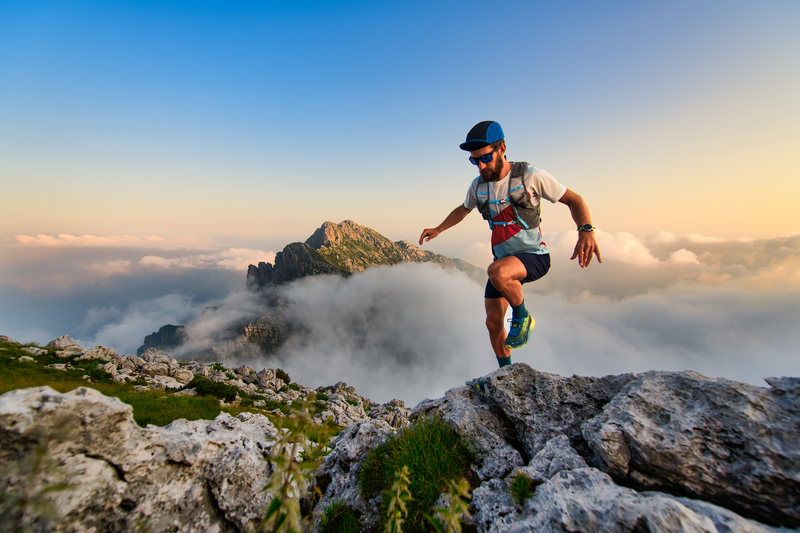
94% of researchers rate our articles as excellent or good
Learn more about the work of our research integrity team to safeguard the quality of each article we publish.
Find out more
ORIGINAL RESEARCH article
Front. Vet. Sci. , 08 July 2022
Sec. Animal Reproduction - Theriogenology
Volume 9 - 2022 | https://doi.org/10.3389/fvets.2022.890979
Ovulation is a complicated physiological process that is regulated by a multitude of different pathways. In comparison to mammalian studies, there are few reports of ovulation in Muscovy ducks, and the molecular mechanism of ovarian development remained unclear. In order to identify candidate genes and metabolites related to Muscovy duck follicular ovulation, the study combined Oxford Nanopore Technologies (ONT) full-length transcriptome and metabolomics to analyze the differences in gene expression and metabolite accumulation in the ovaries between pre-ovulation (PO) and consecutive ovulation (CO) Muscovy ducks. 83 differentially accumulated metabolites (DAMs) were identified using metabolomics analysis, 33 of which are related to lipids. Combined with data from previous transcriptomic analyses found that DEGs and DAMs were particularly enriched in processes including the regulation of glycerophospholipid metabolism pathway, arachidonic acid metabolic pathway and the steroid biosynthetic pathway. In summary, the novel potential mechanisms that affect ovulation in Muscovy ducks may be related to lipid metabolism, and the findings provide new insights into the mechanisms of ovulation in waterfowl and will contribute to a better understanding of changes in the waterfowl ovarian development regulatory network.
The Muscovy duck (Cairina moschata) is an outstanding meat breed with high protein content and superb meat quality (1), but its strong nesting behavior causes brooding behavior, which leads to low egg production and severely limits the current duck industry's development requirements. Waterfowl ovaries contain numerous follicles of various sizes and stages of development which resemble a cluster of grapes, with each follicle acting as an individual grape. A sluggish growing process occurs of the follicle development from primordial follicle development to ovulation, a strict hierarchical system and selection of dominant follicles are required (2). After developing into hierarchical follicles, they will be filled with yolk and grow quickly. At peak period of duck production, the biggest follicle from the hierarchical follicles will select to ovulate every day, which is a unique characteristic of waterfowl follicle growth and ovulation (3, 4). Only a few follicles can become dominant follicles, and the majority of them have the possibility of atresia at different phases of development (5). However, the mechanism affecting Muscovy duck ovulation is still unclear, and it might be linked to a series of complicated processes.
For more than 10 years, short-read RNA-Seq has been utilized, in which the RNA or cDNA needs to be fragmented during the sample preparation procedure, resulting in the loss of some information in the original transcript. In recent years, the technology for gene sequencing has advanced tremendously, and Oxford Nanopore Technologies (ONT) is currently at the forefront of the area (6, 7). ONT has overcome the read-length barrier to accomplish ultra-long read, single-base detection at the genome-wide level (8, 9). Metabolomics is a technique for detecting metabolites (small compounds) in blood, tissues, and other biological materials, with the presence and relative concentration of these molecules acting as can be used as evidence of processes and functions (10). The “omics” sciences of metabolomics, proteomics, transcriptomics, and genomics are closely linked and are considered the cornerstones of systems biology (11). A single omics approach provides limited insights into complex molecular pathways and complex biological events in cells and organisms. With the maturation of multi-omics analysis methods, more and more omics are being utilized together to more systematically explain the complicated correlation between phenotype and mechanism (12, 13).
In recent years, many research on waterfowl follicles genomics have been published, most of which have focused on single transcriptome data, such as Xu et al. (14) analyzed the transcriptome sequencing of pigeon ovaries at pre-ovulation, post-ovulation and 5–6 days after ovulation, Zhang et al. (15) analyzed and compared the transcriptome of ovarian tissues from chickens with relatively greater and lesser egg production, and Hu et al. (16) studied the dynamics of the transcriptome of goose ovaries during late embryonic and early post-hatching stages. However, there has been few published research on a comprehensive comparative metabolome–transcriptome analysis of waterfowl follicles. We explored the changes in the development of female Muscovy ducks from metabolites and gene expression, as well as the potential underlying mechanisms affecting ovulation in Muscovy ducks, using metabolomics combined with transcriptomics analysis. It is hoped that this research will contribute to increasing the theoretical guidance of Muscovy duck genetic breeding and improving the actual breeding efficiency.
Muscovy duck was achieved from Shaanxi Anda Agricultural Development Co., Ltd (Shannxi, China). The ovaries of 12 Muscovy ducks were sampled, including six ovaries from pre-ovulated (PO, 22 weeks) and six ovaries from consecutive-ovulated (CO, 40 weeks) Muscovy duck. Each set of ducks had a similar body weight and had the same genetic background and appearance. They were also grown under the same feeding management conditions. All methods and procedures were conducted in accordance with the relevant guidelines formulated by the Ministry of Agriculture of the People's Republic of China and involved animal manipulation was approved by the Faculty Animal Care and Use Committee of Northwest A&F University (Shannxi, China). All samples were immediately frozen in liquid nitrogen and transferred to −80°C for further use. After the samples were thawed on ice, weigh the sample 50 mg, add 1,000 μl of pre-cooled extractant (70% aqueous methanol solution containing 1 μg/ml of 2-chlorophenylalanine as internal standard), add pre-cooled steel beads, homogenize for 3 min at 30 Hz, remove the beads, vortex for 1 min, let stand on ice for 15 min, centrifuge for 10 min at 4°C, 12,000 r/min, remove the supernatant into the injection vial liner tube. The supernatant was taken into the liner tube of the injection vial and used for LC-MS/MS analysis, and cDNA sequencing was performed on a MinION platform (Oxford Nanopore Technologies, Oxford, UK) (17).
The sample extracts were analyzed using an LC-ESI-MS/MS system (UPLC, Shim-pack UFLC SHIMADZU CBM A system, https://www.shimadzu.com/; MS, QTRAP® System, https://sciex.com/). The analytical conditions were as follows, UPLC: column, Waters ACQUITY UPLC HSS T3 C18 (1.8 μm, 2.1 mm * 100 mm); column temperature, 40°C; flow rate, 0.4 ml/min; injection volume, 2 μl; solvent system, water (0.04% acetic acid): acetonitrile (0.04% acetic acid); gradient program, 95:5 V/V at 0 min, 5:95 V/V at 11.0 min, 5:95 V/V at 12.0 min, 95:5 V/V at 12.1 min, 95:5 V/V at 14.0 min. LIT and triple quadrupole (QQQ) scans were acquired on a triple quadrupole-linear ion trap mass spectrometer (QTRAP), QTRAP® LC-MS/MS System, equipped with an ESI Turbo Ion-Spray interface, operating in positive and negative ion mode and controlled by Analyst 1.6.3 software (Sciex). The ESI source operation parameters were as follows: source temperature 500°C; ion spray voltage (IS) 5,500 V (positive), −4,500 V (negative); ion source gas I (GSI), gas II (GSII), curtain gas (CUR) was set at 55, 60, and 25.0 psi, respectively; the collision gas (CAD) was high. Instrument tuning and mass calibration were performed with 10 and 100 μmol/L polypropylene glycol solutions in QQQ and LIT modes, respectively. A specific set of MRM transitions were monitored for each period according to the metabolites eluted within this period.
Principal component analysis was used to observe the degree of variability between different groups and between samples within groups. The variables with less correlation are analyzed by Partial Least Squares-Discriminant Analysis (PLS-DA). PLS-DA is a multivariate statistical analysis method that extracts independent variable X and dependent variable Y, then calculates the correlation between the components. Compared with PCA, PLS-DA can maximize the distinction between groups, which is conducive to finding different metabolites. Orthogonal Partial Least Squares Discriminant Analysis (OPLS-DA) combines orthogonal signal correction (OSC) and PLS-DA methods to screen difference variables by removing irrelevant differences. The data obtained for differentially accumulated metabolites is by the preliminary screening of differential metabolites from the VIP value (Variable Importance in Projection, VIP) of the OPLS-DA model, and then combining the P-value and fold change of univariate analysis to further screen out differential metabolites. Threshold of variables determined to be important in the projection (VIP) scores ≥1.0 together with fold change ≥2 or ≤ 0.5 was adopted to assess differentially accumulated metabolites. Kyoto Encyclopedia of Genes and Genomes (KEGG, http://www.genome.jp/kegg/) was utilized to search for the metabolite pathways. Furthermore, we conducted integrative studies of metabolomics with our previous transcriptomics data (18) by displaying metabolites related with genes on the pathways. Integrated analysis was performed using BMKCloud (www.biocloud.net).
For this study, transcriptomic and metabolomic analyses used samples from the same participants and time points. Three independent biological replicates were tested for transcriptomic analysis, and six independent biological replicates were conducted for metabolic analysis. The same sample is used for combined transcriptome and metabolome analysis.
To better understand the changes in the CO-PO group, the PCA and OPLS-DA score plots that we constructed using the acquired metabolomic data. The PCA and OPLS-DA modes of Muscovy Duck are shown in Figure 1. Segregation trends between groups in metabolic physiology were lightly detected using the unsupervised PCA of the entire set of measured analytes. The result showed that the two sets of samples show a good separation trend as can be seen from the PCA model (Figure 1A). To maximize the discrimination between the two groups, and thus, we performed orthogonal partial least squares discriminant analysis (OPLS-DA). The OPLS-DA model maximizes the discrimination between groups and is more conducive to the subsequent search for differential metabolites (Figure 1B). To verify this model from a statistical point of view, this model performed 200 random permutation and combination experiments on the data, the model parameters were obtained as R2X = 0.493, R2Y = 0.927, and Q2 = 0.886 (Figure 1C). According to the prediction parameters of the evaluation model, R2X, R2Y and Q2 indicated that OPLS-DA model suffered from neither excessive randomness nor overfitting, which indicated that the model was stable and reliable and had a high predictive ability. Therefore, these data could be used for subsequent analysis.
Figure 1. Qualitative and quantitative analysis of the metabolomics data. (A) Principal component analysis (PCA) of metabolomics. (B) Orthogonal partial least squares discriminant analysis (OPLS-DA) scores. (C) OPLS-DA model validation. (D) Variable importance in projection (VIP) values, the metabolites closer to the upper right and lower left corner indicate their more significant differences. Red dots indicate VIP values ≥1 for these metabolites, and green dots indicate VIP values <1 for these metabolites. (E) Volcano plots of all identified metabolites, each point in the volcano plot represents a metabolite. Green dots in the plot represent down-regulated differentially expressed metabolites, red dots represent up-regulated differentially expressed metabolites, and black represents metabolites detected but with insignificant differences. The X-axis represents the logarithmic value of the quantitative difference multiples of a metabolite in two samples; the Y-axis represents the VIP value.
According to the basis of the OPLS-DA results, the variable importance projection (VIP) of the OPLS-DA model obtained from the multivariate analysis can also be combined with the P-value of univariate analysis or the fold change value (fold change) to further filter different metabolites. Variable importance in projection (VIP) >1.0 together with fold change ≥2 or ≤ 0.5 was used as the screening standard for differential abundant metabolites (Figure 1D), a total of 83 metabolites showed significant differences in the PO vs. CO comparison, 55 metabolites in the PO group were significantly upregulated compared with those in the CO group. By contrast, 28 metabolites were significantly downregulated. Their corresponding information was also shown in volcano plots, which could intuitively indicate the differences in the metabolites between the PO-CO groups (Figure 1E). The DAMs in CO vs. PO could be categorized into 10 classes, the classes of Lipids (39.76%), Nucleotide metabolomics (15.66%), Amino acid metabolomics (8.42%), Benzene and substituted derivatives (7.23%) and Organic Acid and Its Derivatives (7.23%) accounted for a large proportion (Figure 2A). It is surprising that the most of top 20 up-regulated DAMs belong to the classification of Lipids Others Phospholipid (Figure 2B). The classes of Lipids and Others Phospholipid including Lysopg 18:1, Lysope 18:1, Lysope 18:0, Lysope 16:0, Lysope 14:0, Lysopa 18:0, Lysopa 16:0, Lysopc 14:0, Lysopc 16:0, Lysopc 16:1, Lysopc 18:0, Lysopc 18:1, Lysopc 18:2, Lysopc 20:1, Lysopc 20:2, Lysopc 17:0, Lysopc 15:0, Lysopc 18:3 were increased (Supplementary Table 1). Pathway analysis was subsequently conducted based on the Kyoto Encyclopedia of Genes and Genomes (KEGG) database. DAMs are mainly grouped under the terms of Glycerophospholipid metabolism, Choline metabolism in cancer, Antifolate resistance, Purine metabolism, Autoimmune thyroid disease (Figure 3).
Figure 2. The classes of differentially accumulated metabolites. (A) Pie charts showing different classes of total DAMs, different colors indicate different classes. (B) The top 20 up-regulated and down-regulated DAMs were illustrated in the Sankey diagram.
Figure 3. Top 20 Kyoto Encyclopedia of Genes and Genomes (KEGG) pathway terms (P < 0.05) enriched by differentially accumulated metabolites (DAMs). The X-axis means rich factor. The Y-axis represents the KEGG pathway terms.
In order to track the change process of the metabolome more deeply, we join the transcriptome for multi-omics joint analysis. Previous transcriptomics data showed that a total of 3,046 DEGs (1,046 up-regulated and 2,000 down-regulated) were identified in the PO compared with the CO group (18). A comprehensive analysis of metabolomics and transcriptomics was performed, and 55 Co-enriched pathways were derived (Figure 4), the lipid metabolism-related pathways were dramatically changed, of which we focused on three pathways including Arachidonic Acid metabolism, Glycerophospholipid metabolism and Steroid hormone biosynthesis pathways. We present the relationship between the differential gene expression and differentially abundant metabolites associated with these pathways with pictures in the figure (Figure 5). Integrated analysis among these three altered pathways revealed that Glycerophospholipid metabolism was the top enriched pathway, with 14 DEGs and 12 DAMs, including Lysopa 16:0, Lysopc 14:0, Lysopc 16:0, Lysopc 16:1, Lysopc 18:0, Lysopc 18:1, Lysopc 18:2, Lysopc 20:1, Lysopc 20:2, Lysopc 17:0, Lysopc 15:0 and O-Phosphorylethanolamine, all these LPCs and LPAs involved in glycerophospholipid metabolism pathways were significantly increased, whereas the O-Phosphorylethanolamine was down-regulated. Through ARA and steroid hormone biosynthesis pathway analysis, PGEs and estrone 3-sulfate were found to be dramatically changed in the PO group. 12 DEGs were increased, whereas 13 DEGs were decreased, according to the transcriptional levels (Supplementary Tables 2, 3).
Figure 4. KEGG Pathway co-enriched analysis of DEGs and DAMs. Bar chart showing the number of metabolites covered by each enriched pathway. The Y-axis indicates –Log10 (P-value) of metabolites while the X-axis indicates the pathway name. Red: gene; blue: metabolites.
Figure 5. Constructed three key pathways that integrated relative metabolite contents and gene expression. Different colors were used to represent down-regulated DEGs (blue), up-regulated DEGs (red). The heatmaps are drawn according to the metabolomics data. Columns and rows in the heatmap represent groups and metabolites, respectively. The color scale indicates fold changes (Log10) in gene expression.
There are a complex series of changes that occur during follicular development in Muscovy ducks. Only a few follicles are chosen to develop into the dominant follicle and the majority of follicles undergo atretic degeneration before ovulation (5). Follicular development and eventual ovulation are essential to ensure egg production and promote fertility in waterfowl. Multi-Omics technologies create an encouraging environment for researchers to explore biology mechanisms of complex systems (19, 20). Here, we integrated transcriptomics and metabolomics to analyze DEGs and DAMs of ovaries, explored the correlation between gene expression changes and metabolite abundance in the enriched pathway, as well as the further elucidated potential mechanisms that may influence ovulation in Muscovy ducks. Analyzing the DAMs at different phases of ovarian follicle development revealed a considerable degree of lipid metabolism change. Lipids play a variety of roles in the body, including energy storage, biofilm formation, and signaling molecules (21, 22). Understanding the lipid metabolic pathways and the genes involved is essential for further research into lipid biological functions. To date, there have been a lot of studies focusing on the mechanisms by which mammalian lipid metabolism regulates ovulation (23–25), while very little has been reported in waterfowl studies.
Arachidonic acid pathway was one of the pathways that dramatically changed. Arachidonic acid is a polyunsaturated fatty acid (PUFA) that is converted to cyclic compounds such as prostaglandins, prostacyclins, and thromboxanes by cyclooxygenase (COX), and non-cyclic compounds by lipoxygenase (LOX) to generate HETE and leukotrienes (LT) (26, 27). According to studies, arachidonic acid levels are higher in the ovaries after ovulation than the ovaries before ovulation. Arachidonic acid influences ovulation by regulating granulosa cell-oocyte communication and oocyte maturation paracrine signals (22). Ovulation has also been linked to the activation of metabolism-related alterations in COX and LOX (28). PGG2 can be further catalyzed by PTGS2 to form prostaglandin H2 (PGH2) (29), and then under the action of prostaglandin E synthase (PGES), which is encoded by the PTGES gene, to produce PGE2. Through its surface G-protein-coupled receptor, PGE2 can function in concert with gonadotropin (hCG) signaling (30). It is worth mentioning that PTGS2 is the rate-limiting enzyme in prostaglandin E2 (PGE2) synthesis and is expressed at high levels in pre-ovulatory follicle granulosa cells, which is consistent with our transcriptome findings. According to Fujimori et al. (31), the results of the study strongly indicate that the prostaglandin E2 produced by COX-2 is involved in the ovulation of the Medaka. Zebrafish have increased PTGS2 expression during ovulation, whereas mice with deficient PTGS2 expression are unable to ovulate (32). The PTGS2/PGE2 biosynthesis pathway may at least partly regulate the induction of genes involved in the steroid biosynthesis pathway. Extensive literature has shown that the PTGS2-activated pathway is required for successful ovulation (32, 33). Indeed, both 5- LOX and 15-LOX levels have been proven to be significantly increased during pregnancy (34). GPX is a prominent antioxidant enzyme that is significantly more expressed in mature oocytes than in immature oocytes, and it can protect cells from oxidative damage during ovulation. Consistent with the literature, our research found that the transcript level of GPX1, GPX2 and GPX8 all significantly increased. Uterine contractions are induced by 5-HETE, which is produced by 5-LOX via GPX (35). 15-LOX generates 15-HETE through GPX, and studies have revealed that 15 (S)-HETE levels are considerably higher during ovulation in both cattle and females (36). Ovulation is actually an inflammatory reaction, and LTs have inflammatory and immune functions and play essential roles in follicle maturation, ovulation, and luteal formation. LTA4 is a precursor of LT biosynthesis that is very unstable and readily metabolized by LTA4 hydrolase to LTB4 or by LTC4 synthase to LTC4. More leukotrienes are produced in the plasma of pregnant women. LTB4 regulates the recruitment of NK and T cells (37, 38) and enhances the innate immune defense of pregnant women (39). LTC4 is significantly increased during ovulation in rats, and our study also corroborates this result.
One of the most critical processes for ovarian development and reproduction in animals is the steroid biosynthesis pathway (40, 41). In mammalian follicles, estrogen is mostly synthesized in granulosa cells, the precursor of estrogen synthesis (androgens) is provided by membrane cells for granulosa cells, and progesterone is primarily secreted by the corpus luteum after ovulation (42, 43). Whereas it is very different between waterfowl and mammals (44), the waterfowl has a unique hierarchical follicular system and does not form a corpus luteum after ovulation, and steroid hormones are synthesized and secreted by the granulosa and membranous cell layers of the follicle (45, 46). Progesterone, a steroid hormone secreted by the gonads, is produced by the granulosa cells of the pre-ovulatory follicle, and androgens and estrogens are synthesized in the inner and outer layers of the membrane cells, respectively. Starting with the formation of pregnenolone (P5) which is catalyzed by cholesterol via cholesterol side-chain cleavage enzyme (P450SCC/CYP11A1), followed by the conversion of pregnenolone to progesterone by 3β-hydroxysteroid dehydrogenase (3β-HSD) (42, 47). Despite the evident differences in follicular development, numerous studies have shown that the pathway catalyzing cholesterol to progesterone is basically the same as that of mammals. The other branch, the conversion from pregnenolone to DHEA and subsequently from DHEA to A4, is regulated by 3β-hydroxysteroid dehydrogenase (3βHSD) (48). A4 generates estrone which in turn generates estrone 3-sulfate, and Estrone generates E2 in response to HSD17B7. A4 can also be converted to testosterone (T) by catalysis of 17βHSD2 and eventually aromatized to estradiol (E2) by cytochrome P450 aromatase (P450arom/CYP19) (49). Here, our transcriptome analysis data enrichment analysis showed significant differences in the PO-CO group for the genes HSD-3β, HSD17b1, HSD17b2, HSD17b7, and CYP19a AKD1R1 involved in the steroid biosynthesis pathway, and metabolomic results showed a significant increase in the accumulation of progesterone and estrone as well. HSD-3β is one of the key enzymes in the steroidogenesis pathway, whose expression steadily increases in granulosa cells of growing hierarchical follicles (50, 51). Through a series of endocrine processes in the embryonic ovary, HSD-3β and gonadotropins are likely to promote the initiation of germ cell meiosis (52). HSD17B2 is an endoplasmic reticulum enzyme that inactivates hormones. The gene encoding HSD17B2 is expressed in the endometrium and placental capillaries (53–55). Aromatase is encoded by the CYP19A1 gene. CYP19A1 knockout mice are unable to synthesize estrogen, resulting in granulosa cell apoptosis and follicular atresia, indicating that CYP19A1 plays a decisive role in the normal ovulation of the ovary (56, 57). The steroid biosynthetic pathway may be involved in follicle development and eventually ovulation, and its regulatory in ducks need to be proven in further experiments.
Furthermore, Glycerophospholipid metabolism is the top enriched metabolism pathway. Glycerophospholipids are the most abundant phospholipids and serve as a source of energy for cellular metabolism. They also play an important role in cell signaling and cell membrane development. Diacylglycerol (DAG) is a glycerolipid metabolic intermediate and the world's first known lipid second messenger (23). Phosphatidylinositol (4, 5)-biphospholipids are hydrolyzed to produce DAG, and protein kinase C (PKC) is the major effector (58). Diacylglycerol kinase (DGK) cleaves diacylglycerol to form phosphatidic acid (PA), and PLA-type enzymes deacylated PA to produce LPA, which refers to 1-acyl-2-hydroxy-sn-glycero-3-phosphate and belongs to a group of lysogenic glycerolipids, although other forms exist as well (59). Metabolomic analysis showed that Lysopa 18:0 and Lysopa 16:0 were highly accumulated in the PO-CO group in our study. Lysopa 16:0 is the most abundant form of LPA in humans, and LPA has been attributed to follicular growth and ovulation in human (60), bovine (61), and porcine (62). LPA is released in the endometrium of cattle, induces the expression of COX-2, progesterone, and PGE2, and is significantly increased during human pregnancy (63). Moreover, numerous studies have suggested that LPA may plays a role in oocytes (64). In human oocytes cultured in vitro, the addition of lysophosphatidic acid to immature oocytes increased cell cycle protein B1 levels in MI and MII stage oocytes and boosted the maturation rate of oocytes in vitro considerably (62). LPA has also been proven to activate the mitogen-activated protein kinase (MAPK) pathway, which enhances oocyte nuclear maturation (62, 65). These findings suggest that ovarian-derived LPA is an essential regulator of normal ovarian development and ovulation. In addition, our metabolome profiling various types of LPC involved in glycerophospholipid metabolism were altered, with Lysopc 14:0, Lysopc 16:0, Lysopc 16:1, Lysopc 18:0, Lysopc 18:1, Lysopc 18:2, Lysopc 20:1, and Lysopc 20:2 significantly upregulated in the PO-CO group. Phospholipase A2 (PLA2) metabolizes membrane lipids to arachidonic acid (AA) and LPC. LPC is highly mobile within intact cells, acts as a cytoplasmic messenger to signal downstream processes and gene expression in the nucleus (66), and is also capable of activating multiple second messengers, and LPC in follicular fluid is involved in many essential processes in signal transduction and cell-to-cell communication. Human follicular metabolome results indicate that LPC (13:0) and LPC (18:0) are highest in oocytes and that LPC may be involved in the regulation of follicular development and oocyte maturation, which may be closely related to ovulation (67, 68). LysoPCs are a source of long-chain fatty acids. At the start of egg-laying, Muscovy ducks may generate inflammation in local tissues such as the reproductive tract. As a substrate for macrophage membrane remodeling, lysoPCs assist macrophages to invade tissues rapidly during inflammation. LysoPCs are tightly linked with ovulation (69).
In conclusion, we identified underlying metabolomics differences among pre-ovulation and consecutive ovulation Muscovy ducks, conducted the integrated analysis with transcriptomics. The results suggested that Arachidonic Acid metabolism, Glycerophospholipid metabolism, and Steroid hormone biosynthesis pathways may be the key processes associated with the mechanism of Muscovy duck ovulation. Candidate genes, metabolites and pathways identified in this study require further experiments to demonstrate. In addition, this finding, combined with mammalian systems, contributes to a more comprehensive understanding of the mechanisms that regulate ovarian development in animals and new strategies to improve fertility.
The datasets presented in this study can be found in online repositories. The names of the repository/repositories and accession number(s) can be found below: https://www.ncbi.nlm.nih.gov/, SRR12836353, SRR12836352, SRR12836351, SRR12836356, SRR12836355, and SRR12836354.
The animal study was reviewed and approved by Faculty Animal Care and Use Committee of Northwest A&F University (Shannxi, China).
JL and XL conceived and co-ordinated the study. JL performed the study and wrote the manuscript. JL and LG carried out the bioinformatics. XM, YN, CC, SH, and XL gave advices about concept and revised manuscript. All authors read and approved the final manuscript.
This study supported by China Agriculture Research System of MOF and MARA (CARS-42) and the Research on the Effect of New Breeding of the Black Muscovy Duck (2019QYPY-130).
The authors declare that the research was conducted in the absence of any commercial or financial relationships that could be construed as a potential conflict of interest.
All claims expressed in this article are solely those of the authors and do not necessarily represent those of their affiliated organizations, or those of the publisher, the editors and the reviewers. Any product that may be evaluated in this article, or claim that may be made by its manufacturer, is not guaranteed or endorsed by the publisher.
We thank Biomarker Technologies for helping with ONT and Metabolomics technologies.
The Supplementary Material for this article can be found online at: https://www.frontiersin.org/articles/10.3389/fvets.2022.890979/full#supplementary-material
1. Aronal AP, Huda N, Ahmad R. Amino acid and fatty acid profiles of peking and Muscovy duck meat. Int J Poult Sci. (2012) 11:229–36. doi: 10.3923/ijps.2012.229.236
2. Johnson AL. Ovarian follicle selection and granulosa cell differentiation. Poult Sci. (2014) 94:781–5. doi: 10.3382/ps/peu008
3. Onagbesan O, Bruggeman V, Decuypere E. Intra-ovarian growth factors regulating ovarian function in avian species: a review. Anim Reprod Sci. (2009) 111:121–40. doi: 10.1016/j.anireprosci.2008.09.017
4. Woods DC, Johnson AL. Regulation of follicle-stimulating hormone-receptor messenger RNA in hen granulosa cells relative to follicle selection. Biol Reprod. (2005) 72:643–50. doi: 10.1095/biolreprod.104.033902
5. Gilbert AB, Perry MM, Waddington D, Hardie MA. Role of atresia in establishing the follicular hierarchy in the ovary of the domestic hen (Gallus domesticus). J Reprod Fertil. (1983) 69:221–7. doi: 10.1530/jrf.0.0690221
6. Lin B, Hui J, Mao H. Nanopore technology and its applications in gene sequencing. Biosensors. (2021) 11:214. doi: 10.3390/bios11070214
7. Liu Y, Rosikiewicz W, Pan Z, Jillette N, Wang P, Taghbalout A, et al. DNA methylation-calling tools for Oxford nanopore sequencing: a survey and human epigenome-wide evaluation. Genome Biology. (2021) 22:295. doi: 10.1186/s13059-021-02510-z
8. Levy SE, Myers RM. Advancements in next-generation sequencing. Annu Rev Genomics Hum Genet. (2016) 17:95–115. doi: 10.1146/annurev-genom-083115-022413
9. Goodwin S, McPherson JD, McCombie WR. Coming of age: 10 years of next-generation sequencing technologies. Nat Rev Genet. (2016) 17:333–51. doi: 10.1038/nrg.2016.49
10. Chen C, Gonzalez FJ, Idle JR. LC-MS-based metabolomics in drug metabolism. Drug Metab Rev. (2007) 39:581–97. doi: 10.1080/03602530701497804
11. Xia J, Gill EE, Hancock REW. Network analyst for statistical, visual and network-based meta-analysis of gene expression data. Nat Protoc. (2015) 10:823–44. doi: 10.1038/nprot.2015.052
12. Chong J, Xia J. Computational approaches for integrative analysis of the metabolome and microbiome. Metabolites. (2017) 7:62. doi: 10.3390/metabo7040062
13. Ritchie MD, Holzinger ER, Li R, Pendergrass SA, Kim D. Methods of integrating data to uncover genotype-phenotype interactions. Nat Rev Genet. (2015) 16:85–97. doi: 10.1038/nrg3868
14. Xu X, Zhao X, Lu L, Duan X, Qin H, Du X, et al. Transcriptomic analysis of different stages of pigeon ovaries by RNA-sequencing. Mol Reprod Dev. (2016) 83:640–8. doi: 10.1002/mrd.22670
15. Zhang T, Chen L, Han K, Zhang X, Zhang G, Dai G, et al. Transcriptome analysis of ovary in relatively greater and lesser egg producing Jinghai yellow chicken. Anim Reprod Sci. (2019) 208:106114. doi: 10.1016/j.anireprosci.2019.106114
16. Hu S, Yang S, Lu Y, Deng Y, Li L, Zhu J, et al. Dynamics of the transcriptome and accessible chromatin landscapes during early goose ovarian development. Front Cell Dev Biol. (2020) 8:1–17. doi: 10.3389/fcell.2020.00196
17. Byrne A, Supple MA, Volden R, Laidre KL, Shapiro B, Vollmers C. Depletion of hemoglobin transcripts and long read sequencing improves the transcriptome annotation of the polar bear (Ursus maritimus). Front Genet. (2019) 10:1–28. doi: 10.3389/fgene.2019.00643
18. Lin J, Guan L, Ge L, Liu G, Bai Y, Liu X. Nanopore-based full-length transcriptome sequencing of Muscovy duck (Cairina moschata) ovary. Poult Sci. (2021) 100:101246. doi: 10.1016/j.psj.2021.101246
19. Jin J, Zhang H, Zhang J, Liu P, Chen X, Li Z, et al. Integrated transcriptomics and metabolomics analysis to characterize cold stress responses in Nicotiana tabacum. BMC Genomics. (2017) 18:1–15. doi: 10.1186/s12864-017-3871-7
20. Son SY, Park YJ, Jung ES, Singh D, Lee YW, Kim JG, et al. Integrated metabolomics and transcriptomics unravel the metabolic pathway variations for different sized beech mushrooms. Int J Mol Sci. (2019) 20:6007. doi: 10.3390/ijms20236007
21. Zhang Y, Zou X, Ding Y, Wang H, Wu X, Liang B. Comparative genomics and functional study of lipid metabolic genes in Caenorhabditis elegans. BMC Genomics. (2013) 14:1. doi: 10.1186/1471-2164-14-164
22. Khan R, Jiang X, Hameed U, Shi Q. Role of lipid metabolism and signaling in mammalian oocyte maturation, quality, and acquisition of competence. Front Cell Dev Biol. (2021) 9:639704. doi: 10.3389/fcell.2021.639704
23. Gu L, Liu H, Gu X, Boots C, Moley KH, Wang Q. Metabolic control of oocyte development: linking maternal nutrition and reproductive outcomes. Cell Mol Life Sci. (2015) 72:251–71. doi: 10.1007/s00018-014-1739-4
24. Dubeibe Marin DF, da Costa NN, di Paula Bessa Santana P, de Souza EB, Ohashi OM, Marín DFD, et al. Importance of lipid metabolism on oocyte maturation and early embryo development: can we apply what we know to buffalo? Anim Reprod Sci. (2019) 211:106220. doi: 10.1016/j.anireprosci.2019.106220
25. Campbell DI, Ferreira CR, Eberlin LS, Cooks RG. Improved spatial resolution in the imaging of biological tissue using desorption electrospray ionization. Anal Bioanal Chem. (2012) 404:389–98. doi: 10.1007/s00216-012-6173-6
26. Kouba S, Ouldamer L, Garcia C, Fontaine D, Chantome A, Vandier C, et al. Lipid metabolism and calcium signaling in epithelial ovarian cancer. Cell Calcium. (2019) 81:38–50. doi: 10.1016/j.ceca.2019.06.002
27. Bai X, Jia J, Kang Q, Fu Y, Zhou Y, Zhong Y, et al. Integrated metabolomics and lipidomics analysis reveal remodeling of lipid metabolism and amino acid metabolism in glucagon receptor–deficient zebrafish. Front Cell Dev Biol. (2021) 8:1–15. doi: 10.3389/fcell.2020.605979
28. Newman JW, Stok JE, Vidal JD, Corbin CJ, Huang Q, Hammock BD, et al. Cytochrome P450-dependent lipid metabolism in pre-ovulatory follicles. Endocrinology. (2004) 145:5097–105. doi: 10.1210/en.2004-0710
29. Gomes RN, Souza F da C, Colquhoun A. Eicosanoids and cancer. Clinics. (2018) 73:1–10. doi: 10.6061/clinics/2018/e530s
30. Takahashi T, Morrow JD, Wang H, Dey SK. Cyclooxygenase-2-derived prostaglandin E2 directs oocyte maturation by differentially influencing multiple signaling pathways. J Biol Chem. (2006) 281:37117–29. doi: 10.1074/jbc.M608202200
31. Fujimori C, Ogiwara K, Hagiwara A, Rajapakse S, Kimura A, Takahashi T. Expression of cyclooxygenase-2 and prostaglandin receptor EP4b mRNA in the ovary of the Medaka fish, Oryzias latipes: possible involvement in ovulation. Mol Cell Endocrinol. (2011) 332:67–77. doi: 10.1016/j.mce.2010.09.015
32. Park CJ, Lin PC, Zhou S, Barakat R, Bashir ST, Choi JM, et al. Progesterone receptor serves the ovary as a trigger of ovulation and a terminator of inflammation. Cell Rep. (2020) 31:107496. doi: 10.1016/j.celrep.2020.03.060
33. Nuttinck F, Marquant-Le Guienne B, Clément L, Reinaud P, Charpigny G, Grimard B. Expression of genes involved in prostaglandin E2 and progesterone production in bovine cumulus-oocyte complexes during in vitro maturation and fertilization. Reproduction. (2008) 135:593–603. doi: 10.1530/REP-07-0453
34. Kikut J, Komorniak N, Zietek M, Palma J, Szczuko M. Inflammation with the participation of arachidonic (AA) and linoleic acid (LA) derivatives (HETEs and HODEs) is necessary in the course of a normal reproductive cycle and pregnancy. J Reprod Immunol. (2020) 141:103177. doi: 10.1016/j.jri.2020.103177
35. Schaible AM, Koeberle A, Northoff H, Lawrenz B, Weinigel C, Barz D, et al. High capacity for leukotriene biosynthesis in peripheral blood during pregnancy. Prostaglandins Leukot. Essent. Fatty Acids. (2013) 89:245–55. doi: 10.1016/j.plefa.2013.06.004
36. Sponchiado M, Gonella-Diaza AM, Rocha CC, Turco EGL, Pugliesi G, Leroy JLMR, et al. The pre-hatching bovine embryo transforms the uterine luminal metabolite composition in vivo. Sci Rep. (2019) 9:1–14. doi: 10.1038/s41598-019-44590-9
37. Sheikh S, Parhar RS, Bakheet R, Saleh S, Collison K, Al-Mohanna F. Immobilization of rolling NK cells on platelet-borne P-selectin under flow by pro-inflammatory stimuli, interleukin-12, and leukotriene B 4. J Leukoc Biol. (2004) 76:603–8. doi: 10.1189/jlb.0204106
38. Tager AM, Bromley SK, Medoff BD, Islam SA, Bercury SD, Friedrich EB, et al. Leukotriene B4 receptor BLT1 mediates early effector T cell recruitment. Nat Immunol. (2003) 4:982–90. doi: 10.1038/ni970
39. Soares EM, Mason KL, Rogers LM, Serezani CH, Faccioli LH, Aronoff DM. Leukotriene B 4 Enhances Innate Immune Defense against the Puerperal Sepsis Agent Streptococcus pyogenes. J. Immunol. (2013) 190:1614–22. doi: 10.4049/jimmunol.1202932
40. Hu J, Zhang Z, Shen WJ, Azhar S. Cellular cholesterol delivery, intracellular processing and utilization for biosynthesis of steroid hormones. Nutr Metab. (2010) 7:7–9. doi: 10.1186/1743-7075-7-47
41. Lebbe M, Taylor AE, Visser JA, Kirkman-Brown JC, Woodruff TK, Arlt W. The steroid metabolome in the isolated ovarian follicle and its response to androgen exposure and antagonism. Endocrinology. (2017) 158:1474–85. doi: 10.1210/en.2016-1851
42. Strauss JF III, FitzGerald GA. Steroid Hormones and Other Lipid Molecules Involved in Human Reproduction. In: Strauss JF III, Barbieri RL, editors. Yen and Jaffe's Reproductive Endocrinology: Physiology, Pathophysiology, and Clinical Management, 8th Edn. Philadelphia, PA: Saunders Elsevier (2019). p. 75–114.
43. Strauss JF III. Organization of Ovarian Steroidogenic Cells and Cholesterol Metabolism. In: Leung PCK, Adashi EY, editors. The Ovary, 3rd Edn. Pittsburgh, PA: Academic Press (2019). p. 83–94.
44. Diaz FJ, Anthony K, Halfhill AN. Early avian follicular development is characterized by changes in transcripts involved in steroidogenesis, paracrine signaling and transcription. Mol Reprod Dev. (2011) 78:212–23. doi: 10.1002/mrd.21288
45. Johnson AL, Lee J. Granulosa cell responsiveness to follicle stimulating hormone during early growth of hen ovarian follicles. Poult Sci. (2016) 95:108–14. doi: 10.3382/ps/pev318
46. Johnson AL, Woods DC. Dynamics of avian ovarian follicle development: cellular mechanisms of granulosa cell differentiation. Gen Comp Endocrinol. (2009) 163:12–7. doi: 10.1016/j.ygcen.2008.11.012
47. Groothuis TGG, Schwabl H. Hormone-mediated maternal effects in birds: mechanisms matter but what do we know of them? Philos Trans R Soc Lond B Biol Sci. (2008) 363:1647–61. doi: 10.1098/rstb.2007.0007
48. Wojtysiak D, Okólski A, Sechman A. Structure and steroidogenic activity of the granulosa layer of F1 pre-ovulatory ovarian follicles of the hen (Gallus domesticus). Folia Biologica. (2011) 59:59–64. doi: 10.3409/fb59_1-2.59-64
49. Okuliarova M, Meddle SL, Zeman M. Egg deposition of maternal testosterone is primarily controlled by the pre-ovulatory peak of luteinizing hormone in Japanese quail. Gen Comp Endocrinol. (2018) 256:23–9. doi: 10.1016/j.ygcen.2017.05.004
50. Nitta H, Mason JI, Bahr JM. Localization of 3β-hydroxysteroid dehydrogenase in the chicken ovarian follicle shifts from the theca layer to granulosa layer with follicular maturation. Biol Reprod. (1993) 48:110–6. doi: 10.1095/biolreprod48.1.110
51. Marrone BL, Sebring RJ. Quantitative cytochemistry of 3 beta-hydroxysteroid dehydrogenase activity in avian granulosa cells during follicular maturation. Biol Reprod. (1989) 40:1007–11. doi: 10.1095/biolreprod40.5.1007
52. Olea GB, Carou MC, Aguirre MV, Lombardo DM. Expression of GnRH receptor and 3βHSD during meiosis and foliculogénesis in Columba livia (Aves: Columbiformes): histological and immunohistochemical analysis. Gen Comp Endocrinol. (2020) 285:113230. doi: 10.1016/j.ygcen.2019.113230
53. George MM, New MI, Ten S, Sultan C, Bhangoo A. The clinical and molecular heterogeneity of 17βHSD-3 enzyme deficiency. Horm Res Paediatr. (2010) 74:229–40. doi: 10.1159/000318004
54. Marchais-Oberwinkler S, Henn C, Möller G, Klein T, Negri M, Oster A, et al. 17β-Hydroxysteroid dehydrogenases (17β-HSDs) as therapeutic targets: protein structures, functions, and recent progress in inhibitor development. J Steroid Biochem Mol Biol. (2011) 125:66–82. doi: 10.1016/j.jsbmb.2010.12.013
55. Verghese. Aromatase, estrone sulfatase, and 17β-hydroxysteroid dehydrogenase: structure-function studies and inhibitor development. Bone. (2011) 23:1–7. doi: 10.1016/j.mce.2010.09.012.Aromatase
56. Strauss JF III, Modi B, McAllister JM. Defects in Ovarian Steroid Hormone Biosynthesis. In: Ulloa-Aguirre A, Conn PM, editors. Cellular Endocrinology in Health and Disease. Pittsburgh, PA: Academic Press (2014). p. 285–309.
57. Jing R, Gu L, Li J, Gong Y. A transcriptomic comparison of theca and granulosa cells in chicken and cattle follicles reveals ESR2 as a potential regulator of CYP19A1 expression in the theca cells of chicken follicles. Comp Biochem Physiol Part D Genomics Proteomics. (2018) 27:40–53. doi: 10.1016/j.cbd.2018.04.002
58. Eichmann TO, Lass A. DAG tales: the multiple faces of diacylglycerol-stereochemistry, metabolism, and signaling. Cell Mol Life Sci. (2015) 72:3931–52. doi: 10.1007/s00018-015-1982-3
59. Ramesh S, Govindarajulu M, Suppiramaniam V, Moore T. Autotaxin–lysophosphatidic acid signaling in Alzheimer's disease. Int J Mol Sci. (2018) 19:1827. doi: 10.3390/ijms19071827
60. Wocławek-Potocka I, Rawińska P, Kowalczyk-Zieba I, Boruszewska D, Sinderewicz E, Waśniewski T, et al. Lysophosphatidic acid (LPA) signaling in human and ruminant reproductive tract. Mediators Inflamm. (2014) 2014:649702. doi: 10.1155/2014/649702
61. Boruszewska D, Torres AC, Kowalczyk-Zieba I, Diniz P, Batista M, Lopes-Da-Costa L, et al. The effect of lysophosphatidic acid during in vitro maturation of bovine oocytes: embryonic development and mRNA abundances of genes involved in apoptosis and oocyte competence. Mediators Inflamm. (2014) 2014:670670. doi: 10.1155/2014/670670
62. Zhang JY, Jiang Y, Lin T, Kang JW, Lee JE, Jin D. Lysophosphatidic acid improves porcine oocyte maturation and embryo development in vitro. Mol Reprod Dev. (2015) 82:66–77. doi: 10.1002/mrd.22447
63. Tokumura A, Kanaya Y, Miyake M, Yamano S, Irahara M, Fukuzawa K. Increased production of bioactive lysophosphatidic acid by serum lysophospholipase D in human pregnancy. Biol Reprod. (2002) 67:1386–92. doi: 10.1095/biolreprod.102.004051
64. Xie Q, Xing Y, Zhou J, Wang L, Wu J, Chian RC. The effect of lysophosphatidic acid-supplemented culture medium on human immature oocytes matured in vitro. Reprod Biol Endocrinol. (2021) 19:1–8. doi: 10.1186/s12958-021-00771-8
65. Ye X. Lysophospholipid signaling in the function and pathology of the reproductive system. Hum Reprod Update. (2008) 14:519–36. doi: 10.1093/humupd/dmn023
66. Drissner D, Kunze G, Callewaert N, Gehrig P, Tamasloukht M, Boller T, et al. Lyso-phosphatidylcholine is a signal in the arbuscular mycorrhizal symbiosis. Science (1979). (2007) 318:265–8. doi: 10.1126/science.1146487
67. Zhang X, Wang T, Song J, Deng J, Sun Z. Study on follicular fluid metabolomics components at different ages based on lipid metabolism. Reprod Biol Endocrinol. (2020) 18:1–8. doi: 10.1186/s12958-020-00599-8
68. Bertevello PS, Teixeira-Gomes AP, Seyer A, Carvalho AV, Labas V, Blache M-CC, et al. Lipid identification and transcriptional analysis of controlling enzymes in bovine ovarian follicle. Int J Mol Sci. (2018) 19:3261. doi: 10.3390/ijms19103261
Keywords: ovulation, Muscovy duck, full-length transcriptome, metabolomics, lipid metabolism
Citation: Lin J, Ge L, Mei X, Niu Y, Chen C, Hou S and Liu X (2022) Integrated ONT Full-Length Transcriptome and Metabolism Reveal the Mechanism Affecting Ovulation in Muscovy Duck (Cairina moschata). Front. Vet. Sci. 9:890979. doi: 10.3389/fvets.2022.890979
Received: 07 March 2022; Accepted: 16 June 2022;
Published: 08 July 2022.
Edited by:
Aline Silva Mello Cesar, University of São Paulo, BrazilReviewed by:
Loan To Nguyen, University of Queensland, AustraliaCopyright © 2022 Lin, Ge, Mei, Niu, Chen, Hou and Liu. This is an open-access article distributed under the terms of the Creative Commons Attribution License (CC BY). The use, distribution or reproduction in other forums is permitted, provided the original author(s) and the copyright owner(s) are credited and that the original publication in this journal is cited, in accordance with accepted academic practice. No use, distribution or reproduction is permitted which does not comply with these terms.
*Correspondence: Shuisheng Hou, aG91c3NAMjYzLm5ldA==; Xiaolin Liu, bGl1eGlhb2xpbkBud2FmdS5lZHUuY24=
Disclaimer: All claims expressed in this article are solely those of the authors and do not necessarily represent those of their affiliated organizations, or those of the publisher, the editors and the reviewers. Any product that may be evaluated in this article or claim that may be made by its manufacturer is not guaranteed or endorsed by the publisher.
Research integrity at Frontiers
Learn more about the work of our research integrity team to safeguard the quality of each article we publish.