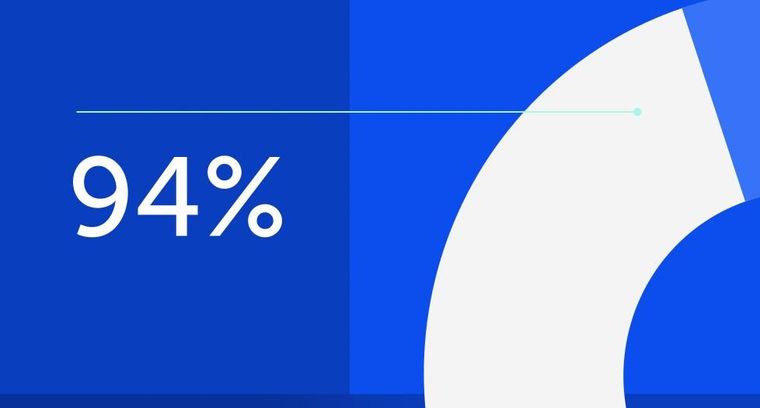
94% of researchers rate our articles as excellent or good
Learn more about the work of our research integrity team to safeguard the quality of each article we publish.
Find out more
ORIGINAL RESEARCH article
Front. Vet. Sci., 11 January 2024
Sec. Veterinary Epidemiology and Economics
Volume 10 - 2023 | https://doi.org/10.3389/fvets.2023.1301392
Aggregated diagnostic data collected over time from swine production systems is an important data source to investigate swine productivity and health, especially when combined with records concerning the pre-weaning and post-weaning phases of production. The combination of multiple data streams collected over the lifetime of the pigs is the essence of the whole-herd epidemiological investigation. This approach is particularly valuable for investigating the multifaceted and ever-changing factors contributing to wean-to-finish (W2F) swine mortality. The objective of this study was to use a retrospective dataset (“master table”) containing information on 1,742 groups of pigs marketed over time to identify the major risk factors associated with W2F mortality. The master table was built by combining historical breed-to-market performance and health data with disease diagnostic records (Dx Codes) from marketed groups of growing pigs. After building the master table, univariate analyses were conducted to screen for risk factors to be included in the initial multivariable model. After a stepwise backward model selection approach, 5 variables and 2 interactions remained in the final model. Notably, the diagnosis variable significantly associated with W2F mortality was porcine reproductive and respiratory syndrome virus (PRRSV). Closeouts with clinical signs suggestive of Salmonella spp. or Escherichia coli infection were also associated with higher W2F mortality. Source sow farm factors that remained significantly associated with W2F mortality were the sow farm PRRS status, average weaning age, and the average pre-weaning mortality. After testing for the possible interactions in the final model, two interactions were significantly associated with wean-to-finish pig mortality: (1) sow farm PRRS status and a laboratory diagnosis of PRRSV and (2) average weaning age and a laboratory diagnosis of PRRS. Closeouts originating from PRRS epidemic or PRRS negative sow farms, when diagnosed with PRRS in the growing phase, had the highest W2F mortality rates. Likewise, PRRS diagnosis in the growing phase was an important factor in mortality, regardless of the average weaning age of the closeouts. Overall, this study demonstrated the utility of a whole-herd approach when analyzing diagnostic information along with breeding-to-market productivity and health information, to measure the major risk factors associated with W2F mortality in specified time frames and pig populations.
The increasing implementation of information technologies in the swine industry has resulted in an abundance of diverse, but usually disconnected, data streams recording most of the aspects of raising pigs, e.g., productivity, health, environment, diagnostic, logistics, and infrastructure. If the data streams are connected, producers have the potential to measure the effect of specific risk factors on performance under the specific production system’s conditions and, thereafter, tailor swine health and production management to specific conditions and swine populations/pig flows.
Swine wean-to-finish (W2F) mortality is a key performance indicator (KPI) in modern swine production, and it is the result of interactions among multiple infectious and non-infectious agents (1, 2) which involve the epidemiological triad of disease, i.e., a dynamic interaction between pathogen, host, and environmental characteristics occurring sequentially from birth-to-market (~6 months) that are constantly changing over time. Although most swine producers collect information relevant to these factors, the data is usually being stored in multiple formats and scattered across different software or files (3).
An example of a data stream available to many swine production systems is diagnostic information from veterinary diagnostic laboratories (VDL’s). Veterinarians and producers collect and submit samples to VDL’s to diagnose cause(s) of disease, to monitor health, and/or respond to immediate disease threats. Diagnostic result is generally utilized immediately after the final diagnostic by swine veterinarians for utility in intervention decisions, and then stored in data files without further application. In fact, the aggregate retrospective diagnostic results collected over the lifetime of multiple closeouts (cohorts) represents a potentially important data source as demonstrated in other studies (4–7).
In this study, productivity, health, and diagnostic laboratory data were integrated to analyze the association between growing pig performance and wean-to-finish mortality (W2F). Thus, the objective of this study was to characterize associations between disease and mortality in groups of growing pigs, along with relevant health and performance observations from birth-to-market.
A retrospective study was conducted on wean-to-finish (W2F) mortality for 1,742 “closeouts.” A “closeout” was defined as a group of pigs marketed within one United States commercial swine production system between January 2018 and June 2019. A closeout may have originated from either wean-to-finish sites or nursery followed by finisher sites. The outcome variable of interest was the number of pigs in each closeout that died in the growing phase as a proportion of those placed on feed. Closeout data available for the analysis are described in Table 1 and included 18 variables concerning the breed-to-market phase of production, i.e., disease status (e.g., PRRSV) and production performance data from the breeding herd(s) of origin, placement history (single-stocked or double-stocked groups; wean-to-finish flow or nursery followed by finisher), post-weaning productivity & health, and diagnostic data for each diagnostic case that included tissues and originated from the closeouts in this study. The columns of each variable included on Table 1 represents the categories within each variable.
Diagnostic evaluations were performed at the Iowa State University Veterinary Diagnostic Laboratory (ISU VDL, Ames, Iowa). Diagnostic data from closeouts’ cases with samples submitted for diagnosis at the ISU VDL included clinical information, laboratory assays performed, evaluation of macroscopic and/or microscopic lesions, and the diagnostic code (Dx Code) ultimately assigned to each tissue case by the veterinary diagnostician. Diagnostic data from non-tissue submissions were not included in this study.
The ISU VDL disease diagnostic coding system (Dx Code) is used to succinctly summarize pathologic process(s) and etiology(s) found in submissions of tissues from diseased pigs, used to describe diagnostic alignment between pathogens detected and lesions observed in tissues submitted for diagnostic evaluation. These codes are organized in terms of body system affected/insult type/predominant lesions(s) detected/confirmed or highly likely specific agent(s), and provide a precise etiological diagnosis when data aligns (8). One or more DX codes are assigned per case depending on these evaluations. For example, a case containing a lung tissue submitted for evaluation with a clinical background of respiratory disease, macroscopic and/or microscopic lesions compatible with Mycoplasma hyopneumoniae (Mhp) infection, and a PCR positive result for Mhp would have a Dx Code of respiratory/bacterial/ pneumonia/Mycoplasma hyopneumoniae. Notably, despite the tests utilized for each case being expected to be different, the final Dx Code is provided in a standardized format for all cases. All Dx Codes assigned to a closeout were included in the final master table, as well as closeouts that had two or more Dx Codes from multiple case submissions over the growing period or from one submission with multiple pathogens, as described in Table 1 as Dx Code frequency and Dx Code diversity, respectively.
Breeding herds were classified as PRRSV epidemic for the first 16 weeks after a PRRSV outbreak and then as “endemic” from week 17 until they were classified as “negative” based on the absence of wild-type PRRSV RNA detection and absence of clinical signs consistent with PRRSV infections. Notably, PRRSV negative farms are not considered naïve as the presence of PRRSV antibodies in the sows is expected. The major difference compared to the epidemic and endemic status is the absence of clinical signs combined with negative results for PRRSV. All herds were vaccinated with commercial modified live virus (MLV) PRRSV vaccine and detection of vaccine-like PRRSV did not disqualify negative herds. Average pre-weaning mortality and average weaning age were the remaining variables related to the breeding herd included in this study and described the average performance of the cohort of weaned pigs moved to the growing phase. When multiple weaned groups (i.e., from multiple sow farms) are placed together in growing sites, the weighted average was calculated for the variables mentioned above based on each farm performance and the number of animals from each origin. These variables were categorized by quartiles and the mean of the variables in the continuous format was reported in Table 1.
SAS® Version 9.4 (SAS Institute, Inc., Cary, NC) was utilized to build algorithms used to import, manage, and integrate the production data and diagnostic data for 1,742 closeouts from a commercial swine production system, as fully described by (9). Data cleaning was performed after aggregating the data streams into the master table, i.e., closeouts with missing data were excluded from the final dataset. This process produced a single master table containing 1,720 closeouts (~5,000,000 pigs) marketed from January 2018 to June 2019, which was utilized on the analyzes of the multivariable model.
The final master table contained retrospective data from 1,720 closeouts and 18 variables representing diagnostic data, sow farm factors, and growing phase characteristics (Tables 1, 2). Initially, to meet the assumption of normality the outcome was log-transformed, and the assumption was tested using the Shapiro–Wilk test. Thereafter, the 18 variables were evaluated separately for their association with W2F mortality using a univariate linear mixed model (SAS PROC GLIMMIX) with the sow farm(s) from which the pigs in the closeout originated as a random effect. While informative, univariate analyses have well-recognized limitations, e.g., confounding, that can be addressed using multivariable models.
The initial multivariable model included all variables with a moderate association to W2F mortality (p < 0.10, univariate analysis). To achieve the final multivariable model, manual stepwise backward model selection was used to identify variables significantly associated with W2F mortality (p < 0.05) as demonstrated in Table 2. Thereafter, multicollinearity in the final model was assessed, and variables with a variance inflation factor (VIF) value >5 were excluded. Once the final multivariable model was built, Tukey–Kramer pairwise comparisons were done to identify significant differences between levels of categorical variables.
The geometric mean log-W2F mortality for the 1,720 closeouts in the study was 8.55%, (95% CI 8.37, 8.73%). A total of 392 closeouts had >1 Dx Code(s), while 1,328 groups had no tissue submissions and, therefore, no Dx Codes. The results of the univariate analyses on Dx Code variables are presented in Table 3. “No Dx Code,” i.e., the category representing closeouts with no tissue submissions to the diagnostic laboratory, served as the baseline of comparison and denoted groups of pigs with absence of clinical signs to trigger diagnostic investigation.
In summary, the univariate analysis revealed a notable disparity in W2F mortality (10.1% vs. 7.9%) between closeouts with more than one Dx Code and those without any Dx Code (refer to Table 3). When considering submission age, the average W2F closeout mortality rates were 10.6, 10.2, and 9.4%, respectively, for cohorts with Dx Codes in early nursery, late nursery, and finisher stages. Additionally, in the comparison between closeouts with a single submission and those with more than two submissions, the mean W2F mortality rates were 10.0 and 11.1%, respectively. Similarly, when evaluating “single pathogen” versus “multiple pathogens,” the mean W2F mortality rates were 9.8 and 10.6%, respectively.
From the univariate analyses, 17 of 18 variables were included in the initial multivariable model. Manual stepwise model selection resulted in the exclusion of 12 variables that did not meet the eligibility criteria (p < 0.05) and one variable due to multicollinearity (VIF > 5), as demonstrated in Table 2. The final multivariable model included PRRS Dx Code, sow farm PRRSV status, average weaning age, average pre-weaning mortality, and enteric disease (Table 4), and the final VIF values are available in Supplementary Table S1.
As shown in Table 4, groups with PRRS Dx Codes, groups diagnosed with other pathogens (not PRRS), and groups with No Dx Code had W2F mortalities of 13.5, 10.3 and 9.0%, respectively. The final multivariable analysis demonstrated that “Dx Code - PRRS” was the only significant diagnosis, i.e., the pathogens identified as statistically significant in the univariate model were confounded by PRRSV. To verify this, interactions between Dx Code - PRRS and each of the other pathogen-specific Dx Codes were tested separately in the final multivariable model, but none were significant. Also, the W2F mortality values for PRRS Dx Code categories changed when comparing the univariate and the multivariable results, which is a consequence of adjusting the estimates according to the remaining covariates included in the final multivariable model.
Sow farm PRRSV status at the time the cohorts were weaned was identified as a significant factor through the model selection process. Weaned groups originating from sow farms with epidemic PRRSV status subsequently had higher downstream W2F mortality (11.8%) versus cohorts from PRRSV endemic (10.5%) or negative sow farms (10.1%).
Average pre-weaning mortality on the sow farm was also significant, i.e., the observed W2F mortality for groups weaned with pre-weaning mortality quartiles of 10.7, 13.1, 14.8, and 18.0%, were 10.4, 10.2, 10.6, and 12.0%, respectively. In other words, closeouts that originated from sow farms where the average pre-weaning mortality was high were associated with higher downstream W2F mortality as well. Weaning age was likewise statistically associated with the downstream W2F mortality. As weaning age quartiles increased (15.3, 16.8, 17.9, and 20.3 days of age at weaning), W2F mortality decreased (10.9, 11.7, 10.4, and 10.1%).
A growing phase variable that remained significant in the multivariable model was the determination of enteric disease in the growing phase by the herd veterinarian. Specifically, the mean W2F mortality for groups without clinically apparent enteric disease was 9.4%, while mortality in groups with clinical disease typical of E. coli, Salmonella, or both E. coli and Salmonella was 11.1, 10.4, and 12.7%, respectively.
The two significant interactions included in the final multivariable model were (PRRS Dx Code*sow farm PRRSV status), and (Dx Code - PRRSV*average weaning age), as shown in Figures 1, 2, respectively.
Figure 1. Interaction between Sow farm PRRSV status and PRRSV diagnosis Dx Code data. abcDifferent superscript letters indicate significant differences (Tukey test, p < 0.05). PRRS, porcine reproductive and respiratory syndrome; W2F, wean-to-finish.
Figure 2. Interaction between PRRSV Dx Code data and average weaning age of the closeouts. abcDifferent superscript letters indicate significant differences (Tukey test, p < 0.05). PRRS, porcine reproductive and respiratory syndrome; W2F, wean-to-finish.
The relationship between sow farm PRRSV status, PRRS Dx Code, and W2F mortality was complex (Figure 1). Overall, the diagnosis of PRRSV during the growing period resulted in both numeric and statistically significant increases in mortality compared to groups with “Dx Code - None” or “Dx Code - Other (not PRRS).” The highest mortalities were observed in closeouts with a PRRS diagnosis (Dx Code – PRRS) weaned from PRRSV epidemic and negative sow farms. Further, diagnosing a pathogen other than PRRSV resulted in a numeric (but not a significant) increase in mortality compared to closeouts with no Dx Codes across all sow farm PRRS status categories.
The analysis of W2F mortality by weaning age showed a similar trend (Figure 2). That is, closeouts classified as “Dx Code – None” had the lowest mortality followed closely by closeouts classified as “Dx Code other - (not PRRS).” Thus, the highest W2F mortality was observed in “Dx Code – PRRS” closeouts. Declining mortality with increased weaning age was observed for the “Dx Code – None” and “Dx Code - other (not PRRS)” categories. For both categories, the lowest W2F mortality was observed in groups weaned in the oldest weaning age quartile (20.3 days). On the other hand, the highest mortality occurred in the youngest weaning age quartile (15.3 days). Thus, in the absence of a PRRS diagnosis in the growing phase, weaning age was an important risk factor for W2F mortality. Conversely, PRRS diagnosis in the growing phase negates the weaning age effect and results in higher mortality across all weaning ages compared to groups without PRRS diagnosis.
Writing at the time when the swine industry began to transition from many herds with relatively few animals on each premise to fewer farms with larger populations, (10) described the need to move from single-agent causality to multifactorial causes. Today, identifying and addressing the multiple factors that drive pig mortality is the basis of effective disease control and prevention, as well as a key to the economic viability of swine enterprises. To achieve this requires taking a holistic or whole-herd approach when investigating swine health issues. For the wean-to-finish phase of production, this approach is increasingly feasible because of the availability of performance and health data collected routinely over the course of the pigs’ production cycle (pre and post-weaning phase), as has been previously described in other observational studies (9, 11–18). In this study, the whole-herd approach was achievable because the data streams included premise identification and dates for each recorded event. These unique identifiers and the use of SAS algorithms allowed automated data wrangling and the development of a master table containing retrospective information for each closeout marketed in the study period. This study also demonstrated the power of capturing and merging multiple birth-to-market data streams (whole-herd approach) in terms of revealing the impact of “distant” spatiotemporal events, e.g., sow herd health status, on downstream grower pigs. That is, combining diagnostic data with other data streams made it possible to more fully understand health dynamics by connecting earlier events to current disease problems.
Diagnostic data is generally utilized to estimate health status and disease pressure at a point in time, thus guiding producers and veterinarians as they respond to protect the health and productivity of the population (8). This was confirmed in the present study as W2F mortality was higher for groups with diagnostic codes assigned compared to flows without diagnostic codes, indicating that the submission of tissues for diagnosis was associated with evidence of disease expression at the population level, therefore being a proxy of disease activity in growing pigs.
A diagnosis is an assessment based on the pertinent cumulative information, i.e., herd history, geographical location, clinical signs, lesions, and test results, available for each case. Overall, this study demonstrated that the standalone interpretation of Dx Code results, e.g., univariate analyses or pivot tables, should be done cautiously because such estimates are not adjusted for important confounders (19). Thus, various Dx Code explanatory variables were significant in the univariate analyses (Table 3), but a multivariable model that accounted for other covariates and confounders revealed that only one (PRRS Dx Code) was significant for W2F mortality. In particular, in the absence of PRRS, other pathogens were not significantly associated with W2F mortality but their importance for other W2F metrics, e.g., average daily weight gain (ADWG), cannot be ruled out, as demonstrated in previous studies on Mhp (20–22), PEDV (23) and influenza A virus (24, 25).
Among the five variables in the final multivariable model, three concerned productivity and health in the pre-weaning phase of production. This confirmed the importance of breeding herd characteristics on the downstream performance of growing pigs, as previously shown in other studies (26–31). Consistent with the literature, the multivariable model indicated that the impact of sow farm factors on growing pig mortality is complex, with PRRSV infection meriting special consideration both on the sow farm and subsequently in growing pigs (32–40). For example, closeouts originating from PRRS epidemic sow farms, i.e., cohorts weaned within the first 16 weeks after a Sow farm PRRSV outbreak, had the highest downstream mortality rates.
The remaining two variables in the multivariable model represented PRRS infection (PRRS Dx Code) and enteric challenge in the post-weaning phase of production. Closeouts diagnosed with PRRS in the growing phase, i.e., Dx Code – PRRS, had significantly higher W2F mortality compared to closeouts classified as “Dx Code – Other (not PRRS)” or no diagnosis (“Dx Code – None”). Similarly, a previous growing pig study in “pig-dense” areas reported that >90% of the groups detected wild-type PRRSV-2 in ≥1 sampling (37), indicating high virus circulation in growing pigs. Furthermore, groups with reported enteric challenges suggestive of both E. coli and Salmonella had higher W2F mortality than those without enteric challenges. Unfortunately, the precise cause of enteric disease was not always confirmed by diagnostic testing in this study, and thus, the specific contributions of either agent or other causes of enteric disease cannot be determined and warrant further investigation in future analyses. Other investigators have reported similar patterns for PRRS and enteric challenges on W2F mortality (16, 41–45).
An analysis of the interaction between closeouts diagnosed with PRRS in the growing phase (PRRS Dx Code) and the PRRSV status of the sow farm at the cohorts’ time of weaning (Figure 1) highlighted the importance of PRRSV as a risk factor. That is, cohorts with a PRRSV diagnosis had the highest mortality across all categories, particularly groups weaned from sow farms with either a PRRSV negative or PRRSV epidemic status. These results suggested that grower pigs from PRRSV wild-type negative sow farms may have had no prior exposure to wild-type PRRSV and were, therefore, less able to respond immunologically to a wild-type PRRSV infection in the growing phase, even though negative sow farms were all vaccinated for PRRSV, similarly to the PRRS stable Category 2-vx sow farms described by (46). On the other hand, the poor performance of pigs weaned from epidemic sow farms implies that they may have been suboptimal in terms of immunity as a consequence of the clinical manifestation of PRRS in sow farms through the acute phase after the outbreak (47). In contrast to PRRSV epidemic and wild-type negative sow farms, closeouts from PRRSV endemic sow farms had numerically lower mortality, presumably because these pigs had the advantage of maternal immunity against PRRSV, but this assumption can only be confirmed by analyzing potential confounders not included in this study such as the different PRRS virus strains and/or the presence of multiple strains in a farm. A key observation from this analysis was that, even in the absence of a PRRS Dx Code, both the “Dx Code - None” and “Dx Code – Other (not PRRS)” categories had higher W2F mortality when the closeouts originated from PRRSV epidemic sow farms.
Likewise, the interaction between PRRS Dx Code and the average weaning age of the groups (Figure 2) demonstrated similar results, i.e., groups with PRRS “Dx Code – PRRS” had higher W2F mortality values, independent of the weaning age categories. On the other hand, a trend toward numerically higher W2F mortalities was observed for groups with younger average weaning age and in the absence of PRRS diagnosis in the growing phase. For closeouts without any diagnosis throughout the growing phase (Dx Code – None), closeouts weaned from the younger weaning age category (15.3 days) had 1.4, 1.6, and 1.9% higher W2F mortality when compared to the remaining weaning age categories (16.8 days, 17.9 days, and 20.3 days, respectively). Others have also reported lower W2F mortality and better growth performance with increased average weaning age (16, 26, 28, 31, 48).
The limitations of this study primarily involve the diagnostic data. First, the tissue samples submitted to the veterinary diagnostic laboratory for evaluation were selected by individual veterinarians or field staff. Within the production system, it is reasonable to assume variability among veterinarians in the choice and timing of pigs/samples for submission. Furthermore, disease circulation in large populations is rarely homogeneous and also varies over time, thus limiting the external validity of this study to other populations of market pigs. Also, most of the tissues submitted for Dx Code in this study originated from point-in-time sampling collection(s), which lacks continuous diagnostic monitoring based on a standardized protocol for all processes involving the submission of tissues for diagnosis, thus, not being consistently applied across all closeouts. Regardless, integrating disease diagnosis information with other data streams, including the performance of the sow farms at the time growing groups were farrowed and weaned, can assist field veterinarians in identifying data-driven solutions intended to improve herd performance.
This study demonstrated the application of the whole-herd approach in identifying the major risk factors associated with W2F mortality based on the analysis of an integrated master table containing both disease diagnostic information and pre- and post-weaning data related to productivity and health. The differences between univariable and multivariable analyses illustrate that standalone data assessment, i.e., pivot tables or univariate analyses, should be avoided in favor of the whole-herd approach. Further, the multivariable analysis showed that PRRSV infection continues to impact pig health, productivity, and W2F mortality in commercial herds throughout the growing phase and that the sow farm plays a major role in the downstream survivability of growing pigs. Notably, the interactions revealed in the data analyzed in this study are expected to change over time or between production systems, thus, the process requires ongoing data integration and analysis.
The datasets presented in this article are not readily available because the data presented in this study are available on reasonable request from the corresponding author. The data are not publicly available due to privacy. Requests to access the datasets should be directed to ZWRpc29uQGlhc3RhdGUuZWR1.
EM: Conceptualization, Data curation, Formal analysis, Methodology, Writing – original draft, Writing – review & editing. JZ: Writing – original draft, Writing – review & editing. PT: Conceptualization, Writing – review & editing. CM: Conceptualization, Writing – review & editing. GT: Conceptualization, Writing – review & editing. KS: Conceptualization, Writing – review & editing. EB: Conceptualization, Writing – review & editing. DH: Investigation, Methodology, Writing – review & editing. CW: Data curation, Formal analysis, Validation, Writing – review & editing. CR: Investigation, Methodology, Writing – review & editing. GS: Data curation, Formal analysis, Investigation, Methodology, Validation, Writing – review & editing. DL: Conceptualization, Funding acquisition, Investigation, Methodology, Project administration, Supervision, Writing – review & editing.
The author(s) declare financial support was received for the research, authorship, and/or publication of this article. This study was funded by the American Association of Swine Veterinarians Foundation (AASV Foundation) and by the U.S. Department of Agriculture - National Institute of Food and Agriculture (USDA-NIFA) grant #022-68014-36668.
The authors wish to thank the diagnosticians at the ISU VDL who evaluated the tissue submissions and assigned the Dx Codes used in the analysis.
The authors declare that the research was conducted in the absence of any commercial or financial relationships that could be construed as a potential conflict of interest.
The author(s) declared that they were an editorial board member of Frontiers, at the time of submission. This had no impact on the peer review process and the final decision.
All claims expressed in this article are solely those of the authors and do not necessarily represent those of their affiliated organizations, or those of the publisher, the editors and the reviewers. Any product that may be evaluated in this article, or claim that may be made by its manufacturer, is not guaranteed or endorsed by the publisher.
The Supplementary material for this article can be found online at: https://www.frontiersin.org/articles/10.3389/fvets.2023.1301392/full#supplementary-material
1. Gebhardt, JT, Tokach, MD, Dritz, SS, DeRouchey, JM, Woodworth, JC, Goodband, RD, et al. Postweaning mortality in commercial swine production. I: review of non-infectious contributing factors. Transl Anim Sci. (2020) 4:462–84. doi: 10.1093/TAS/TXAA068
2. Gebhardt, JT, Tokach, MD, Dritz, SS, DeRouchey, JM, Woodworth, JC, Goodband, RD, et al. Postweaning mortality in commercial swine production II: review of infectious contributing factors. Transl Anim Sci. (2020) 4:485–506. doi: 10.1093/TAS/TXAA052
3. Banhazi, TM, and Black, JL. Precision livestock farming: a suite of electronic systems to ensure the application of best practice management on livestock farms. Aust J Multi-Disciplinary Eng. (2009) 7:1–14. doi: 10.1080/14488388.2009.11464794
4. Osemeke, OH, Donovan, T, Dion, K, Holtkamp, DJ, and Linhares, DCL. Characterization of changes in productivity parameters as breeding herds transitioned through the 2021 PRRSV breeding herd classification system. J Swine Health Prod. (2022) 30:145–8. doi: 10.54846/JSHAP/1269
5. Shamsabardeh, M, Rezaei, S, Gomez, JP, Martínez-López, B, and Liu, X. A novel way to predict PRRS outbreaks in the swine industry using multiple spatio-temporal features and machine learning approaches. Front Vet Sci. (2019) 6:85. doi: 10.3389/CONF.FVETS.2019.05.00085
6. Vlaminck, J, Düsseldorf, S, Heres, L, and Geldhof, P. Serological examination of fattening pigs reveals associations between Ascaris suum, lung pathogens and technical performance parameters. Vet Parasitol. (2015) 210:151–8. doi: 10.1016/J.VETPAR.2015.04.012
7. Bjustrom-Kraft, J, Woodard, K, Giménez-Lirola, L, Rotolo, M, Wang, C, Sun, Y, et al. Porcine epidemic diarrhea virus (PEDV) detection and antibody response in commercial growing pigs. BMC Vet Res. (2016) 12:99. doi: 10.1186/S12917-016-0725-5
8. Derscheid, RJ, Rahe, MC, Burrough, ER, Schwartz, KJ, and Arruda, B. Disease diagnostic coding to facilitate evidence-based medicine: current and future perspectives. J Vet Diagnostic Investig. (2021) 33:419–27. doi: 10.1177/1040638721999373
9. Magalhães, ES, Zimmerman, JJ, Thomas, P, Moura, CAA, Trevisan, G, Holtkamp, DJ, et al. Whole-herd risk factors associated with wean-to-finish mortality under the conditions of a Midwestern USA swine production system. Prev Vet Med. (2022) 198:105545. doi: 10.1016/J.PREVETMED.2021.105545
10. Schwabe, C. The current epidemiological revolution in veterinary medicine. Part I. Prev Vet Med. (1982) 1:5–15. doi: 10.1016/0167-5877(82)90003-4
11. Agostini, PDS, Manzanilla, EG, De Blas, C, Fahey, AG, Da Silva, CA, and Gasa, J. Managing variability in decision making in swine growing-finishing units. Ir Vet J. (2015) 68:20. doi: 10.1186/s13620-015-0048-z
12. Agostini, PS, Fahey, AG, Manzanilla, EG, O’Doherty, JV, De Blas, C, and Gasa, J. Management factors affecting mortality, feed intake and feed conversion ratio of grow-finishing pigs. Animal. (2014) 8:1312–8. doi: 10.1017/S1751731113001912
13. Goumon, S, and Faucitano, L. Influence of loading handling and facilities on the subsequent response to pre-slaughter stress in pigs. Livest Sci. (2017) 200:6–13. doi: 10.1016/j.livsci.2017.03.021
14. Larriestra, AJ, Maes, DG, Deen, J, and Morrison, RB. Mixed models applied to the study of variation of grower-finisher mortality and culling rates of a large swine production system. Can J Vet Res. (2005) 69:26–31.
15. Larriestra, AJ, Morrison, RB, Deen, J, and Abvp, D. Peer reviewed production tool a decision-making framework for evaluating interventions used at weaning to reduce mortality in lightweight pigs and improve weight gains in the nursery. J Swine Health Prod. (2005) 13:143–9.
16. Losinger, WC, Bush, EJ, Smith, MA, and Corso, BA. An analysis of mortality in the grower/finisher phase of swine production in the United States. Prev Vet Med. (1998) 33:121–45. doi: 10.1016/S0167-5877(97)00052-4
17. Oliveira, J, Yus, E, and Guitián, FJ. Effects of management, environmental and temporal factors on mortality and feed consumption in integrated swine fattening farms. Livest Sci. (2009) 123:221–9. doi: 10.1016/j.livsci.2008.11.016
18. Passafaro, TL, Van De Stroet, D, Bello, NM, Williams, NH, and Rosa, GJM. Generalized additive mixed model on the analysis of total transport losses of market-weight pigs. J Anim Sci. (2019) 97:2025–34. doi: 10.1093/jas/skz087
19. Biondi-Zoccai, G, Romagnoli, E, Agostoni, P, Capodanno, D, Castagno, D, D’Ascenzo, F, et al. Are propensity scores really superior to standard multivariable analysis? Contemp Clin Trials. (2011) 32:731–40. doi: 10.1016/J.CCT.2011.05.006
20. Ferraz, MES, Almeida, HMS, Storino, GY, Sonálio, K, Souza, MR, Moura, CAA, et al. Lung consolidation caused by Mycoplasma hyopneumoniae has a negative effect on productive performance and economic revenue in finishing pigs. Prev Vet Med. (2020) 182:105091. doi: 10.1016/J.PREVETMED.2020.105091
21. Maes, D, Sibila, M, Kuhnert, P, Segalés, J, Haesebrouck, F, and Pieters, M. Update on Mycoplasma hyopneumoniae infections in pigs: knowledge gaps for improved disease control. Transbound Emerg Dis. (2018) 65:110–24. doi: 10.1111/TBED.12677
22. Poeta Silva, APS, Marostica, TP, McDaniel, A, Arruda, BL, Alonso, C, Derscheid, R, et al. Comparison of Mycoplasma hyopneumoniae response to infection by route of exposure. Vet Microbiol. (2021) 258:109118. doi: 10.1016/J.VETMIC.2021.109118
23. Alvarez, J, Sarradell, J, Morrison, R, and Perez, A. Impact of porcine epidemic diarrhea on performance of growing pigs. PLoS One. (2015) 10:e0120532. doi: 10.1371/JOURNAL.PONE.0120532
24. Er, C, Skjerve, E, Brun, E, Hofmo, PO, Framstad, T, and Lium, B. Production impact of influenza a(H1N1)pdm09 virus infection on fattening pigs in Norway. J Anim Sci. (2016) 94:751–9. doi: 10.2527/JAS.2015-9251
25. Er, C, Lium, B, Tavornpanich, S, Hofmo, PO, Forberg, H, Hauge, AG, et al. Adverse effects of influenza a(H1N1)pdm09 virus infection on growth performance of Norwegian pigs – a longitudinal study at a boar testing station. BMC Vet Res. (2014) 10:1–14. doi: 10.1186/S12917-014-0284-6
26. Davis, ME, Sears, SC, Apple, JK, Maxwell, CV, and Johnson, ZB. Effect of weaning age and commingling after the nursery phase of pigs in a wean-to-finish facility on growth, and humoral and behavioral indicators of well-being. J Anim Sci. (2006) 84:743–56. doi: 10.2527/2006.843743x
27. Declerck, I, Dewulf, J, Sarrazin, S, and Maes, D. Long-term effects of colostrum intake in piglet mortality and performance1. J Anim Sci. (2016) 94:1633–43. doi: 10.2527/jas.2015-9564
28. Leliveld, LMC, Riemensperger, AV, Gardiner, GE, O’Doherty, JV, Lynch, PB, and Lawlor, PG. Effect of weaning age and postweaning feeding programme on the growth performance of pigs to 10 weeks of age. Livest Sci. (2013) 157:225–33. doi: 10.1016/j.livsci.2013.06.030
29. López-Vergé, S, Gasa, J, Farré, M, Coma, J, Bonet, J, and Solà-Oriol, D. Potential risk factors related to pig body weight variability from birth to slaughter in commercial conditions. Transl Anim Sci. (2018) 2:383–95. doi: 10.1093/TAS/TXY082
30. Zotti, E, Resmini, FA, Schutz, LG, Volz, N, Milani, RP, Bridi, AM, et al. Impact of piglet birthweight and sow parity on mortality rates, growth performance, and carcass traits in pigs. Rev Bras Zootec. (2017) 46:856–62. doi: 10.1590/S1806-92902017001100004
31. Main, RG, Dritz, SS, Tokach, MD, Goodband, RD, and Nelssen, JL. Increasing weaning age improves pig performance in a multisite production system. J Anim Sci. (2004) 82:1499–507. doi: 10.2527/2004.8251499x
32. Almeida, MN, Rotto, H, Schneider, P, Robb, C, Zimmerman, JJ, Holtkamp, DJ, et al. Collecting oral fluid samples from due-to-wean litters. Prev Vet Med. (2020) 174:104810. doi: 10.1016/j.prevetmed.2019.104810
33. Alvarez, J, Sarradell, J, Kerkaert, B, Bandyopadhyay, D, Torremorell, M, Morrison, R, et al. Association of the presence of influenza a virus and porcine reproductive and respiratory syndrome virus in sow farms with post-weaning mortality. Prev Vet Med. (2015) 121:240–5. doi: 10.1016/j.prevetmed.2015.07.003
34. Dong, JG, Yu, LY, Wang, PP, Zhang, LY, Liu, YL, Liang, PS, et al. A new recombined porcine reproductive and respiratory syndrome virus virulent strain in China. J Vet Sci. (2018) 19:89–98. doi: 10.4142/JVS.2018.19.1.89
35. Fablet, C, Rose, N, Grasland, B, Robert, N, Lewandowski, E, and Gosselin, M. Factors associated with the growing-finishing performances of swine herds: an exploratory study on serological and herd level indicators. Porc Health Manag. (2018) 4:1–11. doi: 10.1186/s40813-018-0082-9
36. Holtkamp, DJ, Kliebenstein, JB, Neumann, EJ, Zimmerman, JJ, Rotto, HF, Yoder, TK, et al. Assessment of the economic impact of porcine reproductive and respiratory syndrome virus on United States pork producers. J Swine Health Prod. (2013) 21:72–84. doi: 10.2460/javma.2005.227.385
37. Moura, CAA, Johnson, C, Baker, SR, Holtkamp, DJ, Wang, C, and Linhares, DCL. Assessment of immediate production impact following attenuated PRRS type 2 virus vaccination in swine breeding herds. Porc Health Manag. (2019) 5:1–6. doi: 10.1186/S40813-019-0120-2
38. Moura, CAA, Philips, R, Silva, GS, Ramirez, A, Gauger, PC, Holtkamp, DJ, et al. Association of wild-type PRRSV detection patterns with mortality of MLV-vaccinated growing pig groups. Prev Vet Med. (2021) 189:105270. doi: 10.1016/J.PREVETMED.2021.105270
39. Moura, CAA, Philips, R, Silva, GS, Holtkamp, DJ, and Linhares, DCL. Comparison of virus detection, productivity, and economic performance between lots of growing pigs vaccinated with two doses or one dose of PRRS MLV vaccine, under field conditions. Prev Vet Med. (2022) 204:105669. doi: 10.1016/J.PREVETMED.2022.105669
40. Trevisan, G, Robbins, RC, Angulo, J, Dufresne, L, Lopez, WA, Macedo, NL, et al. Relationship between weekly porcine reproductive and respiratory syndrome virus exposure in breeding herds and subsequent viral shedding and mortality in the nursery. J Swine Health Prod. (2020) 28:244–53.
41. Amezcua, R, Friendship, RM, Dewey, CE, Gyles, C, and Fairbrother, JM. Presentation of postweaning Escherichia coli diarrhea in southern Ontario, prevalence of hemolytic E. coli serogroups involved, and their antimicrobial resistance patterns. Can J Vet Res. (2002) 66:73.
42. Griffith, RW, Carlson, SA, and Krull, AC. Salmonellosis. In: Diseases of Swine. JJ Zimmerman, LA Karriker, A Ramirez, KJ Schwartz, GW Stevenson, and J Zhang, editors. Diseases of Swine. Hoboken, NJ, USA: Wiley (2019). 685–708.
43. John, M, and Fairbrother, ÉN. Colibacillosis In: Diseases of Swine. Eds. JJ Zimmerman, LA Karriker, A Ramirez, KJ Schwartz, GW. Stevenson, and J Zhang Diseases of Swine. Hoboken, NJ, USA: Wiley (2019). 685–708.
44. Pedersen, K, Sørensen, G, Löfström, C, Leekitcharoenphon, P, Nielsen, B, Wingstrand, A, et al. Reappearance of salmonella serovar Choleraesuis var. Kunzendorf in Danish pig herds. Vet Microbiol. (2015) 176:282–91. doi: 10.1016/J.VETMIC.2015.01.004
45. Zimmerman, J, Bjustrom-Kraft, J, Christopher-Hennings, J, Daly, R, Main, R, Torrison, J, et al. The use of oral fluid diagnostics in swine medicine. J Swine Health Prod. (2018) 26:262–9. doi: 10.54846/jshap/1091
46. Holtkamp, DJ, Torremorell, M, Corzo, CA, Linhares, D, Almeida, M, Yeske, P, et al. Proposed modifications to porcine reproductive and respiratory syndrome virus herd classification. J Swine Health Prod. (2021) 29:261–70. doi: 10.54846/jshap/1218
47. Zimmerman, JJ, Dee, SA, Holtkamp, DJ, Murtaugh, MP, Stadejek, T, Stevenson, GW, et al. Porcine reproductive and respiratory syndrome viruses (porcine arteriviruses). In: Diseases of Swine. JJ Zimmerman, LA Karriker, A Ramirez, KJ Schwartz, GW Stevenson, and J Zhang, editors. Diseases of Swine. Hoboken, NJ, USA: Wiley (2019). 685–708.
Keywords: swine, wean-to-finish, diagnostic data, risk factors, mortality
Citation: Magalhães ES, Zimmerman JJ, Thomas P, Moura CAA, Trevisan G, Schwartz KJ, Burrough E, Holtkamp DJ, Wang C, Rademacher CJ, Silva GS and Linhares DCL (2024) Utilizing productivity and health breeding-to-market information along with disease diagnostic data to identify pig mortality risk factors in a U.S. swine production system. Front. Vet. Sci. 10:1301392. doi: 10.3389/fvets.2023.1301392
Received: 24 September 2023; Accepted: 29 November 2023;
Published: 11 January 2024.
Edited by:
Julio Alvarez, VISAVET Health Surveillance Centre (UCM), SpainReviewed by:
Jason Ardila Galvis, North Carolina State University, United StatesCopyright © 2024 Magalhães, Zimmerman, Thomas, Moura, Trevisan, Schwartz, Burrough, Holtkamp, Wang, Rademacher, Silva and Linhares. This is an open-access article distributed under the terms of the Creative Commons Attribution License (CC BY). The use, distribution or reproduction in other forums is permitted, provided the original author(s) and the copyright owner(s) are credited and that the original publication in this journal is cited, in accordance with accepted academic practice. No use, distribution or reproduction is permitted which does not comply with these terms.
*Correspondence: Edison S. Magalhães, ZWRpc29uQGlhc3RhdGUuZWR1
Disclaimer: All claims expressed in this article are solely those of the authors and do not necessarily represent those of their affiliated organizations, or those of the publisher, the editors and the reviewers. Any product that may be evaluated in this article or claim that may be made by its manufacturer is not guaranteed or endorsed by the publisher.
Research integrity at Frontiers
Learn more about the work of our research integrity team to safeguard the quality of each article we publish.