- 1College of Veterinary Medicine, Northeast Agricultural University, Harbin, China
- 2College of Computer Science, Huanggang Normal University, Huanggang, China
- 3Institute of Chinese Materia Medica, Heilongjiang Academy of Chinese Medicine Sciences, Harbin, China
- 4Heilongjiang Key Laboratory for Animal Disease Control and Pharmaceutical Development, Harbin, China
Natural products and their unique polypharmacology offer significant advantages for finding novel therapeutics particularly for the treatment of complex diseases. Meanwhile, Traditional Chinese Medicine exerts overall clinical benefits through a multi-component and multi-target approach. In this study, we used the previously established co-infection model of Mycoplasma gallisepticum and Escherichia coli as a representative of complex diseases. A new combination consisting of 6 herbs were obtained by using network pharmacology combined with transcriptomic analysis to reverse screen TCMs from the Chinese medicine database, containing Isatdis Radix, Forsythia Fructus, Ginkgo Folium, Mori Cortex, Licorice, and Radix Salviae. The results of therapeutic trials showed that the Chinese herbal compounds screened by the target network played a good therapeutic effect in the case of co-infection. In summary, these data suggested a new method to validate target combinations of natural products that can be used to optimize their multiple structure-activity relationships to obtain drug-like natural product derivatives.
Introduction
Polypharmacology has been widely recognized as a new approach of modern drug discovery (1, 2), which might enable natural products to surpass the use of the traditional single-target drugs in terms of efficiency (3). Natural products with polypharmacological profiles show a promising prospect for the development of novel therapeutics for various complex diseases (4–6). The methods commonly used in polypharmacology include Molecular docking (7, 8), Network-based approaches (9, 10), and Omics-based systems biology approaches (11–13) along with the development of in silico pharmacology (14). In comparison to modern medicine, one of the advantages of TCM is the principle of herb compatibility, known as TCM formulae. However, the rationale of combining specific herb combinations remains unclear, and the space of herb combinations can be explored more effectively by using network pharmacology method (15, 16). More and more research methods on Chinese herbal compounds have been reported in recent years. Pan et al.'s study focused on the intervention of Huanglian Decoction in rats with type 2 diabetes mellitus by the methods of network pharmacology and metabolomics (17). Zhou et al. probed into the effect of Liuwei Dihuang decoction on the neuroendocrine immunomodulation network (18). In the present study, network pharmacology and systems biology will enable us to deliberately design lead molecules with expected polypharmacology as well as offer opportunities for TCM formula repurposing.
Due to the intensification of commercial poultry production, explosive multiple respiratory infections have become an urgent problem (19, 20). This co-pathogenesis is characterized by complex interactions between co-infection pathogens and the host (21, 22). Combined with the previous researches on the co-infection of Mycoplasma gallisepticum (MG) and Escherichia coli (E. coli) in our laboratory (20, 23), we tried to screen a new Chinese medicinal compound (NCMC) with the method of polypharmacology. There are many examples of natural products being used in drug discovery efforts that are directed at a wide range of indications beyond their traditional strengths with the development of research technologies (24–27). The study could establish a new methodology for the research of Chinese herbal medicinal extract and provide a new multi-target treatment method for the occurrence of co-infection.
Materials and methods
Bacterial infection and experimental groupings
MG Rlow (Mycoplasma gallisepticum strain R) strain was obtained from the Harbin Institute of Veterinary Medicine (Chinese Academy of Agricultural Sciences), which was grown in a Modified Hayflicks medium. Escherichia coli O78 was isolated from chickens infected with colibacillosis in our laboratory and cultured in Nutrient Broth (Beijing Aoboxing BIO-TECH Co., Ltd.). The concentration of MG and E. coli were 1 × 109 CCU/ml and 109 CFU/ml, respectively. The detection of the density for MG and E. coli were consistent as explained in our previous study (23, 28).
Forty (1 day old) commercial Leghorn chickens were obtained from Chia Chau Chicken Farm (Harbin, Heilongjiang, China) and were assigned randomly to 2 groups (5 replicates of 20 chickens per group) namely the Control group and Co-infection group as described in our earlier study (23), (A) Control group: Fed only basal diet; (B) Co-infection group: 0.2 ml of MG medium (1 × 109 CCU/ml) was injected into the left caudal thoracic air sac on the 7th day, and 0.1 ml of E. coli bacteria (109 CFU/ml) was injected intraperitoneally on day 10. On the 13th day, 20 chickens from each group were euthanized using the method of cardiac blood collection. Lung samples in each group were collected for RNA-seq, while the serum samples were collected for non-targeted metabolomics.
Collection of co-infection target genes and construction of TCM-target network
RNA was extracted by Trizol reagent (Invitrogen Inc., Carlsbad, CA) from lung tissue and was utilized to construct the final library (BGISEQ-500 RNA-Seq Library) (23). DEG-seq (Differentially Expressed Gene-sequencing) method was based on the Poisson distribution (Fold Change > 2 and Adjusted P-value < 0.001) (29, 30). According to the KEGG (Kyoto Encyclopedia of Genes and Genomes) annotation results and the official classification, we separately classified the functional and biological pathways of the DEGs. The DEGs from different sub-categories were compared to the STRING database by DIAMOND (www.diamondsearch.org/) (31). To obtain PPI (Protein-protein Interaction) diagram, the gene interactions were obtained by homology with known proteins. The network relations with a score ≥ 300 were screened out for mapping. After that, the genes with the highest score in each PPI diagrams were selected as target genes and used for subsequent network construction.
In the process of network construction, we first collected all the information of the TCMSP database (http://tcmspw.com/tcmsp.php) and obtained the total network of “TCM-component-target”, which was submitted in Supplementary material 1. We then put the collected target genes information into the total network for the reverse screening of TCM. The TCM was sorted according to the correlation degree of nodes in the reverse screening network. Furthermore, we classified the TCM based on different functions (Chinese Pharmacopoeia 2015 Edition) and chose the top1 of each classification, including Isatdis Radix, Forsythia Fructus, Ginkgo Folium, Mori Cortex, Licorice, and Radix Salviae. The details for these single Chinese medicinal herbs and materials and their roles based on TCM theory are listed in Supplementary material 2. Finally, the TCM-component-target network was established using Cytoscape 3.6.1 software (Bethesda, MD, USA).
Preparation of NCMC
To verify whether the selected compound has a better therapeutic effect and determine the proportion of six herbs. Hence, we had made a treatment experiment based on the uniform design with the methods of pharmacodynamics and minimum inhibitory concentration (MIC). The multi-nonlinear regression equation was established by data processing system according to the comprehensive index. The results showed that the ratio of Isatdis Radix, Forsythia Fructus, Ginkgo Folium, Mori Cortex, Licorice, and Radix Salviae were 14:7:11:12:5:3 showed effective treatment. The design optimization details of NCMC for the treatment of co-infection was provided in Supplementary material 3.
Six herbs were purchased from Runhe Chinese medicine processing plant Ltd. Aqueous extract of NCMC was prepared as the following procedure. The medicinal materials were mixed in proportion and were macerated for 1 h in 10-folds distilled water (v/w), and then decocted for 1 h, after which the filtrate was collected and the residue was decocted again for 1 h up to 6-folds (v/w) in distilled water. The filtrates were mixed and condensed and then dried by vacuum-drier at 60°C (32, 33). The final concentration of the aqueous extract is 1 mg/mL.
UHPLC-QTOF-MS analysis for components quantification
100 μL sample was added to 400 μL of an extracted solution containing 1.25 μg/mL of internal standard which was dissolved in water. After 30 s vortex, the samples were sonicated for 10 min in an ice-water bath. After the samples were incubated at −40°C for 1 h, then the sample was centrifuged at 12,000 rpm for 15 min at 4°C. Finally, the supernatant was put in a fresh 2 mL tube and 200 μL was transferred to a fresh glass vial for LC-MS/MS analysis (34, 35). LC separation was performed on the Nexera UHPLC LC-30A system (SHIMADZU, Japan) with a Waters BEH C18 column (1.7 μm*2.1*100 mm, Waters, USA). Mobile phases, water with 0.1% formic acid (A) and acetonitrile (B), were applied with gradient elution and the flow rate was kept at 0.4 mL/min. AB 5600 Triple TOF system (SCIEX, USA) was used to collect primary and secondary MS data based on IDA function under the control software (Analyst TF 1.7 (AB Sciex). Instrument dependent parameters: curtain gas = 35 psi, IonSpray voltage = +5,500 (POS)/−4,000 (NEG) V, nebulizer gas = 55 psi, heater gas = 55 psi, source temperature = 550°C. The original mass spectrometry data was imported using Progenesis QI software. The corresponding TCM metabolic database in the compound was established, and the peaks containing MS/MS data were identified by the self-built secondary mass spectrometry database (Biotree Biomedical Technology Co., LTD, China).
NCMC treatment and groupings
A total of 120 White Leghorn chickens was purchased from Chia Chau Chicken Farm (Harbin, Heilongjiang, China), which were divided randomly into 4 groups as follows. Control group (A): Chickens in the Control group were fed in the same environment and kept until the end of experiments; Co-infection group (B): The co-infection model was constructed as previously; Co-infection + NCMC administration group (C): The same infection model as mentioned, then treated with the aqueous extract of NCMC, and given orally by gavage. The treatment started on day 13 and continued for 5 days, once a day at a dose of 450 mg/kg; NCMC control group (D): The same dose of NCMC (450 mg/kg) was given orally to chickens by gavage started at day 13 and continued for 5 days. On the 18th day, 20 chickens from each group were euthanized using the method of cardiac blood collection. Lung, tracheal, and serum samples in each group were collected for further analysis.
Gross and microscopic examination
The severity of the gross air sac lesions and tracheal lesion scores were calculated on the basis of 0–3 scoring system as mentioned in our previous study (36). The tracheal tissues were fixed in 10% formalin, dehydrated, and immersed in the transparent samples of wax, cut into slices (4 μm), stained with hematoxylin and eosin (H&E). Secondly, the tracheal tissues were trimmed into small pieces of 1 mm3 and fixed overnight in 2.5% glutaraldehyde. They were washed with PBS twice and post-fixed in 1% osmium tetroxide at 4°C for 1 h. Next, the tissues were dehydrated by ethanol series and 100% acetone, embedded in epoxy resins. The ultrathin sections were stained with uranyl acetate and lead citrate and then observed under a GEM-1200ES transmission electron microscope (JEOL Ltd., Tokyo, Japan). Lastly, 1 mm3 piece of tracheal tissues was also observed under scanning electron microscopy (SEM, SU8010, HITACHI Ltd., Japan).
Statistical analysis
The KEGG classification and the PPI maps were made by the BGI data mining online website (http://report.bgi.com). NCMC component classification diagram was made by RAW graphs (https://rawgraphs.io/). The data of microscopic lesion scores were analyzed with the Mann–Whitney U-test and the other data were analyzed with the one-way analysis method of the GraphPad Prism (version 8.02), using Tukey's student test method. The value of P ≤ 0.05 is considered statistically significant and P ≤ 0.01 as extremely significant.
Results
Transcriptome sequencing of co-infection group and targets screening
In this study, multi-omics analyses were performed on the co-infection model as explained previously (23). According to the results of RNA-seq, 3,115 DEGs were found between the co-infection and the control groups, including 1,456 genes upregulated and 1,659 genes downregulated. These DEGs were widely distributed in 44 sub-categories in six major categories in the KEGG pathway database, as shown in Figure 1A. The PPI results in each KEGG classification were submitted in Figure 1B.
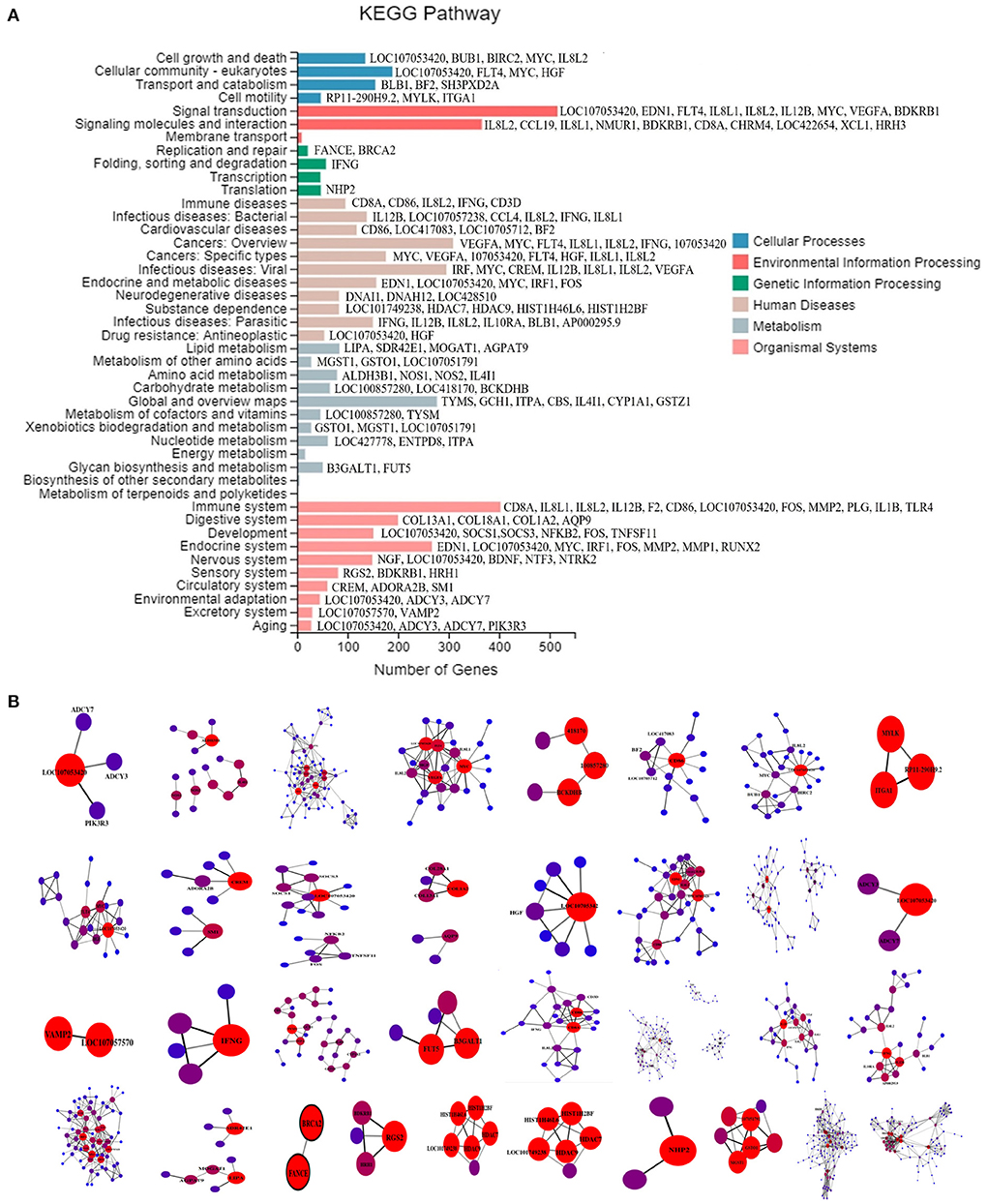
Figure 1. (A) KEGG classification map of DEGs. KEGG categorization of the DEGs in the transcriptome of chicken lung induced by co-infection. The X-axis is the number of genes annotated to a KEGG Pathway classification, and the Y-axis is the KEGG Pathway classification. The gene symbols behind the histogram are the target genes screened by PPI. (B) PPI maps of DEGs in co-infection group. The Gene marked with Gene Symbol is the target Gene whose interaction relationship is >300. The brighter the color is, the greater the node correlation and the stronger the interaction relationship is.
Reverse screening of NCMC in TCMSP database
We first collected the entire data of the TCMSP Chinese Medicine Database including 499 TCMs and their constituent information (13,889 TCM molecules) and corresponding drug targets (702), and organized them into a TCM network containing 113,265 relationships which was submitted in Supplementary material 1. Fifty-seven target genes screened by PPI were substituted into the Drug bank database (Table 1) and 25 effective targets were obtained. These 25 targets were brought into the TCMSP total network for the reverse screening of Chinese medicine, and the results showed that 15 targets were matched with the TCMSP database (Reverse network as shown in Supplementary material 4). Afterward, we sorted the correlation degree of Chinese medicine nodes and selected six high-ranked Chinese medicines according to the classification of Chinese medicines. The six herbs were Isatdis Radix, Forsythia Fructus, Ginkgo Folium, Mori Cortex, Licorice, and Radix Salviae, and the final constructed “target-component-TCM” network of TCM compound was shown in Figure 2.
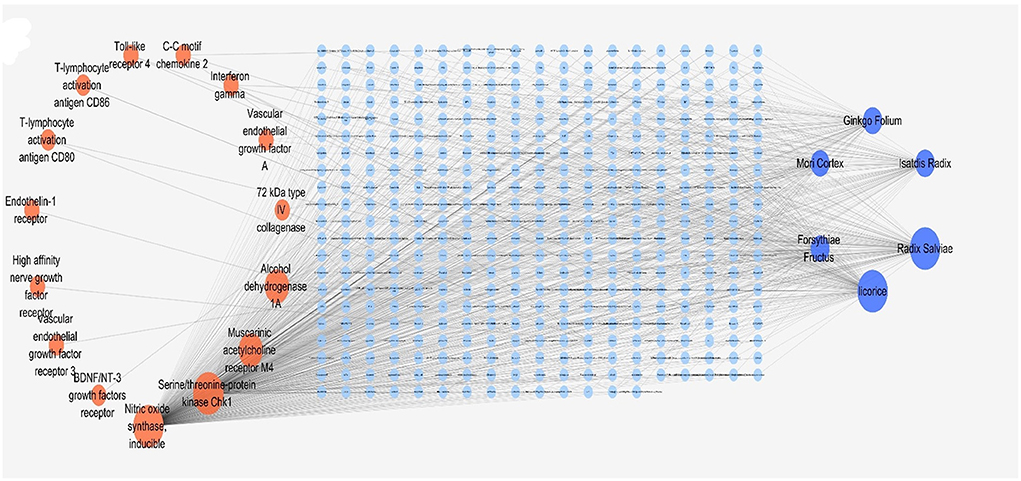
Figure 2. The predicted “target-component-TCM” network. The orange dots were the effective targets, the dark blue dots were the six Chinese medicines obtained by reverse screening, and the light blue dots were the components of Chinese medicines.
Component compounds of NCMC aqueous extraction
To clarify the effective ingredients of NCMC, a non-targeted metabolomics method was used to study the aqueous extraction. The results showed that 260 compounds (including positive and negative modes) were detected on the receiver side using UPLC-QTOF-MS/MS. Among them, 85 compounds belong to flavonoids, 64 compounds belong to organic acids, and 17 compounds belong to phenylpropanoids, and so on, as shown in Figure 3. The complete composition data of NCMC is provided in Supplementary material 5.
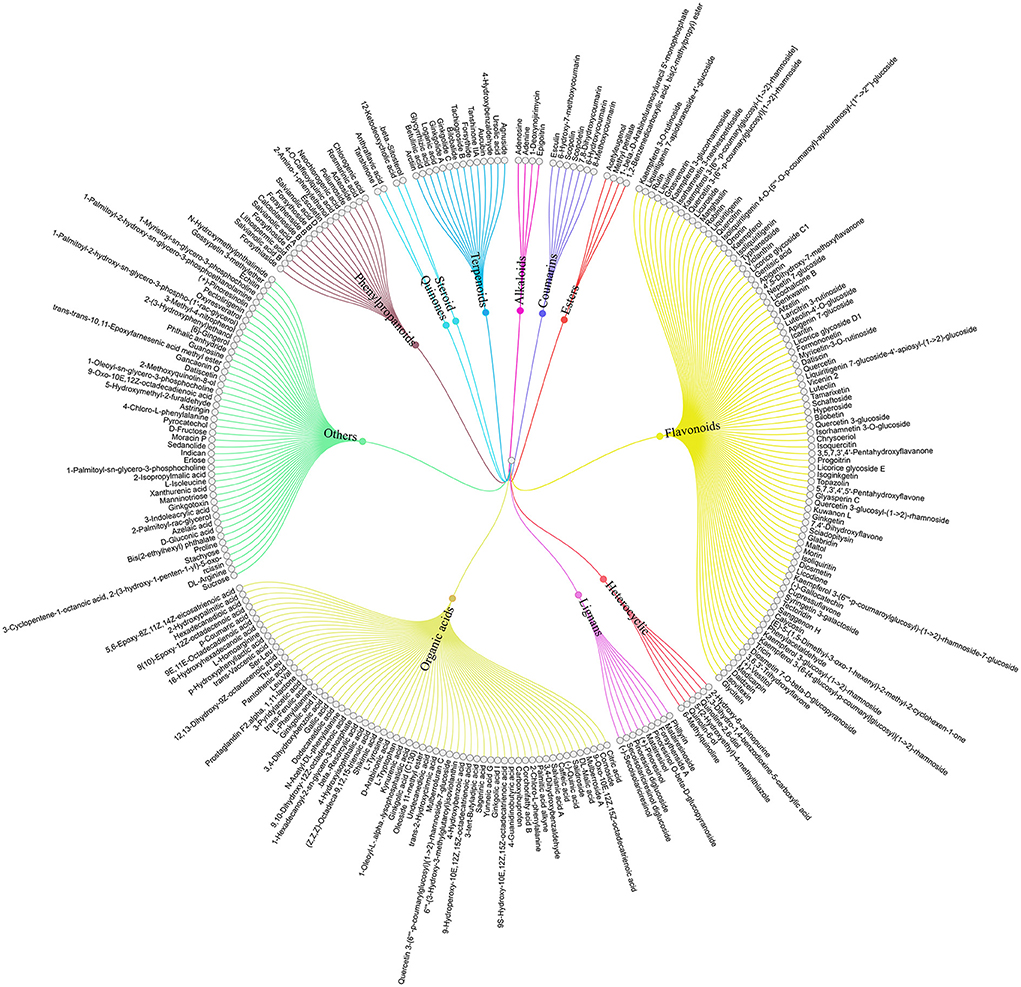
Figure 3. Tree diagram of NCMC aqueous extraction classification. Total 260 compounds belong to 12 categories represented by different colors.
Pharmacodynamics' evaluation of NCMC
The chickens in co-infection group compared with the other three groups had severe air sac lesions with diffuse yellowish thickening with caseous exudates, and the control group had no significant changes. There was no significant difference between A and C for the gross air sac lesion score. The same trend was observed for the tracheal lesion scores in the four groups (Figure 4). Pathological and ultrastructural changes (Figure 5) were performed to better understand the effects of NCMC treatment on the chicken trachea. In the co-infection group (Figure 5A), the cilia are exfoliated and there is a proliferation of goblet cells in the upper mucosa. While the cilia are well arranged and there is still a small amount of inflammatory cells infiltrations after NCMC treatment (Figure 5B). Scanning electron microscopy (SEM) showed that the cilia were significantly more abundant and intact after treatment of co-infection (Figures 5C,D). TEM examination showed that cilia were ruptured, matrix electron density decreased, and the formation of umbrella-like structure to the extracellular in the co-infection group (Figure 5E). Although some cilia were shed, the symptoms of the treatment group were significantly improved compared with the co-infection group (Figure 5F).
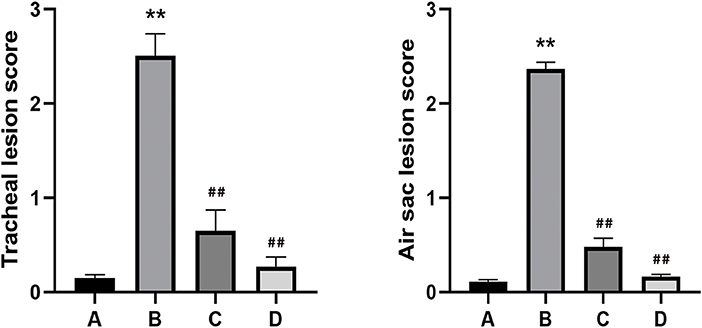
Figure 4. Gross and tracheal lesion evaluation of all groups, (A) Control group. (B) Co-infection group. (C) Co-infection + NCMC administration group. (D) NCMC control group. Bar graphs represent mean results ± SD. Statistical significance were represented as **P < 0.05 vs. control group, ##P < 0.05 vs. model group. Error bars represent the standard error of the mean.
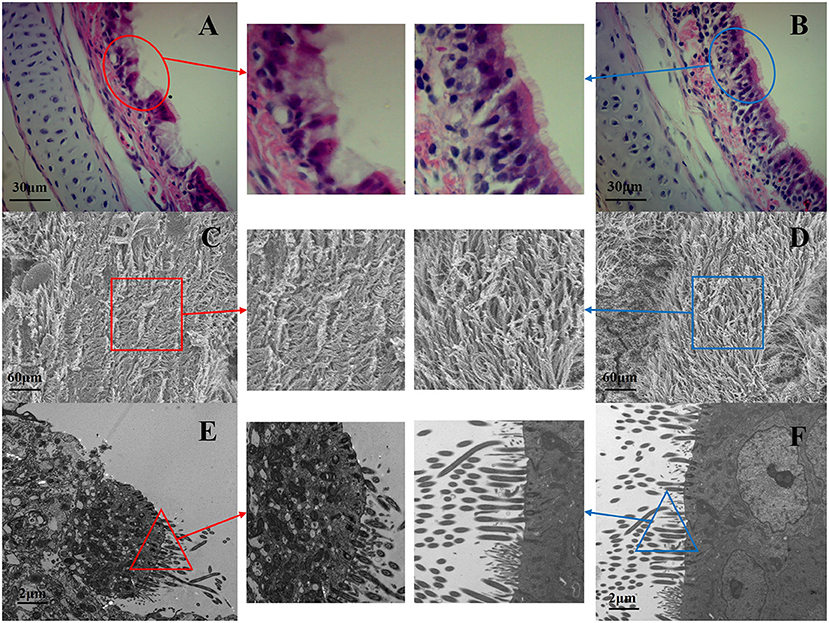
Figure 5. Pathological and ultrastructural changes of NCMC treatment on chicken trachea, (A,C,E from the co-infection group; B,D,F from the NCMC treatment group). Paraffin sections of tracheal tissues from co-infection group and co-infection + NCMC group were stained with hematoxylin-eosin (100×). (A) The red circle shows the cilia are exfoliated and there is a proliferation of goblet cells. (B) The blue circle indicates that the cilia are well arranged and only a small amount of inflammatory cell infiltration. SEM of (C,D) at a magnification of 2,500×, and TEM of (E,F) at a magnification of 8,000×. (D) The cilia in blue square significantly more abundant and intact after treatment of co-infection (C). (E) The cilia were ruptured, matrix electron density decreased in red triangle of co-infection group. (F) The blue triangle shows some cilia were shed, but the symptoms of the treatment group were significantly improved.
Discussion
In this study, we conducted multi-target screening for co-infection of MG and E. coli based on omics research and investigated the polypharmacology mechanism of NCMC with multiple components. The whole research was based on the establishment of a co-infection model and the subsequent target screening. According to the previous transcriptome studies on co-infection (23), we obtained a total of 3,115 DEGs and the corresponding KEGG classification. As the previous study showed that oxidative stress transcriptomics and drug discovery approaches could identify and target neurotoxic innate immune populations and lead to the development of selective neuroprotective strategies (37, 38). However, we hope to screen the representative DEGs from the overall level of in vivo studies. Hence, we have carried out the DEGs from 33 sub-categories of KEGG have carried out PPI analysis, respectively, as explained in previous studies (39–41). The screened target genes cannot be applied directly but are matched to the corresponding target according to the Information in the DrugBank database.
Hopkins proposed the concept of network pharmacology in 2007, which aims to design a new generation of drugs by incorporating biological networks rather than single target (42). Network pharmacology-based strategy predicted liver injury targets of matrine against COVID-19 and further confirmed Matrine can maintain liver function homeostasis, regulate immunity and antivirus through direct target action and signal pathway regulation in vivo (43). For complex diseases, the method of applying network pharmacology was also used to reveal the mechanism of action of TCM (44, 45). We collected the whole data of TCMSP for the construction of the total network “TCM-component-target”, and then the targets were substituted into the total network for the reverse screening of TCMs. This method of reverse operation is similar to existing relevant studies (46, 47). Our study combined the usual methods of network pharmacology in the past, starting from the complex diseases in clinical practice, and re-screening TCM compounds through the study of natural products. However, this is the first attempt to apply the reverse screening method directly to the TCM network.
After obtaining the order of Chinese herbs, we then selected different categories based on the compatibility theory of Chinese medicines. According to the degree of node correlation, the order was as follows: Isatdis Radix (4th, yang tonic) (48), Forsythia Fructus (11th, Antipyretic and antidote) (49), Ginkgo Folium (5th, Antitussive and antiasthmatic drugs) (50), Mori Cortex (3rd, Antitussive and antiasthmatic drugs) (51), Licorice (1st, reinforcing drugs) (52), and Radix Salviae (2nd, Drugs with the efficacy of modifying rheological properties of blood) (53). Aqueous extract of Chinese medicinal compound is the most common method to study compound components (54, 55). Hence, we explored the optimization design studies (56, 57). In preliminary experiments, a multi-nonlinear regression analysis was used for searching the optimal combination based on the minimal inhibitory concentration of MG and E. coli in vitro, air sac and tracheal lesion scores in vivo. Then, the different proportion combinations were further optimized through uniform design. Finally, we obtained the optimal combination of NCMC aqueous extract for subsequent targeted therapy experiments. In the treatment experiment of NCMC aqueous extract for co-infection, multiple pathological methods were employed to observe whether a certain curative effect was achieved. Pathological and ultrastructural results showed that the symptoms of co-infection were significantly relieved after treatment, which indicated that NCMC played a significant therapeutic role.
In summary, the target network for the co-infection was dug through the joint analysis of transcriptomics and metabolomics, and the mapping between the target network and the total TCM network was used to reverse screen TCMs. We started from the network and carried out targeted verification from multiple dimensions to preliminarily reveal the polypharmacological effects of NCMC. These data suggest that we may provide a new method to validate target combinations of natural products, which perhaps to optimize their multiple structure-activity relationships to obtain drug-like natural product derivatives. The research on the product of new TCM formula provides direction and guidance for the development of drugs for respiratory diseases in veterinary clinic.
Data availability statement
The original contributions presented in the study are included in the article/Supplementary material, further inquiries can be directed to the corresponding authors.
Ethics statement
The animal study was reviewed and approved by Laboratory Animal Ethics Committee of Northeast Agricultural University.
Author contributions
ZWu and JL designed the study. JB, YW, SW, DN, ZWu, YZ, and RL performed and collected data from experiment and analyzed data. ZWu and MI wrote the manuscript. All authors read and approved the final manuscript.
Funding
This work was supported by the National Natural Science Foundation of China (32273062) and Special Funding for Postdoctoral of Heilongjiang Province (LBH-TZ2119).
Acknowledgments
We would like to thank Professor Ming Ge of the Department of Clinical Veterinary Medicine (Northeast Agricultural University) in our university for his great assistance in screening TCMs. We appreciate Professor Guohua Zhang (Southern Medical University, China) for sharing the whole TCMSP database and providing corresponding guidance. We also thank the Biotree Biotech Co., Ltd. (Shanghai, China) for metabolomics analysis and data interpretation.
Conflict of interest
The authors declare that the research was conducted in the absence of any commercial or financial relationships that could be construed as a potential conflict of interest.
Publisher's note
All claims expressed in this article are solely those of the authors and do not necessarily represent those of their affiliated organizations, or those of the publisher, the editors and the reviewers. Any product that may be evaluated in this article, or claim that may be made by its manufacturer, is not guaranteed or endorsed by the publisher.
Supplementary material
The Supplementary Material for this article can be found online at: https://www.frontiersin.org/articles/10.3389/fvets.2022.972245/full#supplementary-material
References
1. Anighoro A, Bajorath J, Rastelli G. Polypharmacology: challenges and opportunities in drug discovery. J Med Chem. (2014) 57:7874–87. doi: 10.1021/jm5006463
2. Kuenzi BM, Remsing Rix LL, Stewart PA, Fang B, Kinose F, Bryant AT, et al. Polypharmacology-based ceritinib repurposing using integrated functional proteomics. Nat Chem Biol. (2017) 13:1222–31. doi: 10.1038/nchembio.2489
3. Ho TT, Tran QT, Chai CL. The polypharmacology of natural products. Future Med Chem. (2018) 10:1361–8. doi: 10.4155/fmc-2017-0294
4. Fang J, Liu C, Wang Q, Lin P, Cheng F. In silico polypharmacology of natural products. Brief Bioinform. (2018) 19:1153–71. doi: 10.1093/bib/bbx045
5. Ishfaq M, Zhang W, Liu Y, Wang J, Wu Z, Shah SW, et al. Baicalin attenuated Mycoplasma gallisepticum induced immune impairment in the chicken Bursa of fabricius through modulation of autophagy and inhibited inflammation and apoptosis. J Sci Food Agricul. (2021) 101:880–90. doi: 10.1002/jsfa.10695
6. Wang J, Ishfaq M, Li J. Baicalin ameliorates Mycoplasma gallisepticum-induced inflammatory injury in the chicken lung through regulating the intestinal microbiota and phenylalanine metabolism. Food Funct. (2021) 12:4092–104. doi: 10.1039/d1fo00055a
7. Liu LJ, Leung KH, Chan DS, Wang YT, Ma DL, Leung CH. Identification of a natural product-like STAT3 dimerization inhibitor by structure-based virtual screening. Cell Death Dis. (2014) 5:e1293. doi: 10.1038/cddis.2014.250
8. Zhong HJ, Lee BR, Boyle JW, Wang W, Ma DL, Hong Chan PW, et al. Structure-based screening and optimization of cytisine derivatives as inhibitors of the menin-MLL interaction. Chem Commun. (2016) 52:5788–91. doi: 10.1039/C6CC01079B
9. Cheng F, Liu C, Jiang J, Lu W, Li W, Liu G, et al. Prediction of drug-target interactions and drug repositioning via network-based inference. PLoS Comput Biol. (2012) 8:e1002503. doi: 10.1371/journal.pcbi.1002503
10. Wu Z, Lu W, Wu D, Luo A, Bian H, Li J, et al. In silico prediction of chemical mechanism of action via an improved network-based inference method. Br J Pharmacol. (2016) 173:3372–85. doi: 10.1111/bph.13629
11. Birkenstock T, Liebeke M, Winstel V, Krismer B, Gekeler C, Niemiec MJ, et al. Exometabolome analysis identifies pyruvate dehydrogenase as a target for the antibiotic triphenylbismuthdichloride in multiresistant bacterial pathogens. J Biol Chem. (2012) 287:2887–95. doi: 10.1074/jbc.M111.288894
12. Hieronymus H, Lamb J, Ross KN, Peng XP, Clement C, Rodina A, et al. Gene expression signature-based chemical genomic prediction identifies a novel class of HSP90 pathway modulators. Cancer Cell. (2006) 10:321–30. doi: 10.1016/j.ccr.2006.09.005
13. Savitski MM, Reinhard FB, Franken H, Werner T, Savitski MF, Eberhard D, et al. Tracking cancer drugs in living cells by thermal profiling of the proteome. Science. (2014) 346:1255784. doi: 10.1126/science.1255784
14. Noori HR, Spanagel R. In silico pharmacology: drug design and discovery's gate to the future. In Silico Pharmacol. (2013) 1:1. doi: 10.1186/2193-9616-1-1
15. Jafari M, Wang Y, Amiryousefi A, Tang J. Unsupervised learning and multipartite network models: a promising approach for understanding traditional medicine. Front Pharmacol. (2020) 11:1319. doi: 10.3389/fphar.2020.01319
16. Wang Y, Yang H, Chen L, Jafari M, Tang J. Network-based modeling of herb combinations in traditional Chinese medicine. Brief Bioinform. (2021) 22:bbab106. doi: 10.1093/bib/bbab106
17. Pan L, Li Z, Wang Y, Zhang B, Liu G, Liu J. Network pharmacology and metabolomics study on the intervention of traditional Chinese medicine Huanglian Decoction in rats with type 2 diabetes mellitus. J Ethnopharmacol. (2020) 258:112842. doi: 10.1016/j.jep.2020.112842
18. Zhou W, Cheng X, Zhang Y. Effect of Liuwei Dihuang decoction, a traditional Chinese medicinal prescription, on the neuroendocrine immunomodulation network. Pharmacol Ther. (2016) 162:170–8. doi: 10.1016/j.pharmthera.2016.02.004
19. Hu W, Zhang W, Shah S, Ishfaq M, Developmental JLJ, Immunology C. Mycoplasma gallisepticum infection triggered histopathological changes, oxidative stress and apoptosis in chicken thymus and spleen. Dev Comp Immunaol. (2020) 114:103832. doi: 10.1016/j.dci.2020.103832
20. Wu Z, Chen C, Zhang Q, Bao J, Fan Q, Li R, et al. Arachidonic acid metabolism is elevated in Mycoplasma gallisepticum and Escherichia coli co-infection and induces LTC4 in serum as the biomarker for detecting poultry respiratory disease. Virulence. (2020) 11:730–8. doi: 10.1080/21505594.2020.1772653
21. Umar S, Delverdier M, Delpont M, Belkasmi SFZ, Teillaud A, Bleuart C, et al. Co-infection of turkeys with Escherichia coli (O78) and H6N1 avian influenza virus. Avian Pathol. (2018) 47:314–24. doi: 10.1080/03079457.2018.1449942
22. Yan T, Zhu S, Wang H, Li C, Diao Y, Tang Y. Synergistic pathogenicity in sequential coinfection with fowl adenovirus type 4 and avian orthoreovirus. Vet Microbiol. (2020) 251:108880. doi: 10.1016/j.vetmic.2020.108880
23. Wu Z, Ding L, Bao J, Liu Y, Zhang Q, Wang J, et al. Co-infection of Mycoplasma gallisepticum and Escherichia coli triggers inflammatory injury involving the IL-17 signaling pathway. Front Microbiol. (2019) 10:2615. doi: 10.3389/fmicb.2019.02615
24. Ji HY, Liu KH, Kong TY, Jeong HU, Choi SZ, Son M, et al. Evaluation of DA-9801, a new herbal drug for diabetic neuropathy, on metabolism-mediated interaction. Arch Pharm Res. (2013) 36:1–5. doi: 10.1007/s12272-013-0014-9
25. Langeder J, Grienke U, Chen Y, Kirchmair J, Schmidtke M, Rollinger JM. Natural products against acute respiratory infections: strategies and lessons learned. J Ethnopharmacol. (2020) 248:112298. doi: 10.1016/j.jep.2019.112298
26. Wang J, Ishfaq M, Li J. Lactobacillus salivarius ameliorated Mycoplasma gallisepticum-induced inflammatory injury and secondary Escherichia coli infection in chickens: involvement of intestinal microbiota. Vet Immunol Immunopathol. (2021) 233:110192. doi: 10.1016/j.vetimm.2021.110192
27. Wang Y, Chen S, Yu O. Metabolic engineering of flavonoids in plants and microorganisms. Appl Microbiol Biotechnol. (2011) 91:949–56. doi: 10.1007/s00253-011-3449-2
28. Ishfaq M, Chen C, Bao J, Zhang W, Wu Z, Wang J, et al. Baicalin ameliorates oxidative stress and apoptosis by restoring mitochondrial dynamics in the spleen of chickens via the opposite modulation of NF-kappaB and Nrf2/HO-1 signaling pathway during Mycoplasma gallisepticum infection. Poult Sci. (2019) 98:6296–310. doi: 10.3382/ps/pez406
29. Langmead B, Salzberg SL. Fast gapped-read alignment with Bowtie 2. Nat Methods. (2012) 9:357–9. doi: 10.1038/nmeth.1923
30. Wang L, Feng Z, Wang X, Wang X, Zhang X. DEGseq: an R package for identifying differentially expressed genes from RNA-seq data. Bioinformatics. (2010) 26:136–8. doi: 10.1093/bioinformatics/btp612
31. Hernández-Salmerón JE, Moreno-Hagelsieb G. Progress in quickly finding orthologs as reciprocal best hits: comparing blast, last, diamond and MMseqs2. BMC Genomics. (2020) 21:741. doi: 10.1186/s12864-020-07132-6
32. Kou J, Zhu D, Yan Y. Neuroprotective effects of the aqueous extract of the Chinese medicine Danggui-Shaoyao-san on aged mice. J Ethnopharmacol. (2005) 97:313–8. doi: 10.1016/j.jep.2004.11.020
33. Yin JB, Zhou KC, Wu HH, Hu W, Ding T, Zhang T, et al. Analgesic effects of danggui-shaoyao-san on various “Phenotypes” of nociception and inflammation in a formalin pain model. Mol Neurobiol. (2016) 53:6835–48. doi: 10.1007/s12035-015-9606-3
34. Li J, Li M, Ye K, Jiang Q, Wang M, Wen X, et al. Chemical profile of Xian-He-Cao-Chang-Yan formula and its effects on ulcerative colitis. J Ethnopharmacol. (2021) 267:113517. doi: 10.1016/j.jep.2020.113517
35. Li Y, Guo M, Li X, Yang XJM. Simultaneous qualitative and quantitative evaluation of the coptidis rhizoma and euodiae fructus herbal pair by using UHPLC-ESI-QTOF-MS and UHPLC-DAD. Molecules. (2020) 25:4782. doi: 10.3390/molecules25204782
36. Bao J, Wu Z, Ishfaq M, Miao Y, Li R, Clifton AC, et al. Comparison of experimental infection of normal and immunosuppressed chickens with Mycoplasma gallisepticum. J Comp Pathol. (2020) 175:5–12. doi: 10.1016/j.jcpa.2019.12.001
37. Mendiola AS, Ryu JK, Bardehle S, Meyer-Franke A, Ang KK, Wilson C, et al. Transcriptional profiling and therapeutic targeting of oxidative stress in neuroinflammation. Nat Immunol. (2020) 21:513–24. doi: 10.1038/s41590-020-0654-0
38. Zhang W, Liu Y, Zhang Q, Shah S, Li J. Mycoplasma gallisepticum infection impaired the structural integrity and immune function of bursa of fabricius in chicken: implication of oxidative stress and apoptosis. Front Vet Sci. (2020) 7:225. doi: 10.3389/fvets.2020.00225
39. Ji R, Chen Y, Chen W, Wang Y, Gong F, Huang S, et al. Identification of significant genes and pathways in acute pancreatitis via bioinformatical analysis. Dig Dis Sci. (2021) 66:3045–53. doi: 10.1007/s10620-020-06598-4
40. Liu C, Liu Y, Liang L, Cui S, Zhang Y. RNA-Seq based transcriptome analysis during bovine viral diarrhoea virus (BVDV) infection. BMC Genomics. (2019) 20:774. doi: 10.1186/s12864-019-6120-4
41. Ren Y, Li J, Guo L, Liu JN, Wan H, Meng Q, et al. Full-length transcriptome and long non-coding RNA profiling of whiteleg shrimp Penaeus vannamei hemocytes in response to Spiroplasma eriocheiris infection. Fish Shellfish Immunol. (2020) 106:876–86. doi: 10.1016/j.fsi.2020.06.057
43. Liu F, Li Y, Yang Y, Li M, Du Y, Zhang Y, et al. Study on mechanism of matrine in treatment of COVID-19 combined with liver injury by network pharmacology and molecular docking technology. Drug Deliv. (2021) 28:325–42. doi: 10.1080/10717544.2021.1879313
44. Pan X, Liu Z. Total synthesis and antibacterial activity evaluation of griseofamine A and 16- epi-griseofamine A. Org Lett. (2019) 21:2393–6. doi: 10.1021/acs.orglett.9b00672
45. Wang E, Wang L, Ding R, Zhai M, Ge R, Zhou P, et al. Astragaloside IV acts through multi-scale mechanisms to effectively reduce diabetic nephropathy. Pharmacol Res. (2020) 157:104831. doi: 10.1016/j.phrs.2020.104831
46. Hou J, Chen W, Lu H, Zhao H, Gao S, Liu W, et al. Exploring the therapeutic mechanism of desmodium styracifolium on oxalate crystal-induced kidney injuries using comprehensive approaches based on proteomics and network pharmacology. Front Pharmacol. (2018) 9:620. doi: 10.3389/fphar.2018.00620
47. Lauro G, Romano A, Riccio R, Bifulco G. Inverse virtual screening of antitumor targets: pilot study on a small database of natural bioactive compounds. J Nat Prod. (2011) 74:1401–7. doi: 10.1021/np100935s
48. Chan HL, Yip HY, Mak NK, Leung KN. Modulatory effects and action mechanisms of tryptanthrin on murine myeloid leukemia cells. Cell Mol Immunol. (2009) 6:335–42. doi: 10.1038/cmi.2009.44
49. Jiao J, Ma DH, Gai QY, Wang W, Luo M, Fu YJ, et al. Rapid analysis of Fructus forsythiae essential oil by ionic liquids-assisted microwave distillation coupled with headspace single-drop microextraction followed by gas chromatography-mass spectrometry. Anal Chim Acta. (2013) 804:143–50. doi: 10.1016/j.aca.2013.10.035
50. Lou JS, Xia YT, Wang HY, Kong XP, Yao P, Dong TTX, et al. The WT1/MVP-mediated stabilization on mTOR/AKT axis enhances the effects of cisplatin in non-small cell lung cancer by a reformulated yu ping feng san herbal preparation. Front Pharmacol. (2018) 9:853. doi: 10.3389/fphar.2018.00853
51. Hou XD, Ge GB, Weng ZM, Dai ZR, Leng YH, Ding LL, et al. Natural constituents from Cortex Mori Radicis as new pancreatic lipase inhibitors. Bioorg Chem. (2018) 80:577–84. doi: 10.1016/j.bioorg.2018.07.011
52. Yu Y, Pauli GF, Huang L, Gan LS, van Breemen RB, Li D, et al. Classification of flavonoid metabolomes via data mining and quantification of hydroxyl NMR signals. Anal Chem. (2020) 92:4954–62. doi: 10.1021/acs.analchem.9b05084
53. Kai G, Hao X, Cui L, Ni X, Zekria D, Wu J. WITHDRAWN: metabolic engineering and biotechnological approaches for production of bioactive diterpene tanshinones in Salvia miltiorrhiza. Biotechnol Adv. (2014). doi: 10.1016/j.biotechadv.2014.10.001. [Epub ahead of print].
54. Duan X, Pan L, Bao Q, Peng D. UPLC-Q-TOF-MS study of the mechanism of THSWD for breast cancer treatment. Front Pharmacol. (2019) 10:1625. doi: 10.3389/fphar.2019.01625
55. Yang J, Zhu A, Xiao S, Zhang T, Wang L, Wang Q, et al. Anthraquinones in the aqueous extract of Cassiae semen cause liver injury in rats through lipid metabolism disorder. Phytomedicine. (2019) 64:153059. doi: 10.1016/j.phymed.2019.153059
56. Fang KT, Lin DKJ, Winker P, Zhang Y. Uniform design: theory and application. Technometrics. (2000) 42:237–48. doi: 10.1080/00401706.2000.10486045
Keywords: polypharmacology, Traditional Chinese Medicine, transcriptomics, multiple targets, network pharmacology
Citation: Bao J, Wang Y, Wang S, Niu D, Wang Z, Li R, Zheng Y, Ishfaq M, Wu Z and Li J (2022) Polypharmacology-based approach for screening TCM against coinfection of Mycoplasma gallisepticum and Escherichia coli. Front. Vet. Sci. 9:972245. doi: 10.3389/fvets.2022.972245
Received: 25 June 2022; Accepted: 05 September 2022;
Published: 26 September 2022.
Edited by:
Nicola Pugliese, University of Bari Aldo Moro, ItalyReviewed by:
Hazim Khalifa, Kafrelsheikh University, EgyptJing Tang, University of Helsinki, Finland
Hongxu Du, Southwest University, China
Copyright © 2022 Bao, Wang, Wang, Niu, Wang, Li, Zheng, Ishfaq, Wu and Li. This is an open-access article distributed under the terms of the Creative Commons Attribution License (CC BY). The use, distribution or reproduction in other forums is permitted, provided the original author(s) and the copyright owner(s) are credited and that the original publication in this journal is cited, in accordance with accepted academic practice. No use, distribution or reproduction is permitted which does not comply with these terms.
*Correspondence: Zhiyong Wu, d3V6aGl5b25nQG5lYXUuZWR1LmNu; Jichang Li, bGlqaWNoYW5nQG5lYXUuZWR1LmNu