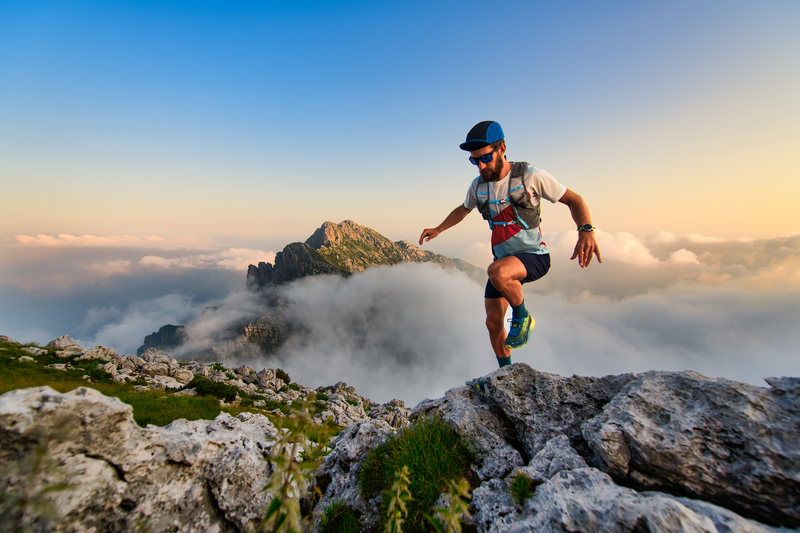
94% of researchers rate our articles as excellent or good
Learn more about the work of our research integrity team to safeguard the quality of each article we publish.
Find out more
MINI REVIEW article
Front. Vet. Sci. , 23 May 2022
Sec. Veterinary Neurology and Neurosurgery
Volume 9 - 2022 | https://doi.org/10.3389/fvets.2022.896030
This article is part of the Research Topic Managing Dog and Owner Health in Canine Epilepsy View all 5 articles
The unpredictable nature of seizures is challenging for caregivers of epileptic dogs, which calls the need for other management strategies such as seizure detection devices. Seizure detection devices are systems that rely on non-electroencephalographic (non-EEG) ictal changes, designed to detect seizures. The aim for its use in dogs would be to provide owners with a more complete history of their dog's seizures and to help install prompt (and potentially life-saving) intervention. Although seizure detection via wearable intracranial EEG recordings is associated with a higher sensitivity in humans, there is robust evidence for reliable detection of generalized tonic-clonic seizures (GTCS) using non-EEG devices. Promising non-EEG changes described in epileptic humans, include heart rate variability (HRV), accelerometry (ACM), electrodermal activity (EDA), and electromyography (EMG). Their sensitivity and false detection rate to detect seizures vary, however direct comparison of studies is nearly impossible, as there are many differences in study design and standards for testing. A way to improve sensitivity and decrease false-positive alarms is to combine the different parameters thereby profiting from the strengths of each one. Given the challenges of using EEG in veterinary clinical practice, non-EEG ictal changes could be a promising alternative to monitor seizures more objectively. This review summarizes various seizure detection devices described in the human literature, discusses their potential use and limitations in veterinary medicine and describes what is currently known in the veterinary literature.
A reduction in seizures is typically used as the outcome measure in research papers in canine epilepsy largely focussing on anticonvulsant medication (1–4). However, there is increasing recognition that other factors influence outcome such as adverse effects of medication and the effect that caring for a dog with epilepsy has on the owner. Several studies have investigated the quality of life in dogs with idiopathic epilepsy and owners' perspective on long-term management (5–9). Interestingly, around 50% of dog owners reported that their dog's seizures affected the ability to leave their dog unsupervised, afraid to miss a seizure (7) and 82% of dog owners, are reported to keep track of their dog's seizure activity (9). Although the gold standard for identifying seizures is the detection of ictal or inter-ictal electroencephalographic (EEG) abnormalities (10), this is impractical for long-term and real-life setting use (11). Seizure detection is currently done by owners' visual recognition. However, accurate recognition and recording of seizures has proven to be unreliable in people as half of the seizures recording during video-EEG are not known to the patient (12). This discrepancy between seizure recognition and seizure occurrence is also suspected to be true in dogs (13). Hence, there is a need to investigate other strategies in order to reliably measure response to treatment but also to improve the management of canine epilepsy for owners which in turn improves dog-owner connection. Wearable technology for dogs populates today's market with those that are commercially available mainly used for tracking location, however some claim use also for medical diagnosis and treatment (14).
Studies on wearable devices to detect seizures are extensively available in the human literature. These devices are based on detecting physiological changes before or during a seizure such as alterations in movement, heart rate and electrical activity in muscles (15). Considering the increasing interest and number of publications on these devices in human medicine, it would be interesting to investigate whether this could be translated to dogs. This review summarizes various seizure detection devices described in the humane literature, discusses their potential use and limitations in veterinary medicine and describes what is currently known in the veterinary literature.
Most investigated parameters include autonomic changes (cardiovascular, respiratory, and transpiration) and changes in movement (accelerometry, surface electromyography) (9). The devices this review will focus on, include heart rate (ECG), electrodermal activity (EDA), accelerometry (ACM), and surface electromyography (sEMG) (Table 1). The false alarm rate (FAR) of a device refers to the average number of times that the detector incorrectly declared the onset of seizures in a 24-h period (10). Should only a small proportion of the alarms be relevant to the caregiver (i.e., high false alarm rate), then the caregiver may stop responding to alarms, a phenomenon called “alarm fatigue.” In these cases, alarm fatigue may defeat the purpose of the device (11).
Table 1. ACM, accelerometer; CS, clonic seizure; ECG, electrocardiography; EDA, electrodermal activity; EMG, electromyography; FS, focal seizures; GTCS, generalized tonic–clonic seizure; HR, heart rate; NA, not available; Spo2, arterial oxygenation; TS, tonic seizure.
Cardiac changes have been most extensively investigated before, during and after seizures as an extracerebral physiological parameter (12, 13). Cardiovascular changes are particularly important as they are linked to sudden unexplained death in epilepsy (SUDEP) in humans (15). Heart rate changes include tachycardia, bradycardia, and asystole. These changes are most prominent in generalized tonic-clonic type seizures, but unlike devices measuring movement, they can also detect non-convulsive seizures (16). Tachycardia is most consistently recorded during epileptic seizures and can be explained by an increased motor activity, release of catecholamines, sympathetic and parasympathetic shifts, activation of limbic structures, increased neuronal firing, or a combination of these and other unknown factors (17). Ictal bradycardia is less common and is more frequently associated with focal seizures (18, 19). Given the variable autonomic nervous system changes, heart rate variability (HRV) is preferred for the use of detecting seizures. In addition, there is also individual variation reflecting the unique individual spread and evolution of seizure activity (20, 21). This warrants the development of a patient-specific detection algorithm for HRV, and several proposals have been brought forward (8, 22). Most studies measuring HRV are retrospective validation studies (23–25). Sensitivity rates of 81.3–96.1% have been described, and a higher sensitivity has been associated with higher false alarms rate (up to 5.4/h). A prospective validation study was published recently which used a predefined detection algorithm based on heart HRV using patient-specific cut-off values. Responders were defined as patients who had a >50 beats/min ictal change in heart rate. The algorithm detected 9 out of 10 convulsive seizures with a false alarm rate of 0.9/24 h (0.22/night) (26). The study design, including video-EEG as long-term monitoring, and results are extremely promising and may form footing for further large-scale multicentre validation studies.
There are no veterinary studies investigating heart rate changes during the ictal phase. One study analyzed interictal HRV in presumed idiopathic epileptic dogs in comparison with non-epileptic dogs. Their findings revealed that dogs with idiopathic epilepsy were associated with an increased P wave dispersion QT interval, suggesting these dogs have cardiac electrical abnormalities similar to conductibility delays of the electric impulse observed in dogs with primary heart disease or electrolyte imbalances (27). Although these results cannot be directly used to detect seizures, the findings are interesting as such abnormalities are considered markers for severe arrhythmias associated with SUDEP in people (28).
Electrodermal activity (EDA), also known as skin conductance, reflects the activity of the sympathetic nerve on sweat glands (29). Epileptic seizures have shown to transiently increase EDA (30). This can be explained by the increased conductance of an applied current (such as by an EDA device) by sweat. Only a few studies have investigated EDA during the ictal and post-ictal phase. Their results showed that epileptic seizures induced a decrease in skin resistance by sweating. The epileptic seizures induce a surge in EDA and these changes are more prominent in GTCS which reflects a massive sympathetic discharge. The first long-term, video-EEG controlled study including seven patients, found that in GTCS, EDA increased in all (100%) patients by over 20 μS. In addition, EDA also remained significantly elevated for a longer time during GTCS compared to other types of seizures (31). The disadvantages of the use of EDA are the susceptibility to motion and pressure artifacts (32). Therefore, EDA used alone is associated with a high FAR and EDA is now increasingly used in combination with other non-EEG changes such as ACM (16).
Electrodermal activity has been used in a veterinary study to assess postoperative orthopedic pain (33). The product used in this study is currently off the market and there are no other studies investigating its use for detecting seizures. Also, sweat glands that actively participate in central thermoregulation in dogs are limited to the merocrine glands in the footpads (34). This is impractical for home use but could be considered in a hospital setting.
Accelerometry (ACM) is the rate of change of velocity of the body in its own rest frame. It can be used to detect changes in velocity during a GTCS. Recent advances in technology have resulted in the development of ambulatory devices which are usually small, portable and easy to use (35, 36). An accelerometer has been proven to be able to detect a variety of seizures including focal seizures, GTCS and myoclonic, clonic, tonic, and hypermotor seizures (37). Initial studies used healthy subjects simulating a motor seizure, but this has evolved, and most studies nowadays use clinical subjects, a predefined or even patient-specific algorithm and video-EEG as long-term monitoring. More than a dozen studies have used accelerometers and most of them have implemented the ACM in a wrist-worn device (38). Three well-known commercially available human wrist-worn devices are the EpiWatch®, the SmartWatch®, and the Embrace®. The median sensitivity of the EpiWatch (Epi-Care, Danish Technology, Denmark) investigated by two studies was found to be 90% with a FAR of 0.1/day. The first study used video-EEG as seizure monitoring and the second study relied on seizure count by patient or caregiver as part of a field study (39, 40). The results of the SmartWatch were disappointing as they found a sensitivity of only 31% for detecting seizures using their device (SmartWatch, California, USA). This was a large prospective study using video-EEG as seizure monitoring and false alarms were not recorded in this study (41). The third device (Embrace, Boston, USA) was tested via a smaller, video-EEG controlled, prospective study. Sensitivity was 95% with a FAR of 0.7/day (42). Although sensitivity was high, there was noticeable inter-patient variation, and the sensitivity and specificity are dependent on the algorithm that was used for that patient.
Another recent wrist-worn device was developed within a non-commercial platform, and it evaluated three classification algorithms comparing them with video-EEG. The algorithm with the highest sensitivity for measuring tonic-clonic seizures was 100% with a FAR of 1.2/day and the algorithm with the lowest false positive rate had a sensitivity of 90% with a FAR 0.24/day (43), illustrating the influence of sensitivity on FAR. Non-convulsive movements were challenging to detect and detection also depends on the movement of the limb to which the device is attached. The use of the lower arm is recommended in humans, especially for capturing motor seizures (44). As a result, there is a risk to both over-and under detect seizures and future studies will focus on specific algorithms to increase sensitivity and specificity (16).
Similar problems have been encountered in veterinary medicine. A prospective single center study was performed to assess the accuracy of a collar-mounted accelerometer in dogs to detect seizure activity. The study consisted of two phases; a predefined algorithm was used to detect seizures during the first study phase, and an individualized algorithm was subsequently used during the second study phase. Seizures were manually recorded by owners. Both predefined and individualized algorithms had low sensitivities (18.6 and 22.1% respectively) to detect seizures with a low FAR (0.096/day and 0.054/day respectively). Reasons for these disappointing results could include the position of the device and the algorithms used in the study. The neck may not present such vigorous rhythmic movements during generalized tonic-clonic seizures, as seen for example in the limbs (45). However, long-term use of a device secured to the limb seems impractical in a dog with increased risk of device damage or ingestion.
Surface electromyography (sEMG) records muscle activity with as little as one channel and has emerged as a promising modality for detecting motor seizures in people (17, 46, 47). EMG signals provide direct information about electrical activity in the motor cortex as muscles are in direct synaptic contact with motor neurons. The mechanism of muscle activation has shown to differ between convulsive epileptic seizures and voluntary muscle activation as they show different electrographic patterns (16). The amplitude was for example found to be higher in patients with GTCS compared with patients with psychogenic non-epileptic seizures (PNES) and epileptic seizures had a larger duration in EMG activity (46). A recent prospective, video-EEG controlled, multicentre and blinded study used a relatively small device which could be worn under normal clothing and the device was attached by a self-adhesive hypoallergenic hydrogel patch. They found a high sensitivity (93.8%), short detection latency (9 s) and low number of false alarms (0.67/d) (47). Long-term recording of surface EMG activity is technically easy to perform, however disadvantages often are discomfort or skin irritation caused by the electrode patches (48). sEMG is an effective modality for the detection of seizures with a motor component but false negatives still occur, often triggered by common movements such as physical exercise (16).
Surface electromyography has been frequently used in veterinary medicine for analyzing muscle activity patterns during walking (49–52). As with EDA, no studies in veterinary medicine have focussed on the use of sEMG for detecting seizures. A possible advantage could be that the device can be placed almost anywhere on the body, for example the neck. A likely major disadvantage is the artifact of movement.
A more recent development in human medicine is using a multimodal approach for detecting GTCS (38). Several wrist-worn devices have been developed combining non-EEG parameters. Results show that combining parameters improves sensitivity and lowers false-positive alarms by profiting from the strengths of each individual parameter (Table 1).
Most of these studies used accelerometers in combination with other parameters. A wristband measuring three-axis accelerometers and electrodermal activity yielded a high sensitivity (>92%) with a FAR of 0.2–1/day (42). Sensitivity and FAR improved during rest, still indicating the interference of non-convulsive movements. Another study found a sensitivity of 91% and a FAR of 0.2/day but was limited to nocturnal seizures only, which reduces the involvement of non-convulsive movements (53). Most recent studies are focused on detecting GTCS using multimodal parameters within a single device and using personalized algorithms (16).
Most studies investigating wearable seizure detection devices commercially available for people have focussed on overall performance including sensitivity and false alarm rate (FAR) (10). Despite the rapidly growing development of these devices, studies on accuracy remain scarce and the overall quality of the studies is low (9). Published studies have focussed on both motor and non-motor seizures, but their study designs are heterogenous and confusing (40, 49, 54). There are several shortcomings of device validation studies. Firstly, there is a lack of generalizability as there are studies with a small sample size, studies selecting highly specific patient populations and/or studies with a short study period. Some validation studies for example included <5 patients with recordings <5 days and others only included specific type of patients such as adult patients with impaired mental faculties. Second, most devices are tested in a hospital setting which enables the use of EEG to assess its performance. This probably does not reflect everyday ambulatory activity and using a mobile-EEG as control for outpatients would be recommended in these studies. Lastly, seizure type was not always specified and differentiation between day and night should be considered in case of self-reported seizure detection (55).
There are several systematic reviews available which have used a previously published general tool for systematic reviewing of diagnostic accuracy (35, 56). Beniczky and Ryvlin have recently attempted to standardize testing for validating specifically seizure detection devices, by providing a list of key features essential for studies on seizure detection. Depending on how these key features were incorporated, studies were classified into categories (phases) from 0 to 4, with 0 corresponding to initial studies on starting up or developing a new method, and 4 consisting of in-field studies of seizure detection devices in the home environment of the patients. The essential features of this system were grouped as subjects (simulated data or healthy subjects vs. low or high number of patients with seizures), recordings (conventional method vs. dedicated device, discontinuous vs. continuous, single center vs. multicentre, prospective vs. retrospective), analysis & alarm (training and testing using the dataset vs. predefined algorithm and cut off values, offline vs. real time, not blinded vs. blinded) and reference standard (none vs. video or video-EEG recordings) (35). But there are also technical factors that should be considered when measuring the accuracy and performance of a device, such as for example the device deficiency time (proportion of the time period when the device was not functioning), false alarm rate and detection latency (time in seconds from seizure onset of the targeted seizure to the time of seizure detection) (17, 57). So far, the best evidence to reliably detect a seizure in people, has been found for the seizure-type generalized tonic-clonic seizures (GTCS) (3). This is encouraging as this is the most frequently reported seizure type in dogs with idiopathic epilepsy (58, 59).
There are no published standards for study designs in the veterinary literature. Investigating non-EEG changes during the pre-ictal or ictal phase in dogs will require extensive research and, with the knowledge from human medicine, this should ideally be performed via a specific set of standards. Initial studies on starting up or developing a new method may include only a small number of subjects using a conventional or already existing device, and training and testing the algorithm will likely be done on the same data set without the use of the gold standard (video-EEG). If successful, this could lead to large prospective and real-time multicenter studies using predefined algorithms and cut-off values. Video-EEG as a long-term monitor would be the gold standard but due to its impracticality, EEG has never been established as a routine test in canine epilepsy in most referral centers (3). A modified version from the widely accepted human “international 10–20 system” for electrode scalp placement has been used but still no standardized EEG technique had been made in veterinary medicine although recently studies have been aiming to standardize EEG conditions (58–60). For now, veterinary studies continue to rely on visual information on seizure onset obtained in hospital or by the caregiver.
Monitoring dogs with epilepsy can be challenging for dog owners, but also for veterinarians and clinical staff managing hospitalised epileptic dogs. A reliable device that monitors and records seizures may help caregivers feel a sense of control, which may in turn reduce the burden of managing an epileptic dog. So far, there is only one non-EEG device tested in dogs, leaving many opportunities to further explore non-EEG seizure detection devices in veterinary medicine. User needs for dog owners appear similar to caregivers for people as for example they both value a high accuracy but would also accept a high false alarm rate (2, 61). Although the information on study design and device properties in humans is extensive and could potentially be translated to animals, there are many more challenges faced during the design of such a study in veterinary medicine. For example, deciding the anatomical location where the device should be placed and how to obtain a large enough sample size. Figure 1 summarises some of the key features for the study design and for the device itself. Nonetheless, given the challenges of EEG in veterinary medicine for seizure detection, using non-EEG parameters could be a promising alternative. Its use in dogs may help improve emergency intervention and improve hospitalization of epilepsy patients as they are currently kept for observation in an often-overstimulating ICU. If reliable, they may also provide a more accurate seizure count by not relying on the observations of an omnipresent carer for record keeping.
JB and CS: study conception and design. JB: draft manuscript preparation. CS and RG-Q: editing the manuscript. All authors revised the review and approved the final version of the manuscript.
The authors declare that the research was conducted in the absence of any commercial or financial relationships that could be construed as a potential conflict of interest.
All claims expressed in this article are solely those of the authors and do not necessarily represent those of their affiliated organizations, or those of the publisher, the editors and the reviewers. Any product that may be evaluated in this article, or claim that may be made by its manufacturer, is not guaranteed or endorsed by the publisher.
1. Nettifee JA, Munana KR, Griffith EH. Evaluation of the impacts of epilepsy in dogs on their caregivers. J Am Anim Hosp Assoc. (2017) 53:143–9. doi: 10.5326/JAAHA-MS-6537
2. Bongers J, Gutierrez-Quintana R, Stalin CE. Owner's perception of seizure detection devices in idiopathic epileptic dogs. Front Vet Sci. (2021) 8:792647. doi: 10.3389/fvets.2021.792647
3. De Risio L, Bhatti S, Muñana K, Penderis J, Stein V, Tipold A, et al. International veterinary epilepsy task force consensus proposal: diagnostic approach to epilepsy in dogs. BMC Vet Res. (2015) 11:148. doi: 10.1186/s12917-015-0462-1
4. Volk HA. International Veterinary Epilepsy Task Force consensus reports on epilepsy definition, classification and terminology, affected dog breeds, diagnosis, treatment, outcome measures of therapeutic trials, neuroimaging and neuropathology in companion animals. BMC Vet Res. (2015) 11:174. doi: 10.1186/s12917-015-0460-3
5. Ukai M, Parmentier T, Cortez MA, Fischer A, Gaitero L, Lohi H, et al. Seizure frequency discrepancy between subjective and objective ictal electroencephalography data in dogs. J Vet Intern Med. (2021) 35:1819–25. doi: 10.1111/jvim.16158
6. Karoly P, Goldenholz DM, Cook M. Are the days of counting seizures numbered? Curr Opin Neurol. (2018) 31:162–8. doi: 10.1097/WCO.0000000000000533
7. Väätäjä H, Majaranta P, Isokoski P, Gizatdinova Y, Kujala MV, Somppi S, et al. Happy Dogs and Happy Owners - Using Dog Activity Monitoring Technology in Everyday Life. ACM International Conference Proceeding Series (2018).
8. Jory C, Shankar R, Coker D, McLean B, Hanna J, Newman C. Safe and sound? A systematic literature review of seizure detection methods for personal use. Seizure. (2016) 36:4–15. doi: 10.1016/j.seizure.2016.01.013
9. van Westrhenen A, De Cooman T, Lazeron RHC, Van Huffel S, Thijs RD. Ictal autonomic changes as a tool for seizure detection: a systematic review. Clin Auton Res. (2019) 29:161–81. doi: 10.1007/s10286-018-0568-1
10. Kharbouch A, Shoeb A, Guttag J, Cash SS. An algorithm for seizure onset detection using intracranial EEG. Epilepsy Behav. (2011) 22:S29–35. doi: 10.1016/j.yebeh.2011.08.031
11. Fisher RS, Blum DE, DiVentura B, Vannest J, Hixson JD, Moss R, et al. Seizure diaries for clinical research and practice: limitations and future prospects. Epilepsy Behav. (2012) 24:304–10. doi: 10.1016/j.yebeh.2012.04.128
12. Osorio I, Schachter S. Extracerebral detection of seizures: a new era in epileptology? Epilepsy Behav. (2011) 22:S82–7. doi: 10.1016/j.yebeh.2011.09.012
13. Vaughn BV, Quint SR, Tennison MB, Messenheimer JA. Monitoring heart period variability changes during seizures. II. Diversity and trends. J Epilepsy. (1996) 9:27–34. doi: 10.1016/0896-6974(95)00061-5
14. Wessmann A, Volk HA, Packer RMA, Ortega M, Anderson TJ. Quality-of-life aspects in idiopathic epilepsy in dogs. Vet Rec. (2016) 179:229. doi: 10.1136/vr.103355
15. Opherk C, Coromilas J, Hirsch LJ. Heart rate and EKG changes in 102 seizures: analysis of influencing factors. Epilepsy Res. (2002) 52:117–27. doi: 10.1016/S0920-1211(02)00215-2
16. Atwood AC, Drees CN. Five new things: seizure detection devices. Neurol Clin Pract. (2021) 11:367–71. doi: 10.1212/CPJ.0000000000001044
17. Ulate-Campos A, Coughlin F, Gaínza-Lein M, Fernández IS, Pearl PL, Loddenkemper T. Automated seizure detection systems and their effectiveness for each type of seizure. Seizure. (2016) 40:88–101. doi: 10.1016/j.seizure.2016.06.008
18. Blumhardt LD, Smith PEM, Owen L. Electrocardiographic accompaniments of temporal lobe epileptic seizures. Lancet. (1986) 327:1051–6. doi: 10.1016/S0140-6736(86)91328-0
19. Keilson MJ, Allen Hauser WA, Magrill JP. Electrocardiographic changes during electrographic seizures. Arch Neurol. (1989) 46:1169–70. doi: 10.1001/archneur.1989.00520470023018
20. Kerem DH, Geva AB. Forecasting epilepsy from the heart rate signal. Med Biol Eng Comput. (2005) 43:230–9. doi: 10.1007/BF02345960
21. Leutmezer F, Schernthaner C, Lurger S, Pötzelberger K, Baumgartner C. Electrocardiographic changes at the onset of epileptic seizures. Epilepsia. (2003) 44:348–54. doi: 10.1046/j.1528-1157.2003.34702.x
22. Billeci Id L, Marino Id D, Insana L, Vatti G, Varanini M. Patient-specific seizure prediction based on heart rate variability and recurrence quantification analysis. PLoS ONE. (2018) 13:e0204339. doi: 10.1371/journal.pone.0204339
23. De Cooman T, Kjær TW, Van Huffel S, Sorensen HB. Adaptive heart rate-based epileptic seizure detection using real-time user feedback. Physiol Meas. (2018) 39:014005. doi: 10.1088/1361-6579/aaa216
24. De Cooman T, Varon C, Hunyadi B, Van Paesschen W, Lagae L, Van Huffel S. Online automated seizure detection in temporal lobe epilepsy patients using single-lead ECG. Int J Neural Syst. (2017) 27:1750022. doi: 10.1142/S0129065717500228
25. Jeppesen J, Beniczky S, Johansen P, Sidenius P, Fuglsang-Frederiksen A. Detection of epileptic seizures with a modified heart rate variability algorithm based on Lorenz plot. Seizure. (2015) 24:1–7. doi: 10.1016/j.seizure.2014.11.004
26. Jeppesen J, Fuglsang-Frederiksen A, Johansen P, Christensen J, Wüstenhagen S, Tankisi H, et al. Seizure detection using heart rate variability: a prospective validation study. Epilepsia. (2020) 61:S41–6. doi: 10.1111/epi.16511
27. Musteata M, Mocanu D, Stanciu GD, Armasu M, Solcan G. Interictal cardiac autonomic nervous system disturbances in dogs with idiopathic epilepsy. Vet J. (2017) 228:41–5. doi: 10.1016/j.tvjl.2017.10.008
28. Ravindran K, Powell KL, Todaro M, O'Brien TJ. The pathophysiology of cardiac dysfunction in epilepsy. Epilepsy Res. (2016) 127:19–29. doi: 10.1016/j.eplepsyres.2016.08.007
29. Amiez C, Procyk E. Midcingulate somatomotor and autonomic functions. Handb Clin Neurol. (2019) 166:53–71. doi: 10.1016/B978-0-444-64196-0.00004-2
30. Beniczky S, Arbune AA, Jeppesen J, Ryvlin P. Biomarkers of seizure severity derived from wearable devices. Epilepsia. (2020) 61:S61–6. doi: 10.1111/epi.16492
31. Poh M, Loddenkemper T. Continuous monitoring of electrodermal activity during epileptic seizures using a wearable sensor. Annu Int Conf IEEE Eng Med Biol Soc. (2010) 2010:4415–8. doi: 10.1109/IEMBS.2010.5625988
32. Poh MZ, Swenson NC, Picard RW. A wearable sensor for unobtrusive, long-term assessment of electrodermal activity. IEEE Trans Biomed Eng. (2010) 57:1243–52. doi: 10.1109/TBME.2009.2038487
33. Rialland P, Authier S, Guillot M, Del Castillo JR, Veilleux-Lemieux D, Frank D, et al. Validation of orthopedic postoperative pain assessment methods for dogs: a prospective, blinded, randomized, placebo-controlled study. PLoS ONE. (2012) 7:e49480. doi: 10.1371/journal.pone.0049480
34. Slatter DH. Textbook of small animal surgery. 3rd ed. Douglas Slatter, editor. Philidephlia: Saunders; (1993).
35. Beniczky S, Ryvlin P. Standards for testing and clinical validation of seizure detection devices. Epilepsia. (2018) 59:9–13. doi: 10.1111/epi.14049
36. Narechania AP, Garić II, Sen-Gupta I, Macken MP, Gerard EE, Schuele SU. Assessment of a quasi-piezoelectric mattress monitor as a detection system for generalized convulsions. Epilepsy Behav. (2013) 28:172–6. doi: 10.1016/j.yebeh.2013.04.017
37. Zhao X, Lhatoo SD. Seizure detection: do current devices work? And when can they be useful? Curr Neurol Neurosci Rep. (2018) 18:40. doi: 10.1007/s11910-018-0849-z
38. Beniczky S, Jeppesen J. Non-electroencephalography-based seizure detection. Curr Opin Neurol. (2019) 32:198–204. doi: 10.1097/WCO.0000000000000658
39. Beniczky S, Polster T, Kjaer TW, Hjalgrim H. Detection of generalized tonic-clonic seizures by a wireless wrist accelerometer: a prospective, multicenter study. Epilepsia. (2013) 54:e58–61. doi: 10.1111/epi.12120
40. Meritam P, Ryvlin P, Beniczky S. User-based evaluation of applicability and usability of a wearable accelerometer device for detecting bilateral tonic–clonic seizures: a field study. Epilepsia. (2018) 59:48–52. doi: 10.1111/epi.14051
41. Patterson AL, Mudigoudar B, Fulton S, McGregor A, Poppel KV, Wheless MC, et al. SmartWatch by SmartMonitor: assessment of seizure detection efficacy for various seizure types in children, a large prospective single-center study. Pediatr Neurol. (2015). 53:309–11. doi: 10.1016/j.pediatrneurol.2015.07.002
42. Regalia G, Onorati F, Lai M, Caborni C, Picard RW. Multimodal wrist-worn devices for seizure detection and advancing research: focus on the Empatica wristbands. Epilepsy Res. (2019) 153:79–82. doi: 10.1016/j.eplepsyres.2019.02.007
43. Johansson D, Ohlsson F, Krýsl D, Rydenhag B, Czarnecki M, Gustafsson N, et al. Tonic-clonic seizure detection using accelerometry-based wearable sensors: a prospective, video-EEG controlled study. (2018) 65:48–54. doi: 10.1016/j.seizure.2018.12.024
44. van Elmpt WJC, Nijsen TME, Griep PAM, Arends JBAM. A model of heart rate changes to detect seizures in severe epilepsy. Seizure. (2006) 15:366–75. doi: 10.1016/j.seizure.2006.03.005
45. Muñana KR, Nettifee JA, Griffith EH, Early PJ, Yoder NC. Evaluation of a collar-mounted accelerometer for detecting seizure activity in dogs. J Vet Intern Med. (2020) 34:1239–47. doi: 10.1111/jvim.15760
46. van Andel J, Thijs RD, de Weerd A, Arends J, Leijten F. Non-EEG based ambulatory seizure detection designed for home use: what is available and how will it influence epilepsy care? Epilepsy Behav. (2016) 57:82–9. doi: 10.1016/j.yebeh.2016.01.003
47. Stimulus-responsive psychomotor epilepsy in a Doberman pinscher [Internet]. Available online at: https://agris.fao.org/agris-search/search.do?recordID=US8905454 (accessed: May 28, 2020).
48. Behavioral changes associated with suspected complex partial seizures in Bull Terriers. J - Google Search [Internet]. [cited (2020). May 28].
49. Srenk P, Jaggy A. Interictal electroencephalographic findings in a family of golden retrievers with idiopathic epilepsy. J Small Anim Pract. (1996) 37:317–21. doi: 10.1111/j.1748-5827.1996.tb02398.x
50. Milosevic M, Van De Vel A, Bonroy B, Ceulemans B, Lagae L, Vanrumste B, et al. Automated detection of tonic-clonic seizures using 3-D accelerometry and surface electromyography in pediatric patients. IEEE J Biomed Health Inform. (2016) 20:1333–41. doi: 10.1109/JBHI.2015.2462079
51. Poppel KV, Fulton SP, Mcgregor A, Ellis M, Patters A, Wheless J, et al. Prospective study of the Emfit movement monitor. J Child Neurol. 28:1434–6. doi: 10.1177/0883073812471858
52. Kurada AV, Srinivasan T, Hammond S, Ulate-Campos A, Bidwell J. Seizure detection devices for use in antiseizure medication clinical trials: a systematic review. Seizure. (2019) 66:61–9. doi: 10.1016/j.seizure.2019.02.007
53. Whiting PF, Rutjes AWS, Westwood ME, Mallett S, Deeks JJ, Reitsma JB, et al. Quadas-2: a revised tool for the quality assessment of diagnostic accuracy studies. Ann Intern Med. (2011) 155:529–36. doi: 10.7326/0003-4819-155-8-201110180-00009
54. Van de Vel A, Cuppens K, Bonroy B, Milosevic M, Jansen K, Van Huffel S, et al. Non-EEG seizure detection systems and potential SUDEP prevention: state of the art: review and update. Seizure. (2016) 41:141–53. doi: 10.1016/j.seizure.2016.07.012
55. Beniczky S, Wiebe S, Jeppesen J, Tatum WO, Brazdil M, Wang Y, et al. Automated seizure detection using wearable devices: a clinical practice guideline of the International League Against Epilepsy and the International Federation of Clinical Neurophysiology. Clin Neurophysiol. (2021) 132:1173–84. doi: 10.1016/j.clinph.2020.12.009
56. Huenerfauth E, Nessler J, Erath J, Tipold A. Probable Sudden Unexpected Death in Dogs With Epilepsy (pSUDED). Front Vet Sci. (2021) 8:600307. doi: 10.3389/fvets.2021.600307
57. Stabile F, van Dijk J, Barnett CR, De Risio L. Epileptic seizure frequency and semiology in dogs with idiopathic epilepsy after initiation of imepitoin or phenobarbital monotherapy. Vet J. (2019) 249:53–7. doi: 10.1016/j.tvjl.2019.05.007
58. Berendt M, Høgenhaven H, Flagstad A, Dam M. Electroencephalography in dogs with epilepsy: similarities between human and canine findings. Acta Neurol Scand. (1999) 99:276–83. doi: 10.1111/j.1600-0404.1999.tb00676.x
59. James FMK, Allen DG, Bersenas AME, Grovum WL, Kerr CL, Monteith G, et al. Investigation of the use of three electroencephalographic electrodes for long-term electroencephalographic recording in awake and sedated dogs. Am J Vet Res. (2011) 72:384–90. doi: 10.2460/ajvr.72.3.384
60. James FMK, Cortez MA, Monteith G, Jokinen TS, Sanders S, Wielaender F, et al. Diagnostic utility of wireless video-electroencephalography in unsedated dogs. J Vet Intern Med. (2017) 31:1469–76. doi: 10.1111/jvim.14789
61. van Westrhenen A, Souhoka T, Ballieux ME, Thijs RD. Seizure detection devices: exploring caregivers' needs and wishes. Epilepsy Behav. (2021) 116:107723. doi: 10.1016/j.yebeh.2020.107723
Keywords: wearable, technology, canine, epilepsy, review, seizure detection, dog
Citation: Bongers J, Gutierrez-Quintana R and Stalin CE (2022) The Prospects of Non-EEG Seizure Detection Devices in Dogs. Front. Vet. Sci. 9:896030. doi: 10.3389/fvets.2022.896030
Received: 14 March 2022; Accepted: 28 April 2022;
Published: 23 May 2022.
Edited by:
Steven De Decker, Royal Veterinary College (RVC), United KingdomReviewed by:
Sam Long, Veterinary Referral Hospital, AustraliaCopyright © 2022 Bongers, Gutierrez-Quintana and Stalin. This is an open-access article distributed under the terms of the Creative Commons Attribution License (CC BY). The use, distribution or reproduction in other forums is permitted, provided the original author(s) and the copyright owner(s) are credited and that the original publication in this journal is cited, in accordance with accepted academic practice. No use, distribution or reproduction is permitted which does not comply with these terms.
*Correspondence: Catherine Elizabeth Stalin, Y2F0aGVyaW5lLnN0YWxpbkBnbGFzZ293LmFjLnVr
Disclaimer: All claims expressed in this article are solely those of the authors and do not necessarily represent those of their affiliated organizations, or those of the publisher, the editors and the reviewers. Any product that may be evaluated in this article or claim that may be made by its manufacturer is not guaranteed or endorsed by the publisher.
Research integrity at Frontiers
Learn more about the work of our research integrity team to safeguard the quality of each article we publish.