scenario 3 is therefore congruent with the experimental results we have found, although other mechanisms cannot be ruled out. For example, if mixed infections restrict the movement of a multipartite virus, the effects of genome formula drift could be stronger, as the virus will invade fewer host tissues and population bottlenecks could become narrower.
Reflection and outlook on the modeling approach used
Mathematical models are powerful tools in biology, and there is a wide range of modeling approaches with different strengths and weaknesses that suit different research aims (42, 43). Here, we explore a new research question for which there is little previous work to build and only limited data available. There are only a few empirical (3, 4, 9, 40) and modeling (10) studies on the genome formula, and to date only relatively simple “proof-of-principle” models of multipartite virus infections have been developed (2). The advantage of these simple models for exploratory work is that they require less knowledge about a system because they focus on one or a few key processes, have fewer free model parameters, and are more readily interpretable due to their limited complexity. By contrast, these models are not very useful for making specific predictions for real-world situations (42).
One key simplification in the modeling approach used was to model a bisegmented multipartite virus, even though our model system, CMV, had a tripartite genome. We think this approach is fully justified because of the aim of our modeling work: understanding in general terms whether mixed infections can possibly affect the genome formula. Our goal was to explore whether mechanisms of co-option of viral proteins, limiting cellular infection levels, or virus niches can affect the genome formula under a set of plausible assumptions and conditions. Given this goal, our model did not include functional differences between virus gene products but rather made assumptions about what levels of these products are needed. Having a third genome segment and its gene products would therefore add model complexity and free parameters and reduce the interpretability of the model and the clarity of its presentation. By contrast, it would not make model predictions more relevant to a specific virus (i.e., CMV), because in the model interactions between virus genome segments and proteins are determined by simple statistical distributions rather than a mechanistic model. Given this high level of abstraction and the focus on a proof of principle, we can be confident that the number of genome segments will not affect the conclusions drawn based on these model results.
Although we chose to work with simple models of infection, we still needed to set free model parameters to specific values or ranges of values. For many of these free parameters, reasonable model values could be determined from previous work or empirical estimates. For example, we set the MOI to a low value (λ = 2) because in other modeling work, this value led to rapid adaptation by the genome formula, thereby limiting the number of rounds of infection needed to study changes (10). Moreover, this value was within the range of empirical values reported for plant viruses (44). For the parameter that determined the sensitivity of virus–particle yield to changes in the genome formula, we chose a value that led to high sensitivity (σ2 = 10). For this value of σ2, multipartite viruses are expected to outcompete their monopartite cognates (10), making this a logical choice for modeling a virus–host interaction that is conducive to the propagation of a multipartite virus. Note that this parameter choice would not affect the performance of monopartite viruses here, as we fixed the infection level of the monopartite virus in our models to ensure a constant environment in which the genome formula could reach an equilibrium value. Finally, some model parameter values were dictated by the scenarios we have put forward. For example, the parameter μ determined the value of the genome formula that renders optimal virus particle yield. In Scenarios 2 and 3, we postulated the existence of two different selective environments with the host (niches), dictating that different values of μ should be chosen for each environment.
In sum, we chose to work with a simple model of infection and used a heuristic approach to determine many model parameters. We recognize that this approach cannot predict how common genome formula shifts in mixed infections might be. What we can conclude is that there are clearly regions in the parameter space where genome formula shifts do and do not occur, and in all three scenarios there are specific conditions that have to be met to see genome formula shifts (i.e., Scenario 1: functional replacement of all products on a segment; Scenario 2: μ ≠ 0; Scenario 3: different μ values for the two within-host environments). We, therefore, speculate that although the model results suggest that mixed infections can induce genome formula shifts, these changes are not ubiquitous because the required conditions are often not met.
Localization of the virus in the host and timing of infection
It is important to consider whether each of the viruses used in this study can exist in the same cellular compartment, as co-localization in the same tissues was one of the mechanisms proposed to drive genome formula change in our modeling scenarios. CMV genome segments are localized in the cytoplasm of N. tabacum, with replication taking place near intracellular membranes (45). CMV proteins involved in replication (1a and 2a) have been shown to co-localize in association with the tonoplast, as have AMV replication proteins P1 and P2 (45, 46). Potyviruses replicate in cytoplasmic vesicles associated with ER membrane systems (47). In summary, CMV and AMV gene products associated with replication co-localize in the same cellular compartments, whereas PVY co-localization with AMV and CMV is speculative.
An additional factor that may affect the accumulation and the genome formula is the timing of infection. Disease progression is not the same across the viral species used in our experiments. The PVY strain used (PVY-757) was a tuber necrotic strain (PVY-NTN), which induces veinal necrosis in N. tabacum. We expect the low PVY titer we observed may be due to the PVY infection already being past its peak titer at the time of harvest, 14 days post inoculation (dpi). Currently, we have a snapshot of the accumulation within the whole plant at 14 dpi due to the sampling design that is destructive to the plant. Ideally, we would have multiple time points per replicate for which both the titer and genome formula are measured. Similarly, AMV infections may have been at different stages of infection due to stochasticity in the progression of infection, which may explain the high variability in their titers. The timing and order of inoculation may also play a role in how viruses accumulate in the host over time and the ensuing virus–virus interactions. Infections can be initiated as co-infections, where multiple viruses are inoculated at the same time, or super-infections, where one virus is inoculated first and another virus is inoculated later. For simplicity, we used co-infections in our experiments. Under co-infection conditions, related viruses localize to separate cell clusters through mutual exclusion instead of co-localizing, as has been shown for potyvirus mixed infections (26) and CMV mixed infections (48). In the real world, plant hosts are likely to be exposed to different viruses at different times, making it relevant to study the effects of super-infections on the genome formula.
“Transcriptome” versus “genome” formula
Seminal work on the “genome formula” was developed using FBNSV as a model system. Notably, the viruses used in this study differ from FBNSV in that their genomes were composed of (+)ssRNA as opposed to circular ssDNA. For multipartite DNA viruses, a distinction is made between the “transcriptome formula” and the “genome formula” (9), where the former refers to the relative accumulation of RNA transcripts and the latter to the relative accumulation of genomic segments. For (+)ssRNA viruses, the genome and transcriptome—with the exception of subgenomic RNA—are one and the same, and distinguishing between the two is not possible for a total RNA sample. If the mechanism by which the genome formula is adaptive is through stable ratios of transcripts, as suggested for FBNSV, where the genome formula is distinct per host but the transcriptome formula is similar per host (9), then the genome formula for an RNA virus is expected to be relatively stable and unchanged upon environmental change. These changing environments may include mixed infections, which may explain why the CMV genome formula did not move to a different equilibrium frequency but instead was constrained to a smaller part of the genome formula space. On the contrary, the genome formula of (+)ssRNA virus AMV does appear to be variable. A strong effect of host plant species on the genome formula has been observed (4), highlighting the plasticity of the AMV genome formula. Here we found that mixed infections led to higher variability in AMV titers, while we could not discern an effect on the AMV genome formula. Earlier work shows the limited and variable systemic movement of AMV in tobacco (49). Antagonistic interactions with other viruses, mediated by host immune responses (50), rather than direct interactions between viruses like superinfection exclusion, may exacerbate this variation, resulting in the patterns we observed. Finally, an important difference between AMV and CMV is the lack of appreciable silencing suppression activity in AMV (51). We speculate that this omission could result in higher AMV genome formula variability, either by obliging AMV to adapt to different hosts’ immune responses through the genome formula or by allowing host RNA interference to spur (non-adaptive) genome formula changes, or both.
Methods
Experimental effect of viral mixed infection on the genome formula
Plants and virus strains
Nicotiana tabacum (cv. “White Burley”) was used for the amplification of all viruses and all experiments.
Natural virus isolates were used in all experiments: CMV subgroup I isolate I17F (52), AMV isolate 425 (“Wisc425”) (53), and PVY isolate 757, an isolate collected in the Netherlands in 2006 for which virus identity was confirmed serologically and by sequencing. All isolates were obtained through the virus collection of Wageningen Plant Research (www.primediagnostics.com).
Inoculation and harvest
N. tabacum seeds were surface sterilized using 5% bleach and allowed to germinate on filter paper in a Petri dish for 1 week in a climate chamber with a day/night cycle consisting of 16 h light at 20°C and 8 h dark at 16°C. Seedlings were potted in sterile potting soil and grown for 21 days in the greenhouse under standard conditions (day length of 16 h, temperature of 22°C day/18°C night, and 60% humidity), where they were watered with 25 mL every 2 days.
After 21 days in the greenhouse, plants were mechanically inoculated with combinations of CMV, AMV, and PVY. Treatments were assigned to plants randomly using MS Excel. Inoculations were done with a varying number of replicates (n = 10–20) to ensure sufficient replicates remained given that inoculations are not 100% successful (Table S3). Briefly, infectious plant material from AMV, CMV, and PVY was homogenized in a phosphate inoculation buffer (composed of a 49:51 mix of two solutions: 1.362 g KH2PO4 dissolved in 1 L deionized water and 1.781 g Na2HPO4·2H2O dissolved in 1 L deionized water, respectively; 0.01 M, pH 7) (54) and stored in separate tubes. Mixed infection master mixes were made by adding equal amounts of each virus inoculum to a new tube. The youngest fully expanded leaf was dusted with carborundum powder, after which 30 μL of inoculum (either one of the prepared mixes or an inoculation buffer in the case of mock inoculation) was pipetted onto the leaf and gently rubbed. Plants were rinsed with water and grown in the greenhouse for 14 days under standard conditions (above), with 25 mL of water provided every other day.
Plants were harvested at 14 dpi. At the time of harvest, plants showed five–seven true leaves. Plant height (cm) and fresh weight (g) of total aboveground biomass were measured, and viral symptoms were scored. Leaf discs from each plant were collected for diagnostic testing of the presence of CMV and PVY using a 1.7-mL Eppendorf tube by closing the tube with one of the young leaves between the lid and the tube itself. The remaining aboveground biomass was stored at −80°C until further processing.
RNA extraction, diagnostics, and PCR
RNA was extracted from the total aboveground biomass of each plant using the Zymo direct-zol RNA mini-prep kit, following the manufacturer’s protocol. As part of the extraction procedure, on-column DNase treatment was performed, and then the RNA was quantified with a Nanodrop spectrophotometer. For each sample, 500 ng of total RNA was converted into cDNA using the iScript Reverse Transcription Supermix for RT-qPCR (BioRad), which uses a combination of random primers and oligo(dT).
Infection status for each plant was determined using either a lateral flow immunochromatography assay (BioReba Agristrip) for CMV and PVY or an RT-PCR for AMV using primers P3 and P4 (55) targeting the AMV coat protein gene. Cycling consisted of one initial cycle of 5 m at 94°C, followed by 33 cycles of 30 s at 94°C, 30 s at 58°C, 1 m at 72°C, and lastly a final extension step of 7 m at 72°C. Infection status was determined by gel electrophoresis (1% agarose 0.5 M TBE gel).
Cucumber mosaic virus genome formula estimation
The genome formula of CMV was quantified using RT-qPCR. The accumulation of each viral genome segment was quantified using an SYBR Green-based assay (iQ SYBR Green Supermix, BioRad). Cycling consisted of one initial cycle of 3 m at 95°C, followed by 40 cycles of 10 s at 95°C,30 s at 60°C, 10 s at 95°C, and, lastly, a melt curve was performed using 0.5°C increments ranging from 65°C to 95°C.
Viral titer quantification relative to Nicotiana tabacum reference genes
For each treatment, we proceeded with four randomly selected infected plants for analysis of the viral titer. Random selection was done using a random number generator. Accumulation of each viral genome segment was quantified using an SYBR Green-based RT-qPCR (iQ SYBR Green Supermix, BioRad). AMV, PVY, and CMV accumulation were estimated relative to host reference transcripts. For all RT-qPCRs, cycling consisted of one initial cycle of 3 m at 95°C, followed by 40 cycles of 10 s at 95°C and 30 s at 60°C, 10 s at 95°C, and lastly, a melt curve was performed using 0.5°C increments ranging from 65°C to 95°C. Reaction efficiencies for primers were measured—using a dilution series of each target—and determined to be comparable (>98% efficiency). An overview of the primers used can be found in Tables S2 and S4. Accumulation was estimated using the ΔΔCt method (56), where the accumulation of each segment is calculated relative to the geometric mean of accumulation for the L25 ribosomal, β-Tubulin, and Ntubc2 transcripts (36).
Statistical analysis of empirical data
To analyze the genome formula data, we determined the pairwise distance (d) between two observed sets of genome formula values for samples a and b using the vegdist function such that:
where j is the number (1-3) of the CMV genome segment. Here, da,b simply correspond to the distance between the two observations in three-dimensional genome formula space. We then performed a PERMANOVA using the adonis function and a PERMDISP2 using the permutest.betadisper function, permuting the data 104 times for both tests. All pairwise comparisons between treatments (i.e., viruses present) were made for the PERMDISP2 procedure, with a Holm-Bonferroni correction for multiple comparisons. The vegdist, adonis, and permutest.betadisper functions are part of the R package vegan (35).
To analyze the titer, RT-qPCR-based accumulation values were summed and log-transformed before further statistical analysis. To test the effect of mixed infections on the titer of CMV, we performed an ANOVA. We ensured ANOVA assumptions were met by performing a Shapiro-Wilk test for normality. We tested and confirmed the homogeneity of variance using Bartlett’s test. Pairwise differences in CMV accumulation between treatment groups were tested using Dunnett’s test with a single CMV infection as the control group.
For the analysis of plant phenotype (fresh weight, g), an insignificant result for Levene’s test for equality of variances indicated that data transformation was not needed. Pairwise comparisons of plant fresh weight (g) were performed using a t-test, and p-values were adjusted for multiple testing using the Holm-Bonferroni correction. Descriptive figures of plant phenotypes can be found in Figure S4. All statistical analyses were performed in R version R-4.2.1 (57).
Models of mixed viral infections
Using simulation models, we explored the three mechanistic hypotheses described in the introduction: co-opting of viral gene products (Scenario 1), co-infection exclusion (Scenario 2), and co-infection exclusion through niche differentiation (Scenario 3). The overall goal of our modeling approach was to determine whether there is a parameter space in which mixed infections affect the genome formula and to highlight possible mechanisms by which this might occur. Our model had some key assumptions: (i) the mean total number of infecting virus particles of each virus (MOI) was fixed, while realizations of this number followed a Poisson distribution over cells; (ii) there was perfect mixing of the virus particles produced by infected cells after each round of infection; (iii) the relationship between the log of the genome formula and virus particle yield followed the probability density function of the Normal distribution; and (iv) there were no effects of the multipartite virus on the monopartite virus.
Models were developed by adapting an existing simulation model of the evolution of the genome formula for a bipartite virus when in competition with a monopartite virus (10). We first provide a brief description of the model, justify the parameter values used here for all scenarios, and finally describe how the model was adapted to explore the three scenarios put forward here. The model allowed the number of invading virus particles to vary stochastically over a population of cells following the means of λ, λ1, and λ2 for the total number of multipartite virus particles, segment 1 virus particles, and segment 2 virus particles, respectively. This bottleneck generated variation in the ratio of genome regions (i.e., the two segments of the multipartite virus) present in a cell (r) where r = f1/f2, where f is the relative frequency of a genome segment. The virus particle yield of each infected cell followed the probability density function of a normal distribution, such that exp[-(log10r-μ)2/2σ2], with a mean μ and variance σ2. The highest virus particle yield was obtained when r = μ, whereas the variance indicated how sensitive virus yield is to changes in the genome formula. The yield was then distributed over the two virus particle types by the frequencies f1 and f2 meaning that there was no within-cell competition between the two genome segments. After each round in cinf effectively infected cells, all virus particles were pooled to determine the frequencies f1 and f2 used in the inoculum for the next round of infection.
For all simulations described here, we used the following parameter settings. The total MOI of the multipartite virus was set to an intermediate value (λ = 2), to ensure rapid genome formula adaptation of the multipartite virus. We set the number of effectively infected cells as cinf = 1,000, although note that in Scenarios 2 and 3, this became (approximately) the maximum number of cells infected by the multipartite virus. The initial frequency of the two multipartite genome segments in the inoculum was balanced, so . We set σ2 = 0.01, therefore choosing conditions under which virus yield was highly sensitive to changes in the ratio of genome products: a parameter range in which multipartite viruses are likely to outcompete their cognate monopartite viruses (10). The only model parameter that varied across the different scenarios was μ. Five rounds of infection were carried out in all cases, and we performed 1,000 independent simulations for each set of conditions. In Scenarios 2 and 3, the extinction of viral populations was possible. In these cases, we monitored the number of extinctions, to ensure that a representative number of surviving populations were retained.
Scenario 1 allowed some of the gene products of a monopartite virus to be co-opted by the multipartite virus. To introduce the monopartite virus, we allowed the number of infecting virus particles to follow a Poisson distribution with mean λvirus2 over all cells. We assumed that the monopartite virus introduced a viral gene product that was a perfect substitute for the gene product on multipartite segment 2, with expression levels equivalent to those per segment copy number for the multipartite virus. Based on these assumptions, . To simplify the simulations and focus on the effects of the second virus on the multipartite virus’s genome formula, we fixed λvirus2 to a given value and allowed only the frequencies f1 and f2 to carry over to the next round of infection. In other words, the second virus infected a stochastic number of cells in each round of infection, irrespective of the infection outcomes for both viruses in the previous round of infection. It is important to note that the presence of the second virus did not simply supplement gene products, it could displace r from its optimal value leading to the selection of lower values for f2. The parameter values used were μ = 0 (i.e., balanced optimal genome formula) and .
Scenario 2 introduced a monopartite virus that exhibited co-infection exclusion, blocking the multipartite virus from generating any virus particle yield in co-infected cells. There were no further interactions between the viruses. The number of infecting virus particles again followed a Poisson distribution with mean λvirus2 over all cells. The parameter values used were μ = 1 (i.e., unbalanced optimal genome formula) and λvirus2 = {0, 0.01, 0.03, 0.1, 0.3, 1, 3, 5, 5.5, 6}. Unlike in the original model and Scenario 1, here we determined the total number of cells to simulate to have cinf effectively infected cells in the first round of infection without taking into account the action of the monopartite virus, and then held this number constant over all values of λvirus2. In effect, the monopartite virus, therefore, decreased the number of effectively infected cells. The λvirus2 values were chosen so that the number of effectively infected cells became small, but not all replicate populations went extinct.
Scenario 3 built on Scenario 2, but in this case, there were two subpopulations of cells. These two subpopulations had different values of μ, μs1, and μs2, and the monopartite virus could infect only the s1 subpopulation. Half of the cells were assigned to each subpopulation. The parameter values used were μs1 = -0.5, μs2 = 0.5, and λvirus2 = {0, 10-2, 10-15, 10-1, (…), 102}.
All simulation code was implemented in R 4.2.1 (57).
Data availability statement
The original contributions presented in the study are included in the article/Supplementary Materials. Further inquiries can be directed to the corresponding authors.
Author contributions
DB: Conceptualization, Methodology, Investigation, Formal analysis, Visualization, Writing—original draft. MV: Methodology, Investigation, Formal analysis, Writing—review, and editing. MJ: Methodology, Writing—review and editing. CM: Formal analysis; Writing—review and editing. RV: Resources, Writing—review and editing. MZ: Supervision, Formal analysis, Funding acquisition, Writing—original draft; Writing—review and editing. All authors contributed to the article and approved the submitted version.
Funding
MJ and MZ were supported by a VIDI grant from The Netherlands Organization for Scientific Research (NWO 016.Vidi.171.061) to MZ. CM was supported by MSU AgBioResearch.
Acknowledgments
We thank Maria Hundscheid for rearing the plants used in the experiment prior to inoculation.
Conflict of interest
The authors declare that the research was conducted in the absence of any commercial or financial relationships that could be construed as a potential conflict of interest.
MZ declares that they were an editorial board member of Frontiers at the time of submission.
Publisher’s note
All claims expressed in this article are solely those of the authors and do not necessarily represent those of their affiliated organizations, or those of the publisher, the editors and the reviewers. Any product that may be evaluated in this article, or claim that may be made by its manufacturer, is not guaranteed or endorsed by the publisher.
Supplementary material
The Supplementary Material for this article can be found online at: https://www.frontiersin.org/articles/10.3389/fviro.2023.1225818/full#supplementary-material
Abbreviations
AMV, alfalfa mosaic virus; CMV, cucumber mosaic virus; FBNSV, faba bean necrotic stunt virus; MOI, multiplicity of infection; PVY, potato virus Y; RT, reverse transcription; (RT)-qPCR, reverse transcription quantitative polymerase chain reaction.
References
1. Fulton RW. The effect of dilution on necrotic ringspot virus infectivity and the enhancement of infectivity by noninfective virus. Virology (1962) 18:477–85. doi: 10.1016/0042-6822(62)90038-7
2. Iranzo J, Manrubia SC. Evolutionary dynamics of genome segmentation in multipartite viruses. Proc Biol Sci (2012) 279:3812–9. doi: 10.1098/rspb.2012.1086
3. Sicard A, Yvon M, Timchenko T, Gronenborn B, Michalakis Y, Gutierrez S, et al. Gene copy number is differentially regulated in a multipartite virus. Nat Commun (2013) 4:2248. doi: 10.1038/ncomms3248
4. Wu B, Zwart MP, Sánchez-Navarro JA, Elena SF. Within-host evolution of segments ratio for the tripartite genome of alfalfa mosaic virus. Sci Rep (2017) 7:5004. doi: 10.1038/s41598-017-05335-8
5. Sicard A, Pirolles E, Gallet R, Vernerey M-S, Yvon M, Urbino C, et al. A multicellular way of life for a multipartite virus. Elife (2019) 8:e43599. doi: 10.7554/eLife.43599
6. Obrępalska-Stęplowska A, Renaut J, Planchon S, Przybylska A, Wieczorek P, Barylski J, et al. Effect of temperature on the pathogenesis, accumulation of viral and satellite RNAs and on plant proteome in peanut stunt virus and satellite RNA-infected plants. Front Plant Sci (2015) 6:903. doi: 10.3389/fpls.2015.00903
7. Mansourpour M, Gallet R, Abbasi A, Blanc S, Dizadji A, Zeddam J-L. Effects of an alphasatellite on the life cycle of the nanovirus faba bean necrotic yellows virus. J Virol (2022) 96:e0138821. doi: 10.1128/JVI.01388-21
8. Sicard A, Michalakis Y, Gutiérrez S, Blanc S. The strange lifestyle of multipartite viruses. PloS Pathog (2016) 12:e1005819. doi: 10.1371/journal.ppat.1005819
9. Gallet R, Di Mattia J, Ravel S, Zeddam J-L, Vitalis R, Michalakis Y, et al. Gene copy number variations at the within-host population level modulate gene expression in a multipartite virus. Virus Evol (2022) 8:veac058. doi: 10.1093/ve/veac058
10. Zwart MP, Elena SF. Modeling multipartite virus evolution: the genome formula facilitates rapid adaptation to heterogeneous environments†. Virus Evol (2020) 6:veaa022. doi: 10.1093/ve/veaa022
11. Wang Y, Gaba V, Yang J, Palukaitis P, Gal-On A. Characterization of synergy between Cucumber mosaic virus and potyviruses in cucurbit hosts. Phytopathology (2002) 92:51–8. doi: 10.1094/PHYTO.2002.92.1.51
12. Moreno AB, López-Moya JJ. When viruses play team sports: Mixed infections in plants. Phytopathology (2020) 110:29–48. doi: 10.1094/PHYTO-07-19-0250-FI
13. Alcaide C, Rabadán MP, Moreno-Pérez MG, Gómez P. Chapter Five - Implications of mixed viral infections on plant disease ecology and evolution. In: Kielian M, Mettenleiter TC, Roossinck MJ, editors. Advances in virus research. (Amsterdam: Elsevier) (2020). p. 145–69.
14. Seabloom EW, Hosseini PR, Power AG, Borer ET. Diversity and composition of viral communities: coinfection of barley and cereal yellow dwarf viruses in California grasslands. Am Nat (2009) 173:E79–98. doi: 10.1086/596529
15. Kendig AE, Borer ET, Mitchell CE, Power AG, Seabloom EW. Characteristics and drivers of plant virus community spatial patterns in US west coast grasslands. Oikos (2017) 126:1281–90. doi: 10.1111/oik.04178
16. Rochow WF, Ross AF. Virus multiplication in plants doubly infected by potato viruses X and Y. Virology (1955) 1:10–27. doi: 10.1016/0042-6822(55)90003-9
17. Vance VB. Replication of potato virus X RNA is altered in coinfections with potato virus Y. Virology (1991) 182:486–94. doi: 10.1016/0042-6822(91)90589-4
18. Palukaitis P, Kaplan IB. Synergy of virus accumulation and pathology in transgenic plants expressing viral sequences. In: Virus-Resistant transgenic plants: Potential ecological impact. Berlin Heidelberg: Springer (1997). p. 77–84.
19. Pruss G, Ge X, Shi XM, Carrington JC, Bowman Vance V. Plant viral synergism: the potyviral genome encodes a broad-range pathogenicity enhancer that transactivates replication of heterologous viruses. Plant Cell (1997) 9:859–68. doi: 10.1105/tpc.9.6.859
20. Wege C, Siegmund D. Synergism of a DNA and an RNA virus: enhanced tissue infiltration of the begomovirus Abutilon mosaic virus (AbMV) mediated by Cucumber mosaic virus (CMV). Virology (2007) 357:10–28. doi: 10.1016/j.virol.2006.07.043
21. Mascia T, Cillo F, Fanelli V, Finetti-Sialer MM, De Stradis A, Palukaitis P, et al. Characterization of the interactions between Cucumber mosaic virus and Potato virus Y in mixed infections in tomato. Mol Plant Microbe Interact (2010) 23:1514–24. doi: 10.1094/MPMI-03-10-0064
22. Wintermantel WM, Cortez AA, Anchieta AG, Gulati-Sakhuja A, Hladky LL. Co-infection by two criniviruses alters accumulation of each virus in a host-specific manner and influences efficiency of virus transmission. Phytopathology (2008) 98:1340–5. doi: 10.1094/PHYTO-98-12-1340
23. Alizon S, de Roode JC, Michalakis Y. Multiple infections and the evolution of virulence. Ecol Lett (2013) 16:556–67. doi: 10.1111/ele.12076
24. Salaman RN. Protective inoculation against a plant virus. Nature (1933) 131:468. doi: 10.1038/131468a0
25. Gutiérrez S, Pirolles E, Yvon M, Baecker V, Michalakis Y, Blanc S. The multiplicity of cellular infection changes depending on the route of cell infection in a plant virus. J Virol (2015) 89:9665–75. doi: 10.1128/JVI.00537-15
26. Dietrich C, Maiss E. Fluorescent labelling reveals spatial separation of potyvirus populations in mixed infected Nicotiana benthamiana plants. J Gen Virol (2003) 84:2871–6. doi: 10.1099/vir.0.19245-0
27. Syller J. Facilitative and antagonistic interactions between plant viruses in mixed infections. Mol Plant Pathol (2012) 13:204–16. doi: 10.1111/j.1364-3703.2011.00734.x
28. Luria SE, Delbrück M. Mutations of bacteria from virus sensitivity to virus resistance. Genetics (1943) 28:491–511. doi: 10.1093/genetics/28.6.491
29. Choi SK, Yoon JY, Ryu KH, Choi JK, Palukaitis P, Park WM. Systemic movement of a movement-deficient strain of Cucumber mosaic virus in zucchini squash is facilitated by a cucurbit-infecting potyvirus. J Gen Virol (2002) 83:3173–8. doi: 10.1099/0022-1317-83-12-3173
30. Holmes JC. Effects of concurrent infections on Hymenolepis diminuta (Cestoda) and Moniliformis dubius (Acanthocephala). I. General effects and comparison with crowding. J Parasitol (1961) 47:209–16. doi: 10.2307/3275291
31. Colwell RK, Fuentes ER. Experimental studies of the niche. Annu Rev Ecol Syst (1975) 6:281–310. doi: 10.1146/annurev.es.06.110175.001433
32. He L, Wang Q, Gu Z, Liao Q, Palukaitis P, Du Z. A conserved RNA structure is essential for a satellite RNA-mediated inhibition of helper virus accumulation. Nucleic Acids Res (2019) 47:8255–71. doi: 10.1093/nar/gkz564
33. Boezen D, Johnson ML, Grum-Grzhimaylo AA, van der Vlugt RA, Zwart MP. Evaluation of sequencing and PCR-based methods for the quantification of the viral genome formula. Virus Res (2023) 326:199064. doi: 10.1016/j.virusres.2023.199064
34. Anderson MJ. A new method for non-parametric multivariate analysis of variance. Austral Ecol (2001) 26:32–46. doi: 10.1111/j.1442-9993.2001.01070.pp.x
35. Oksanen J, Simpson GL, Blanchet FG, Kindt R, Legendre P, Minchin PR, et al. vegan: Community ecology package (2022). Available at: https://CRAN.R-project.org/package=vegan.
36. Schmidt GW, Delaney SK. Stable internal reference genes for norMalization of real-time RT-PCR in tobacco (Nicotiana tabacum) during development and abiotic stress. Mol Genet Genomics (2010) 283:233–41. doi: 10.1007/s00438-010-0511-1
37. Moreau Y, Gil P, Exbrayat A, Rakotoarivony I, Bréard E, Sailleau C, et al. The genome segments of bluetongue virus differ in copy number in a host-specific manner. J Virol (2020) 95:e01834-20. doi: 10.1128/JVI.01834-20
38. Fattouh FA. Double infection of a cucurbit host by zucchini yellow mosaic virus and cucumber mosaic virus. Plant Pathol J (2003) 2:85–90. doi: 10.3923/ppj.2003.85.90
39. Palukaitis P, García-Arenal F. Cucumber mosaic virus. St. Paul, MN: The American Phytopathological Society Press (2019).
40. Kennedy GG, Sharpee W, Jacobson AL, Wambugu M, Mware B, Hanley-Bowdoin L. Genome segment ratios change during whitefly transmission of two bipartite cassava mosaic begomoviruses. Sci Rep (2023) 13:10059. doi: 10.1038/s41598-023-37278-8
41. Ali A, Roossinck MJ. A simple technique for separation of Cowpea chlorotic mottle virus from Cucumber mosaic virus in natural mixed infections. J Virol Methods (2008) 153:163–7. doi: 10.1016/j.jviromet.2008.07.023
42. Keeling MJ, Rohani P. Modeling infectious diseases in humans and animals. Princeton, NJ: Princeton University Press (2008).
43. Jorgenson SE. Chapter 1 - introduction: an overview. In: Developments in environmental modeling, vol. 28. (Amsterdam: Elsevier). (2016). p. 1–11.
44. Zwart MP, Elena SF. Matters of size: Genetic bottlenecks in virus infection and their potential impact on evolution. Annu Rev Virol (2015) 2:161-79. doi: 10.1146/annurev-virology-100114-055135
45. Cillo F, Roberts IM, Palukaitis P. In situ localization and tissue distribution of the replication-associated proteins of Cucumber mosaic virus in tobacco and cucumber. J Virol (2002) 76:10654–64. doi: 10.1128/JVI.76.21.10654-10664.2002
46. Van Der Heijden MW, Carette JE, Reinhoud PJ, Haegi A, Bol JF. Alfalfa mosaic virus replicase proteins P1 and P2 interact and colocalize at the vacuolar membrane. J Virol (2001) 75:1879–87. doi: 10.1128/JVI.75.4.1879-1887.2001
47. Revers F, García JA. Molecular biology of potyviruses. Adv Virus Res (2015) 92:101–99. doi: 10.1016/bs.aivir.2014.11.006
48. Takeshita M, Shigemune N, Kikuhara K, Furuya N, Takanami Y. Spatial analysis for exclusive interactions between subgroups I and II of Cucumber mosaic virus in cowpea. Virology (2004) 328:45–51. doi: 10.1016/j.virol.2004.06.046
49. Sánchez-Navarro JA, Zwart MP, Elena SF. Effects of the number of genome segments on primary and systemic infections with a multipartite plant RNA virus. J Virol (2013) 87:10805–15. doi: 10.1128/JVI.01402-13
50. Alazem M, Lin N-S. Roles of plant hormones in the regulation of host-virus interactions. Mol Plant Pathol (2015) 16:529–40. doi: 10.1111/mpp.12204
51. Voinnet O, Pinto YM, Baulcombe DC. Suppression of gene silencing: a general strategy used by diverse DNA and RNA viruses of plants. Proc Natl Acad Sci U. S. A. (1999) 96:14147–52. doi: 10.1073/pnas.96.24.14147
52. Jacquemond M, Lot H. L’ARN satellite du virus de la mosaïque du concombre I. – Comparaison de l’aptitude à induire la nécrose de la tomate d’ARN satellites isolés de plusieurs souches du virus. Agronomie (1981) 1:927–32. doi: 10.1051/agro:19811015
53. Hagedorn DJ, Hanson EW. A strain of alfalfa mosaic virus severe on Trifolium pratense and Melilotus alba. Phytopathology (1963) 53:188–92.
54. Hull R. Mechanical inoculation of plant viruses. Curr Protoc Microbiol (2009) 16:16B.6. doi: 10.1002/9780471729259.mc16b06s13
55. Navarre DA, Shakya R, Holden J, Crosslin JM. LC-MS analysis of phenolic compounds in tubers showing zebra chip symptoms. Am J Potato Res (2009) 86:88–95. doi: 10.1007/s12230-008-9060-0
56. Livak KJ, Schmittgen TD. Analysis of relative gene expression data using real-time quantitative PCR and the 2(-Delta C(T)) Method. Methods (2001) 25:402–8. doi: 10.1006/meth.2001.1262
57. R Core Team. R: A language and environment for statistical computing. Vienna, Austria: R Foundation for Statistical Computing (2022). Available at: https://www.R-project.org/.
Keywords: multipartite virus, genome formula, titer, mixed infection, cucumber mosaic virus, alfalfa mosaic virus, potato virus Y
Citation: Boezen D, Vermeulen M, Johnson ML, van der Vlugt RAA, Malmstrom CM and Zwart MP (2023) Mixed viral infection constrains the genome formula of multipartite cucumber mosaic virus. Front. Virol. 3:1225818. doi: 10.3389/fviro.2023.1225818
Received: 19 May 2023; Accepted: 07 August 2023;
Published: 07 September 2023.
Edited by:
Selma Gago-Zachert, Martin Luther University of Halle-Wittenberg, GermanyReviewed by:
Juan Jose Lopez-Moya, Spanish National Research Council (CSIC), SpainIsrael Pagan, Polytechnic University of Madrid, Spain
Copyright © 2023 Boezen, Vermeulen, Johnson, van der Vlugt, Malmstrom and Zwart. This is an open-access article distributed under the terms of the Creative Commons Attribution License (CC BY). The use, distribution or reproduction in other forums is permitted, provided the original author(s) and the copyright owner(s) are credited and that the original publication in this journal is cited, in accordance with accepted academic practice. No use, distribution or reproduction is permitted which does not comply with these terms.
*Correspondence: Dieke Boezen, d.boezen@nioo.knaw.nl; Mark P. Zwart, m.zwart@nioo.knaw.nl
†Present address: Maritta Vermeulen, HAN University of Applied Science, Nijmegen, the Netherlands