- 1Facultad de Veterinaria, Instituto de Ciencias Biomédicas, Universidad Cardenal Herrera-CEU, CEU Universities, Valencia, Spain
- 2IRTA, Programa de Sanitat Animal, CReSA, Collaborating Centre of the World Organisation for Animal Health for Research and Control of Emerging and Re-Emerging Pig Diseases in Europe, Barcelona, Spain
- 3Unitat mixta d’Investigació IRTA-UAB en Sanitat Animal, Centre de Recerca en Sanitat Animal (CReSA), Campus de la Universitat Autònoma de Barcelona, Barcelona, Spain
- 4Microbiology Department, Dr. Balmis University General Hospital, Microbiology Division, Miguel Hernández University, ISABIAL, Alicante, Spain
- 5Área de Microbiología, Departamento de Farmacia, Instituto de Ciencias Biomédicas, Facultad de Ciencias de la Salud, Universidad Cardenal Herrera-CEU, CEU Universities, Valencia, Spain
- 6Institute of Science and Animal Technology, Universitat Politècnica de Valencia, Valencia, Spain
Introduction: Stringent regulations in pig farming, such as antibiotic control and the ban on certain additives and disinfectants, complicate disease control efforts. Despite the evolution of microbial communities inside the house environment, they maintain stability over the years, exhibiting characteristics specific to each type of production and, in some cases, unique to a particular company or farm production type. In addition, some infectious diseases are recurrent in specific farms, while other farms never present these diseases, suggesting a connection between the presence of these microorganisms in animals or their environment. Therefore, the aim of this study was to characterise environmental microbiomes of farms with high and low sanitary status, establishing the relationships between both, health status, environmental microbial ecology and its functionality.
Methods: For this purpose, 6 pig farms were environmentally sampled. Farms were affiliated with a production company that handle the majority of the pigs slaughtered in Spain. This study investigated the relationship among high health and low health status farms using high throughput 16S rRNA gene sequencing. In addition, to identify ecologically relevant functions and potential pathogens based on the 16S rRNA gene sequences obtained, functional Annotation with PROkaryotic TAXa (FAPROTAX) was performed.
Results and Discussion: This study reveals notable differences in microbial communities between farms with persistent health issues and those with good health outcomes, suggesting a need for protocols tailored to address specific challenges. The variation in microbial populations among farms underscores the need for specific and eco-friendly cleaning and disinfection protocols. These measures are key to enhancing the sustainability of livestock farming, ensuring safer products and boosting competitive edge in the market.
1 Introduction
Decisions made by European authorities, such as the prohibition of antibiotics as prophylactics (since 2006), the recently restricted use of colistin and the banning of zinc oxide as treatment, have led to a significant increase in on-farm diseases, such as Salmonella spp. or post-weaning diarrhoea (1). Moreover, this leads to an increase in the excretion of these pathogens into the environment and, consequently, an increase in environmental infectious pressure and alteration of the microbial ecology of the farm environment (2). For all these reasons, searching for cost-effective, animal-friendly, and environmentally respectful alternatives to antibiotics are necessary; all of them aim to enable the animal to be immunocompetent, resilient to fight infectious diseases and to recover as quickly as possible in the event of illness (3).
Among the various alternatives to antibiotics at field level, the most studied are management, the development of new vaccines, feed supplements, and strict biosecurity, including comprehensive cleaning and disinfection (C&D) protocols. Martelli et al. (4) demonstrated that an adequate C&D schedule significantly reduces residual contamination in pig facilities. However, persistent pathogens such as Salmonella spp. remain in the environment among batches. Therefore, routine C&D protocols used in the field must be complemented with other control measures throughout the pig breeding and production chain (4).
In recent years, an increasing number of studies have highlighted the potential role of biocides in selecting cross-resistance to antibiotics in bacteria. However, the vast majority of them were conducted using pure culture models under laboratory conditions (5, 6). The current absence of field data makes it difficult to assess the significant risk of selecting bacteria with heightened antibiotic resistance due to the use of biocides in industries, as well as to determine which biocides are linked to the highest risk of cross-selection of antibiotic resistance (7).
Traditionally, different types of disinfectants have been used at field level for surface hygiene in livestock, such as products based on quaternary ammonium compounds containing glutaraldehyde, formaldehyde, peroxide-based compounds or peracetic acid, iodine-based compounds or chlorocresols. However, it is widely known that formaldehyde is one of the most effective disinfectants against zoonotic pathogens of such importance as Salmonella spp. (8). Restrictions in Europe on the use of formaldehyde, given its categorisation as a carcinogen, mutagen, and highly toxic, have meant the imminent need for the sector to seek alternatives for farm hygiene (8, 9). In this context, C&D is key to reducing the environmental pressure of pathogens and the number of infected individuals, but the threat of persistence of the most resistant strains in the environment remains, as they can persist between consecutive animal batches, increasing the risk of infection (10).
Farm environment can be a complex stable community of microorganisms, mostly bacteria, but also includes viruses, archaea, fungi, yeasts and protozoa, that persists among different animal batches. The microorganisms that inhabit the farm environment can strongly influence the associated animal health, food quality and safety (11). In this sense, there is a growing research effort to unravel the ecological mechanisms that encompass this microbiome. Among them, it is noteworthy that these communities may find protection through structures known as biofilms. Biofilms are formations attached to a surface where microorganisms are embedded and shielded by an exopolysaccharide matrix. The forming of biofilms improves the producer’s ability to endure in a specific environment (12, 13). Moreover, communities safeguarded by a biofilm exhibit 100 times more resistance against C&D protocols (14, 15). Additionally, adaptive stress responses contribute to the efficiency of these communities in surviving the farm environment (16).
Despite the evolution of microbial communities inside the house environment (11), these communities maintain stability over the years, exhibiting characteristics specific to each type of production and, in some cases, unique to a particular company or production type (17). On several occasions, clones of microorganisms, such as Salmonella spp., or Campylobacter spp., found in animal-derived products have been commonly detected at the farm of origin (18, 19). In addition, some infectious diseases are always associated with specific farms, while other farms never present diseases (high health status farms vs low health status farms), suggesting a connection between the presence of these microorganisms in the animals and their surrounding environment at the farm.
Thus, the aim of this study was to characterise environmental microbiomes of both, high and low sanitary status farms, establishing the relationships between health status, environmental microbial ecology and its functionality.
2 Materials and methods
2.1 Study sample
Over a 4-month period, 6 houses from 6 pig farms were environmentally sampled. Farms were affiliated with a production company that handles the majority of the pigs slaughtered in Spain.
2.2 Selection of high and low sanitary status farms to define “healthy” and “unhealthy” microbiomes
A farm with a High Health Status (HHS) should meet the following criteria: (i) An outstanding production performance. Production parameters measured as average daily gain and feed conversion rate falling within the best 25% of the whole pig population. (ii) Outstanding health records reaching at least the best 10% of the whole pig population. These parameters are mortality, percentage of substandard pigs during the rearing period and treatment cost due to antimicrobials. The expected mortality rate from weaning to slaughter, percentage of substandard pigs and treatment cost for these farms would be 4%, 2–3% and 1–1.5 € per pig, respectively. These farms are usually selection and multiplication farms.
Accordingly, Low Health Status (LHS) farms in this case will be those farms with recurrent problems of post-weaning diarrhoea within 2 weeks after weaning and at least 10–15% morbidity.
Based on the information provided by the company enrolled for the study, management practices were standardised across all their farms. Still, HHS and LHS farms have all passed the Biocheck UGent survey. However, farms with HHS scored higher for some of the features included in both, external and internal biosecurity, especially, “purchase of breeding pigs,” “personnel and people visiting the farm,” “measures between compartments, “working lines and use of equipment” and “cleaning and disinfection.” Along with the Biocheck UGent, the company had established its own risk index for the main swine pathogens based on their occurrence at their farms during the last 10 years. HHS farms were free of these main swine pathogens; porcine reproductive and respiratory syndrome virus (PRRSV), swine dysentery and Mycoplasma hyopneumoniae and had mortality rates lower than 4%.
2.3 Sampling
During January and June 2023, six pig farms were enrolled for the study (3 HHS and 3 LHS). One house from each farm was selected according to the described criteria. At each farm, one house from the nursery facility containing sows with 3 weeks-old piglets was selected. Thereafter, 10 pens within each house were sampled (walls and slats), i.e., four and six pens from the corners and the middle of the house, respectively (Figure 1). In addition, two slurry pit samples were collected. For this purpose, two 500 mL sterile pots were filled from different points of the house pits to ensure a representative sample from each house studied. Wall and slat samples were collected by wiping 1 m2 of surfaces using both sides of the sterile wipe (Whirl-Pak®, Scharlab, Madrid). First, wall samples (10 per farm, one per pen) were taken at an approximate height of 70 cm above the pen floor. Then, the slat samples (10 per farm, one per pen) were collected, between and under the grates whenever possible. Once collected, all samples were placed individually in a sterile bag with sterile diluent and transported to the laboratory in refrigerated conditions (4°C) and processed within 12 h.
Once in the laboratory, wall and slat samples were processed identically. Two pools of 5 wipes each (1/4 of a wipe per pool) were generated for each type of sample. The remaining wipes were stored in freezer for further studies. Then, each pool and each slurry sample were placed in a stomacher (BagFilter® 400 mL, Scharlab, Madrid) and homogenised with 15 mL of PBS (5 min, 260 rpm).
2.4 DNA extraction, 16S rRNA gene amplification, and MiSeq sequencing
The DNA was extracted from 250 μL of each homogenised environmental sample (wall and slats) and from 250 μL of slurry following the manufacturer’s instructions (QIAamp Power Fecal Pro DNA kit, Werfen, Barcelona, Spain). The DNA quality was determined using Nanodrop ND − 1000 spectrophotometer (Thermo Scientific, Wilmington, DE, United States) and DNA was quantified using Qubit fluorometer (Life Technologies, Paisley, United Kingdom). The DNA was frozen at −20°C for shipment at the Instituto de Investigación Sanitaria y Biomédica de Alicante—ISABIAL (Alicante, Spain). Once there, 16S rRNA gene amplification and MiSeq (Illumina) sequencing was performed according to Montoro-Dasi et al. (20). To this end, 12.5 ng of DNA from each sample, quantified using Qubit, was used to prepare the library according to the 16S rRNA Metagenomic Sequencing Library Preparation protocol (Illumina). The primers targeted the V3 and V4 regions of the 16S rRNA gene, and included Illumina adapters: 16S Amplicon PCR Forward Primer = 5′ TCGTCGGCAGCGTCAGATGTGTATAAGAGACAGCCTACGGGNGGCWGCAG; and 16S Amplicon PCR Reverse Primer = 5′ GTCTCGTGGGCTCGGAGATGTGTATAAG AGACAGGA CTACHVGGGTATCTAATCC. NGS Libraries were analysed using the Agilent 4200 TapeStation System to ensure their integrity. Sequencing was performed on a MiSeq (21) system in 2 × 300 bp format. The quality of the raw reads was evaluated using the FastQC software (22).
2.5 Bioinformatic analysis
Bioinformatic analyses were performed separately for each type of sample (wall, slat, and slurry). For this purpose, the Amplicon Sequence Variant (ASV) picking, and analysis was performed with QIIME2 (v2021.4) pipeline (23). Demultiplexed paired FASTQ sequences were imported into the QIIME2 v2021.4. The DADA2 plugin incorporated into QIIME2 was used to quality filter, denoise, combine, and remove chimaera from the sequences. The raw sequences were truncated from the first low-quality base site whose number of low-quality values (default quality threshold ≤30) and the primer sequences were removed from all reads. Then, sequences were grouped into amplicon sequence variants (ASVs) with 99% identification. Taxonomy was assigned to ASVs using the classify-sklearn naïve Bayes taxonomy classifier in the feature-classifier plugin against the SILVA v138 database (24, 25). Sequences not assigned to any taxa or classified as Eukaryote, Archaea or only bacteria were filtered out (24, 25).
2.6 Statistical analysis
The statistical analysis of wall, slats and slurry was conducted using the following methodology (26). No outlier samples were identified via principal component analysis. Genera with almost 25% zeros within each treatment were excluded. The remaining zeros were replaced by one for microbiome data and by half of the minimum value detected for each genus. A total of 57, 95, and 259 genera, from the wall, slat and slurry samples, respectively, remained in the datasets. Datasets were transformed using the additive log-ratio (ALR) transformation as follows (Equation 1):
Here, j represents the total number of variables in the dataset, 𝑥j is the value for the genus j and 𝑥𝑟𝑒𝑓 is the reference variable used for data transformation. The reference variable was chosen as the one with the lowest coefficient of variation: Cellulosilyticum for wall samples, p-2534-18B5_gut_group for slat samples, and Dojkabacteria for slurry samples. The lack of isometry was checked using Procrustes correlation (27). ALRs were autoscaled with mean of 0 and standard deviation of 1.
A partial least-squares discriminant analysis (PLS-DA) was employed to identify the genera that allowed the classification or discrimination of the sanitary status (28). For the PLS-DA models, the sanitary status served as the categorical vector y, and the ALR-transformed dataset for genera was utilised as the matrix X. The balance error rate (BER) for the Mahalanobis distance, calculated using a 4-fold cross-validation repeated 100 times, was employed to determine the optimal number of components for the model in each iteration. During each iteration, variables with a variable importance prediction (VIP) score below 1 were excluded from the X matrix, as they did not contribute significantly to the classification among the treatments (29). Following variable selection, a new PLS-DA model was computed. This process of variable selection and PLS-DA model computation were repeated until the lowest BER was obtained. The prediction accuracy of the final PLS-DA model was assessed by constructing a confusion matrix and a permuted confusion matrix, using 4-fold cross-validation repeated 10,000 times. The confusion matrix enabled the evaluation of the model’s ability to predict each treatment based on the selected variables, while the permuted confusion matrix assessed whether the observed performance was due to a random selection of variables throughout the PLS-DA iterations. The prediction performance was deemed spurious when the percentage of true positives for each treatment was far from their random probabilities (50% for two categories).
In addition to PLS-DA, Bayesian statistics were employed to assess the relevance of the differences in genera abundance between the HHS and LHS groups. A model with a single effect of “environment” and flat priors was fitted. The marginal posterior distribution of the unknowns was estimated using MCMC with four chains of 50,000 iterations, with a burn-in of 1,000 and a lag of 10. The posterior mean of the differences between the HHS and LHS was used to estimate the posterior mean of the differences in genera abundance between these groups. These estimates were expressed in units of standard deviation (SD) for each variable. Differences in the mean abundance of genera between the HHS and the LHS groups were considered relevant when the probability of the differences (30) being greater (if the difference is positive) or lower (if negative) than 0 (P0) was higher than 0.95.
Alpha and beta diversity were calculated using the ALR at the genus level to assess differences in microbiome composition among groups in wall, slat and slurry samples. Alpha diversity was evaluated with the Shannon diversity and the inverse Simpson indexes to analyse the species diversity and evenness. Differences in alpha diversity distribution among groups were considered significant when the p value from a Mann–Whitney U test was lower than 0.05. Beta diversity was assessed using the Bray–Curtis dissimilarity matrix, and a nonmetric multidimensional scaling (NMDS) was performed to obtain the loadings of the first two dimensions. Differences in microbial genera composition were examined using a permutational multivariate analysis of variance (PERMANOVA; p value < 0.05) on the loadings of the two first MDS dimensions (31, 32).
2.7 Bacterial functional annotation and distribution
Finally, the Functional Annotation of PROkaryotic TAXa (FAPROTAX) database (33, 34) was implemented to identify ecologically relevant functions, potential pathogens based on the 16S rRNA gene sequences for each sample, and for more-related-environmental samples (wall and slat).
3 Results
A total of 36 samples were collected from 3 HHS and 3 LHS farms. On each farm, 10 samples from wall (2 pooled samples/farm), 10 from slat (2 pooled samples/farm), and 2 from slurry pit were collected.
3.1 16S rRNA sequencing
The summary of sequences and ASVs obtained in this study for wall, slat, and slurry samples can be seen in Table 1, detailing the results obtained from each sample type.
The datasets generated and analysed are available at NCBI’s BioProject PRJNA1079579 and BioSample SAMN40044702.
3.2 Taxonomic characterisation of environmental microbial communities
To better establish the microbial community composition of pig farm environments according to their health status, organisms present at different taxonomic levels and their relative abundance were evaluated. Alignment of ASVs against the SILVA database resulted in identification of 23 bacterial phyla and 389 bacterial genera. While the majority of OTUs were identified at genus level (255), some were only classified at the phylum, class, order, or family level.
At phylum level, Firmicutes represented the dominant phylum of the environmental community in all the sample types collected. In the case of wall and slat samples, this phylum was followed by Proteobacteria. For wall samples, relative abundance of Proteobacteria comprised 25.0 and 25.4%, for HHS and LHS farms, respectively, of total relative abundance, whereas for slat samples was 21.3 and 18.5%, respectively. For slurry samples, Firmicutes was followed by Bacteroidota, comprising 25.6 and 19.8% of the relative abundance, for HHS and LHS farms, respectively, and Proteobacteria represented 8.6 and 4.3% of the relative abundance for HHS and LHS. The relative abundance of all the phyla present is shown in Figure 2. A more similar distribution can be observed among the environmental samples (wall and slat), indicating a distinct pattern compared to the slurry samples.
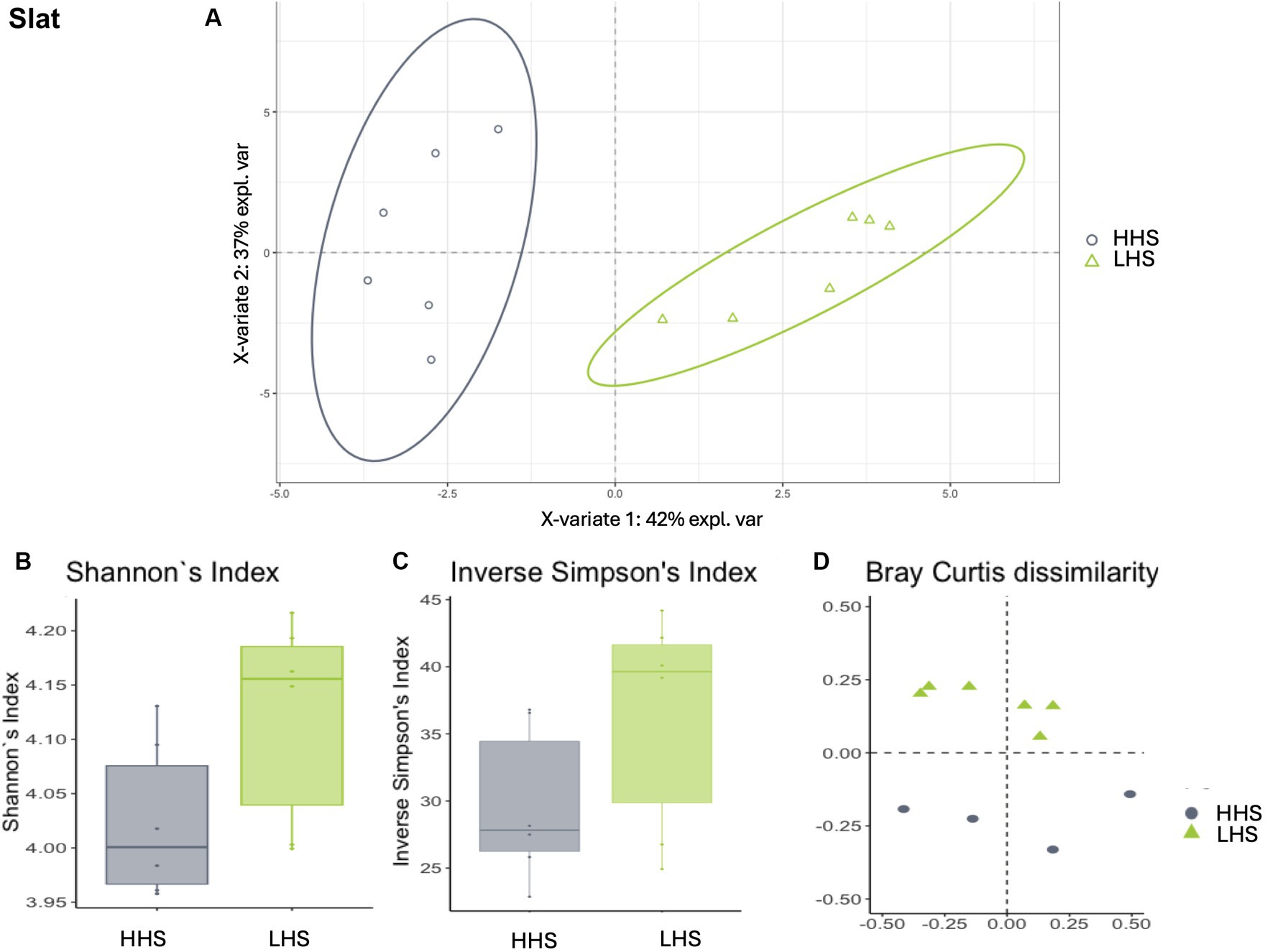
Figure 2. Environmental microbiota modulation induced by sanitary status in pig farms in wall samples. (A) Two-dimensional PLS-DA score plot was constructed using microbiota genera as variables, representing the distribution of the samples between the first two components in the model, which correspond to the sanitary status (HHS vs. LHS). Alpha diversity was computed using the (B) Shannon diversity index and (C) inverse Simpson index. Beta diversity was computed by calculating (D) the Bray–Curtis dissimilarity matrix. Differences among populations were established as having a p-value lower than 0.05. HHS, high health status; LHS, low health status.
Two hundred and fifty-five genera were identified across all the samples (wall, slat, and slurry). Among them, 4 genera were detected in all the samples analysed (Corynebacterium, Turicibacter, Lactobacillus and Terrisporobacter), a total of 18 genera were exclusive for HHS farms (Terrabacter, Chryseobacterium, Soonwooa, Asteroleplasma, Pseudoramibacter, Butyrivibrio, Intestinimonas, Oscillospira, Anaerotruncus, Caproiciproducens, Schwartzia, Veillonella, Candidatus saccharimonas, Acetobacter, Devosia, Shewanella, Vitreoscilla and Morganella) and 30 were exclusive for LHS farms (Gordonia, Gelidibacter, Sulfuricurvum, Desulfatiglans, Desulfatiferula, Elusimicrobium, Endomicrobium, Caryophanon, Solibacillus, Atopostipes, Anaerofustis, Epulopiscium, Papillibacter, Sporobacter, Dehalobacterium, Hydrogenedesnsaceae, Dojkabacteria, Moranbacteria, Parcubacteria, Paenochrobactrum, Paracoccus, Mitochondria, Sphingopyxis, Thauera, Wohlfahrtiimonas, Aestuariicella, Rickettsiella, Oceanobacter, Thiopseudomonas and Arenimonas).
Moreover, 11 were exclusive for wall samples (Barchybacterium, Terrabacter, Micrococcus, Soonwoa, Desemzia, Veillonella, Devosia, Shewanella, Morganella, Serratia and Vibrio); between them, Terrabacter, Soonwoa, Veillonella, Devosia, Shewanella and Morganella were only observed in wall samples collected from HHS farms. On the other hand, 5 genera were exclusive for slat samples (Timotella, Savagea, Alloiococcus, Fusobacterium and Wohlfahrtiimonas), of which Wohlfahrtiimonas was only detected in HHS farms. Finally, 147 genera were exclusive for slurry samples (the most prevalent were Treponema, Fastidiosipila, Arcobacter, Trichococcus, Candidatus nomurabacteria, Proteiniphilum, Tissierella, Cloacimonas, Acholeplasma and Sedimentibacter), of which 9 and 25 were exclusive for HHS and LHS samples, respectively.
3.3 Sanitary status environmental microbiota modulation
A PLS-DA with ALR-transformed variables was employed to evaluate the impact of sanitary status (HHS vs. LHS) on the environmental microbiota across wall, slat, and slurry samples. The analysis identified the most relevant genera, i.e., those that reached the highest prediction performance in each type of sample.
For three types of environmental samples, the model revealed a dependency of the microbiome on the farms´ sanitary status, distinguishing between both states effectively (Procrustes correlation and prediction performance). Wall samples, for instance, exhibited a high Procrustes correlation of 0.92, identifying 14 out of 57 genera as relevant variables and achieving prediction performance rates of 99.3% for HHS and 95.3% for LHS (Figure 2A). The results showed that 25% of the genera enable discrimination between both sanitary statuses in the wall samples, as listed in Table 2.
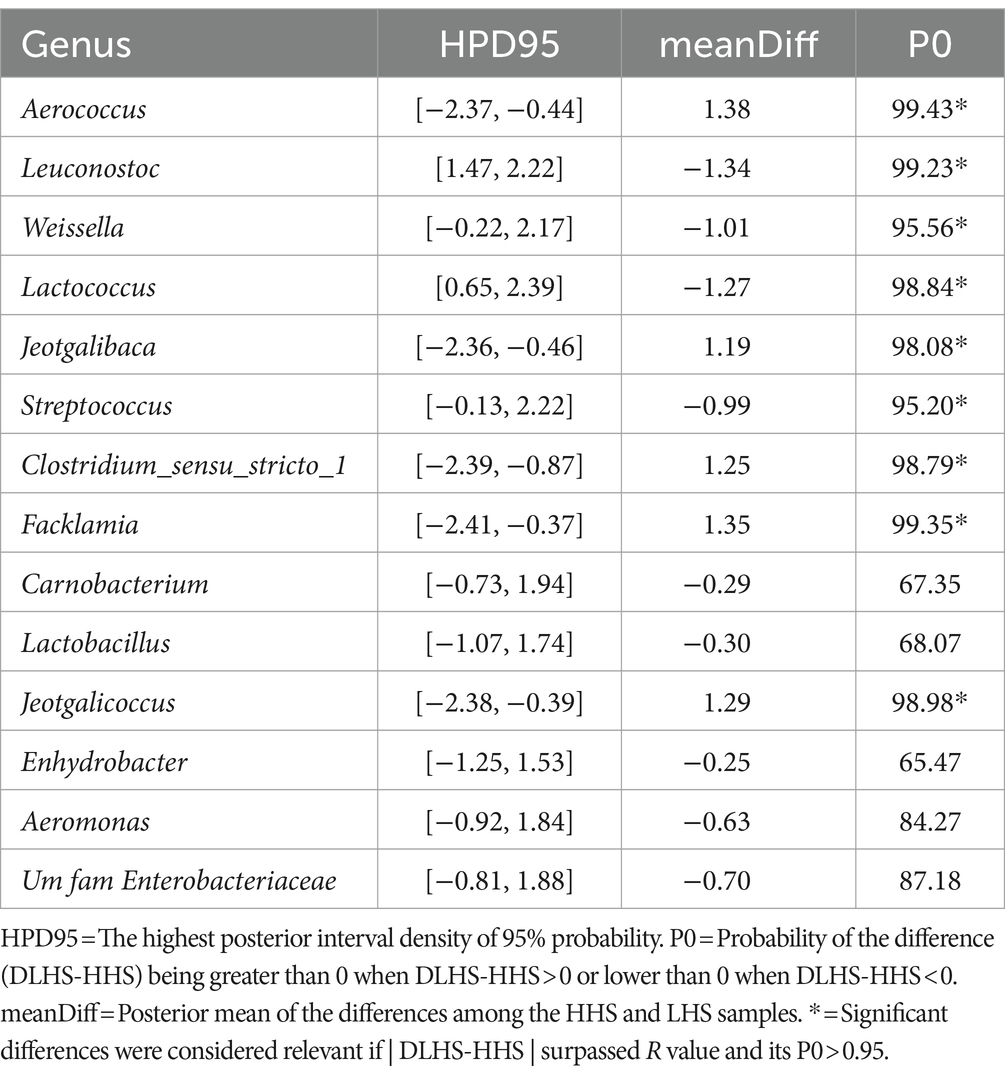
Table 2. Results of the Bayesian statistical analysis conducted on the key genera identified through partial least-squares discriminant analysis (PLS-DA) in wall samples.
Similarly, slat samples displayed a Procrustes correlation of 0.92, with 24 out of 95 genera identified as relevant variables, achieving a prediction performance rate of 93.0% for HHS and 100% for LHS (Figure 3A). Again, 25% of the genera were required for discriminating between the sanitary statuses in the slat samples, as detailed in Table 3.
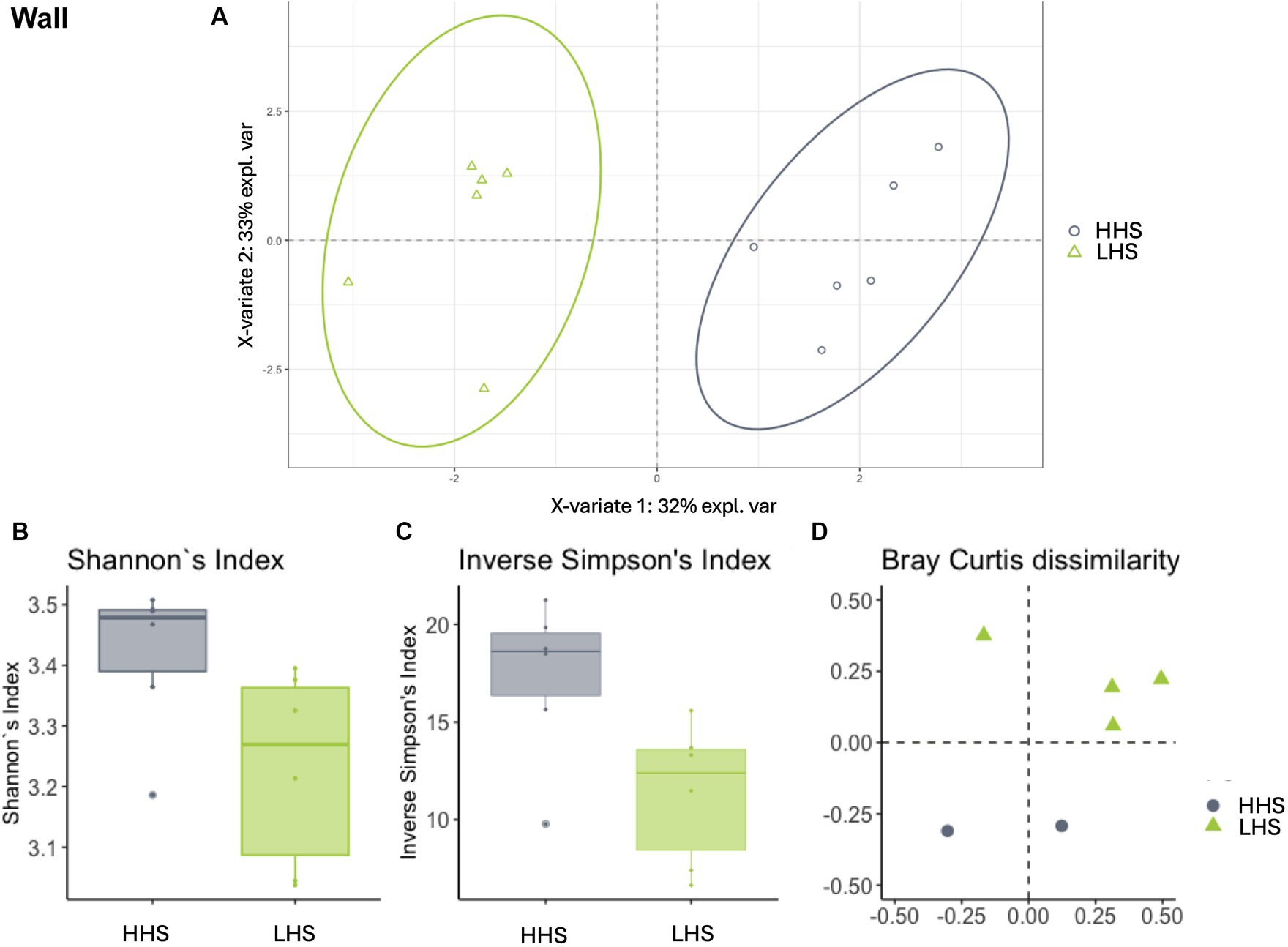
Figure 3. Environmental microbiota modulation induced by sanitary status in pig farms in slat samples. (A) Two-dimensional PLS-DA score plot was constructed using microbiota genera as variables, representing the distribution of the samples between the first two components in the model, which correspond to the sanitary status (HHS vs. LHS). Alpha diversity was computed using the (B) Shannon diversity index and (C) inverse Simpson index. Beta diversity was computed by calculating (D) the Bray–Curtis dissimilarity matrix. Differences among populations were established as having a p-value lower than 0.05. HHS, high health status; LHS, low health status.
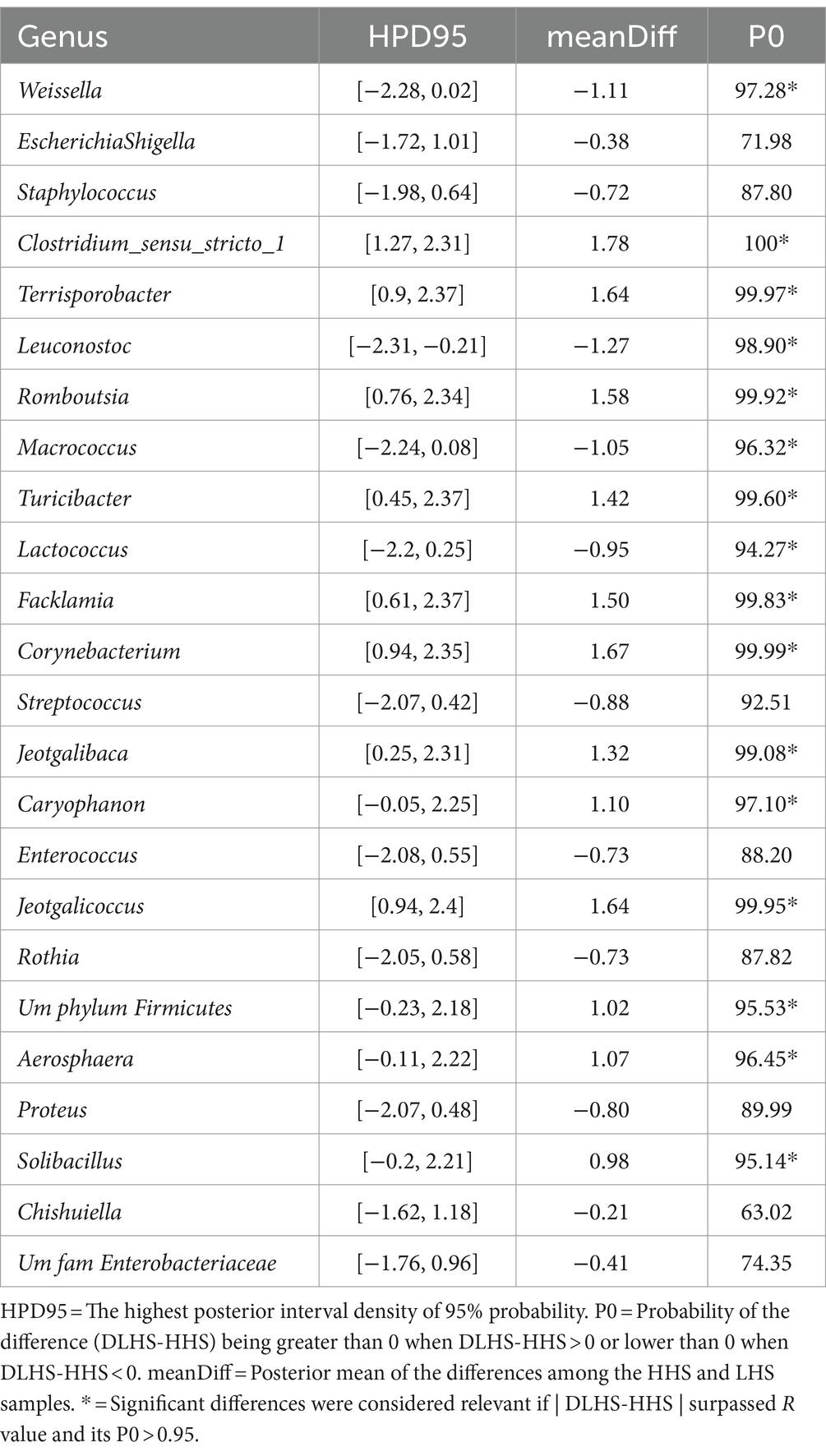
Table 3. Results of the Bayesian statistical analysis conducted on the key genera identified through partial least-squares discriminant analysis (PLS-DA) in slat samples.
Finally, slurry samples also exhibited a high Procrustes correlation of 0.82, with 23 out of 259 genera identified as relevant variables, achieving prediction performance rates of 100% for HHS and 99.8% for LHS (Figure 4A). The results showed that only 8% of the genera enable discrimination between both sanitary statuses in the slat samples, as outlined in Table 4.
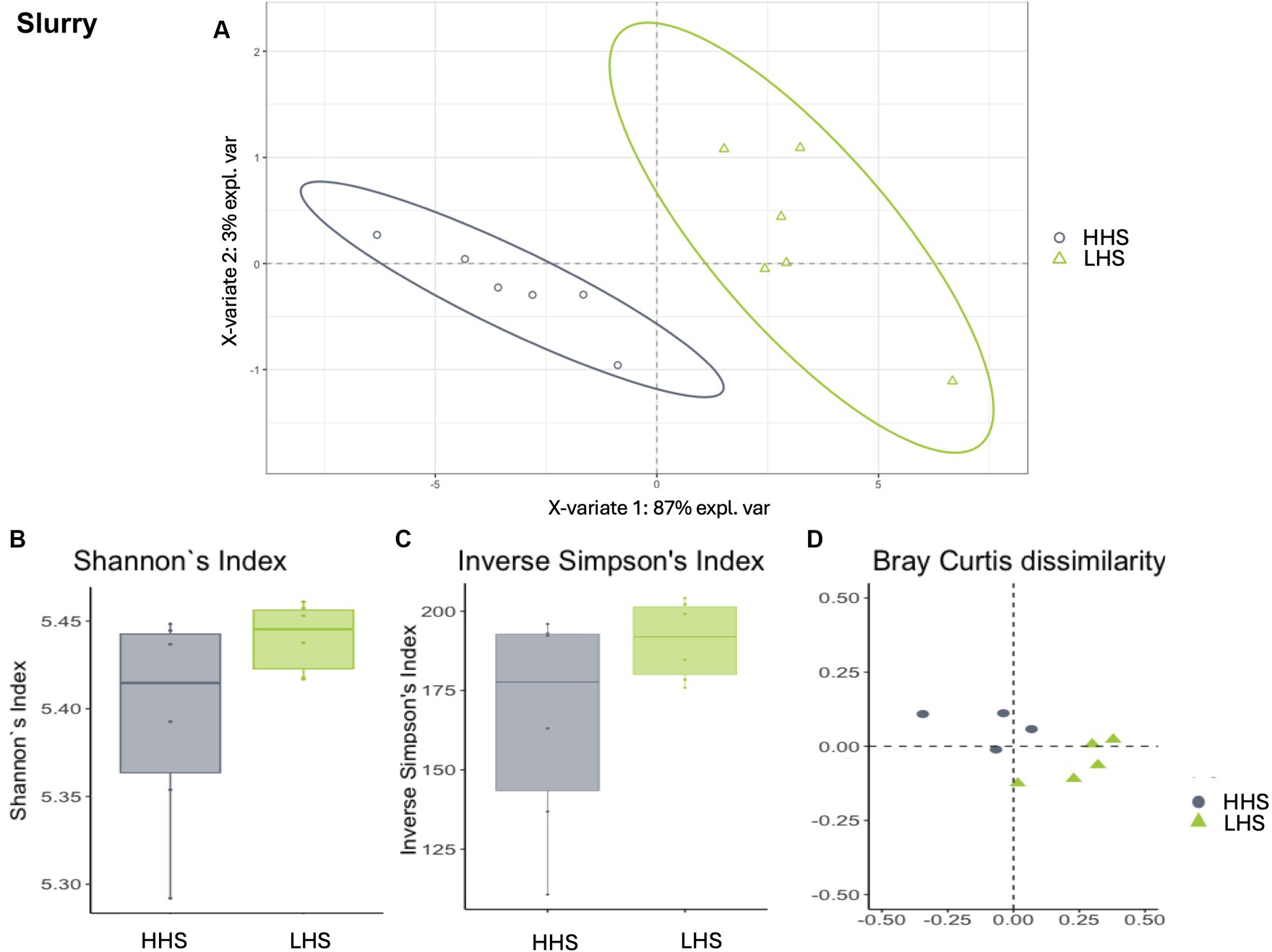
Figure 4. Environmental microbiota modulation induced by sanitary status in pig farms in slurry samples. (A) Two-dimensional PLS-DA score plot was constructed using microbiota genera as variables, representing the distribution of the samples between the first two components in the model, which correspond to the sanitary status (HHS vs. LHS). Alpha diversity was computed using the (B) Shannon diversity index and (C) inverse Simpson index. Beta diversity was computed by calculating (D) the Bray–Curtis dissimilarity matrix. Differences among populations were established as having a p-value lower than 0.05. HHS, high health status; LHS, low health status.
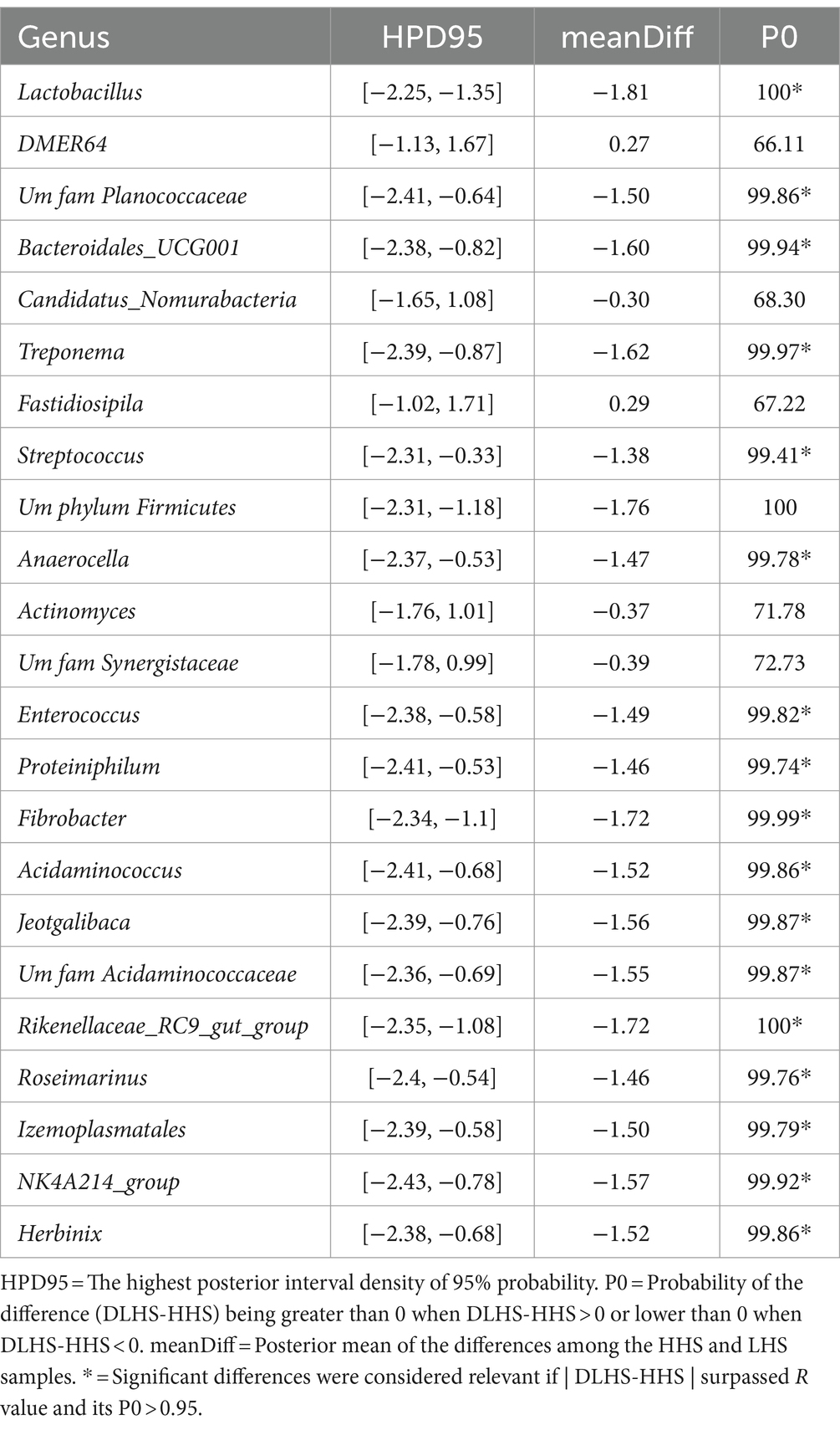
Table 4. Results of the Bayesian statistical analysis conducted on the key genera identified through partial least-squares discriminant analysis (PLS-DA) in slurry samples.
Once the discriminative power of the model was established, the analysis of the microbiota composition was conducted, revealing similar patterns in both sanitary states for the environmental samples. The Shannon diversity index, which is more sensitive to species richness, indicated that microbiota diversity in wall, slat and slurry samples was similar under both sanitary conditions (Kruskal–Wallis test, HHS vs. LHS: p-value = 0.06, 0.06 and 0.13, respectively, Figures 2B, 3B, 4B). The inverse Simpson index, which is more sensitive to species evenness, revealed significantly higher diversity in HHS compared to LHS in wall samples (Kruskal-Wallis test, HHS vs. LHS: p-value = 0.03, Figure 2C), while no differences were observed in slat and slurry samples (Kruskal-Wallis test, HHS vs. LHS: p-value = 0.18, both, Figures 3C, 4C). Furthermore, pairwise PERMANOVA comparisons between groups using Bray–Curtis dissimilarity matrices showed similarity in microbiome composition between both sanitary statuses in wall, slat and slurry samples (p-value = 0.545, 0.058 and 0.324, respectively; Figures 2D, 3D, 4D).
To gain a deeper understanding of the impact of sanitary status on the environmental microbiome, a Bayesian statistical analysis was performed from the initial relevant genera identified through PLS-DA. For wall samples, as shown in Table 2, the Bayesian statistical analysis revealed 9 genera with relevant differences (P0 > 0.95) in abundance between the two sanitary statuses (HHS vs. LHS). Among them, Leuconostoc, Weissella, Lactococcus, and Streptococcus were more abundant in HHS; while Aerococcus, Jeotgalibaca, Clostridium_sensu_stricto_1, Facklamia, and Jeotgalicoccus were more abundant in LHS.
For slat samples, as shown in Table 3, the Bayesian statistical analysis revealed 9 genera with relevant differences (P0 > 0.95) in abundance between the two sanitary statuses (HHS vs. LHS). Among them, Weissella, Leuconostoc, and Macrococcus were more abundant in HHS; while Clostridium_sensu_stricto_1, Terrisporobacter, Romboutsia, Turicibacter, Facklamia, Corynebacterium, Jeotgalibaca, Caryophanon, Jeotgalicoccus, UmphylumFirmicutes, Aerosphaera, and Solibacillus were more abundant in LHS.
Finally, for slurry, as shown in Table 4, the Bayesian statistical analysis revealed 9 genera with relevant differences (P0 > 0.95) in abundance between the two sanitary statuses (HHS vs. LHS). Among them, all were more abundant in HHS: Lactobacillus, UMFAMPlanococcaceae, Bacteroidales_UCG001, Treponema, Streptococcus, UmphylumFirmicutes, Anaerocella, Enterococcus, Proteiniphilum, Fibrobacter, Acidaminococcus, Jeotgalibaca, UmfamAcidaminococcaceae, Rikenellaceae_RC9_gut_group, Roseimarinus, Izemoplasmatales, NK4A214_group, and Herbinix.
3.4 Bacterial functional annotation and distribution
In a general analysis all the genera identified in this study were included. A total of 33 categories of microbial functions linked to the bacterial communities were detected, of which 1 and 11 were exclusive for samples collected from HHS and LHS farms, respectively. As shown in Table 5, for both groups the predominant microbial functions assigned were chemoheterotrophy, fermentation, aerobic chemoheterotrophy and aromatic compound degradation. Moreover, as mentioned above, iron respiration was exclusive to HHS, and functions related to thiosulfate respiration, methanol oxidation, methylotrophy, nitrate denitrification, nitrite denitrification and respiration, denitrification, nitrous oxide denitrification and intracellular parasites were exclusive to LHS farms.
Regarding the functional annotation for each sample type, functions associated with the microbiota of wall samples were fermentation, chemoheterotrophy, aerobic chemoheterotrophy, nitrate respiration and reduction and nitrogen respiration, all of them included genera identified in both, HHS and LHS farms. Regarding slat samples, the more relevant functions observed were fermentation and chemoheterotrophy, both related with the genera Fusobacterium, present in both experimental groups. Moreover, the functional annotation of more-environment-related samples (wall and slat) was performed. Results showed a total of 9 functional groups, of which the following are noteworthy: human pathogens, human-associated and animal parasites (related to Moraxella genus, and present in HHS and LHS samples), and nitrate and nitrogen respiration (related to Shewanella genus, only present in HHS samples).
Finally, for slurry samples, 29 functional groups were identified, of which it is interesting to highlight those related only to the genera Paracoccus (methanol oxidation, methylotrophy, nitrate denitrification, nitrite denitrification, nitrous oxide denitrification, and denitrification), aromatic compound degradation (Gordonia and Desulfatiglans), and intracellular parasites (Mitochondria), only identified in LHS farms.
4 Discussion
It is clearly demonstrated that controlling pathogenic microorganisms without the administration of antibiotics is one of the main challenges in modern livestock farming (35). Furthermore, strict antibiotic control in pig farming, the prohibition zinc oxide or the ban on highly effective field-level disinfectants such as formaldehyde make it very difficult to control animal diseases. After the implementation of all these mandatory regulations, this study has highlighted a significant difference in microbial communities established between farms that consistently experience health issues and those that consistently have good health outcomes. By understanding the differences between these populations in depth, we can develop tailored and effective protocols for each specific issue.
The microbial diversity of the samples was analysed according to alpha and beta diversity indexes. Although there were some differences in alpha diversity according to sample type (wall, slat or slurry), for all samples collected, significantly higher levels in beta diversity were observed for LHS farms. These findings are in agreement with data presented by Bridier et al. (2), which revealed that strict C&D procedures in pig slaughterhouses reduced the diversity of the bacterial community (2). This aligns with other studies indicating a decrease in microbial diversity when exposed to disinfectants (2, 36–40).
Regarding phyla composition, Firmicutes represented the dominant phylum of the environmental community in all the samples collected, followed by Proteobacteria in wall and slat samples, and by Bacteroidota in slurry samples. According to previous studies related to intestinal microbiota in pigs, Firmicutes is the dominant phylum during all the production cycle, followed by Proteobacteria at birth, and by Bacteroidota (formerly known as Bacteroides) during the rest of the production cycle, similar to the slurry results (41, 42). Both phyla are related to overall health of the animal, Firmicutes is involved in maintaining energy balance in the body, and Bacteroidota is associated with butyrate production and T cell-mediated immune responses, limiting the gastrointestinal colonisation by pathogens. In contrast, increased levels of Proteobacteria are repeatedly associated to intestinal inflammatory disorders (42–44).
At the genus level, 4 genera were present in all the samples analysed: Corynebacterium, Turicibacter, Lactobacillus and Terrisporobacter. Within them, Lactobacillus is known as a common inhabitant of the mammalian gastrointestinal tract, related to intestinal and immune system regulation, correct development of the intestinal microbiota and induction of competitive exclusion of pathogens by both adhesion to absorption sites of the intestinal mucosa and competition for nutrients (42, 45). Moreover, Turicibacter and Terrisporobacter, both significantly more abundant in LHS farms, are related to ether extract and protein digestibility, respectively, but considered opportunistic pathogens (41, 46, 47). However, the Corynebacterium genus, also significantly more abundant in LHS farms, contains opportunistic human pathogens asymptomatically carried by pigs (48).
Among the genera exclusively associated with HHS farms, beneficial and detrimental bacteria were observed. Some of the bacteria are related to beneficial effects to the host, such as Butyrivibrio, which belongs to butyrate-producing bacteria, with demonstrated beneficial effects on animals (49); Oscillospira, considered as a candidate for new probiotic generation with a strong association with body weight and animal health (50); Caproiciproducens, able to inhibit pathogenic bacteria, enhance animal immunity, and promote animal growth due to its ability to produce caproic acid (51, 52); or Devosia, a genus reported to be able to effectively reduce the toxicity of deoxynivalenol in diets for growing-finishing pigs (53). Furthermore, other genera are related to environmental health, such as Terrabacter, initially isolated from soil mixed with pig hair from Spain (54) and considered of ecological importance for its involvement in biological phosphate removal from wastewater and bioremediation processes (55). However, other genera were considered detrimental microbes, such as Asteroleplasma and Intestimonas, opportunistic pathogens related to heat stress and gut inflammation in growing pigs (50), Candidatus saccharimonas, involved in chronic inflammation, or Shewanella and Morganella, considered unusual opportunistic pathogens (56, 57).
Regarding the specific genera observed in LHS farms, more that are considered detrimental bacteria were observed. Some of them related to the host, such as Solibacillus, negatively correlated with crypt depth (58); or Sporobacter, suggested to have a negative role in gut health and associated with Salmonella positive free-range pigs (59, 60). There were also pathogens associated with ectoparasites, such as Wohlfahrtiimonas, causing several diseases in humans related to traumatic skin lesions, myiasis, wound contamination and sepsis (61); or Rickettsia, an obligate intracellular pathogen that causes diseases in humans, as well as domestic and wild animals, through vector transmission (62). Moreover, some genera were environment related, including Gelidibacter, associated with decomposition of pig carcasses (63); and Sulfuricurvum and Desulfatiferula, sulphur-reducing genera (64). On the other hand, two beneficial bacteria were detected, Anaerofustis, positively correlated with crude fibre digestibility (65), and Arenimonas, considered a soil probiotic (66).
Moreover, there were genera present in both experimental groups, but with significant differences in their abundance. Of these, noteworthy are 4 genera significantly more abundant in HHS farms: Leuconosctoc, with probiotic potential and antimicrobial effect such as lactic acid-producing bacteria (67); Weissella, also considered a potential probiotic with antimicrobial effect by producing compounds such as bacteriocins (68); Lactococcus, negatively correlated with methicillin-resistant Staphylococcus in healthy piglets, and with protective action in post-weaning diarrhoea and other intestinal infections in piglets (69, 70) and, in contrast, Streptococcus, one of the most important pathogens of pigs, causing septicemia, meningitis, arthritis, and endocarditis, especially in post-weaning pigs (71). Regarding the genera significantly more abundant in environmental samples (wall and slat) from LHS farms, it should be highlighted Clostridium_sensu_stricto_1, Terrisporobacter, and Turicibacter, previously associated to heat stress in pigs and considered opportunistic pathogens (46).
In addition, it is important to highlight some genera identified in the functional annotation analysis (FAPROTAX) because of their significant role in some important metabolic functions. There were some genera more-environmentally-related, including Fusobacterium (related to fermentation and chemoheterotrophy in slat samples), a genus with strong negative correlations with body weight gain in piglets and associated with diarrhoea and enteritis processes (72, 73); and Moraxella (human pathogen present in wall and slat samples), associated with infrequent opportunistic infections in humans and pigs. Furthermore, Moraxella has been described as a potential source of colistin resistance genes in pig farms (mcr-like genes, including new variants observed on pig production in China, mcr − 1.35 and mcr-1.36), as well as other antibiotic groups such as ampicillin, penicillin, quinolones, macrolides and tetracyclines (74, 75). Regarding the genera observed in each experimental group, it is important to note the role of Shewanella in iron respiration, a genus isolated from HHS group (as reported above). This process attracts much social and scientific attention due to its contribution to environmental pollution control and remediation field, promoting degradation of contaminants, such as aromatic compounds (76, 77). It is interesting to mention that many disinfectants used at the farm contain aromatic compounds among their active ingredients, that might have facilitated the persistence of this genus in the house environment. Finally, Paracoccus, a genus isolated from LHS farms and related to different functions (methanol oxidation, methylotrophy, nitrate denitrification, nitrite denitrification, nitrous oxide denitrification and denitrification), has previously been isolated from different environments, exhibiting excellent environmental adaptability, with a wide pH range and high concentrations of ammonia (78). In fact, it is reported that these bacteria facilitate different denitrification processes and participate in methanol oxidation, a process related to the presence of organic matter and exclusively from LHS farms (79, 80). However, it is essential to consider that functional annotations based on the FAPROTAX database provide inferred functions relying on 16S rRNA fragments, which may not be as precise as a comprehensive shotgun metagenomic study. For that reason, further studies such as metabolomics are needed to verify the functions and roles of these specific pathways in the different health status pig farms.
All the differences observed between the microbial populations of HHS and LHS, as well as the study of the functionality of the most prominent populations, emphasise the importance of knowing the individual situation of each farm when developing control methodologies, such as C&D protocols. With more specific, eco-friendly, and safe C&D protocols targeted against pathogenic microorganisms, we can achieve a much more sustainable livestock farming, as well as safer products for consumers and competitiveness in the market. While our results provide an initial insight into the relationship between environmental microbiota and farm health status, it’s important to note that pooling of samples was necessary to ensure adequate DNA quantity and quality. Further research is required to deepen our understanding of the role of environmental microbiota in farms with varying health statuses.
Data availability statement
The datasets generated and analysed are available at NCBI’s BioProject PRJNA1079579 and BioSample SAMN40044702.
Author contributions
CM: Conceptualization, Data curation, Funding acquisition, Investigation, Methodology, Project administration, Resources, Supervision, Validation, Visualization, Writing – original draft, Writing – review & editing. LM-G: Conceptualization, Data curation, Investigation, Methodology, Project administration, Resources, Supervision, Validation, Visualization, Writing – original draft, Writing – review & editing. JR: Investigation, Writing – review & editing. M-PV: Investigation, Writing – review & editing. MP-G: Investigation, Writing – review & editing. SV: Investigation, Writing – review & editing. CT-M: Investigation, Writing – review & editing. AM-F: Investigation, Writing – review & editing. LL-R: Data curation, Formal analysis, Investigation, Methodology, Software, Visualization, Writing – review & editing. LM-D: Data curation, Formal analysis, Investigation, Methodology, Software, Visualization, Writing – original draft, Writing – review & editing.
Funding
The author(s) declare financial support was received for the research, authorship, and/or publication of this article. This study was supported by the I + D + I National Program PID2021-125641OB-C22 and PID2021-125641OB-C21, MCIN/ AEI / 10.13039/501100011033 / FEDER, UE. We also acknowledge the CERCA program (Generalitat de Catalunya). CT-M is a PhD student from the Autonomous University of Barcelona, Microbiology Program, with an IRTA fellowship.
Acknowledgments
The authors of this paper would like to thank the swine industry sector and the involved companies for their technical support during the execution of this work. The authors also want to express gratitude to Dr. Avelino Álvarez-Ordóñez from the Department of Food Hygiene and Technology, Faculty of Veterinary, Universidad de León, León, Spain for his assistance. The English text version was revised by N. Macowan English Language Service.
Conflict of interest
The authors declare that the research was conducted in the absence of any commercial or financial relationships that could be construed as a potential conflict of interest.
The author(s) declared that they were an editorial board member of Frontiers, at the time of submission. This had no impact on the peer review process and the final decision.
Publisher’s note
All claims expressed in this article are solely those of the authors and do not necessarily represent those of their affiliated organizations, or those of the publisher, the editors and the reviewers. Any product that may be evaluated in this article, or claim that may be made by its manufacturer, is not guaranteed or endorsed by the publisher.
References
1. Casanova-Higes, A, Marín-Alcalá, CM, Andrés-Barranco, S, Cebollada-Solanas, A, Alvarez, J, and Mainar-Jaime, RC. Weaned piglets: another factor to be considered for the control of Salmonella infection in breeding pig farms. Vet Res. (2019) 50:45. doi: 10.1186/s13567-019-0666-7
2. Bridier, A, Le Grandois, P, Moreau, M, Prénom, C, Le Roux, A, Feurer, C, et al. Impact of cleaning and disinfection procedures on microbial ecology and Salmonella antimicrobial resistance in a pig slaughterhouse. Sci Rep. (2019) 9:12947–13. doi: 10.1038/s41598-019-49464-8
3. Andres, VM, and Davies, RH. Biosecurity measures to control Salmonella and other infectious agents in pig farms: a review. Compr Rev Food Sci Food Saf. (2015):14. doi: 10.1111/1541-4337.12137
4. Martelli, F, Lambert, M, Butt, P, Cheney, T, Tatone, FA, Callaby, R, et al. Evaluation of an enhanced cleaning and disinfection protocol in Salmonella contaminated pig holdings in the United Kingdom. PLoS One. (2017) 12:e0178897. doi: 10.1371/journal.pone.0178897
5. Coombs, K, Rodriguez-Quijada, C, Clevenger, JO, and Sauer-Budge, AF. Current understanding of potential linkages between biocide tolerance and antibiotic cross-resistance. Microorganisms. (2023) 11:2000. doi: 10.3390/microorganisms11082000
6. Callaway, TR, Morrow, JL, Edrington, TS, Genovese, KJ, Dowd, S, Carroll, J, et al. Social stress increases fecal shedding of Salmonella typhimurium by early weaned piglets. Curr Issues Intest Microbiol. (2006) 7:65–71.
7. Fox, LJ, Kelly, PP, Humphreys, GJ, Waigh, TA, Lu, JR, and McBain, AJ. Assessing the risk of resistance to cationic biocides incorporating realism-based and biophysical approaches. J Ind Microbiol Biotechnol. (2021) 49:kuab074. doi: 10.1093/jimb/kuab074
8. Keïta, A, Huneau-Salaün, A, Guillot, A, Galliot, P, Tavares, M, and Puterflam, J. A multi-pronged approach to the search for an alternative to formaldehyde as an egg disinfectant without affecting worker health, hatching, or broiler production parameters. Poult Sci. (2016) 95:1609–16. doi: 10.3382/ps/pew058
9. European Commission. Chemicals: the EU restricts exposure to carcinogenic substance formaldehyde in consumer products. (2023) [updated 14 July]. Available at: https://single-market-economy.ec.europa.eu/news/chemicals-eu-restricts-exposure-carcinogenic-substance-formaldehyde-consumer-products-2023-07-14_en (Accessed Feb 19, 2024).
10. Castañeda-Gulla, K, Sattlegger, E, and Mutukumira, AN. Persistent contamination of Salmonella, Campylobacter, Escherichia coli, and Staphylococcus aureus at a broiler farm in New Zealand. Can J Microbiol. (2020) 66:171–85. doi: 10.1139/cjm-2019-0280
11. Cobo-Díaz, JF, Alvarez-Molina, A, Alexa, EA, Walsh, CJ, Mencía-Ares, O, Puente-Gómez, P, et al. Microbial colonization and resistome dynamics in food processing environments of a newly opened pork cutting industry during 1.5 years of activity. Microbiome. (2021) 9:204. doi: 10.1186/s40168-021-01131-9
12. Álvarez-Ordóñez, A, and Briandet, R. Editorial: biofilms from a food microbiology perspective: structures, functions, and control strategies. Front Microbiol. (2016) 7:1938. doi: 10.3389/fmicb.2016.01938
13. Alvarez-Ordóñez, A, Coughlan, LM, Briandet, R, and Cotter, PD. Biofilms in food processing environments: challenges and opportunities. Annu Rev Food Sci Technol. (2019) 10:173–95. doi: 10.1146/annurev-food-032818-121805
14. Marin, C, Hernandiz, A, and Lainez, M. Biofilm development capacity of Salmonella strains isolated in poultry risk factors and their resistance against disinfectants. Poult Sci. (2009) 88:424–31. doi: 10.3382/ps.2008-00241
15. Gosling, RJ, Mawhinney, I, Vaughan, K, Davies, RH, and Smith, RP. Efficacy of disinfectants and detergents intended for a pig farm environment where Salmonella is present – ScienceDirect. Vet Microbiol. (2017) 204:46–53. doi: 10.1016/j.vetmic.2017.04.004
16. Alvarez-Ordóñez, A, Broussolle, V, Colin, P, Nguyen-The, C, and Prieto, M. The adaptive response of bacterial food-borne pathogens in the environment, host and food: implications for food safety. Int J Food Microbiol. (2015) 213:99–109. doi: 10.1016/j.ijfoodmicro.2015.06.004
17. Marin, C, Cerdà-Cuéllar, M, González-Bodi, S, Lorenzo-Rebenaque, L, and Vega, S. Research note: persistent Salmonella problems in slaughterhouses related to clones linked to poultry companies – ScienceDirect. Poult Sci. (2022) 101:101. doi: 10.1016/j.psj.2022.101968
18. Marin, C, Lorenzo-Rebenaque, L, Moreno-Moliner, J, Sevilla-Navarro, S, Montero, E, Chinillac, MC, et al. Multidrug-resistant Campylobacer jejuni on swine processing at a slaughterhouse in eastern Spain. Animals. (2021) 11:11. doi: 10.3390/ani11051339
19. Marin, C, Chinillac, MC, Cerdà-Cuéllar, M, Montoro-Dasi, L, Sevilla-Navarro, S, Ayats, T, et al. Contamination of pig carcass with Salmonella enterica serovar Typhimurium monophasic variant 1,4[5],12:i:- originates mainly in live animals. Sci Total Environ. (2020):703. doi: 10.1016/j.scitotenv.2019.134609
20. Montoro-Dasi, L, Lorenzo-Rebenaque, L, Ramon-Moragues, A, Pérez-Gracia, MT, de Toro, M, Marin, C, et al. Antibiotic removal does not affect cecal microbiota balance and productive parameters in LP robust rabbit line. Front Vet Sci. (2022) 9:1038218. doi: 10.3389/fvets.2022.1038218
21. Illumina. 16S metagenomic sequencing library preparation preparing 16S ribosomal RNA gene amplicons for the Illumina MiSeq system. (2022). Available at: https://support.illumina.com/documents/documentation/chemistry_documentation/16s/16s-metagenomic-library-prep-guide-15044223-b.pdf (Accessed May 25, 2024).
22. Babraham Bioinformatics. A quality control tool for high throughput sequence data. (2023). Available at: https://www.bioinformatics.babraham.ac.uk/projects/fastqc/ (Accessed Sept 13, 2022).
23. Bolyen, E, Rideout, JR, Dillon, MR, Bokulich, NA, Abnet, CC, Al-Ghalith, GA, et al. Reproducible, interactive, scalable and extensible microbiome data science using QIIME 2. Nat Biotechnol. (2019) 37:852–7. doi: 10.1038/s41587-019-0209-9
24. Quast, C, Pruesse, E, Yilmaz, P, Gerken, J, Schweer, T, Yarza, P, et al. The SILVA ribosomal RNA gene database project: improved data processing and web-based tools. Nucleic Acids Res. (2013) 41:D590–6. doi: 10.1093/nar/gks1219
25. Campos, PM, Darwish, N, Shao, J, and Proszkowiec-Weglarz, M. Research note: choice of microbiota database affects data analysis and interpretation in chicken cecal microbiota. Poult Sci. (2022) 101:101971. doi: 10.1016/j.psj.2022.101971
26. Lorenzo-Rebenaque, L, Casto-Rebollo, C, Diretto, G, Frusciante, S, Rodríguez, JC, et al. Examining the effects of Salmonella phage on the caecal microbiota and metabolome features in Salmonella-free broilers. Front Genet. (2022) 13:1060713. doi: 10.3389/fgene.2022.1060713
27. Lorenzo-Rebenaque, L, Casto-Rebollo, C, Diretto, G, Frusciante, S, Rodríguez, JC, Ventero, MP, et al. Modulation of Caecal Microbiota and Metabolome Profile in Salmonella-Infected Broilers by Phage Therapy. Int J Mol Sci. (2023) 24:15201. doi: 10.3390/ijms242015201
28. Rohart, F, Gautier, B, Singh, A, and Lê Cao, KA. mixOmics: An R package for ‘omics feature selection and multiple data integration. PLoS Comput Biol. (2017) 13:e1005752. doi: 10.1371/JOURNAL.PCBI.1005752
29. Galindo-Prieto, B, Eriksson, L, and Trygg, J. Variable influence on projection (VIP) for orthogonal projections to latent structures (OPLS). J Chemom. (2014) 28:623–632. doi: 10.1002/CEM.2627
30. Blasco, A. Bayesian data analysis for animal scientists: The basics. Bayesian Data Analysis for Animal Scientists. Basics (2017) 1–275. doi: 10.1007/978-3-319-54274-4/COVER
31. Metsalu, T, and Vilo, J. ClustVis: a web tool for visualizing clustering of multivariate data using principal component analysis and heatmap. Nucleic Acids Res. (2015) 43:W566–70. doi: 10.1093/nar/gkv468
32. Mandal, S, Van Treuren, W, White, RA, Eggesbø, M, Knight, R, and Peddada, SD. Analysis of composition of microbiomes: a novel method for studying microbial composition. Microb Ecol Health Dis. (2015) 26:27663. doi: 10.3402/mehd.v26.27663
33. Louca, S, Parfrey, LW, and Doebeli, M. Decoupling function and taxonomy in the global ocean microbiome. Science. (2016) 353:1272–7. doi: 10.1126/science.aaf4507
34. Zhou, L, Liu, L, Chen, W, Sun, J, Hou, S, Kuang, T, et al. Stochastic determination of the spatial variation of potentially pathogenic bacteria communities in a large subtropical river. Environ Pollut. (2020) 264:114683. doi: 10.1016/j.envpol.2020.114683
35. Mencía-Ares, O, Cabrera-Rubio, R, Cobo-Díaz, JF, Álvarez-Ordóñez, A, Gómez-García, M, Puente, H, et al. Antimicrobial use and production system shape the fecal, environmental, and slurry resistomes of pig farms. Microbiome (2020) 8:164. doi: 10.1186/s40168-020-00941-7
36. Costa, D, Mercier, A, Gravouil, K, Lesobre, J, Verdon, J, and Imbert, C. Occurrence and diversity of both bacterial and fungal communities in dental unit waterlines subjected to disinfectants. Pathog Dis. (2016) 74:ftw094. doi: 10.1093/femspd/ftw094
37. Forbes, S, Cowley, N, Humphreys, G, Mistry, H, Amézquita, A, and McBain, AJ. Formulation of biocides increases antimicrobial potency and mitigates the enrichment of nonsusceptible Bacteria in multispecies biofilms. Appl Environ Microbiol. (2017) 83:3054. doi: 10.1128/AEM.03054-16
38. Bertelli, C, Courtois, S, Rosikiewicz, M, Piriou, P, Aeby, S, Robert, S, et al. Reduced chlorine in drinking water distribution systems impacts bacterial biodiversity in biofilms. Front Microbiol. (2018) 9:2520. doi: 10.3389/fmicb.2018.02520
39. Reynolds-Clausen, K, Surridge-Talbot, K, Botes, M, and Eugene, CT. Bacterial species diversity as an indicator of dibromonitrilopropionamide (DBNPA) biocide efficacy. Water Sci Technol. (2018) 78:320–8. doi: 10.2166/wst.2018.289
40. Jiang, L, Li, M, Tang, J, Zhao, X, Zhang, J, Zhu, H, et al. Effect of different disinfectants on bacterial aerosol diversity in poultry houses. Front Microbiol. (2018) 9:2113. doi: 10.3389/fmicb.2018.02113
41. Shao, M, Wang, Z, He, Y, Tan, Z, and Zhang, J. Fecal microbial composition and functional diversity of Wuzhishan pigs at different growth stages. AMB Express. (2021) 11:88. doi: 10.1186/s13568-021-01249-x
42. Yang, Y, Liu, Y, Liu, J, Wang, H, Guo, Y, Du, M, et al. Composition of the fecal microbiota of piglets at various growth stages. Front Vet Sci. (2021) 8:661671. doi: 10.3389/fvets.2021.661671
43. Yang, Q, Huang, X, Zhao, S, Sun, W, Yan, Z, Wang, P, et al. Structure and function of the fecal microbiota in diarrheic neonatal piglets. Front Microbiol. (2017) 8:502. doi: 10.3389/fmicb.2017.00502
44. Zhang, W, Ma, C, Xie, P, Zhu, Q, Wang, X, Yin, Y, et al. Gut microbiota of newborn piglets with intrauterine growth restriction have lower diversity and different taxonomic abundances. J Appl Microbiol. (2019) 127:354–69. doi: 10.1111/jam.14304
45. Valeriano, VDV, Balolong, MP, and Kang, D. Probiotic roles of Lactobacillus sp. in swine: insights from gut microbiota. J Appl Microbiol. (2017) 122:554–67. doi: 10.1111/jam.13364
46. Hu, C, Niu, X, Chen, S, Wen, J, Bao, M, Mohyuddin, SG, et al. A comprehensive analysis of the colonic flora diversity, short chain fatty acid metabolism, transcripts, and biochemical indexes in heat-stressed pigs. Front Immunol. (2021) 12:717723. doi: 10.3389/FIMMU.2021.717723/BIBTEX
47. Lee, A, Le Bon, M, Connerton, IF, and Mellits, KH. Common colonic community indicators of the suckling pig microbiota where diversity and abundance correlate with performance. FEMS Microbiol Ecol. (2022) 98:fiac048. doi: 10.1093/femsec/fiac048
48. Poor, AP, Moreno, LZ, Matajira, CEC, Parra, BM, Gomes, VTM, Silva, APS, et al. Characterization of Corynebacterium diphtheriae, C. Confusum and C. amycolatum isolated from sows with genitourinary infection. Vet Microbiol. (2017) 207:149–52. doi: 10.1016/j.vetmic.2017.06.008
49. Kubasova, T, Davidova-Gerzova, L, Babak, V, Cejkova, D, Montagne, L, Le-Floc'h, N, et al. Effects of host genetics and environmental conditions on fecal microbiota composition of pigs. PLoS One. (2018) 13:e0201901. doi: 10.1371/journal.pone.0201901
50. Yang, J, Li, Y, Wen, Z, Liu, W, Meng, L, and Huang, H. Oscillospira – a candidate for the next-generation probiotics. Gut Microbes. (2021) 13:1987783. doi: 10.1080/19490976.2021.1987783
51. Hou, L, Wang, L, Qiu, Y, Xiong, Y, Xiao, H, Yi, H, et al. Effects of protein restriction and subsequent Realimentation on body composition, gut microbiota and metabolite profiles in weaned piglets. Animals (Basel). (2021) 11:686. doi: 10.3390/ani11030686
52. Zhang, W, Su, W, Li, P, Rao, H, Lin, Q, Zeng, X, et al. Naoxintong capsule inhibits the development of cardiovascular pathological changes in Bama Minipig through improving gut microbiota. Front Pharmacol. (2019) 10:1128. doi: 10.3389/fphar.2019.01128
53. Li, X, Guo, Y, Zhao, L, Fan, Y, Ji, C, Zhang, J, et al. Protective effects of Devosia sp. ANSB714 on growth performance, immunity function, antioxidant capacity and tissue residues in growing-finishing pigs fed with deoxynivalenol contaminated diets. Food Chem Toxicol. (2018) 121:246–51. doi: 10.1016/j.fct.2018.09.007
54. Montero-Barrientos, M, Rivas, R, Velázquez, E, Monte, E, and Roig, MG. Terrabacter terrae sp. nov., a novel actinomycete isolated from soil in Spain. Int J Syst Evol Microbiol. (2005) 55:2491–5. doi: 10.1099/ijs.0.63768-0
55. Stackebrandt, E, Scheuner, C, Göker, M, and Schumann, P. The family Intrasporangiaceae. In: E Rosenberg, DL EF, S Lory, E Stackebrandt, and F Thompson, editors. The prokaryotes: actinobacteria. Berlin, Heidelberg: Springer Berlin Heidelberg (2014). 397–424.
56. Alves, VBA, Carvalho, E, Madureira, PA, Marino, ED, Vaz, ACN, Vidal, AMC, et al. First isolation and whole-genome sequencing of a Shewanella algae strain from a swine farm in Brazil. BMC Microbiol. (2020) 20:360. doi: 10.1186/s12866-020-02040-x
57. Liu, H, Zhu, J, Hu, Q, and Rao, X. Morganella morganii, a non-negligent opportunistic pathogen. Int J Infect Dis. (2016) 50:10–7. doi: 10.1016/j.ijid.2016.07.006
58. Yang, Y, Li, Y, Xie, Y, Qiao, S, Yang, L, and Pan, H. Comparative study on Jejunal immunity and microbial composition of growing-period Tibetan pigs and Duroc × (landrace × Yorkshire) pigs. Front Vet Sci. (2022) 9:890585. doi: 10.3389/fvets.2022.890585
59. Zhang, W, Zhu, Y, Zhou, D, Wu, Q, Song, D, Dicksved, J, et al. Oral Administration of a Select Mixture of Bacillus probiotics affects the gut microbiota and goblet cell function following Escherichia coli challenge in newly weaned pigs of genotype MUC4 that are supposed to be Enterotoxigenic E. coli F4ab/ac receptor negative. Appl Environ Microbiol. (2017) 83:2747. doi: 10.1128/AEM.02747-16
60. Garrido, V, Migura-García, L, Gaitán, I, Arrieta-Gisasola, A, Martínez-Ballesteros, I, Fraile, L, et al. Prevalence of Salmonella in free-range pigs: risk factors and intestinal microbiota composition. Food Secur. (2021) 10:1410. doi: 10.3390/foods10061410
61. Kopf, A, Bunk, B, Coldewey, SM, Gunzer, F, Riedel, T, and Schröttner, P. Identification and antibiotic profiling of Wohlfahrtiimonas chitiniclastica, an underestimated human pathogen. Front Microbiol. (2021) 12:712775. doi: 10.3389/fmicb.2021.712775
62. Cleveland, CA, Swanepoel, L, Box, EK, De Nicola, A, and Yabsley, MJ. Rickettsia species in ticks collected from wild pigs (Sus scrofa) and Philippine deer (Rusa marianna) on Guam, Marianna Islands, USA. Acta Trop. (2019) 194:89–92. doi: 10.1016/j.actatropica.2019.03.010
63. Ki, B, Kim, YM, Jeon, JM, Ryu, HW, and Cho, K. Characterization of bacterial community dynamics during the decomposition of pig carcasses in simulated soil burial and composting systems. J Microbiol Biotechnol. (2017) 27:2199–210. doi: 10.4014/jmb.1709.09032
64. Mitchell, HM, Rocha, GA, Kaakoush, NO, O’Rourke, JL, and Queiroz, DMM. The family Helicobacteraceae. In: E Rosenberg, EF DeLong, S Lory, E Stackebrandt, and F Thompson, editors. The prokaryotes: Deltaproteobacteria and Epsilonproteobacteria. Berlin, Heidelberg: Springer Berlin Heidelberg (2014). 337–92.
65. Niu, Q, Li, P, Hao, S, Kim, SW, Du, T, Hua, J, et al. Characteristics of gut microbiota in sows and their relationship with apparent nutrient digestibility. Int J Mol Sci. (2019) 20:870. doi: 10.3390/ijms20040870
66. Zhang, Y, Chen, M, Bao, C, Wu, J, Zhou, J, He, W, et al. Application of pig manure compost with different biochar modifies the antibiotic Resistome and bacterial Community in Agriculture Soil. Water Air Soil Pollut. (2022) 233:233. doi: 10.1007/s11270-022-05582-0
67. Espinosa-Gongora, C, Larsen, N, Schønning, K, Fredholm, M, and Guardabassi, L. Differential Analysis of the Nasal Microbiome of Pig Carriers or Non-Carriers of Staphylococcus aureus. PLoS ONE. (2016) 11:e0160331. doi: 10.1371/journal.pone.0160331
68. Fanelli, F, Montemurro, M, Chieffi, D, Cho, GS, Franz, CMAP, Dell’Aquila, A, et al. Novel insights into the phylogeny and biotechnological potential of weissella species. Front Microbiol. (2022) 13:914036. doi: 10.3389/FMICB.2022.914036/BIBTEX
69. Rattigan, R, Wajda, L, Vlasblom, AA, Wolfe, A, Zomer, AL, Duim, B, et al. Safety Evaluation of an Intranasally Applied Cocktail of Lactococcus lactis Strains in Pigs. Animals (2023) 13:3442. doi: 10.3390/ANI13223442/S1
70. Yu, D, Xia, Y, Ge, L, Tan, B, and Chen, S. Effects of Lactococcus lactis on the intestinal functions in weaning piglets. Front Nutr. (2021) 8:713256. doi: 10.3389/FNUT.2021.713256/BIBTEX
71. Gottschalk, M. Lucha para controlar la enfermedad por “Streptococcus suis” en el contexto de la reducción de antibióticos (II). MG - Mundo ganadero. (2024) 35:34–37.
72. Dee, S. Necrotic rhinitis in pigs-respiratory system. (2022) [updated Oct]. Available at: https://www.msdvetmanual.com/respiratory-system/respiratory-diseases-of-pigs/necrotic-rhinitis-in-pigs (Accessed Feb 14, 2024).
73. De Witte, C, Flahou, B, Ducatelle, R, Smet, A, De Bruyne, E, Cnockaert, M, et al. Detection, isolation and characterization of Fusobacterium gastrosuis sp. nov. colonizing the stomach of pigs. Syst Appl Microbiol. (2017) 40:42–50. doi: 10.1016/j.syapm.2016.10.001
74. AbuOun, M, Stubberfield, EJ, Duggett, NA, Kirchner, M, Dormer, L, Nunez-Garcia, J, et al. Mcr-1 and mcr-2 variant genes identified in Moraxella species isolated from pigs in Great Britain from 2014 to 2015. J Antimicrob Chemother. (2017) 72:2745–9. doi: 10.1093/jac/dkx286
75. Che, Y, Wu, R, Li, H, Wang, L, Wu, X, Chen, Q, et al. Characterization of two novel colistin resistance gene mcr-1 variants originated from Moraxella spp. Front Microbiol. (2023) 14:1153740. doi: 10.3389/fmicb.2023.1153740
76. Zou, H, Zhou, Z, Xia, H, Zhao, Q, and Li, X. Characterization of chromosome-mediated BlaOXA-894 in Shewanella xiamenensis isolated from pig wastewater. Int J Environ Res Public Health. (2019) 16:3768. doi: 10.3390/ijerph16193768
77. Li, Z, Ding, L, Zhu, W, and Hang, S. Effects of the increased protein level in small intestine on the colonic microbiota, inflammation and barrier function in growing pigs. BMC Microbiol. (2022) 22:172. doi: 10.1186/s12866-022-02498-x
78. Heo, J, Cho, H, Kim, J, Hong, S, Kwon, S, and Kim, S. Paracoccus suum sp. nov., isolated from a pig farm dust collector. Int J Syst Evol Microbiol. (2019) 69:970–4. doi: 10.1099/ijsem.0.003226
79. Moloantoa, KM, Khetsha, ZP, van Heerden, E, Castillo, JC, and Cason, ED. Nitrate water contamination from industrial activities and complete denitrification as a remediation option. Water. (2022) 14:14. doi: 10.3390/w14050799
Keywords: environmental microbiome, pig production, 16S rRNA sequencing, farm health status, cleaning and disinfection protocols
Citation: Marin C, Migura-García L, Rodríguez JC, Ventero M-P, Pérez-Gracia MT, Vega S, Tort-Miró C, Marco-Fuertes A, Lorenzo-Rebenaque L and Montoro-Dasi L (2024) Swine farm environmental microbiome: exploring microbial ecology and functionality across farms with high and low sanitary status. Front. Vet. Sci. 11:1401561. doi: 10.3389/fvets.2024.1401561
Edited by:
Domenico Vecchio, Experimental Zooprophylactic Institute of Southern Italy (IZSM), ItalyReviewed by:
Terence L. Marsh, Michigan State University, United StatesRichard E. Isaacson, University of Minnesota Twin Cities, United States
Copyright © 2024 Marin, Migura-García, Rodríguez, Ventero, Pérez-Gracia, Vega, Tort-Miró, Marco-Fuertes, Lorenzo-Rebenaque and Montoro-Dasi. This is an open-access article distributed under the terms of the Creative Commons Attribution License (CC BY). The use, distribution or reproduction in other forums is permitted, provided the original author(s) and the copyright owner(s) are credited and that the original publication in this journal is cited, in accordance with accepted academic practice. No use, distribution or reproduction is permitted which does not comply with these terms.
*Correspondence: Clara Marin, Y2xhcmEubWFyaW5AdWNoY2V1LmVz; Lourdes Migura-García, bG91cmRlcy5taWd1cmFAaXJ0YS5jYXQ=