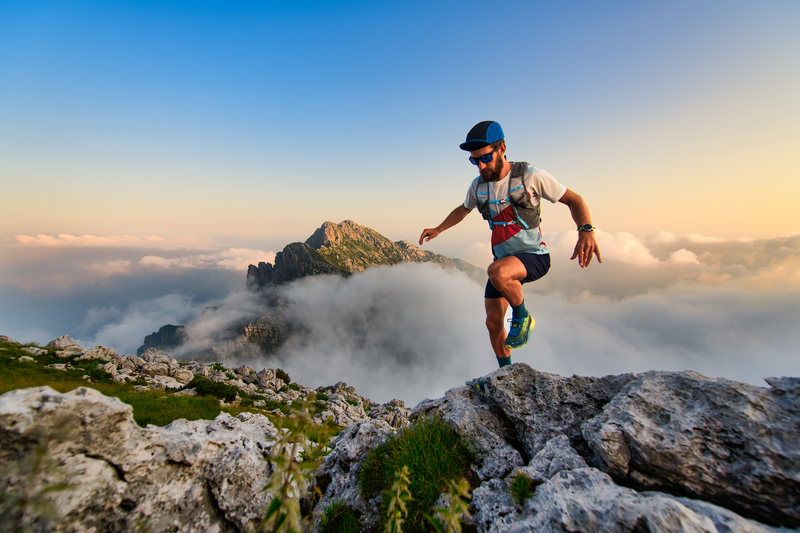
94% of researchers rate our articles as excellent or good
Learn more about the work of our research integrity team to safeguard the quality of each article we publish.
Find out more
ORIGINAL RESEARCH article
Front. Vet. Sci. , 02 May 2024
Sec. Parasitology
Volume 11 - 2024 | https://doi.org/10.3389/fvets.2024.1399772
This article is part of the Research Topic Advancements in Understanding Zoonotic Parasitic Diseases View all 17 articles
Parts of this article's content have been modified or rectified in:
Erratum: Analysis of the current risk of Leishmania infantum transmission for domestic dogs in Spain and Portugal and its future projection in climate change scenarios
Canine leishmaniosis, caused by the protozoan parasite Leishmania infantum, is a cosmopolitan vector-borne zoonosis, transmitted principally by Phlebotomus perniciosus in Spain and Portugal, where it is considered an endemic disease. Ecoinformatics tools such as ecological niche models (ENM) have been successfully tested to model the distribution of the risk of infection of different parasitosis as they take into account environmental variables vital for their survival. The risk map proposed in this study combines the potential distribution of Ph. perniciosus in the Iberian Peninsula and the calculation of the infection rate of the parasite in the vector to model the risk of contracting the disease in a more realistic way. In fact, this weighting strategy improves the predictive power of the resulting model (R2 = 0.42, p = < 0.01) compared to the Ph. perniciosus ENM model alone (R2 = 0.13, p > 0.05). The places with the highest risk of transmission are the southwest and central peninsular area, as well as the Mediterranean coast, the Balearic Islands and the Ebro basin, places where the ideal habitat of Ph. perniciosus and the infection rate is also high. In the case of future projections under climate change scenarios, an increase in the risk of infection by L. infantum can be observed in most of the territory (4.5% in 2040, 71.6% in 2060 and 63% in 2080), mainly in the northern part of the peninsula. The use of ENMs and their weighting with the infection rate in Ph. perniciosus is a useful tool in predicting the risk of infection for L. infantum in dogs for a given area. In this way, a more complete model can be obtained to facilitate prevention and control.
Vector-borne zoonotic diseases pose significant health challenges for both animals and humans, accounting for 61% of human diseases of zoonotic origin (1–4). These diseases are increasingly prevalent across the European continent due to globalization and climate change. Factors such as rising temperatures, vector movement, increased migration and tourism involving infected people and animals, and inadequate management diseases control measure among other factors, contribute to this trend (5–8).
Canine leishmaniosis stands as a vector-borne zoonotic disease caused by Leishmania infantum, a protozoan parasite that affecting both animals and humans alike, with dogs being the main domestic reservoir. Its primary vectors in Iberian Peninsula are Phlebotomus perniciosus and Phlebotomus ariasi species (9, 10). These, when feeding on blood from the definitive host, ingest amastigotes (tissue form), which then develop to promastigotes (infective form) in the intestine of the vector and subsequently migrate to the proboscis (11). This process is temperature-dependent, increasing logarithmically the percentage of sandflies infected by L. infantum between 10 and 30°C, the survival range of the vector (12).
Its distribution is cosmopolitan and dynamic, both spatially and temporally, subject to multiple social and environmental factors. In Europe, the countries located in the Mediterranean basin (France, Greece, Italy, Spain, and Portugal) are endemic, with a much higher incidence of canine leishmaniosis than human leishmaniosis (13). Within the entire peninsular and insular territory of Spain and Portugal, most of its surface is considered endemic. In Spain, reported seroprevalences range from 0.86 to 24.66%, with the highest reports in the south and on the Mediterranean coast (14–16). In Portugal, canine leishmaniosis is found throughout the territory with a heterogeneous distribution, with the highest seroprevalence observed in the center of the country, with values close to 30% (13).
In the context of prevention and control of animal and human leishmaniosis, it is essential to emphasize the various tools used for the prevention of infection (17). One of these is mapping to visualize areas where there is a risk of disease infection, as it allows early identification of risk areas, facilitates planning of interventions, optimizes resource allocation, supports epidemiological surveillance and improves risk communication to the population. Ecoinformatics tools, such as Geographic Information Systems (GIS) and ecological niche models (ENM), can be employed to manage zoonotis parasitosis. These tools facilitate modeling the distribution of the disease by considering the bioclimatic and environmental variables necessary for their maintenance (18). ENMs assign suitability values to the environmental habitats where an organism lives, achieved through the correlation between the known distribution records of the species and the environmental variables that influence it (19). These models have already been used to assess the potential risk of zoonotic disease transmission utilizing records of parasite presence, infected hosts (20, 21) and potential transmitting vectors (22, 23).
For leishmaniosis, these tools have been specifically applied specifically to the Mediterranean basin and other parts of the world to model the risk of infection concerning environmental variables such as precipitation, temperature, and vegetation (17, 24–32). Local studies in the Iberian Peninsula have assessed the risk of L. infantum infection using GIS tools. The initial risk map was constructed in the community of Madrid based on the distribution of vectors (Ph. perniciosus and Ph. ariasi), indicating high risk nuclei in individualized foci in the Center and South of the region (33). The second study, in East-Central Portugal, was focused solely on the presence of infected hosts, and suggests that irrigated crops and olive groves, open forests, and watercourses influence infection distribution (34). However, these studies did not integrate ecological niche models of the vectors with parasite development within them, extracting the full potential of these techniques and being much more realistic. The ability to model the development of the parasite inside the vectors together with the distribution of the latter through ENMs, has made infection risk mapping a supplementary tool in control plans for other vector-borne diseases, such as dirofilariosis on a larger scale (35–37).
The aim of this study was to develop an infection risk map for L. infantum in the Iberian Peninsula (Spain and Portugal) and the Balearic Islands as well as its projection to 2080 through the use of ENM, taking into account the habitat suitability of Ph. perniciosus, its main vector in the study area, and the calculation of the infection rate of L. infantum in the vector.
The Iberian Peninsula (40°14′24” N 4°14′21” W), formed by the countries of Spain and Portugal, and the Balearic Islands (Spain), were established as a study area. This territory is located in the southeast of the European continent, close to Morocco (Africa) and only separated from it by the Strait of Gibraltar (Figure 1). Both Spain and Portugal have overseas territories such as the Canary Islands, the Azores or Madeira, but these have not been taken into account in this study due to the particularity of their biogeographical characteristics, which are very different from those of the continent. The Iberian Peninsula covers a territory of approximately 590,000 km2 and the Balearic Islands 4,992 km2. Most of the peninsular territory is surrounded by coastline, surrounded to the east and south by the Mediterranean Sea, to the north by the Cantabrian Sea and to the west by the Atlantic Ocean. Regarding the continental territory, it is mostly made up of a large plateau with an average altitude of 600 meters crossed by both large hydrographic basins and a multitude of mountain ranges, providing the peninsula with a great diversity of environments. The mountain ranges that divide the peninsula are the Pyrenees, the Cantabrian Mountains, the Iberian System, the Central System and the Penibaetic System. The basins of the Ebro River (northeast) and the Guadalquivir River (south) are the main river basins of the peninsula, followed by other smaller basins such as the Guadiana (southwest), Júcar and Segura (east), Duero and Tajo (west) and Miño (northwest).
The Iberian Peninsula has a wide variety of climates, which give it a great biological importance. The northwest of the peninsula is an area of cool summers, mild winters and high humidity and precipitation throughout the year. The Levantine coast is an area with a Mediterranean climate with hot, dry summers and mild winters. The south is characterized by a warm and dry African-influenced climate with summer drought; while, in the central plateau, whipped by strong winds, the climate is very hot in summer and very cold in winter with rainfall normally restricted to spring and autumn. It is worth noting the notable difference in the climate with respect to the altitude, with the high mountain areas having mild summers and being covered with snow in winter (38).
To model habitat suitability for Ph. perniciosus, the main vector of canine leishmaniosis in the Iberian Peninsula and Europe (14, 39–41), we collected records of presence of this species from published studies (9, 42–44) and Global Biodiversity information Facility (GBIF) (45) to obtain the most representative face-to-face attendance possible. In order to obtain only presence points and eliminate sampling biases, the data were processed at a resolution of 1 km2. Finally, 3,032 vector presence data were obtained for use in the model.
At a spatial resolution of 1 km2, 19 bioclimatic variables related to temperature and precipitation were downloaded from World Clim (46), for present-day conditions and projected scenarios for 2040, 2060, and 2080 (47). Subsequently, a multicollinearity analysis was performed on these variables in the R software using Pearson’s correlation coefficient (48). To avoid cross-correlation between the 19 bioclimatic variables, those with a value of r > ±0.75 were eliminated and taking into account the biological needs of the vector, the variables chosen were BIO1 (Annual Mean Temperature), BIO2 (Mean Diurnal Range: The mean of the monthly temperature ranges), BIO3 (Isothermality: Mean Diurnal Range (BIO2) / Temperature Annual Range (BIO7) × 100), BIO8 (Mean Temperature of Wettest Quarter), BIO12 (Annual Precipitation) and BIO15 (Precipitation Seasonality: Standard deviation of weekly or monthly precipitation values as a percentage of the mean of those values). Next, the environmental variables were downloaded: density of shrubs and herbaceous plants (49) and the human footprint (50) which includes 8 variables (built environment, population density, electric power infrastructure, farmland, grazing land, roads, railways and waterways) reflecting the impact of human activities on the ecosystem. All downloaded data layers were processed in ArcMap 10.8 to ensure uniform extent, resolution (1 km2 per pixel) and coordinates system (GCS_WGS_1984).
The maximum entropy algorithm MaxEnt was used (51) to model the vector’s ecological niche from the Kuenm package of the R software (version 4.3.0) (48), automating the process (52). MaxEnt employs points of presence and environmental variables to estimate habitat suitability, which can be defined as the area in where specific environmental conditions necessary for the survival or reproduction of a species exist (53). To model Ph. perniciosus, 119 models were created with Kuenm for a set of variables, 17 regularization multiplier values (0.1–1.0 at 0.1 intervals, 2–6 at intervals of 1, 8, and 10) and the seven possible combinations of three feature classes (linear, quadratic, and product). The performance of the models created was assessed considering the significance of the partial receiver operating characteristic (partial ROC), with 100 iterations and 50% data for bootstrapping, skip rates (OR = 5%) and model complexity (Akaike information criterion - AIC). From the models that met the evaluation criteria, the final model was chosen based on the mean ratio of the area under the curve (AUC) obtained with points of occurrence independent of the calibration. The best-fit model (final model) was generated using the same parameters selected in the previous step. Ten replicates were developed per bootstrap with logistic outputs, and re-evaluated based on criteria ROC_parcial, OR and AICc.
The infection rate (% of Ph. perniciosus infected by L. infantum) was calculated as Rioux et al. (12), applied to our vector, by using the formula ] ( is % of Ph. perniciosus infected by L. infantum and is the Annual Mean Temperature). The frequency distribution was adjusted to a theoretical ascending logarithmic curve, which allowed estimating the infection rate between 10 and 30°C, which is the temperature range in which the parasite can survive and replicate in the Ph. ariasi vector, as reported by Rioux et al. (12). The infection rate was carried out using the program R-4.3.0.
An infection risk map for L. infantum is a visual representation that identifies the geographical areas, within a given environment, where a certain risk of parasite transmission (high, medium or low) may exist. The risk of L. infantum infection refers to the probability that a host may become infected taking into account different factors such as the presence of the vector, the prevalence of the disease in a given population, the presence of natural reservoirs of the pathogen, environmental conditions favorable for vector reproduction, the availability of standing water for vector reproduction, and the proximity between vectors and hosts, among other factors. To generate the risk map of L. infantum infection in the study area, once the final ENM for Ph. perniciosus was generated, it was multiplied using a weighting approach with the map of the infection rate of L. infantum in Ph. perniciosus.
Our risk map was validated employing a regression analysis between the mean risk of infection and the seroprevalence of canine disease in all the autonomous communities of Spain and regions of Portugal, reported by Almeida et al. (13) and Montoya-Alonso et al. (16). In addition, geolocations of dogs infected by L. infantum were superimposed on the risk map. The geolocation of these infected animals is also derived from the same studies previously employed (13, 16). Simultaneously, seroprevalence data were compared using the unweighted vector ENM with the same approximation.
Three suitable habitats for Ph. perniciosus were generated with the previously selected parameters, incorporating projections of the bioclimatic variables analyzed for the time periods 2021–2040 (2040), 2041–2060 (2060) and 2061–2080 (2080). The RCP 8.5 scenario, which represents high CO2 emissions, was utilized using the HadGEM3-GC21-LL model (54), to study the effect of climate change in the future, because of high greenhouse gas emissions in Europe (55). The infection rate of L. infantum corresponding to each of the three future scenarios was also calculated using the BIO1 (Annual Mean Temperature) of each time period.
The suitability habitats were weighted with the rate of infection of L. infantum and risk maps corresponding to each of the three periods analyzed were generated. Subsequently, the current risk map and the three projected future risk maps were transformed into presence/absence binary maps using the logistic threshold of training presence of the 10th percentile of the current map. This process is essential to perform a range-change analysis in order to establish alterations in the risk of L. infantum infection in the future. Finally, the percentage of cells that gained or lost risk of infection as a result of climate change was calculated for the maps projected to 2040, 2060, and 2080 compared to the present map using the biomod2 package of the R software (56).
Figure 2 shows the developed ENM, indicating the suitability of Ph. perniciosus habitat across the Iberian Peninsula and the Balearic Islands. The maximum suitability value recorded was 0.83 (high suitability), while the minimum value was 0.0004 (low suitability). Table 1 shows the contribution degree of each of the variables in the vector model, with the variables with the highest percentage of contribution attributions to the human footprint (40.14%) and the BIO1 (20.83%). The remaining variables contribute between 9.37 and 2.53%, with the latter being the lowest percentage. Those areas with a high suitability value correspond to the southwest of the peninsula, followed by others such as the center, the Levantine coast and the Balearic Islands, the Ebro basin and some areas of the north coast of the peninsula. Conversely, regions in the interior northwest and the central east, as well as the mountainous areas characterized by lower population density and cooler temperatures, exhibits less suitable habitat.
Figure 2. Ecological niche model (suitable habitat) for Phlebotomus perniciosus in Iberian Peninsula (Portugal and Spain) and Balearic Islands (Spain).
Table 1. Analysis of the contribution of the 9 environmental and bioclimatic variables to the ecological niche model for Phlebotomus perniciosus.
Figure 3 illustrates the potential resulting map depicting the rate of L. infantum infection in Ph. perniciosus across the Iberian Peninsula and the Balearic Islands. Areas with the highest infection rate are concentrated to those of the southwest and south of the peninsula, the Mediterranean coast, the Balearic Islands and the Ebro basin, and the north and northwest coasts of the territory. The northern plateau had a medium infection rate, while the mountain areas, at higher altitudes display percentages close to 0.
Figure 3. Prediction of Phlebotomus perniciosus infection rate in Iberian Peninsula (Portugal and Spain) and Balearic Islands (Spain).
Figure 4 presents the map with the potential risk of transmission by L. infantum in the mainland and the Balearic Islands. Different infection risk values are represented using a color palette from red to blue, with the highest value being 0.56 and the lowest 0. The territory is divided into five risk ranges established by natural jenks (Very High, High, Medium, Low and Very Low), with 23.8% of the study area identified as very high/high risk areas, 23% as medium risk areas, and 53.2% as low/very low risk areas. There is a risk of infection throughout the study area except for high-altitude areas. The places with the highest risk of transmission correspond to the southwest and center of the peninsula, as well as the coast near the Mediterranean Sea, the Balearic Islands and the Ebro basin, places which coincide with areas characterized by ideal Ph. perniciosus habitats and high infection rate. Areas such as the northern plateau and the north and northwest coast have intermediate risk values. Areas of the interior of the peninsula, mountainous areas and higher altitudes with cooler temperatures exhibit risk values close to zero.
Figure 4. Ecological niche model of the risk of Leishmania infantum infection in Iberian Peninsula (Portugal and Spain) and Balearic Islands (Spain).
The result of the regression calculation was a positive and significant relationship between the infection risk map for L. infantum and the seroprevalence in infected dogs by each autonomous community in Spain and regions in Portugal (β ± SE = 61.15 ± 16.39, R2 = 0.42, p < 0.01) (Figure 5). The results obtained from the unweighted vector ENM did not fit significantly with the seroprevalence data (β ± SE = 20.79 ± 12.38, R2 = 0.13, p > 0.05), highlighting the importance of combining it with the infection rate.
Figure 5. Regression plot for the validation of the ecological niche model between the mean risk of infection and disease prevalence in dogs most recently to date in all Spanish autonomous communities and Portuguese regions of the Iberian Peninsula and in the Balearic Islands (Spain) reported by Almeida et al. (13) and Montoya-Alonso et al. (16).
Regarding the dogs infected with L. infantum and geolocated, 82.6% were in areas estimated to be at very high/high risk areas, 13.2% in medium risk areas and 4.2% in low/very low risk areas (Figure 6).
Figure 6. Ecological niche model of the risk of Leishmania infantum infection in Iberian Peninsula (Portugal and Spain) and Balearic Islands (Spain) and the geolocations of infected dogs in Iberian Peninsula and Balearic Islands (Spain).
In the projection of potential transmission risk maps to the three future scenarios (2040, 2060 and 2080) of L. infantum through Ph. perniciosus, a latitudinal shift of the risk of infection toward the north of the peninsula is observed in both 2060 and 2080 (Figure 7). When the range-change analysis was carried out, the percentage of the territory where the risk of infection for L. infantum increases was in the North of the peninsula with 4.5% by 2040, 71.6% in 2060 and 63% in 2080. However, there is also a loss in the percentage of territory where there is a risk of infection, mainly in the south of the peninsula, being 9.6, 14.4 and 27.9% for 2040, 2060 and 2080, respectively.
Figure 7. Projections of the risk of Phlebotomus perniciosus infection for 2040 (A), 2060 (B) and 2080 (C) in Iberian Peninsula (Portugal and Spain) and Balearic Islands (Spain) under the climate change scenario RCP 8.5.
This study provides quantitative data on the risk of L. infantum infection in Iberian Peninsula (Spain and Portugal) and the Balearic Islands (Spain). The novelty of this study lies in the weighted use of both the calculation of habitat suitability by ENM of Ph. perniciosus, and the infection rate of L. infantum in the sandfly to predict the presence of the vector and the infectivity of the disease more accurately.
Prior to our study, the methodology of the ENMs has already been applied to try to model the distribution of leishmaniosis both in Europe and in other continents, using data on either the presence of vectors or infected hosts (17, 24–32). The same is applied to the Iberian Peninsula, where there are only two GIS studies have individually use the records of infected hosts or the distribution of their vectors, respectively (33, 34).
The risk map proposed in this work combines the potential distribution of the main vector of L. infantum in the Iberian Peninsula and the calculation of the parasite infection rate in the vector to model the risk of contracting the disease in a more realistic way. In fact, this weighting strategy improves the predictive power of the resulting model (R2 = 0.42, p = < 0.01) compared to the Ph. perniciosus suitability model alone (R2 = 0.13, p = > 0.05).
The variables that contribute most to explaining the potential distribution of Ph. perniciosus are the human footprint (built environment, population density, electric power infrastructure, cropland, grazing land, roads, railways, and waterways) and BIO1 (Mean Annual Temperature). Areas where human pressure is high are an ideal habitat for the maintenance of Ph. perniciosus populations. These areas with high anthropic presence, such as parks and agricultural land, also have important reservoirs of L. infantum (rabbits, rats, cats) associated with them, making it possible to efficiently maintain the biological cycle of canine leishmaniosis with high loads of infected sandflies (57–63). In addition, high prevalences of L. infantum infection in urban lagomorph populations have been linked to recent outbreaks of human leishmaniosis in Spain (57, 62), where annual incidences in humans (0.4–3.18 cases/100,000 inhabitants) and different prevalences in animals [29% in foxes (Vulpes vulpes), 13% in beech martens (Martes foina), 33% in wolves, 33.3% in rats, 15.6% in stray cats, 100% in rabbits, 8% in badgers and 1/3 of infected Egyptian mongooses] with special presence in southeastern Spain (11, 60–63) have been reported. On the other hand, the average annual temperature has a positive influence on the biology and ecology of the sandfly (rate of egg production, development of juvenile stages, annual number of generations, feeding behavior, period of activity and survival of adults) (64, 65). Other variables with a minor influence on the suitability models obtained include the diurnal mean range and the seasonality of rainfall. This last variable is also associated with the habitat types identified as influencing the distribution of hares and other wild reservoirs of L. infantum (natural grasslands, coniferous forests, lands occupied mainly by agriculture, lands with significant areas of natural vegetation and non-irrigated farmlands) that are characterized by moderate to high annual rainfall (66). Currently, areas with a higher seroprevalence of L. infantum in Spain suffer from drought, which may negatively influence sandfly populations and affect the transmission of the disease.
Regarding the variables associated with the rate of infection of L. infantum, the average annual temperature also influences its development, with the percentage of infected sandflies increasing logarithmically as the temperature rises within their survival ranges (12).
Our combined risk model indicates that actually, areas of the interior of the peninsula, mountainous and higher altitude areas with low temperatures, (which decrease both the habitat suitability of the vector and the rate of infection of these by the parasite) have risk values close to 0. On the other hand, the areas with a higher risk of infection (the south-west and center of the Peninsula, as well as the coast near the Mediterranean Sea, the Balearic Islands and the Ebro basin) coincide with areas with a high human presence, high average annual temperatures and with the basins of large rivers such as the Tajo, the Ebro and the Guadalquivir.
In the case of future projections under climate change scenarios, an increase in the risk of infection by L. infantum can be observed in most of the territory (4.5% in 2040, 71.6% in 2060 and 63% in 2080), mainly in the northern part of the peninsula. However, in some areas of the south of the territory, there would be a decrease in risk over time (9.6% in 2040, 14.4% in 2060 and 27.9% in 2080), which may be due to the foreseeable decrease in water resources, and the reduction of wetlands and vegetation in these areas (67, 68). This work predicts that canine leishmaniosis, in line with other vector-borne diseases, will shift latitudinally and toward higher altitude areas, altering its dynamics both spatially and temporally, colonizing areas where it was previously absent (22, 32, 35, 36, 69, 70). The effect of climate change on the seasonality and distribution of these types of vector-borne diseases will be more pronounced within the temperature ranges conducive to transmission occurs (71, 72).
As future approaches to applying of ENMs in vector-borne zoonotic diseases, it is possible to use the weighting tool not only with the niche model of one of its vectors, but also with more than one that inhabit the same territory, each with different ecological niches, provides sufficient data are available to model their distribution. In this way, a more comprehensive model could be obtained to facilitate the prevention and control of these diseases by veterinary and other specialist personnel.
The raw data supporting the conclusions of this article will be made available by the authors, without undue reservation.
IR-E: Formal analysis, Investigation, Methodology, Validation, Writing – original draft, Writing – review & editing, Data curation, Software. AB-d: Investigation, Methodology, Validation, Writing – original draft, Writing – review & editing, Data curation, Formal analysis, Software. MC-C: Formal analysis, Investigation, Methodology, Writing – review & editing. DB-B: Investigation, Writing – review & editing. SD-E: Investigation, Writing – review & editing. REH-L: Investigation, Supervision, Validation, Writing – review & editing. JÁS-A: Conceptualization, Investigation, Methodology, Supervision, Validation, Writing – review & editing. RM: Conceptualization, Funding acquisition, Investigation, Methodology, Project administration, Resources, Supervision, Validation, Visualization, Writing – original draft, Writing – review & editing.
The author(s) declare that financial support was received for the research, authorship, and/or publication of this article. This study received funding from CEVA Salud Animal S.A. and General Foundation of University of Salamanca (LANZADERA_2023). The funders were not involved in the study design, collection, analysis, interpretation of data, the writing of this article, or the decision to submit it for publication. RH-L was supported by the Spanish Ministerio de Ciencia, Innovación y Universidades through a Juan de la Cierva Grant (JDC2022-050186-I) of the Programa Estatal para Desarrollar, Atraer y Retener Talento, IR-E was supported by University of Salamanca-Banco Santander predoctoral scholarship, MC-C was supported by CLAVE Program and General Foundation of University of Salamanca and AB-d was supported by Margarita Salas grants for the training of young doctors (University of Barcelona), funded by Ministerio de Universidades and Plan de Recuperación, Transformación y Resiliencia (Spain) and Next Generation (European Union).
The authors would like to thank all those who have contributed to the collection of data on vectorial presence and infected animals in the study area.
The authors declare that the research was conducted in the absence of any commercial or financial relationships that could be construed as a potential conflict of interest.
All claims expressed in this article are solely those of the authors and do not necessarily represent those of their affiliated organizations, or those of the publisher, the editors and the reviewers. Any product that may be evaluated in this article, or claim that may be made by its manufacturer, is not guaranteed or endorsed by the publisher.
1. Allen, T, Murray, KA, Zambrana-Torrelio, C, Morse, SS, Rondinini, C, Di Marco, M, et al. Global hotspots and correlates of emerging zoonotic diseases. Nat Commun. (2017) 8:1124. doi: 10.1038/s41467-017-00923-8
2. Cunningham, AA, Daszak, P, and Wood, JLN. One health, emerging infectious diseases and wildlife: two decades of progress? Philos Trans R Soc Lond Ser B Biol Sci. (2017) 372:20160167. doi: 10.1098/rstb.2016.0167
3. Plowright, RK, Parrish, CR, McCallum, H, Hudson, PJ, Ko, AI, Graham, AL, et al. Pathways to zoonotic spillover. Nat Rev Microbiol. (2017) 15:502–10. doi: 10.1038/nrmicro.2017.45
4. Organización Panamericana de la Salud. Día Mundial de las Zoonosis: proteger la salud animal ayuda a preservar la salud humana. (2023). Available at: https://www.paho.org/es/noticias/6-7-2023-dia-mundial-zoonosis-proteger-salud-animal-ayuda-preservar-salud-humana (Accessed February 13, 2024)
5. Rogers, DJ, and Randolph, SE. Climate change and vector-borne diseases. Adv Parasitol. (2006) 62:345–81. doi: 10.1016/S0065-308X(05)62010-6
6. Beugnet, F, and Lou, MJ. Emerging arthropod-borne diseases of companion animals in Europe. Vet Parasitol. (2009) 163:298–305. doi: 10.1016/J.VETPAR.2009.03.028
7. Medlock, JM, Hansford, KM, Versteirt, V, Cull, B, Kampen, H, Fontenille, D, et al. An entomological review of invasive mosquitoes in Europe. Bull Entomol Res. (2015) 105:637–63. doi: 10.1017/S0007485315000103
8. Shaheen, MNF. The concept of one health applied to the problem of zoonotic diseases. Rev Med Virol. (2022) 32:e2326. doi: 10.1002/rmv.2326
9. Bravo-Barriga, D, Ruiz-Arrondo, I, Peña, RE, Lucientes, J, and Delacour-Estrella, S. Phlebotomine sand flies (Diptera, Psychodidae) from Spain: an updated checklist and extended distributions. Zookeys. (2022) 1106:81–99. doi: 10.3897/zookeys.1106.81432
10. González, MA, Ruiz-Arrondo, I, Gutiérrez-López, R, Barceló, C, and Miranda, M. First record of Phlebotomus (Larroussius) perfiliewi (Diptera: Psychodidae), vector of Leishmania infantum and Phleboviruses, in Spain. Diversity. (2023) 15:400. doi: 10.3390/d15030400
11. Solano-Parada, J, Cornet-Gómez, A, Gómez-Samblás, M, and de Pablos-Torró, LM. Leishmanionis In: R Morchón, editor. Biología y Diagnóstico de Enfermedades Parasitarias Humanas Relevantes en España : Ediciones Universidad de Salamanca (2024). 51–62.
12. Rioux, JA, Aboulker, JP, Lanotte, G, Killick-Kendrick, R, and Martini-Dumas, A. Écologie des leishmanioses dans le sud de la France. Ann Parasitol Hum Comp. (1985) 60:221–9. doi: 10.1051/parasite/1985603221
13. Almeida, M, Maia, C, Cristóvão, JM, Morgado, C, Barbosa, I, Ibars, RF, et al. Seroprevalence and risk factors associated with Leishmania infection in dogs from Portugal. Microorganisms. (2022) 10:2262. doi: 10.3390/microorganisms10112262
14. Risueño, J, Ortuño, M, Pérez-Cutillas, P, Goyena, E, Maia, C, Cortes, S, et al. Epidemiological and genetic studies suggest a common Leishmania infantum transmission cycle in wildlife, dogs and humans associated to vector abundance in Southeast Spain. Vet Parasitol. (2018) 259:61–7. doi: 10.1016/J.VETPAR.2018.05.012
15. Gálvez, R, Montoya, A, Cruz, I, Fernández, C, Martín, O, Checa, R, et al. Latest trends in Leishmania infantum infection in dogs in Spain, part I: mapped seroprevalence and sand fly distributions. Parasit Vectors. (2020) 13:204. doi: 10.1186/s13071-020-04081-7
16. Montoya-Alonso, JA, Morchón, R, Costa-Rodríguez, N, Matos, JI, Falcón-Cordón, Y, and Carretón, E. Current distribution of selected vector-borne diseases in dogs in Spain. Front Vet Sci. (2020) 7:564429. doi: 10.3389/fvets.2020.564429
17. Wilson, ME. The traveller and emerging infections: sentinel, courier, transmitter. J Appl Microbiol. (2003) 94:1–11. doi: 10.1046/j.1365-2672.94.s1.1.x
18. Johnson, EE, Escobar, LE, and Zambrana-Torrelio, C. An ecological framework for modeling the geography of disease transmission. Trends Ecol Evol. (2019) 34:655–68. doi: 10.1016/j.tree.2019.03.004
19. Escobar, LE. Ecological niche modeling: an introduction for veterinarians and epidemiologists. Front Vet Sci. (2020) 7:519059. doi: 10.3389/fvets.2020.519059
20. Hill, DE, Dubey, JP, Baroch, JA, Swafford, SR, Fournet, VF, Hawkins-Cooper, D, et al. Surveillance of feral swine for Trichinella spp. and toxoplasma gondii in the USA and host-related factors associated with infection. Vet Parasitol. (2014) 205:653–65. doi: 10.1016/j.vetpar.2014.07.026
21. Di, X, Li, S, Ma, B, Di, X, Li, Y, An, B, et al. How climate, landscape, and economic changes increase the exposure of Echinococcus Spp. BMC Public Health. (2022) 22:2315. doi: 10.1186/s12889-022-14803-4
22. Hanafi-Bojd, AA, Vatandoost, H, and Yaghoobi-Ershadi, MR. Climate change and the risk of malaria transmission in Iran. J Med Entomol. (2020) 57:50–64. doi: 10.1093/jme/tjz131
23. Omar, K, Thabet, HS, TagEldin, RA, Asadu, CC, Chukwuekezie, OC, Ochu, JC, et al. Ecological niche modeling for predicting the potential geographical distribution of Aedes species (Diptera: Culicidae): a case study of Enugu state, Nigeria. Parasite Epidemiol Control. (2021) 15:e00225. doi: 10.1016/j.parepi.2021.e00225
24. Signorini, M, Cassini, R, Drigo, M, Frangipane di Regalbono, A, Pietrobelli, M, Montarsi, F, et al. Ecological niche model of Phlebotomus perniciosus, the main vector of canine leishmaniasis in North-Eastern Italy. Geospat Health. (2014) 9:193–201. doi: 10.4081/gh.2014.16
25. Giannakopoulos, A, Tsokana, CN, Pervanidou, D, Papadopoulos, E, Papaspyropoulos, K, Spyrou, V, et al. Environmental parameters as risk factors for human and canine Leishmania infection in Thessaly, Central Greece. Parasitology. (2016) 143:1179–86. doi: 10.1017/S0031182016000378
26. Koch, LK, Kochmann, J, Klimpel, S, and Cunze, S. Modeling the climatic suitability of leishmaniasis vector species in Europe. Sci Rep. (2017) 7:13325. doi: 10.1038/s41598-017-13822-1
27. Sofizadeh, A, Rassi, Y, Vatandoost, H, Hanafi-Bojd, AA, Mollalo, A, Rafizadeh, S, et al. Predicting the distribution of Phlebotomus papatasi (Diptera: Psychodidae), the primary vector of zoonotic cutaneous Leishmaniasis, in Golestan Province of Iran using ecological niche modeling: comparison of MaxEnt and GARP models. J Med Entomol. (2016) 54:312–20. doi: 10.1093/jme/tjw178
28. Chalghaf, B, Chemkhi, J, Mayala, B, Harrabi, M, Benie, GB, Michael, E, et al. Ecological niche modeling predicting the potential distribution of Leishmania vectors in the Mediterranean basin: impact of climate change. Parasit Vectors. (2018) 11:461. doi: 10.1186/s13071-018-3019-x
29. Chavy, A, Ferreira Dales Nava, A, Luz, SLB, Ramírez, JD, Herrera, G, Vasconcelos dos Santos, T, et al. Ecological niche modelling for predicting the risk of cutaneous leishmaniasis in the Neotropical moist forest biome. PLoS Negl Trop Dis. (2019) 13:e0007629. doi: 10.1371/journal.pntd.0007629
30. Cunze, S, Kochmann, J, Koch, LK, Hasselmann, KJQ, and Klimpel, S. Leishmaniasis in Eurasia and Africa: geographical distribution of vector species and pathogens. R Soc Open Sci. (2019) 6:190334. doi: 10.1098/rsos.190334
31. Kuhls, K, Moskalenko, O, Sukiasyan, A, Manukyan, D, Melik-Andreasyan, G, Atshemyan, L, et al. Microsatellite based molecular epidemiology of Leishmania infantum from re-emerging foci of visceral leishmaniasis in Armenia and pilot risk assessment by ecological niche modeling. PLoS Negl Trop Dis. (2021) 15:e0009288. doi: 10.1371/journal.pntd.0009288
32. Charrahy, Z, Yaghoobi-Ershadi, MR, Shirzadi, MR, Akhavan, AA, Rassi, Y, Hosseini, SZ, et al. Climate change and its effect on the vulnerability to zoonotic cutaneous leishmaniasis in Iran. Transbound Emerg Dis. (2022) 69:1506–20. doi: 10.1111/tbed.14115
33. Gálvez, R, Descalzo, MA, Guerrero, I, Miró, G, and Molina, R. Mapping the current distribution and predicted spread of the leishmaniosis sand fly vector in the Madrid region (Spain) based on environmental variables and expected climate change. Vector Borne Zoonotic Dis. (2011) 11:799–806. doi: 10.1089/vbz.2010.0109
34. Pires, H, Martins, M, Matos, AC, Cardoso, L, Monteiro, F, Roque, N, et al. Geospatial analysis applied to seroepidemiological survey of canine leishmaniosis in east-Central Portugal. Vet Parasitol. (2019) 274:108930. doi: 10.1016/j.vetpar.2019.108930
35. Rodríguez-Escolar, I, Hernández-Lambraño, RE, Sánchez-Agudo, JÁ, Collado, M, Pérez-Pérez, P, and Morchón, R. Current risk of Dirofilariosis transmission in the Iberian Peninsula (Spain and Portugal) and the Balearic Islands (Spain) and its future projection under climate change scenarios. Animals. (2023) 13:1764. doi: 10.3390/ani13111764
36. Morchón, R, Rodríguez-Escolar, I, Lambraño, REH, Agudo, JÁS, Montoya-Alonso, JA, Serafín-Pérez, I, et al. Assessment heartworm disease in the Canary Islands (Spain): risk of transmission in a Hyperendemic area by ecological niche modeling and its future projection. Animals. (2023) 13:3251. doi: 10.3390/ani13203251
37. Genchi, M, Escolar, IR, García, RM, Semeraro, M, Kramer, LH, Colombo, L, et al. Dirofilaria immitis in Italian cats and the risk of exposure by Aedes albopictus. Vector Borne Zoonotic Dis. (2024) 24:151–8. doi: 10.1089/vbz.2023.0097
38. Beck, HE, Zimmermann, NE, McVicar, TR, Vergopolan, N, Berg, A, and Wood, EF. Present and future köppen-Geiger climate classification maps at 1-km resolution. Sci Data. (2018) 5:180214. doi: 10.1038/sdata.2018.214
39. Maia, C, Cristóvão, J, Pereira, A, Kostalova, T, Lestinova, T, Sumova, P, et al. Monitoring Leishmania infection and exposure to Phlebotomus perniciosus using minimal and non-invasive canine samples. Parasit Vectors. (2020) 13:119. doi: 10.1186/s13071-020-3993-7
40. Martín-Sánchez, J, Rodríguez-Granger, J, Morillas-Márquez, F, Merino-Espinosa, G, Sampedro, A, Aliaga, L, et al. Leishmaniasis due to Leishmania infantum: integration of human, animal and environmental data through a one health approach. Transbound Emerg Dis. (2020) 67:2423–34. doi: 10.1111/tbed.13580
41. Díaz-Sáez, V, Corpas-López, V, Merino-Espinosa, G, Morillas-Mancilla, MJ, Abattouy, N, and Martín-Sánchez, J. Seasonal dynamics of phlebotomine sand flies and autochthonous transmission of Leishmania infantum in high-altitude ecosystems in southern Spain. Acta Trop. (2021) 213:105749. doi: 10.1016/j.actatropica.2020.105749
42. Branco, S, Alves-Pires, C, Maia, C, Cortes, S, Cristovão, JMS, Gonçalves, L, et al. Entomological and ecological studies in a new potential zoonotic leishmaniasis focus in Torres Novas municipality, central region, Portugal. Acta Trop. (2013) 125:339–48. doi: 10.1016/j.actatropica.2012.12.008
43. Maia, C, Dionisio, L, Afonso, MO, Neto, L, Cristovao, JM, and Campino, L. Leishmania infection and host-blood feeding preferences of phlebotomine sandflies and canine leishmaniasis in an endemic European area, the Algarve region in Portugal. Mem Inst Oswaldo Cruz. (2013) 108:481–7. doi: 10.1590/S0074-0276108042013014
44. Amaro, F, Zé-Zé, L, Alves, MJ, Börstler, J, Clos, J, Lorenzen, S, et al. Co-circulation of a novel phlebovirus and Massilia virus in sandflies, Portugal. Virol J. (2015) 12:174. doi: 10.1186/s12985-015-0407-0
45. GBIF. (2024). Available at: https://www.gbif.org/species/5981223 (Accessed January 12, 2024).
46. WorldClim. (2022). Bioclimatic variables. Available at: https://www.worldclim.org/data/bioclim.html (Accessed February 12, 2024).
47. Fick, SE, and Hijmans, RJ. WorldClim 2: new 1-km spatial resolution climate surfaces for global land areas. Int J Climatol. (2017) 37:4302–15. doi: 10.1002/joc.5086
48. R Core Team. R: a language and environment for statistical computing. Vienna, Austria: R Foundation for Statistical Computing (2021).
49. EarthEnv. Available at: https://www.earthenv.org/ (Accessed February 14, 2024)
50. Socioeconomic Data and Applications Center. (2024). Available at: https://sedac.ciesin.columbia.edu/ (Accessed February 14, 2024).
51. American Museum of Natural History. (2024). Available at: https://biodiversityinformatics.amnh.org/open_source/maxent/ (Accessed February 15, 2024).
52. Cobos, ME, Peterson, AT, Barve, N, and Osorio-Olvera, L. Kuenm: an R package for detailed development of ecological niche models using Maxent. PeerJ. (2019) 7:e6281. doi: 10.7717/peerj.6281
53. Phillips, SJ, Anderson, RP, and Schapire, RE. Maximum entropy modeling of species geographic distributions. Ecol Model. (2006) 190:231–59. doi: 10.1016/j.ecolmodel.2005.03.026
54. Kuhlbrodt, T, Jones, CG, Sellar, A, Storkey, D, Blockley, E, Stringer, M, et al. The low-resolution version of HadGEM3 GC3.1: development and evaluation for global climate. J Adv Model Earth Syst. (2018) 10:2865–88. doi: 10.1029/2018MS001370
55. Andrews, MB, Ridley, JK, Wood, RA, Andrews, T, Blockley, EW, Booth, B, et al. Historical simulations with HadGEM3-GC3.1 for CMIP6. J Adv Model Earth Syst. (2020) 12:e2019MS001995. doi: 10.1029/2019MS001995
56. Thuiller, W, Lafourcade, B, Engler, R, and Araújo, MB. BIOMOD - a platform for ensemble forecasting of species distributions. Ecography. (2009) 32:369–73. doi: 10.1111/j.1600-0587.2008.05742.x
57. García, N, Moreno, I, Alvarez, J, de la Cruz, ML, Navarro, A, Pérez-Sancho, M, et al. Evidence of Leishmania infantum infection in rabbits (Oryctolagus cuniculus) in a natural area in Madrid, Spain. Biomed Res Int. (2014) 2014:1–5. doi: 10.1155/2014/318254
58. Ntais, P, Sifaki-Pistola, D, Christodoulou, V, Messaritakis, I, Pratlong, F, Poupalos, G, et al. Leishmaniases in Greece. Am J Trop Med Hyg. (2013) 89:906–15. doi: 10.4269/ajtmh.13-0070
59. González, E, Álvarez, A, Ruiz, S, Molina, R, and Jiménez, M. Detection of high Leishmania infantum loads in Phlebotomus perniciosus captured in the leishmaniasis focus of southwestern Madrid region (Spain) by real time PCR. Acta Trop. (2017) 171:68–73. doi: 10.1016/J.ACTATROPICA.2017.03.023
60. Galán-Puchades, MT, Gómez-Samblás, M, Suárez-Morán, JM, Osuna, A, Sanxis-Furió, J, Pascual, J, et al. Leishmaniasis in Norway rats in sewers, Barcelona, Spain. Emerg Infect Dis. (2019) 25:1222–4. doi: 10.3201/eid2506.181027
61. Alcover, MM, Basurco, A, Fernandez, A, Riera, C, Fisa, R, Gonzalez, A, et al. A cross-sectional study of Leishmania infantum infection in stray cats in the city of Zaragoza (Spain) using serology and PCR. Parasit Vectors. (2021) 14:178. doi: 10.1186/s13071-021-04682-w
62. González, E, Molina, R, Iriso, A, Ruiz, S, Aldea, I, Tello, A, et al. Opportunistic feeding behaviour and Leishmania infantum detection in Phlebotomus perniciosus females collected in the human leishmaniasis focus of Madrid, Spain (2012–2018). PLoS Negl Trop Dis. (2021) 15:e0009240. doi: 10.1371/journal.pntd.0009240
63. Galán-Puchades, MT, Solano, J, González, G, Osuna, A, Pascual, J, Bueno-Marí, R, et al. Molecular detection of Leishmania infantum in rats and sand flies in the urban sewers of Barcelona, Spain. Parasit Vectors. (2022) 15:211. doi: 10.1186/s13071-022-05309-4
64. Chamaillé, L, Tran, A, Meunier, A, Bourdoiseau, G, Ready, P, and Dedet, J-P. Environmental risk mapping of canine leishmaniasis in France. Parasit Vectors. (2010) 3:31. doi: 10.1186/1756-3305-3-31
65. Ballart, C, Guerrero, I, Castells, X, Barón, S, Castillejo, S, Alcover, MM, et al. Importance of individual analysis of environmental and climatic factors affecting the density of Leishmania vectors living in the same geographical area: the example of Phlebotomus ariasi and P. perniciosus in Northeast Spain. Geospat Health. (2014) 8:389–403. doi: 10.4081/gh.2014.28
66. Quintana, M, Salomón, O, Guerra, R, Lizarralde de Grosso, M, and Fuenzalida, A. Phlebotominae of epidemiological importance in cutaneous leishmaniasis in northwestern Argentina: risk maps and ecological niche models. Med Vet Entomol. (2013) 27:39–48. doi: 10.1111/j.1365-2915.2012.01033.x
67. Estrela-Segrelles, C, Gómez-Martinez, G, and Pérez-Martín, MÁ. Risk assessment of climate change impacts on Mediterranean coastal wetlands. Application in Júcar River Basin District (Spain). Sci Total Environ. (2021) 790:148032. doi: 10.1016/j.scitotenv.2021.148032
68. García-del-Amo, D, Mortyn, PG, and Reyes-García, V. Local reports of climate change impacts in Sierra Nevada, Spain: sociodemographic and geographical patterns. Reg Environ Chang. (2023) 23:14. doi: 10.1007/s10113-022-01981-5
69. Kartashev, V, Afonin, A, González-Miguel, J, Sepúlveda, R, Simón, L, Morchón, R, et al. Regional warming and emerging vector-borne zoonotic Dirofilariosis in the Russian Federation, Ukraine, and other post-soviet states from 1981 to 2011 and projection by 2030. Biomed Res Int. (2014) 2014:1–11. doi: 10.1155/2014/858936
70. Hongoh, V, Berrang-Ford, L, Scott, ME, and Lindsay, LR. Expanding geographical distribution of the mosquito, Culex pipiens, in Canada under climate change. Appl Geogr. (2012) 33:53–62. doi: 10.1016/j.apgeog.2011.05.015
71. Githeko, AK, Lindsay, SW, Confalonieri, UE, and Patz, JA. Climate change and vector-borne diseases: a regional analysis. Bull World Health Organ. (2000) 78:1136–47.
Keywords: Leishmania infantum, leishmaniosis, Phlebotomus perniciosus, Spain, Portugal, dogs, ecological niche model, infection risk
Citation: Rodríguez-Escolar I, Balmori-de la Puente A, Collado-Cuadrado M, Bravo-Barriga D, Delacour-Estrella S, Hernández-Lambraño RE, Sánchez Agudo JÁ and Morchón R (2024) Analysis of the current risk of Leishmania infantum transmission for domestic dogs in Spain and Portugal and its future projection in climate change scenarios. Front. Vet. Sci. 11:1399772. doi: 10.3389/fvets.2024.1399772
Received: 12 March 2024; Accepted: 22 April 2024;
Published: 02 May 2024.
Edited by:
Emmanuel Serrano Ferron, Autonomous University of Barcelona, SpainReviewed by:
Eduardo Berriatua, University of Murcia, SpainCopyright © 2024 Rodríguez-Escolar, Balmori-de la Puente, Collado-Cuadrado, Bravo-Barriga, Delacour-Estrella, Hernández-Lambraño, Sánchez Agudo and Morchón. This is an open-access article distributed under the terms of the Creative Commons Attribution License (CC BY). The use, distribution or reproduction in other forums is permitted, provided the original author(s) and the copyright owner(s) are credited and that the original publication in this journal is cited, in accordance with accepted academic practice. No use, distribution or reproduction is permitted which does not comply with these terms.
*Correspondence: Alfonso Balmori-de la Puente, YS5iYWxtb3JpQGliZS51cGYtY3NpYy5lcw==; Rodrigo Morchón, cm1vcmdhckB1c2FsLmVz
Disclaimer: All claims expressed in this article are solely those of the authors and do not necessarily represent those of their affiliated organizations, or those of the publisher, the editors and the reviewers. Any product that may be evaluated in this article or claim that may be made by its manufacturer is not guaranteed or endorsed by the publisher.
Research integrity at Frontiers
Learn more about the work of our research integrity team to safeguard the quality of each article we publish.