- 1Centre for Agribusiness, University of New England, Armidale, NSW, Australia
- 2Food Agility CRC, Sydney, NSW, Australia
- 3School of Management and Marketing, Curtin University, Bentley, WA, Australia
- 4College of Science, Health, Engineering and Education, Murdoch University, Murdoch, WA, Australia
Digital technology is being introduced to global agriculture in a wide variety of forms that are collectively known as digital agriculture. In this paper we provide opportunities and value propositions of how this is occurring in livestock production systems, with a consistent emphasis on technology relating to animal health, animal welfare, and product quality for value creation. This is achieved by organizing individual accounts of digital agriculture in livestock systems according to four broad types—commodity-based; value seeking; subsistence and nature-based. Each type presents contrasting modes of value creation in downstream processing; as well as from the perspective of One Health. The ideal result of digital technology adoption is an equitable and substantial diversification of supply chains, increased monetization of animal product quality, and more sensitive management to meet customer demands and environmental threats. Such changes have a significance beyond the immediate value generated because they indicate endogenous growth in livestock systems, and may concern externalities imposed by the pursuit of purely commercial ends.
Introduction
Recent years have resulted in major changes to personal and professional lives through the introduction of digital technologies. Readers of this paper will have experienced some of these: in finance, transport, retailing, construction and other sectors (1). High expectations are placed on the potential of digital technologies to help agriculture meet the challenges it faces in the coming decades (2–6). Digital agriculture is a relatively recent term, appearing since 2015, to describe a wide range of applications of digital technology within agriculture both on the farm and along the supply chain, as well as in the realms of agriculture's delivery of nature services and public goods; Cook et al. (7) provided a four-part categorization of digital agricultural technologies to broadly define their scope: (1) Data: Sensing the system, (2) Control: Responding to insight, (3) Modeling: Working out what complex multi-sensor data means and (4) Networking and Communication: Increasing the flow of data and insight.
The agricultural sector was assessed by McKinsey & Co (8) in 2015 to be the least digitized of 23 in the US economy and 2 years later to be the least digitized of all Australian sectors (9). However, investment in digital agri-food technology has grown strongly over recent years, even during the coronavirus pandemic. Global venture capital in such activities grew to about $51 billion in 2021 and is entering a phase of maturation (10). This paper addresses the innovations taken by these technical and financial developments in livestock systems.
At the same time as the excitement of investors emerges, Shiller (11) added that investor sentiment is driven by narratives that precede certainty. Investors who risk funds—and scientists who risk valuable years of their careers—often pursue these changes in order to separate hype from reality and understand the nature of digital agriculture change. From a review of many different examples of digital agriculture, Cook et al. (7) concluded that major investment in digital agriculture was indeed likely—if for no other reason than simple arbitrage—but that the drivers of change and its direction remained confused among innovation users, creators and investors. We propose that the diverse technical, financial and behavioral nature of these drivers is a consequence of the different needs and value propositions exhibited across livestock system types. In the current paper we map these value propositions to an array of livestock systems, grouped for convenience into some (perhaps overlapping) functionalities and contexts.
The scope of change portrayed by many writers on digital agriculture is broad, and often focused on technologies rather than the problems or opportunities to which technology is targeted. Shepherd et al. [(12). p. 5,084] defined digital agriculture as “the use of detailed digital information to guide decisions along the agricultural value chain.” Many works reviewed for this paper were restricted to pre-defined sectors of change processes, such as implications for sustainable production (13, 14), the social implications (15), or big data operations (16–21), and the likely impacts in specific areas, such as Australia or other specific country and agricultural development globally (2, 22–24). From these we determined that it was helpful to understand the function of food systems (25) in order to organize the many changes offered by digital agriculture, with particular reference to livestock production systems. We observed that unlike its predecessor, precision agriculture, digital agriculture operated throughout food systems, in the domains of production, processing, marketing, capitals and governance (7), even as the systems morphed to respond to opportunities and threats (26).
The potential for change through digital agricultural and its associated technology might be vast, but change will be realized through value propositions that define how technology use creates value and who acquires it (27). The issue relates to characteristic patterns of technology uses in different sectors of the economy (28). We find the detailed classification developed for manufacturing to be helpful (29) because it describes the range of organizations and behaviors likely to be found in food systems, rather than the perhaps over-simplified inventory approach of Manyika et al. (8) which overlooks the diversity of technology use—including technology embedded in agricultural production systems (30).
In what follows, we draw on food systems thinking (31) to capture the diversity of opportunities and value propositions available to the livestock production sector from digital technologies with a consistent focus on animal welfare for value creation in supply chain systems. Food systems thinking draws together technical, economic and social influences and processes, to provide a multi-objective interpretation of change such as technology adoption. A full understanding of livestock's contributions to the environment, to One Health, and to subsistence households' livelihood are examples of objectives which have been brought to the fore as systems thinking and methods have allowed these to be expanded into something more holistic. The links between animal welfare, feed intake, various behavioral variables, sustainability and productivity have been exploited using digital tools (14, 32–34). The tools comprise technology, with appropriate algorithms, to manipulate data generated in various sensor environments. Understanding how actors acquire (or fail to acquire) value from such technology is important to determine whether or not system change is likely. This is vastly different for the range of livestock systems operating in various regions of the world. From this, we attempt to organize the vast range of applications for digital technologies within the fields of animal health and welfare and the implications of these for supply chains. Our purpose is to enable readers to understand the dynamics of adoption and the emerging scientific fields to support them.
Livestock system types
Livestock systems classification is a mature field of knowledge with seminal enquiry emerging in the 1980s [for example (35, 36)] with further contributions being made in more recent times [for example (37, 38)]. Given that livestock production systems are highly dependent upon downstream value creation (i.e., value creation occurs when commodity products like meat, milk and wool are differentiated at the processing, manufacturing and retail end of the supply chain), Pavitt's (29) classification was helpful in considering livestock systems from a value perspective borne from patterns of technical change, rather than the traditional production perspective. Unlike current knowledge on animal-focused classification schemes, the essence of Pavitt's (29) classification is describing and explaining sectoral-levels of technical change. Pavitt's (29) three-part taxonomy created the foundation of thinking about technical change at scale. It provides a framework for understanding sources and directions of how firms change in the context of diversification behavior and how technical skills and advantages are created. Most pertinent to agri-food supply chains, which are arguably characterized as commodity-based, is the acknowledged nexus between technology and industry structure.
Table 1 presents four illustrative livestock system classes which we discuss. The basis for this grouping is the taxonomy offered to explain the contrasting forms of adoption of technology in industry at the sectore-level (29), to which we apply observations from our experience of livestock sectors in Australia, the UK and elsewhere. We also postulate seven forms of benefit available from digital technologies: these provide a spectrum from private goods such as reduced production costs, to shared benefits throughout the supply chain such as enhanced disease surveillance and sales price increases due to product attribute differentiation, and onwards to public goods such as resource conservation. The body of Table 1 lists digital products and applications, and its bottom row lists the features of each system that enables, at least potentially, value generation from the technology. The right-hand column of the table lists, for each form of value, the enabling factors for livestock production and supply chains. As we shall see, not all systems offer up these features.
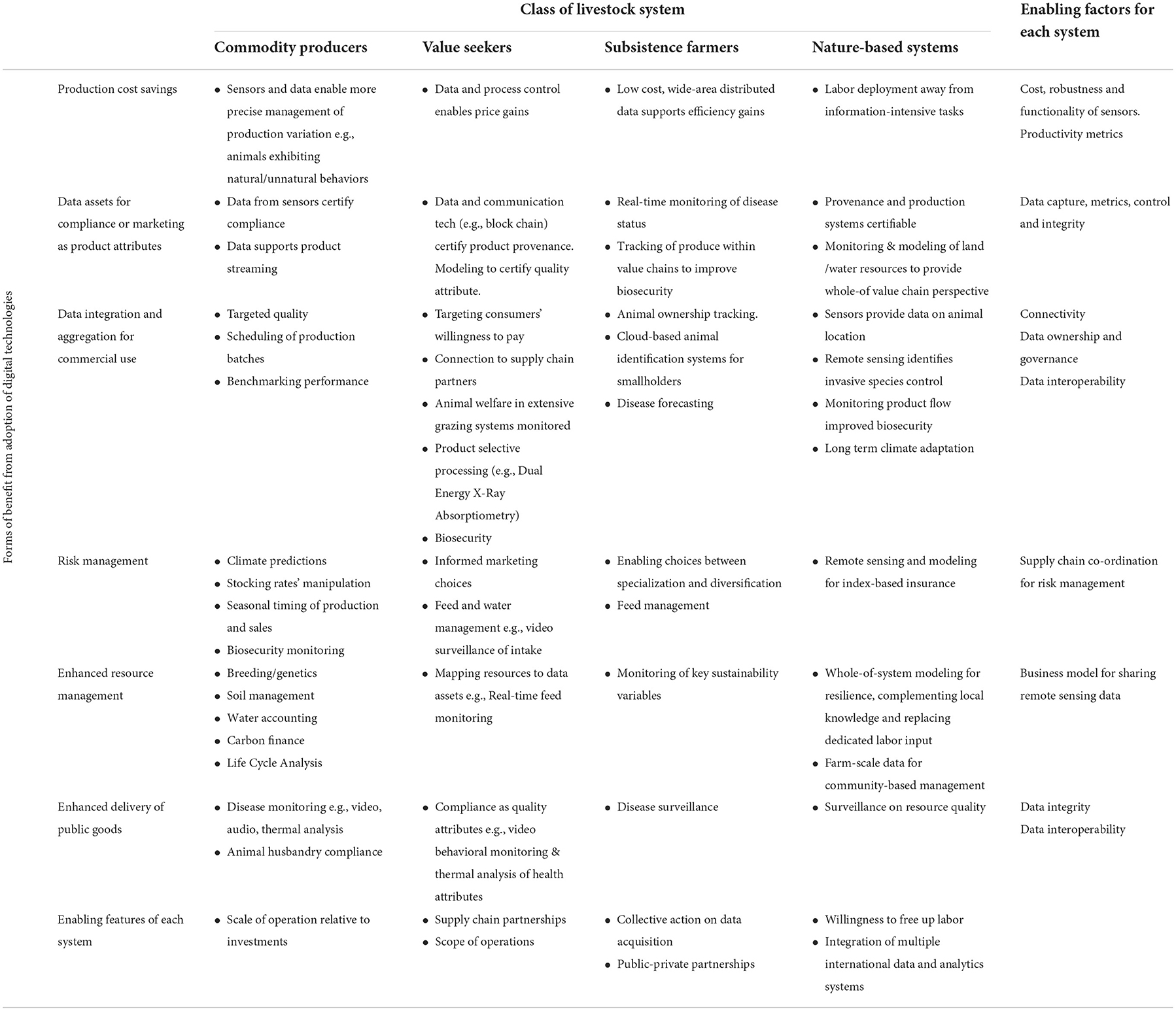
Table 1. General function of digital technologies for animal health, animal welfare and product quality in systems for the four classes of livestock.
We present “commodity producers” as medium to large scale livestock operations serving mature and demanding markets for large volumes of product of consistent quality. Red meat grazing operations in this category are highly seasonal and face associated risks; feedlots and monogastric operations focus on throughput and the risks of price movements. Both feature large scale operations, with cost structures favoring ever more scale. Feed supplies and prices fluctuate, and animal disease is a constant threat managed at significant cost. Information has traditionally been expensive to collect, and rewards difficult to capture. Costs and productivity dominate management objectives, and key metrics include stocking rates, resource efficiency, and timing of operations. Marketing initiatives are led from downstream in the supply chain and are manifest as compliance requirements: particularly for animal welfare and production methods that remain in the responsibility of farmers. Management efficacy is sufficient in that disease control, along with animal welfare, remains a private good in many economies. Enterprise size is sufficient to on-farm water management and emerging needs of farms like mobilizing carbon sequestration.
Our “value seekers” type of livestock operation may be small and mixed with other farm operations, and pursues cost savings on the basis of targeted inputs, and price advantages due to differentiation and the targeting of customers along the supply chain through to consumers. This requires not only collection of information but its transmission and interpretation: first about products' physical attributes but increasingly about provenance and process. The higher value of products invites investment at processing and retail stages of the supply chain, and capturing value features partnerships of various types including data integration. Examples include dairy production targeting consumers seeking consumption and use experiences based on dis-assembly and re-assembly of milk components, and a vertically-coordinated approach to value addition and pricing.
“Subsistence farmers,” as we present them, operate diverse and generally small scale livestock enterprises with products either for family consumption (eggs and milk) or for sale to fund household necessities and social activities. Risks are encountered such as seasonality, market vagaries, and plant and animal disease. Operators are diverse, usually with multiple sources of income, but some threats—notably animal disease—are felt by all and lend themselves to a common response. Readers should note that our classification centres on value and the means of its delivery. Conventional systems' classifications such as “pastoralist” might be invoked for our subsistence type: but the inclusion of scale of operation, shared but intensive resource use, critical timing of operations, and linkage to product and asset markets all mean that we treat some pastoralists as commodity producers, others as nature based, and only some pastoralists as subsistence farmers.
“Nature based systems” are spatially extensive, highly sensitive to climate and disease, and labor intensive livestock systems. Importantly, these systems rely little on purchased or scheduled inputs: communal grazing systems provide an example. Overall management objectives centre on resilience, with animals commonly the store of value as accumulated savings. All operators face the same risks, mostly derived from external factors, and this enables approaches to risk management based on entire systems and landscapes with shared costs. The specification of labor intensiveness refers to these systems' lack of purchased inputs and mechanization: the assets—animals and other assets—are employed jointly with labor which is not generally available for other uses. Tasks such as herding and tending lend themselves strongly to labor saving digital technologies, as we shall see.
An important point to conclude on is that these classification systems of digital agriculture are not limited to corporate agriculture or big business. There is plenty of recent evidence to confirm that digital agriculture is accessible to disparate geographies, demographies, and societies (39–41) so it therefore relatively ubiquitous in democratizing access to technical change. Examples of this equity include the inter-operability of data systems that allow the secure, free-flow of standardized data between stakeholders to minimize information asymmetry and improve decision making (further discussed in section digital technologies) and the early investment of governments and NGOs, particularly in low-income countries, for facilitating the broad-scale adoption of digital agriculture technologies [see (2, 39, 42) as examples].
Digital technologies
Digital applications for better understanding animal welfare and productivity centre on sensors, across a variety of species and technologies (34). Video observation is applied in settings such as feed intake measurement for grazing cattle (43) and flocking behavior in housed poultry (44). These are applicable to the commodity livestock system type. Video-based automated assessment of direct animal health indicators are more applicable to value seekers, such as gait score measurement in poultry (45). Automated detection of specific ailments has employed more specialized optical capabilities in cameras, such as thermal analysis for poultry foot health (46), dermatitis in dairy cattle (47), and African Swine Fever (48).
Audio technology has also addressed animal health and welfare. Surveillance of coughing has been used in detection of respiratory-related wasting disease in pigs (49). Digital analysis of animal vocalizations has yielded management indicators of stress levels in housed chickens (50) and turkeys (51), grazing cattle (52), and stresses associated with ewe-lamb interactions (53). Feather pecking has been monitored by audio sensors (54), and analysis of pecking sounds has been used to measure feed intake and stress in chickens (55). Sensors that monitor odors (electronic nose) can detect animal health and welfare problems for housed poultry (56), and in diagnosing specific bacterial infections in cattle (57). These applications lend themselves to animal welfare surveillance in both commodity and value seeking systems by virtue of costs avoided, and the scale of operation available to offset costs per unit of production. It is notable that for commodity systems the surveillance element is both a cost incurred to assure market access and ethical considerations of animal production, whereas for value seekers the producer attaches consumer value to the product.
For extensive livestock systems, a large number of digital data collection processes are in use. Bahlo et al.'s (58) review identifies the need for decision tools which integrate this variety of data sources, along with the need for inter-operable data systems whereby secure, standardized data sets are shared between stakeholders for improved, evidence-based decision making. These authors identify public benefits arising from the enabling factors shown in the bottom row of Table 1, such that regional data sets are implemented for collaborative resource management; and private benefits in terms of animal productivity and welfare. Index-based drought insurance for East African livestock keepers based on satellite imagery and weather data provides one example (59), and feed supply monitoring with terrestrial sensors in Mongolia (60) another. Furthermore, Elsäßer et al. (42) set out applications of digital systems in Africa that cover farm management, finance, market access, the supply chain and broader macro market intelligence thereby demonstrating the ubiquitous nature of digital systems in a variety of livestock systems (i.e., subsistence, commodity, value-seeking and nature-based system). These require the data inter-operability listed amongst enabling factors in the right hand column of Table 1.
Avoidance or mitigation of disease for the provision of safe and ethically-produced food is offered by digitally enabled surveillance of causal and contributing factors. This has been demonstrated in both intensive animal production (61), and extensive grazing systems (62), and in environmental interactive systems such as tick-livestock relations associated with disease (63). Air quality inside livestock housing and pens is recognized as a contributor to reduced productivity as well as disease (64), and has been subject to control using digital air quality sensors and associated integration of software and hardware (65). This has included measurement of particulate matter and gaseous contaminants (66), and air flow in animal handling systems (67). These interventions address primarily risks and production costs, and existing business models have limited facility for generating consumer value from them. Having said that, no claims are being made herein about these tools being proven indicators of animal welfare; it is acknowledged that human observation of animal welfare remains critical (68). It is implicitly accepted that animal welfare measures are generally more intricate than one set of data on one particular trait and that welfare is a nuanced subject so it is ill-advised to claim good welfare from the results of a single technology. We do maintain however that incentives for management steps taken are strongly rooted in the generation of value along the supply chain, which in turn is enabled by information flows to the manager and onwards to the consumer.
While there is ample evidence of digital systems striving to enhance good animal welfare practice outcomes, sharing of data and data exchange platforms to support the One Health movement are under-explored in the literature. It is therefore recommended that production animals within the wider digital agriculture landscape, and the implications for human health outcomes, are considered in future inquiry.
Conclusions
Digital technology has the potential to enable several important changes in livestock systems. Low-cost, field-robust and high precision sensors provide a substantial influx of cheap data to producers, and potentially onwards to processers, traders and consumers. Available data describes the location, movement and condition of animals, their feed, environment, health, the conditions influencing their welfare, and threats. Meanwhile other technology, like radio frequency identification or blue tooth tags, track, select and control the animal product as it passes through food systems, as per the demand signals from processors and consumers.
High dimensional modeling of multiple data streams offers ever more precise representation of complex food systems using machine learning and artificial intelligence where feasible [see of such technology provided by Fuentes et al. (69)]. As examples, chicken meat systems integrate feed, shed conditions, animal performance and labor availability to automate controllers and optimize such systems as data accumulates. In the case of block chain technology which takes the data along the supply chain, data are shared and stored via distributed cloud systems to enable access to consumers, producers and processors. Such systems are particularly relevant to complex animal production systems, especially those which target specific value opportunities or which face complex problems relating to health, welfare or biosecurity.
The ideal result of adoption is a substantial diversification of supply chains, increased monetization of animal product quality and more sensitive management to meet customer demands and environmental threats. Such changes have a significance beyond the immediate value generated because they indicate endogenous growth in livestock systems: the capacity of actors within livestock systems to manage livestock. We argue that these capacities are evolving along distinct pathways for the different livestock types.
We show a diversity of adoption patterns that conform to a range of economic models as a measure of knowledge to indicate endogenous growth (70). The growth of knowledge that accompanies the technology confers a growing ability to identify, confirm and respond to opportunities and threats in complex livestock systems.
Adoption of digital technologies in animal production systems is likely to expand. Prior to 2015, the term digital agriculture barely appeared. Its growth since then has created a corpus of interest amongst innovators, researchers and investors that requires, as we state in Cook et al. (7), organization according to a food system framework. The scope for the type of endogenous growth demonstrated in Table 1 seems boundless, as the system evolves to meet demands on multiple fronts (71). Adoption is likely to proceed in all four pathways described by Cook et al. [(7). p. 6]: data, control, modeling, and networking. Currently value-driven innovation appears to be ahead of discipline-based research which could support it. We see a bright future for equitable digital systems that create value by managing and reporting animal welfare throughout global agri-food supply chains. We expect future publications will report on advances in these technologies with a focus on sustainability of livestock systems that include digital systems for managing animal welfare and the advantages it brings to the value of livestock and their associated products.
Data availability statement
The original contributions presented in the study are included in the article/reference list, further inquiries can be directed to the corresponding author/s.
Author contributions
All authors listed have made a substantial, direct, and intellectual contribution to the work and approved it for publication.
Funding
Funding contributing to this work is gratefully acknowledged from The World Bank's project—Digital Acceleration of Agricultural Transformation Study: Focus on the Downstream Segment of Agricultural Value Chains, and from Agrifutures and Food Agility CRC project J-011566-Co-designed Scoping Study to Unlock the Power of Digital.
Conflict of interest
The authors declare that the research was conducted in the absence of any commercial or financial relationships that could be construed as a potential conflict of interest.
Publisher's note
All claims expressed in this article are solely those of the authors and do not necessarily represent those of their affiliated organizations, or those of the publisher, the editors and the reviewers. Any product that may be evaluated in this article, or claim that may be made by its manufacturer, is not guaranteed or endorsed by the publisher.
References
1. Trendov NK, Varas S, Zeng M. Digital Technologies in Agriculture Rural Areas - Briefing Paper. Rome: FAO (2019). Available online at: https://www.fao.org/3/ca4887en/ca4887en.pdf (accessed November 21, 2022).
3. World Bank (ed.). World Development Report 2021: Data for Better Lives. World Development Report. Washington: World Bank (2021). Available online at: https://www.worldbank.org/en/publication/wdr2021 (accessed November 21, 2022).
4. World Economic Forum. Food Systems: Data, Digital and Innovation Levers. (2021). Available online at: https://www.weforum.org/events/sustainable-development-impact-summit-2021/sessions/unlocking-food-systems-innovation (accessed November 21, 2022).
5. World Economic Forum. Innovation with a Purpose: Improving Traceability in Food Value Chains Through Technology Innovations. (2019). Available online at: http://www3.weforum.org/docs/WEF_Traceability_in_food_value_chains_Digital.pdf (accessed November 21, 2022).
6. World Economic Forum. Incentivizing Food Systems Transformation. Geneva: World Economic Forum (2020). Available online at: https://www3.weforum.org/docs/WEF_Incentivizing_Food_Systems_Transformation.pdf (accessed November 21, 2022).
7. Cook S, Jackson EL, Fisher MJ, Baker D, Diepeveen D. Embedding digital agriculture into sustainable australian food systems: pathways and pitfalls to value creation. Int J Agricultural Sustain. (2021) 20:346–67. doi: 10.1080/14735903.2021.1937881
8. Manyika J, Ramaswamy S, Khanna S, Sarrazin H, Pinkus G, Sethupathy G, et al. Digital America: A Tale of the Haves and Have-Mores. McKinsey Global Institute. (2015). Available online at: https://www.mckinsey.com/~/media/mckinsey/industries/technology%20media%20and%20telecommunications/high%20tech/our%20insights/digital%20america%20a%20tale%20of%20the%20haves%20and%20have%20mores/digital%20america%20full%20report%20december%202015.pdf (accessed November 21, 2022).
9. Blackburn S, Gartner D, Freeland M, Kelley A, Pickover S, Thomassian S, et al. Digital Australia: Seizing the Opportunity from the Fourth Industrial Revolution. McKinsey and Company. (2017). Available online at: https://www.mckinsey.com/~/media/mckinsey/featured%20insights/asia%20pacific/digital%20australia%20seizing%20the%20opportunity%20from%20the%20fourth%20industrial%20revolution/digital-australia-seizing-the-opportunity-from-the-fourth-industrial-revolution-vf.pdf (accessed November 21, 2022).
10. Burwood-Taylor L, Leclerc R, Chauhan R. AgFunder AgriFoodTech Investment Report. AgFunder. Available online at: Agfunder.com (2022). (accessed November 21, 2022).
11. Shiller RJ. Narrative Economics. Working Paper 23075. Cambridge, MA: NBER (2017). Available online at: http://www.nber.org/papers/w23075 (accessed November 21, 2022).
12. Shepherd MJ, Turner A, Small B, Wheeler D. Priorities for science to overcome hurdles thwarting the full promise of the ‘digital agriculture' revolution. J Sci Food Agric. (2020) 100:5083–92. doi: 10.1002/jsfa.9346
13. Basso B, Antle J. Digital agriculture to design sustainable agricultural systems. Nat Sustain. (2020) 3:254–6. doi: 10.1038/s41893-020-0510-0
14. Lajoie-O'Malley A, Bronson K, van der Burg S, Klerkx L. The future(s) of digital agriculture and sustainable food systems: an analysis of high-level policy documents. Ecosystem Serv. (2020) 45:101183. doi: 10.1016/j.ecoser.2020.101183
15. Klerkx L, Jakku E, Labarthe P. A review of social science on digital agriculture, smart farming and agriculture 4.0: new contributions and a future research agenda. NJAS. (2019) 90:100315. doi: 10.1016/j.njas.2019.100315
16. Barnes TJ. Big data, little history. Dialog Human Geogr. (2013) 3:297–302. doi: 10.1177/2043820613514323
17. Coble KH, Mishra AK, Ferrell S, Griffin T. Big data in agriculture: a challenge for the future. Appl Econ Perspect Policy. (2018) 40:79–96. doi: 10.1093/aepp/ppx056
18. Kunisch M. Big Data in agriculture - perspectives for a service organisation. Landtechnik. (2016) 71:1–3.
19. Kamilaris A, Kartakoullis A, Prenafeta-Boldú FX. A review on the practice of big data analysis in agriculture. Comput Electr Agricult. (2017) 143:23–37. doi: 10.1016/j.compag.2017.09.037
20. NAAS. Big Data Analytics in Agriculture. Policy Paper 101. New Delhi: National Academy of Agricultural Sciences (2021). Available online at: http://naas.org.in/Policy%20Papers/policy%20101.pdf (accessed November 21, 2022).
21. Wolfert S, Ge L, Verdouw C, Bogaardt MJ. Big data in smart farming – a review. Agricul Syst. (2017) 153:69–80. doi: 10.1016/j.agsy.2017.01.023
22. Heath R. An analysis of the potential of digital agriculture for the australian economy. Farm Policy J. (2018) 15:15.
23. Deichmann U, Goyal A, Mishra D. Will digital technologies transform agriculture in developing countries? Policy Research Paper 7669. Washington, DC: World Bank (2016).
25. von Braun J, Afsana K, Ottilie Fresco L, Hassan M, Torero M. Food system concepts and definitions for science and political action. Nature Food. (2021) 2:748–50. doi: 10.1038/s43016-021-00361-2
26. Béné C, Fanzo J, Prager SD, Achicanoy HA, Mapes BR, Alvarez Toro P, et al. Global drivers of food system (un)sustainability: a multi-country correlation analysis. PLoS ONE. (2020) 15:e0231071. doi: 10.1371/journal.pone.0231071
27. Baker D, Cook SE, Jackson E. Digital agriculture as a disruption and transformation in food systems: who gets value from it? In: 4th Online Symposium on Agri-Tech Economics for Sustainable Futures. (2021). p. 20–21.
28. Malerba F. Sectoral systems: how and why innovation differs across sectors. In: Fageberg J, Mowery D, editors. The Oxford Handbook of Innovation. Oxford: Oxford University Press (2006). p. 380–406. doi: 10.1093/oxfordhb/9780199286805.003.0014
29. Pavitt K. Sectoral patterns of technical change: towards a taxonomy and a theory. Res Policy. (1984) 13:343–73. doi: 10.1016/0048-7333(84)90018-0
30. Punzo LF, Feijó C, Puchet M. (eds.). Beyond the global crisis: structural adjustments and regional integration in Europe and Latin America. Routledge Studies in the Modern World Economy 106. Milton Park, Abingdon, Oxon; New York: Routledge (2012). doi: 10.4324/9780203117828
31. Ericksen PJ. Conceptualizing food systems for global environmental change research. Global Environ Change. (2008) 18:234–45. doi: 10.1016/j.gloenvcha.2007.09.002
32. Dawkins MS. Does smart farming improve or damage animal welfare? Technology and what animals want. Front Anim Sci. (2021) 2:736536. doi: 10.3389/fanim.2021.736536
33. Groher T, Heitkämper K, Umstätter C. Digital technology adoption in livestock production with a special focus on ruminant farming. Animal. (2020) 14:2404–13. doi: 10.1017/S1751731120001391
34. Halachmi I, Guarino M, Bewley J, Pastell M. Smart animal agriculture: application of real-time sensors to improve animal well-being and production. Annual Rev Anim Biosci. (2019) 7:403–25. doi: 10.1146/annurev-animal-020518-114851
35. FAO. The Classification of World Livestock Systems. A study prepared for the Animal Production and Health Division of FAO. AGA/MISC/80/3 (1980).
37. Brock J, Lange M, Tratalos JA, More SJ, Graham DA, Guelbenzu-Gonzalo M, et al. Combining expert knowledge and machine-learning to classify herd types in livestock systems. Sci Rep. (2021) 11:2989. doi: 10.1038/s41598-021-82373-3
38. Pandey, H. O., and Upadhyay, D. “Global livestock production systems: classification, status, and future trends,” in Emerging Issues in Climate Smart Livestock Production. (2022). p. 47–70. doi: 10.1016/B978-0-12-822265-2.00017-X
39. Burra D, Hildebrand J, Giles J, Nguyen T, Hasiner E, Schroeder K, et al. Digital agriculture profile: Viet Nam. Rome: FAO (2021). Available online at: https://hdl.handle.net/10568/113515 (accessed November 21, 2022).
40. Osiemo J, Girvetz E, Hasiner E, Schroeder K, Treguer D, Juergenliemk A, et al. Digital Agriculture Profile: Kenya. Rome: FAO (2021). Available online at: https://www.fao.org/3/cb3958en/cb3958en.pdf (accessed November 21, 2022).
41. Tsan M, Totapally S, Hailu M, Addom B. The Digitalisation of African Agriculture Report 2018–2019. Wageningen: CTA/Dalberg Advisers (2019). Available online at: https://hdl.handle.net/10568/101498 (accessed November 21, 2022).
42. Elsäßer R, Hänsel G, Feldt T. Digitalizingt the African Livestock Sector. Bonn: GIS (2021). Available online at: https://www.giz.de/de/downloads/giz2021_en_Digitalizing%20the%20African%20livestock%20sector.pdf (accessed November 21, 2022).
43. Werner J, Leso L, Umstatter C, Niederhauser J, Kennedy E, Geoghegan A, et al. Evaluation of the RumiWatchSystem for measuring grazing behaviour of cows. J Neurosci Methods. (2018) 300:138–46. doi: 10.1016/j.jneumeth.2017.08.022
44. Neves DP, Mehdizadeh SA, Tscharke M, de Alencar Nääs I, Banhazi TM. Detection of flock movement and behaviour of broiler chickens at different feeders using image analysis. Informat Proc Agricult. (2015) 2:177–82. doi: 10.1016/j.inpa.2015.08.002
45. Dawkins MS, Lee H-J, Waitt CD, Roberts SJ. Optical flow patterns in broiler chicken flocks as automated measures of behaviour and gait. Appl Anim Behav Sci. (2009) 119:203–9. doi: 10.1016/j.applanim.2009.04.009
46. Wilcox C, Patterson J, Cheng HW. Use of thermography to screen for subclinical bumblefoot in poultry. Poult Sci. (2009) 88:1176–80. doi: 10.3382/ps.2008-00446
47. Harris-Bridge G, Young L, Handel I, Farish M, Mason C, Mitchell MA, et al. The use of infrared thermography for detecting digital dermatitis in dairy cattle: what is the best measure of temperature and foot location to use? Vet J. (2018) 237:26–33. doi: 10.1016/j.tvjl.2018.05.008
48. Fernández-Carrión E, Martínez-Avilés M, Ivorra B, Martínez-López B, Ramos ÁM, Sánchez-Vizcaíno JM. Motion-based video monitoring for early detection of livestock diseases: the case of African swine fever. PLoS ONE. (2017) 12:e0183793. doi: 10.1371/journal.pone.0183793
49. Chung Y, Oh S, Lee J, Park D, Chang H-H, Kim S. Automatic detection and recognition of pig wasting diseases using sound data in audio surveillance systems. Sensors. (2013) 13:12929–42. doi: 10.3390/s131012929
50. Lee J, Noh B, Jang S, Park D, Chung Y, Chang H-H. Stress detection and classification of laying hens by sound analysis. Asian-australas J Anim Sci. (2015) 28:592. doi: 10.5713/ajas.14.0654
51. Liu L, Ni J-Q, Li Y, Erasmus M, Stevenson R, Shen M. Assessment of heat stress in turkeys using animal vocalization analysis. In: Paper presented at the 2018 ASABE Annual International Meeting. (2018). doi: 10.13031/aim.201801743
52. Meen G, Schellekens M, Slegers M, Leenders N, van Erp-van der Kooij E, Noldus LP. Sound analysis in dairy cattle vocalisation as a potential welfare monitor. Comput Electr Agricult. (2015) 118:111–5. doi: 10.1016/j.compag.2015.08.028
53. Bishop J, Falzon G, Trotter M, Kwan P, Meek P. Sound analysis and detection, and the potential for precision livestock farming-a sheep vocalization case study. In: Paper Presented at the Proceedings of the 1st Asian-Australasian Conference on Precision Pastures and Livestock Farming. Hamilton (2017).
54. Bright A. Vocalisations and acoustic parameters of flock noise from feather pecking and non-feather pecking laying flocks. Br Poult Sci. (2008) 49:241–9. doi: 10.1080/00071660802094172
55. Aydin A, Bahr C, Berckmans D. An innovative monitoring system to measure the feed intake of broiler chickens using pecking sounds. In: 6th European Conference on Precision Livestock Farming. Leuven, Belgium (2013).
56. Sohn JH, Hudson N, Gallagher E, Dunlop M, Zeller L, Atzeni M. Implementation of an electronic nose for continuous odour monitoring in a poultry shed. Sensors Actuators B. (2008) 133:60–9. doi: 10.1016/j.snb.2008.01.053
57. Fend R, Geddes R, Lesellier S, Vordermeier H-M, Corner L, Gormley E, et al. Use of an electronic nose to diagnose Mycobacterium bovis infection in badgers and cattle. J Clin Microbiol. (2005) 43:1745–51. doi: 10.1128/JCM.43.4.1745-1751.2005
58. Bahlo C, Dahlhaus P, Thompson H, Trotter M. The role of interoperable data standards in precision livestock farming in extensive livestock systems: A review. Comput Electr Agriculture. (2019) 156:459–66. doi: 10.1016/j.compag.2018.12.007
59. Banerjee RR, Gobu W, Zewdie, Y. Evaluating the Service Delivery Model for Index-Based Livestock Insurance (IBLI): Insights From Ethiopia. ILRI Research Brief (2020). Available online at: https://cgspace.cgiar.org/handle/10568/109880 (accessed November 21, 2022).
60. Kim J, Kang S, Seo B, Narantsetseg A, Han Y. Estimating fractional green vegetation cover of Mongolian grasslands using digital camera images and MODIS satellite vegetation indices. GIScience Remote Sens. (2020) 57:49–59. doi: 10.1080/15481603.2019.1662166
61. Borgonovo F, Ferrante V, Grilli G, Pascuzzo R, Vantini S, Guarino M. A data-driven prediction method for an early warning of coccidiosis in intensive livestock systems: a preliminary study. Animals. (2020) 10:747. doi: 10.3390/ani10040747
62. Barwick J, Lamb D, Dobos R, Schneider D, Welch M, Trotter M. Predicting lameness in sheep activity using tri-axial acceleration signals. Animals. (2018) 8:12. doi: 10.3390/ani8010012
63. Babayani ND, Makati A. Predictive analytics of cattle host and environmental and micro-climate factors for tick distribution and abundance at the livestock–wildlife interface in the lower Okavango delta of Botswana. Front Vet Sci. (2021) 8:698395. doi: 10.3389/fvets.2021.698395
64. Soliman ES, Moawed SA, Hassan RA. Influence of microclimatic ammonia levels on productive performance of different broilers' breeds estimated with univariate and multivariate approaches. Vet World. (2017) 10:880. doi: 10.14202/vetworld.2017.880-887
65. Pereira WF, da Silva Fonseca L, Putti FF, Góes BC, de Paula Naves L. Environmental monitoring in a poultry farm using an instrument developed with the internet of things concept. Comput Electron Agricult. (2020) 170:105257. doi: 10.1016/j.compag.2020.105257
66. Yasmeen R, Ali Z, Tyrrel S, Nasir ZA. Estimation of particulate matter and gaseous concentrations using low-cost sensors from broiler houses. Environ Monit Assess. (2019) 191:1–10. doi: 10.1007/s10661-019-7582-1
67. Pardo R, González C, Fariña J, Valdés MD, Rodríguez-Andina JJ, Portela M. Measurement of air flow in newborn poultry transportation systems. In: Paper Presented at the IECON 2017-43rd Annual Conference of the IEEE Industrial Electronics Society. Beijing: IEEE (2017). doi: 10.1109/IECON.2017.8216604
68. Berckmans D. Precision livestock farming technologies for welfare management in intensive livestock systems. Revue Sci Technique. (2014) 33:189–96. doi: 10.20506/rst.33.1.2273
69. Fuentes S, Viejo CG, Tongson E, Dunshea FR. The livestock farming digital transformation: implementation of new and emerging technologies using artificial intelligence. Animal Health Res Rev. (2022) 23:59–71. doi: 10.1017/S1466252321000177
70. Wichmann T. Economic development, endogenous growth, and agriculture. In: Agricultural Technical Progress and the Development of a Dual Economy, ed T Wichmann. Contributions to Economics. Heidelberg: Physica-Verlag HD (1997). p. 6–37. doi: 10.1007/978-3-642-46994-7_2
Keywords: digital agriculture, livestock systems, supply chain, product quality, adoption
Citation: Baker D, Jackson EL and Cook S (2022) Perspectives of digital agriculture in diverse types of livestock supply chain systems. Making sense of uses and benefits. Front. Vet. Sci. 9:992882. doi: 10.3389/fvets.2022.992882
Received: 13 July 2022; Accepted: 17 November 2022;
Published: 02 December 2022.
Edited by:
Bouda Vosough Ahmadi, European Commission for the Control of Foot and Mouth Disease (EuFMD), ItalyReviewed by:
Polly Compston, Royal Veterinary College (RVC), United KingdomDannele E. Peck, Agricultural Research Service (USDA), United States
Copyright © 2022 Baker, Jackson and Cook. This is an open-access article distributed under the terms of the Creative Commons Attribution License (CC BY). The use, distribution or reproduction in other forums is permitted, provided the original author(s) and the copyright owner(s) are credited and that the original publication in this journal is cited, in accordance with accepted academic practice. No use, distribution or reproduction is permitted which does not comply with these terms.
*Correspondence: Elizabeth L. Jackson, ZWxpemFiZXRoLmphY2tzb25AY3VydGluLmVkdS5hdQ==