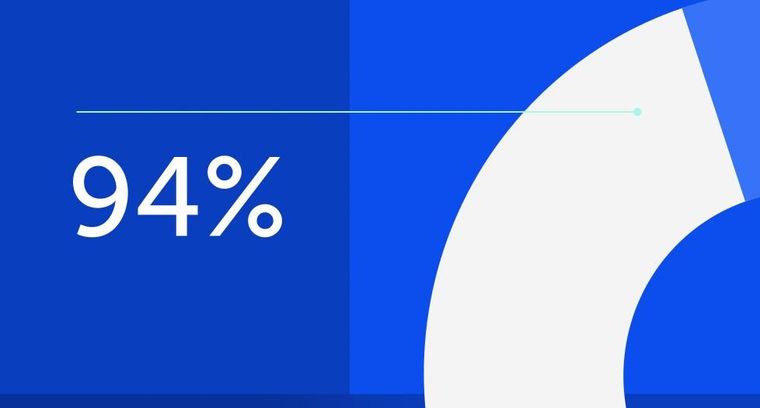
94% of researchers rate our articles as excellent or good
Learn more about the work of our research integrity team to safeguard the quality of each article we publish.
Find out more
BRIEF RESEARCH REPORT article
Front. Virol., 26 April 2023
Sec. Bioinformatic and Predictive Virology
Volume 3 - 2023 | https://doi.org/10.3389/fviro.2023.1172027
This article is part of the Research TopicMethods in Bioinformatic and Predictive VirologyView all 4 articles
The SARS-CoV-2 variant XBB.1.5 is of concern as it has high transmissibility. XBB.1.5 currently accounts for upwards of 30% of new infections in the United States. One year after our group published the predicted structure of the Omicron (B.1.1.529) variant’s receptor binding domain (RBD) and antibody binding affinity, we return to investigate the new mutations seen in XBB.1.5 which is a descendant of Omicron. Using in silico modeling approaches against newer neutralizing antibodies that are shown effective against B.1.1.529, we predict the immune consequences of XBB.1.5’s mutations and show that there is no statistically significant difference in overall antibody evasion when comparing to the B.1.1.529 and other related variants (e.g., BJ.1 andBM.1.1.1). However, noticeable changes in antibody binding affinity were seen due to specific amino acid changes of interest in the newer variants.
In late November 2022, the United States Centers for Diseases Control stated that they began tracking a new SARS-CoV-2 variant known as XBB.1.5. At that time, XBB.1.5 was responsible for around 3% of all infections. Since then, XBB.1.5 has grown to represent 30% of all infections by January 2023 (1, 2). XBB.1.5 is characterized by 40 mutations in the Spike protein, 22 of which are in the receptor binding domain (RBD) (3). The highly prevalent mutations in the RBD are shown in Table 1 below.
A health concern is that XBB.1.5 may evade existing antibodies derived from therapeutics, vaccination, and or previous Omicron (B.1.1.529) infection. It has been proposed that XBB.1.5 is a recombinant strain of the virus from BJ.1 and BM.1.1.1 as portions of the mutated Spike protein appear to be from each parent strain (4, 5). However, alternative hypotheses such as convergent evolution may also explain the similarity of portions of XBB.1.5’s mutated regions to those seen in other variants (6, 7). In our previous work on the prediction of the receptor binding domain (RBD) structure of the Omicron variant, our process provided robust predictions, having a root mean square deviation of atomic positions (RMSD) of 0.574Å between the predicted and empirically derived Omicron RBD structure (PDB: 7t9j) (8). Furthermore, our previous study proved useful as a predictive gauge of antibody efficacy several weeks prior to when empirical validations of the Omicron-antibody binding changes could be performed (9).
In this study, we use the methodology in our previous work to investigate XBB.1.5 and related variants (B.1.1.529, BJ.1, and BM.1.1) and expand the antibodies of interest to include more recently developed anti-Omicron antibodies. At the molecular level, we further elucidate the antibody binding and interfacing residues between three commercially-available antibodies: bamlanivimab, bebtelovimab, and tixagevimab and the RBD structure of each SARS-CoV-2 variant. We consider in vitro studies with existing antibodies and older variants to predict performance on XBB.1.5.
Given the four SARS-CoV-2 variants (B.1.1.529, BJ.1, BM.1.1, and XBB.1.5) and 10 antibodies, 40 in silico docking experiments were performed. As shown in Figure 1, the mean performance of the included neutralizing antibodies is similar across the four variants, with XBB.1.5 binding results being congruent to that of B.1.1.529. Furthermore, across the 10 antibodies tested, the binding affinities seen in XBB.1.5 are not weakened compared to B.1.1.529, nor are the differences statistically significant.
Figure 1 Boxplots of antibody binding performance by SARS-CoV-2 variant. Wilcoxon p-values are shown to assess the statistical significance of the differences between overall variant-antibody performance. (A) represents the composite HADDOCK score (lower is better) and is defined as 1.0 van der Waals energy + 0.2 electrostatic energy + 1.0 desolvation energy + 0.1 restraints energy. (B) represents the van der Waals intermolecular energy (lower is better). (C) represents the electrostatic intermolecular energy (lower is better). (D) represents the desolvation energy (lower is better). (E) represents the buried surface area (higher is better). (F) represents the predicted change in Gibbs energy from PRODIGY (lower is better).
When assessing overall antibody performance against BJ.1, we see weakened Van der Waals energies as compared to the other three variants. This is depicted in the Uniform Manifold Approximation and Projection (UMAP) in Figure 2 where the position of some antibodies on the BJ.1 UMAP are increased (leading to decreased binding affinity). However, desolvation energies and the buried surface areas are slightly improved overall when comparing BJ.1 results to the other three variant results.
Figure 2 UMAP scatter plot of antibody binding affinity metrics with a variant in each quadrant. Note that a higher UMAP value is likely indicative of worse performance.
Note that the comparisons shown in Figure 1 represent the singular best HADDOCK output for each antibody-RBD pair. Further investigations using PRODIGY (10) are reported for the top 4 complexes per antibody-RBD pair in Supplementary Materials, Figure 9.
While there are instances of overall antibody performance increasing or decreasing in singular comparisons, we do not see an overarching pattern that indicates that XBB.1.5 has evolved antibody evasion over B.1.1.529 (or BJ.1 and BM.1.1.1). In other words, XBB.1.5 does not appear to have evolved past current antibody defenses, specifically concerning the ten antibody structures tested in this study.
Of the ten antibodies tested in this study, we focus on the structural bases in which the antibodies LY-CoV555, LY-CoV1404, and AZD8895 work. The neutralization mechanisms of three antibodies have been extensively studied. These three antibodies have been available as therapeutics for treatment against COVID-19 infections (either currently or previously in the United States under Emergency Use Authorization) (11–13).
We see a consistent interaction between Bamlanivimab (LY-CoV555) and the variant RBDs at R/Q493. This differs from (14), which states that F490 and S494 in the RBD are the interfacing residues in this region.
The PyMOL structural visualizations of the potential interaction residues coincides with the overall metrics returned from the HADDOCK analyses shown in Table 2. LY-CoV555 shows the worst overall performance against BJ.1, while the performance against B.1.1.529, BM.1.1.1, and XBB.1.5 are quite similar. Though not shown in Figure 3, the latter three complexes show a higher number of interfacing residues overall than in BJ.1, thus supporting the reported affinity metrics.
Figure 3 Interfacing residues of interest from the LY-CoV555 antibody (in pale pink) against the four RBD structures.
Westendorf et al. (15) demonstrated that Bebtelovimab (LY-CoV1404) antibody binding affinity may not be affected by RBD mutations at E484, F490, Q493. Shown in Figure 4, we see consistent interactions from this antibody across all four variants around most of these positions despite mutations.
Figure 4 Interfacing residues of interest from the LY-CoV1404 antibody (in light purple) against the four RBD structures.
These findings for LY-CoV1404 are congruent with the reported affinity metrics from the HADDOCK analyses shown in Table 3. Overall, HADDOCK scores are stable across the four variant complexes. The antibody LY-CoV1494 is predicted to have a slightly weaker interaction with XBB.1.5 than the other three variants.
For tixagevimab (AZD8895), as reported in Dong et al. (16), there is a critical contact residue at F486 on the RBD. This residue is interfaced in B.1.1.529, BJ.1, and BM.1.1.1. However, the F486 residue is mutated to proline at this position in XBB.1.5, though interactions from the antibody to the adjacent RBD residues at G485 and N487 of the RBD still occur. See Figure 5.
Figure 5 Interfacing residues of interest from the AZD8895 antibody (in bright green) against the four RBD structures.
From the HADDOCK metrics shown in Table 4, this F486P mutation increases the binding affinity with the AZD8895, especially in terms of Van der Waals and electrostatic energies. Interfacing residues are abundant across all four of these AZD8895-RBD complexes (in addition to those shown in Figure 5), thus providing additional agreement to the strong affinity metrics reported by HADDOCK.
In addition, there is considerable agreement between the interfacing residues detected from the HADDOCK complexes with the active and passive residues predicted by CPORT (17), listed in the Supplementary Materials, Table 7 and Figure 8.
Our in silico modeling approach includes the curation or generation of the RBD structures for four SARS-CoV-2 variants and ten neutralizing antibody structures. Next, each antibody structure was docked against each RBD structure and binding affinity metrics were collected for comparison.
The Spike protein structure of the SARS-CoV-2 Omicron variant (B.1.1.529) was obtained from Protein Data Bank (PDB: PDB: 7t9j) (18). This structure was then trimmed to the RBD residues 339-528.
RBD sequences for BJ.1, BM.1.1.1, and XBB.1.5 were derived from representative samples found on GISAID:
BJ.1: EPI_ISL_16182897
BM.1.1: EPI_ISL_15658180
XBB.1.5: EPI_ISL_16505393
The RBD structures of BJ.1, BM.1.1.1, and XBB.1.5 were predicted with these sequences using AlphaFold2 (ColabFold-mmseqs2 version) (19, 20). Next, the most confident structure of each was used in docking analyses.
Representative antibody structures were collected from various Protein Data Bank entries ranging from antibodies derived from infected patients (or patients with breakthrough infections) or commercially available antibodies used in the treatment of COVID-19. See Table 5.
Only a fragment antigen-binding (Fab) region of the antibodies was used in the docking analyses.
To prepare the Fab structures, we renumbered residues according to HADDOCK’s requirements such that there are no overlapping residue IDs between the heavy and light chains of the “Fab's .PDB file.” Residues in the Fab structures’ complementarity-determining regions (CDRs) were selected as “active residues” for the docking analyses.
Residues in the S1 position of the RBD were selected as the “active residues” of the RBD structures. Since all of the input RBD .PDB files were renumbered to numbers 339-528, all of the input RBD files share the same “active residue” numbers.
Each of the ten antibody structures where docked against each of the four RBD structures using HADDOCK v2.4, a biomolecular modeling software that provides docking predictions for provided structures (26).
The HADDOCK system outputs multiple metrics for the predicted binding affinities and an output set of .PDB files containing the antibody docked against the RBD protein. PRODIGY, a tool to predict binding affinities using Gibbs energy, reported as G in Kcal/mol units), was also run on each of the complexes (10).
This process resulted in forty sets of docked structures. Each set contains many antibody-RBD complex conformations, from which we selected the top-performing structure for each antibody-RBD pair. We used this top-performing complex for subsequent structural investigations into interfacing residues and docking positions.
These analyses were performed on the antibody-RBD structure pairs shown in Figure 1. The multiple metrics were used to assess the overall binding affinity changes between SARS-CoV-2 variants across multiple representative antibodies.
Further, the docked Protein Data Bank Files (PDB) were manually reviewed using PyMOL (27) to search for interfacing residues and polar contacts between the RBD and Fab structures that may indicate neutralizing activity.
Building on our previous work (8) in studying Omicron’s structure, we have continued to demonstrate the utility of in silico modeling for predicting whether antibody binding affinity changes with the evolution of new SARS-CoV-2 variants. Given that in vitro assessment of protein structure and antibody binding experiments are costly and take an extended time, in silico computational modeling provides a more economical and faster method near or at empirical resolution. Our previous in silico results were confirmed via an empirical study reported by (9).
With computational modeling we rapidly predict the potential severity of a new variant and provides predictions on antibody binding affinity. These predictions inform public health considerations and provide a method of rational drug design based on expected therapeutic and vaccine (and booster) efficacy. Computational modeling can be used to rapidly infer the public health consequences of a new variant in terms of the loss of efficacy of antibodies, such as breakthrough infections and associated healthcare burden.
For XBB.1.5 specifically, there are residue mutations of interest that may affect antibody binding of the various S1 region-binding antibodies tested here. Comparing previous reports on older variants concerning the three main antibodies discussed here to our computational results shows strong agreement between previous empirical results and our new in silico predictions. Mutations in XBB.1.5 (and BJ.1 and BM.1.1.1) are not predicted to disrupt the overall tertiary structure of the RBD and are predicted to be very similar to B.1.1.529 (RMSD ≤ 0.78Å). See Supplementary Materials, Figure 6 and Table 6. Also, the interfacing residues of interest in the S1 binding site’s loop differ mainly in the side chain angle rather than overall loop conformation. See Supplementary Materials, Figure 7.
For Bamlanivimab (LY-CoV555), Jones et al. (14) reported that mutations at RBD positions V483, E484, F490, and S494 either decrease or eliminate binding and function. Our study does not refute this. However, our computational modeling indicates the S494 is interfaced in the BJ.1 and XBB.1.5 interactions with LY-CoV555. This result suggests that this residue does not decrease the antibody’s binding affinity. Also, R/Q493 forms a polar interaction with LY-CoV555 in B.1.1.529, BJ.1, and BM.1.1.1, but not XBB.1.5.
In Bebtelovimab (LY-CoV1404), Westendorf et al. (15) states that mutations at E484 may confer advantages in antibody evasion capabilities for the virus. This result is supported in our study as A484 in our four variants in this study, is only interfaced in our docked structures for B.1.1.529 and BM.1.1.1. We see G485 as an important interfacing residue, forming a polar contact with LY-CoV1404 with all four RBD variants.
Lastly, Dong et al. (16) reports that aromatic residues from AZD8895 CDR loops form a hydrophobic pocket with the RBD residues G485, F486, and N487. Note that in XBB.1.5 there is a F486P mutation and, interestingly, the adjacent residues (G485 and N487) are interfaced in our predicted complex. It is possible the proline at position 486 provides less steric hindrance than phenylalanine, thus allowing surrounding residue interaction. This result can be tested in future studies.
The increased binding affinity of XBB.1.5 for ACE2 may lead to increased transmissibility at the population level (28). The results here do not indicate that we can expect increased disease severity on an individual level for patients that avail themselves of therapeutics and vaccination.
The climb in cases of COVID-19 disease linked to XBB.1.5 indicates that XBB.1.5 could be a very serious subvariant of Omicron. While other studies are needed to assess transmissibility, virulence, pathogenicity, and other facets of viral severity and epidemiology, this study predicts that many current therapeutic and infection-derived antibodies provide antibody binding affinities similar to B.1.1.529 for XBB.1.5. Thus, the results indicate that the health care outcomes should be positive for the patients that avail themselves of vaccines and therapeutics.
This work estimates potential changes in antibody neutralization effects or antibody neutralizing affinity using in silico protein modeling and computational docking analyses. Given the computational and predictive nature of this study, empirical investigations are necessary to validate these findings. However, these computational approaches provide an economical, scalable, and rapid methodology to understand the severity of new viral variants while the empirical work is being completed. Also, while HADDOCK is considered state-of-the-art in terms of protein docking, there are other docking tools that could pose different results for the comparisons
While we tested 10 representative neutralizing antibody structures against four variants of SARS-CoV-2, there are many more neutralizing antibodies and antibody-variant RBD complexes to be assessed. In future work, we shall improve and automate our docking pipeline to enable to large-scale prediction of antibody binding affinity changes across any future SARS-CoV-2 variants of interest. Also, given the dynamic nature of protein structure conformations, alternative conformations may exist that show other polar contacts and antibody-RBD interfaces than those shown by the best performing HADDOCK complexes. All docking outputs and results, including those not shown in the body of this article, are available in the Supplementary Materials.
All code, data, results, docking parameters, and protein structure files can be found on GitHub at https://github.com/colbyford/SARS-CoV-2_XBB.1.5_Spike-RBD_Predictions. The Supplementary Material for this article can be found online at: https://github.com/colbyford/SARS-CoV-2_XBB.1.5_Spike RBD_Predictions/tree/main/supplementary_materials.
CF performed data preparation, antibody docking analyses, and comparative analyses. DJM, RW, and SY performed the antibody selection and genetic analyses. DJ, RW, and DJM provided an epidemiological review of the current SARS-CoV-2 variants and added biological context to the analysis results. All authors contributed to the article and approved the submitted version.
We acknowledge the following entities at the University of North Carolina at Charlotte: The Center for Computational Intelligence to Predict Health and Environmental Risks (CIPHER), North Carolina Research Center at Kannapolis, The Department of Bioinformatics and Genomics, The College of Computing and Informatics, and the University Research Computing group. For the GISAID sequences EPI_ISL_16505393, EPI_ISL_16182897, and EPI_ISL_15658180, we thank the UNC Charlotte Environmental Monitoring Laboratory, the National Institute of Biomedical Genomics in India, and the British Columbia Centre for Disease Control Public Health Laboratory in Vancouver, Canada, respectively.
Author CF is the owner of Tuple, LLC.
The remaining authors declare that the research was conducted in the absence of any commercial or financial relationships that could be construed as a potential conflict of interest.
All claims expressed in this article are solely those of the authors and do not necessarily represent those of their affiliated organizations, or those of the publisher, the editors and the reviewers. Any product that may be evaluated in this article, or claim that may be made by its manufacturer, is not guaranteed or endorsed by the publisher.
The Supplementary Material for this article can be found online at: https://github.com/colbyford/SARS-CoV-2_XBB.1.5_Spike-RBD_Predictions/tree/main/supplementary_materials
1. Callaway E. Coronavirus variant XBB.1.5 rises in the united states - is it a global threat? Nature (2023) 613(7943):222–3. Available at: https://www.nature.com/articles/d41586-023-00014-3.
2. Callaway E. Is coronavirus variant XBB.1.5 a global threat? Nature (2023) 613:222–3. doi: 10.1038/d41586-023-00014-3
3. Outbreak.info SARS-COV-2 data explorer - XBB.1.5 (2023). Available at: https://outbreak.info/compare-lineages?pango=XBB.1.5&gene=S&threshold=75&nthresh=1&sub=false&dark=false.
4. Karyakarte RP, Das R, Dudhate S, Agarasen J, Pillai P, Chandankhede P, et al. Clinical Characteristics and Outcomes of Laboratory-Confirmed SARS-CoV-2 Cases Infected With Omicron Subvariants and the XBB Recombinant Variant. Cureus (2023), 15(2): e35261. doi: 10.7759/cureus.35261
5. Lasrado N, Collier A-r, Miller J, Hachmann N, Liu J, Sciacca M, et al. Waning immunity against XBB.1.5 following bivalent mrna boosters. bioRxiv (2023), 2023–01. doi: 10.1101/2023.01.22.525079
6. Miller J, Hachmann NP, Collier A-rY, Lasrado N, Mazurek CR, Patio RC, et al. Substantial neutralization escape by SARS-CoV-2 omicron variants BQ.1.1 and XBB.1. New Engl J Med (2023) 388(7):662–4. doi: 10.1056/NEJMc2214314
7. Cao Y, Jian F, Wang J, Yu Y, Song W, Yisimayi A, et al. Imprinted SARS-CoV-2 humoral immunity induces convergent omicron RBD evolution. Nature (2023) 614(7948):521–9. doi: 10.1038/s41586-022-05644-7
8. Ford CT, Machado DJ, Janies DA. Predictions of the SARS-CoV-2 omicron variant (B.1.1.529) spike protein receptor-binding domain structure and neutralizing antibody interactions. Front Virol (2022) 2:830202. doi: 10.3389/fviro.2022.830202
9. VanBlargan LA, Errico JM, Halfmann PJ, Zost SJ, Crowe JE, Purcell LA, et al. An infectious sars-cov-2 b.1.1.529 omicron virus escapes neutralization by therapeutic monoclonal antibodies. Nat Med (2022) 28(3):490–5. doi: 10.1038/s41591-021-01678-y
10. Vangone A, Bonvin AMJJ. Contacts-based prediction of binding affinity in protein–protein complexes. eLife (2015) 4:e07454. doi: 10.7554/eLife
11. US Food and Drug Administration. Emergency use authorization 094 (2022). Available at: https://pi.lilly.com/eua/bam-and-ete-eua-fda-authorization-letter.pdf.
12. US Food and Drug Administration. Emergency use authorization 111 (2022). Available at: https://www.fda.gov/media/156151/download.
13. US Food and Drug Administration. Emergency use authorization 104 (2023). Available at: https://www.fda.gov/media/154704/download.
14. Jones BE, Brown-Augsburger PL, Corbett KS, Westendorf K, Davies J, Cujec TP, et al. The neutralizing antibody, ly-cov555, protects against sars-cov-2 infection in nonhuman primates. Sci Trans Med (2021) 13(593):eabf1906. doi: 10.1126/scitranslmed.abf1906
15. Westendorf K, Žentelis S, Wang L, Foster D, Vaillancourt P, Wiggin M, et al. Ly-cov1404 (bebtelovimab) potently neutralizes sars-cov-2 variants. Cell Rep (2022) 39(7):110812. doi: 10.1016/j.celrep.2022.110812
16. Dong J, Zost SJ, Greaney AJ, Starr TN, Dingens AS, Chen EC, et al. Genetic and structural basis for sars-cov-2 variant neutralization by a two-antibody cocktail. Nat Microbiol 6(10):1233–44. doi: 10.1038/s41564-021-00972-2
17. de Vries SJ, Bonvin AMJJ. Cport: A consensus interface predictor and its performance in prediction-driven docking with haddock. PloS One (2011) 6(3):1–12. doi: 10.1371/journal.pone.0017695
18. Mannar D, Saville JW, Zhu X, Srivastava SS, Berezuk AM, Tuttle KS, et al. SARS-CoV-2 omicron variant: Antibody evasion and cryo-EM structure of spike protein-ACE2 complex. Science (2022) 375(6582):760–4. doi: 10.1126/science.abn7760
19. Jumper J, Evans R, Pritzel A, Green T, Figurnov M, Ronneberger O, et al. Highly accurate protein structure prediction with AlphaFold. Nature (2021) 596(7873):583–9. doi: 10.1038/s41586-021-03819-2
20. Mirdita M, Schütze K, Moriwaki Y, Heo L, Ovchinnikov S, Steinegger M. ColabFold: making protein folding accessible to all. Nat Methods (2022) 19(6):679–82. doi: 10.1038/s41592-022-01488-1
21. Li T, Han X, Gu C, Guo H, Zhang H, Wang Y, et al. Potent SARS-CoV-2 neutralizing antibodies with protective efficacy against newly emerged mutational variants. Nat Commun (2021) 12(1):6304. doi: 10.1038/s41467-021-26539-7
22. Liu H, Yuan M, Huang D, Bangaru S, Zhao F, Lee C-CD, et al. Wilson. A combination of cross-neutralizing antibodies synergizes to prevent SARS-CoV-2 and SARS-CoV pseudovirus infection. Cell Host Microbe (2021) 29(5):806–818.e6. doi: 10.1016/j.chom.2021.04.005
23. Barnes CO, Jette CA, Abernathy ME, Dam K-MA, Esswein SR, Gristick HB, et al. SARS-CoV-2 neutralizing antibody structures inform therapeutic strategies. Nature (2020) 588(7839):682–7. doi: 10.1038/s41586-020-2852-1
24. Fenwick C, Turelli P, Ni D, Perez L, Lau K, Lau C, et al. Patient-derived monoclonal antibody neutralizes SARS-CoV-2 omicron variants and confers full protection in monkeys. Nat Microbiol (2022) 7(9):1376–89. doi: 10.1038/s41564-022-01198-6
25. Nutalai R, Zhou D, Tuekprakhon A, Ginn HM, Supasa P, Liu C, et al. Potent cross-reactive antibodies following omicron breakthrough in vaccinees. Cell (2022) 185(12):2116–31:e18. doi: 10.1016/j.cell.2022.05.014
26. van Zundert GCP, Rodrigues J.P.G.L.M., Trellet M, Schmitz C, Kastritis PL, Karaca E, et al. The HADDOCK2.2 web server: User-friendly integrative modeling of biomolecular complexes. J Mol Biol (2016) 428(4):720–5. doi: 10.1016/j.jmb.2015.09.014
Keywords: SARS-CoV-2, bioinformatics, artificial intelligence, antibody docking, protein structural and functional analysis, protein analysis
Citation: Ford CT, Yasa S, Jacob Machado D, White RA III and Janies DA (2023) Predicting changes in neutralizing antibody activity for SARS-CoV-2 XBB.1.5 using in silico protein modeling. Front. Virol. 3:1172027. doi: 10.3389/fviro.2023.1172027
Received: 22 February 2023; Accepted: 24 March 2023;
Published: 26 April 2023.
Edited by:
Samuel Ken-En Gan, Kean University-Wenzhou, ChinaReviewed by:
Jasdeep Singh, University of Denver, United StatesCopyright © 2023 Ford, Yasa, Jacob Machado, White and Janies. This is an open-access article distributed under the terms of the Creative Commons Attribution License (CC BY). The use, distribution or reproduction in other forums is permitted, provided the original author(s) and the copyright owner(s) are credited and that the original publication in this journal is cited, in accordance with accepted academic practice. No use, distribution or reproduction is permitted which does not comply with these terms.
*Correspondence: Colby T. Ford, Y29sYnkuZm9yZEB1bmNjLmVkdQ==
Disclaimer: All claims expressed in this article are solely those of the authors and do not necessarily represent those of their affiliated organizations, or those of the publisher, the editors and the reviewers. Any product that may be evaluated in this article or claim that may be made by its manufacturer is not guaranteed or endorsed by the publisher.
Research integrity at Frontiers
Learn more about the work of our research integrity team to safeguard the quality of each article we publish.