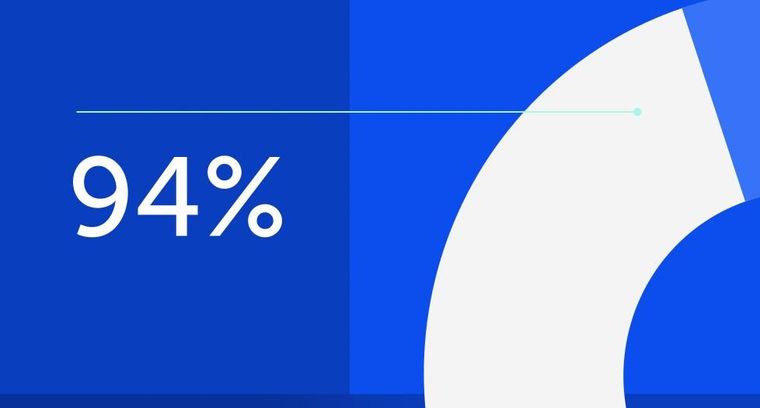
94% of researchers rate our articles as excellent or good
Learn more about the work of our research integrity team to safeguard the quality of each article we publish.
Find out more
HYPOTHESIS AND THEORY article
Front. Vet. Sci., 21 April 2022
Sec. Comparative and Clinical Medicine
Volume 9 - 2022 | https://doi.org/10.3389/fvets.2022.853743
Biological aging is the single most important risk factor for disease, disability, and ultimately death in geriatric dogs. The effects of aging in companion dogs also impose significant financial and psychological burdens on their human caregivers. The underlying physiologic processes of canine aging may be occult, or early signs of aging may be ignored because of the misconception that biological aging is natural and therefore inevitable. The ability to detect, quantify, and mitigate the deleterious processes of canine aging would greatly enhance veterinary preventative medicine and animal welfare. In this paper we propose a new conceptual framework for aging in dogs, the Canine Geriatric Syndrome (CGS). CGS consists of the multiple, interrelated physical, functional, behavioral, and metabolic changes that characterize canine aging as well as the resulting clinical manifestations, including frailty, diminished quality of life, and age-associated disease. We also identify potential key components of a CGS assessment tool, a clinical instrument that would enable veterinarians to diagnose CGS and would facilitate the development and testing of interventions to prolong healthspan and lifespan in dogs by directly targeting the biological mechanisms of aging. There are many gaps in our knowledge of the mechanisms and phenotype of aging in dogs that must be bridged before a CGS assessment tool can be deployed. The conceptual framework of CGS should facilitate identifying these gaps and should stimulate research to better characterize the processes and effects of aging in dogs and to identify the most promising preventative strategies to target these.
After achieving physical and sexual maturity, all dogs experience aging, which can be pragmatically defined as “the progressive accumulation of changes with time associated with or responsible for the ever-increasing susceptibility to disease and death” (1). Aging is the single most important risk factor for a wide variety of superficially unrelated diseases, including neoplasia, osteoarthritis, cardiac disease, neurodegenerative disease, and many others (1, 2). Aging-associated disease severely impacts animal welfare and creates a significant emotional and financial burden for the human caregivers of geriatric dogs (3, 4). Mitigating the impact of aging on morbidity, mortality, and quality of life would address a critical unmet need in veterinary preventative medicine.
The dog is also a particularly appropriate translational model in which to study aging and the interventions intended to prolong lifespan and healthspan (the period of time before the occurrence of significant age-associated disease or disability). Table 1 provides a glossary of geroscience terms used in this article. Unlike traditional laboratory models of aging, such as worms and rodents, companion dogs share living environments and many features of their lifecycle and aging phenotype with humans. They exhibit patterns of age-associated morbidity and mortality that are much more similar to those of humans than other species. However, they also age much more rapidly than humans, making observational and interventional studies of lifespan much more feasible (16–18).
As a species, the dog has exceptional phenotypic and genotypic diversity. This is the result of intensive artificial selection, which has created breeds with lower genetic diversity within each breed and greater differences between breeds than can usually be seen in human populations. This facilitates investigation of genetic variables relevant to differences in lifespan and age-related health outcomes. The extensive annotation of the canine genome further supports such investigation (19).
Companion dogs also typically share the living environment of their human caregivers, including many potential environmental variables that may influence aging and health. Because of their popularity as pets, dogs participate in a large healthcare infrastructure similar to the human healthcare system. This facilitates the collection of extensive data on health risk factors and outcomes in dogs, supporting real-world clinical research on aging mechanisms and interventions. Canine biology and the role of dogs in human society make dogs an excellent translational model for human aging (16–19).
Aging in the dog, as in humans, leads to a loss of robustness (the ability to maintain an optimal state of physiologic functioning) and resilience (the ability to return to this state after perturbations caused by external stressors) (20). Age is traditionally quantified in terms of the passage of time. However, the length of time an organism has been alive (its chronological age) is not the most precise or useful measure of the impact aging has had on an individual's robustness, resilience, or health (its biological age). The two are correlated, but just as species age at different rates, so do individuals within a species. The biological age of a 10-year-old human is much lower than that of a 10-year-old dog even though their chronological age is the same. There are similar, though less dramatic, differences in biological age within a species. Veterinary geroscience currently lacks reliable tools to assess the biological age of individual animals.
A conceptual framework for evaluating age-related changes and a validated clinical assessment tool would allow veterinarians to more effectively assess and manage aging as a risk factor for disease and mortality in individual patients. The purpose of this article is to propose and describe such a framework and to outline potential core components of such a clinical instrument.
We propose the framework of Canine Geriatric Syndrome (CGS) to describe the constellation of key physical, functional, and metabolic changes that characterize aging in dogs and predispose to age-related dysfunction, disease, and death. Diagnosis of CGS would be made using a clinical assessment tool incorporating evaluation of physical function, behavioral and metabolic health, age-associated disease occurrence, frailty, quality of life, and caregiver burden. The components of this tool would include owner and veterinarian assessments, elements from the medical history, physical examination, and routine clinical laboratory testing as well as the use of specific diagnostic instruments to measure age-related change, such as tools for measuring frailty and cognitive function.
Though it is currently impossible to precisely predict the diseases or dysfunction that will result from aging in any individual dog, there are consistent patterns in the manifestations of canine aging. A clearer understanding of the components of the canine aging phenotype would improve our ability to measure biological age and predict health outcomes.
Similarly, there is a complex but limited set of physiologic pathways associated with aging and responsible for its detrimental effects on health and quality of life. Decades of research in multiple species have elucidated many of these pathways and shown them to be very similar across taxa (21–23). Contrary to the common view that aging is inscrutable and inevitable, this research shows that aging is simply another aspect of animal biology, intricate and variable yet comprehensible and amenable to intervention.
While the visible manifestations of aging are readily recognized, the underlying mechanisms often operate for many years before significant changes in appearance and function are detectable. Veterinarians and dog owners are unlikely to be aware of the signs of aging until a relatively late stage. We often dismiss early signs of age-related deterioration as insignificant or “normal,” and therefore not something to assess or treat. The view of aging as a natural and inevitable phenomenon hinders our ability to recognize, monitor, and delay its progression in the clinical setting and to preserve health and function in our aging canine patients.
Use of the CGS framework and associated assessment tool would facilitate earlier identification of individuals at risk for multiple age-related morbidities and mortality, and this assessment would trigger monitoring and intervention to reduce these risks. Such preemptive risk assessment and intervention is already part of veterinary practice, though it is applied to detecting and staging specific diseases rather than as a holistic assessment of aging as a risk factor for multi-morbidity.
A familiar example is the assessment and management of myxomatous mitral valve disease (MMVD). For many years, veterinarians could make a presumptive diagnosis of MMVD based on signalment and physical exam findings. However, without an ability to grade the severity of disease, and without therapeutic interventions shown to delay progression, clinicians were forced to wait until the late-stage onset of congestive heart failure before initiating treatment.
The development of the American College of Veterinary Internal Medicine (ACVIM) staging framework for MMVD (24), and the validation of pimobendan as an effective intervention to delay the onset of CHF in dogs with preclinical MMVD (25), have allowed veterinarians to more effectively identify and mitigate the risk of clinical disease preemptively in dogs with this condition. The CGS framework and clinical assessment could potentially serve a similar function with a much broader scope, staging the impacts of biological aging and facilitating prediction and prevention of the multiple health problems aging causes.
There are conceptual models and associated diagnostic instruments similar to our proposed CGS already in use in human medicine. One of these is Metabolic Syndrome (MS), a set of measurable risk factors for a variety of specific diseases that is used to assess metabolic health and guide preventative clinical interventions (26). The specific components of MS include: visceral obesity, impaired glucose metabolism, dyslipidemia, and hypertension. The syndrome is commonly diagnosed as the presence of three or more of these risk factors (27).
These components of MS are all related to aging, with most occurring more commonly with age across many different human populations (26, 28–30). The elements of MS include both measurable physical exam findings (e.g., waist circumference) and clinical laboratory measurements (e.g., fasting blood glucose). Some components can be defined as clinical disorders in themselves (e.g., hypertension), while others are not considered diseases per se. However, the purpose of assessing these components together is that they represent age-related dysfunction that can signal an increased risk of various clinical disorders, such as heart attacks and stroke, diabetes mellitus, and possibly chronic inflammatory diseases such as rheumatoid arthritis (31) and chronic obstructive pulmonary disease (32).
A CGS diagnostic tool would function similarly to MS, allowing a general assessment of the impact of age-related change on the overall morbidity and mortality risk for canine patients. While some clinical manifestations of aging differ between humans and dogs, many of the core mechanisms operate similarly for both species, and a composite risk assessment strategy would be useful for dogs as it has proven to be for humans.
In order to operationalize the CGS framework and diagnostic instrument, there are many knowledge gaps that must be bridged between the fundamental biology of mammalian aging and the clinical prediction of morbidity and mortality risk in individual dogs. One objective of this paper is to identify areas in which further research is needed to fully develop and refine the CGS concept. Another goal is to identify those knowledge gaps that must be filled in order to design the CGS assessment tool and validate its clinical utility. These gaps represent opportunities for veterinary researchers to advance our understanding of canine aging and support the development of clinical interventions to prolong lifespan and healthspan in dogs.
Research is needed to select specific components of the CGS assessment tool and validate their utility in determining biological age and predicting morbidity and mortality. Some potential components are described below, along with areas for further investigation. Studies linking recognized biological mechanisms of aging to specific clinical manifestations in dogs are also crucial for identifying relevant biomarkers and therapeutic targets. Finally, beyond the biology of aging, a better understanding of the animal welfare consequences of age-related disease in dogs, the extent of the burden these places on human caregivers, and the financial costs of managing age-associated disease in dogs will be invaluable in targeting research efforts and resources most effectively to reduce the negative impacts of aging and age-associated disease.
In addition to the value of CGS in reframing aging as a modifiable risk factor influencing variation in quality of life, frailty, and age-related disease incidence, the ultimate goal is to develop a clinical assessment tool for CGS. The practical implementation of this assessment will require selecting elements of the canine aging phenotype and underlying aging mechanisms that clinicians can readily identify and measure. These elements are likely to come from several broad domains of age-related changes in dogs:
• Physical changes
• Functional changes
• Behavioral changes
• Metabolic changes and biomarker candidates
• Frailty
• Quality of life and caregiver burden
• Age-associated clinical disease
The physical aspects of the canine aging phenotype have been described previously (33). Some of these changes have been shown to be predictive of chronological age, such as the opacification of the lens (34). Others have been shown to be predictive of disease and mortality risk, such as body condition score (35). Still other measurable clinical features of aging in dogs have also been identified in humans as markers of increased risk for disease and death, including visceral adiposity (36) and sarcopenia (37). Finally, there are readily identifiable signs of aging that may or may not correlate with health outcomes, such as graying hair (38, 39).
Including characteristic phenotypic markers of canine aging that can be easily identified in practice and shown to owners during the CGS assessment as evidence of age-related health risk would support more effective preventative care for aging dogs. There have been some attempts to use specific clinical manifestations of aging to predict chronological age, such as lens opacity (40), graying of facial hair (41), and the extent of dental disease (42, 43). However, to date these physical manifestations of aging have only been shown to correlate with chronological age. The goal of the CGS assessment is to measure biological age and identify age-related morbidity and mortality risk. An important gap to be filled in our knowledge of the relationship between physical manifestations of aging and health outcomes is how predictive specific changes are of disease and mortality.
There is some evidence in humans, for example, that common physical manifestations of aging, such as skin wrinkles, hair graying, and even perceived age as rated by others, can predict mortality risk, but the findings are inconsistent (38, 39, 44). Further research in dogs is necessary to clarify the degree to which inclusion of visible physical manifestations of aging would strengthen the clinical utility of the CGS assessment.
Many aspects of physical and behavioral function are key markers of aging and predictors of mortality in humans. Instruments testing physical function, such as the Short Physical Performance Battery (SPPB), and more general assessments of activities of daily living (ADL) are commonly used to assess functional status in humans, and these have been shown to be predictive of mortality (45–47).
Anecdotally, veterinarians and dog owners recognize functional decline in aging dogs, including lower levels of activity, slower walking speed, and difficulty with specific activities, such as climbing stairs or walking on slick surfaces. There are also studies specifically assessing changes in activity with age in dogs. They generally show reductions in spontaneous activity and functional capacity, but the findings are mixed and vary with breed, context, health, and other factors (48–54).
An important functional decline that deserves greater attention is the loss of continence, both fecal and urinary. The relative contributions of behavioral changes, loss of mobility, and decline in the functional competency of the neuromuscular structures involved have not been examined. The implications of this decline for caregiver burden, and the association between incontinence and the risk of euthanasia, is an area ripe for further investigation.
The potential associations between specific activity parameters and lifespan or mortality risk have not been examined in dogs. Given the strong established connection between functional capacity and mortality in humans, this is a critical subject for further investigation in dogs as part of the larger project to develop and operationalize the CGS assessment. Establishing the predictive value of specific changes in activity level would provide a powerful tool for early intervention to extend physical function and lifespan.
Behavioral function also changes significantly with age, in humans and in dogs. There are many challenges in assessing these changes and in delineating the often indistinct boundaries between general aging of the brain, discrete or formally diagnosed neurologic disease, and the behavioral manifestations of aging changes in the rest of the body. For example, an aged dog may be less active due to diminished drive, loss of muscle strength, or musculoskeletal pain, and the relative role of these often concurrent age-related changes can be challenging to disentangle.
Age-associated changes in behavior occur on a continuum, and there is much debate about how we should distinguish normal aging from pathology (55, 56). When behavioral changes in older dogs are attributed to normal aging, veterinarians and owners tend not to pursue further assessment or intervention. Once a formal diagnosis is made, such as Canine Cognitive Dysfunction Syndrome (CDS), then treatment is more likely to be pursued. However, limitations in diagnostic tools for CDS may preclude diagnosis, and treatment options are also limited. Moreover, as in humans, there are likely many potential causes for progressive dementia in dogs, but the clinical, imaging, and histopathological characteristics of conditions other than CDS are poorly described at this time, again limiting our ability to recognize and appropriately treat dementia in dogs.
One purpose of the CGS framework is to encourage the recognition that aging and age-associated changes which diminish function and quality of life should be viewed as targets for prevention and mitigation. Assessing the extent of age-related behavioral change and incorporating this into a global measure of the impact of age on function and resilience will facilitate preventative and palliative measures to limit the effects of aging on both physical and behavioral capacity.
Numerous instruments are commonly used to assess age-associated changes in cognition, mood, and other behavioral domains in humans. Deficits identified by these are often clearly associated with increased mortality risk and diminished quality of life. This holds true even when the impairment does not meet criteria for diagnosis of specific disorders, such as dementia, Alzheimer's disease, or major depression (57–59).
Most instruments used in dogs to assess age-associated behavior change are intended to establish a diagnosis of CDS. These typically ask owners to rate the frequency of behavioral changes related to disorientation, changes in sleep-wake cycle (particularly sleeping during the day and being up during the night), changes in social interactions, new anxiety, and new house soiling. Progression of signs may be most observed in disorientation and social domains (60). In addition to signs of CDS, other behavioral changes that may be seen in aging dogs include pain-related irritable aggression and behavioral changes reflective of declines in sensory capabilities.
Research is limited and unclear about the impact of CDS on survival (61–63). However, the clinical perception among veterinarians is that house soiling and other components of CDS are frequently cited by owners as reasons for euthanasia. More research is needed to determine the impact of age-associated behavioral changes in general, not only CDS, on quality of life and mortality risk in aging dogs. Measures of the behavioral manifestations of aging would be a valuable component of the CGS assessment instrument once the spectrum of changes seen and the impact of these on caregiver burden, quality of life, and mortality are better characterized.
Canine and human aging is characterized by changes in metabolism, and deterioration of metabolic health often precedes age-associated disease and disability. Changes in adipose distribution, insulin sensitivity, and lipid metabolism occur in both humans and dogs. These changes are associated with low grade chronic inflammation and a progressively deteriorating state of metabolic health that represents a key risk factor for age-associated disease in humans and likely in dogs as well. While the role of metabolic dysregulation in association with adverse health outcomes in humans has been well-documented, further research is needed to investigate the utility of metabolic markers in predicting morbidity and mortality risk in the dog. This research will also support the identification and validation of specific biomarkers for metabolic health in dogs that can be incorporated in the CGS definition and assessment.
Despite species differences in the manifestation of metabolic disease, upstream metabolic pathways involved in nutrient and energy metabolism have emerged as core mechanisms contributing to aging across taxa (64, 65). As an example, the metabolic changes seen in overnutrition and obesity are associated with shortened lifespan and increased morbidity and mortality in nematodes, flies, rodents, non-human primates, humans, and dogs (35, 66–68). Influencing these pathways through dietary restriction has proven the most robust intervention to increase lifespan and healthspan by delaying disease onset and mortality in all of these species (67, 69–73).
While metabolic dysregulation is linked with age-associated diseases such as cancer, cardiovascular disease, osteoarthritis, kidney disease, and dementia, early signs of metabolic dysfunction often precede clinical disease (74–78). This suggests that measures of metabolic dysfunction are promising biomarker candidates for earlier detection of at-risk individuals and increased opportunity for preventative interventions. Here we outline key age-associated changes in metabolic physiology observed in dogs that might serve as biomarkers in the CGS assessment. We highlight areas that warrant further investigation in understanding the contribution of metabolic dysregulation to age-associated pathology in dogs and opportunities to validate such potential biomarkers.
As in humans, total body fat (adiposity) increases with age in dogs independent of differences in body condition and nutritional status (79). Adipose tissue undergoes structural changes with age, including change in abundance, distribution (a shifting from subcutaneous to visceral depots), cellular composition, and endocrine signaling (80).
In humans, the age-associated redistribution of adipose from subcutaneous to visceral depots plays a central role in the development of insulin resistance, metabolic dysfunction, inflammation, and impaired regenerative capacity with age (81). A similar increase in the ratio of visceral to subcutaneous fat with age has been recently documented in dogs (82).
The redistribution of adipose in aging dogs is accompanied by functional metabolic changes, including decreased insulin sensitivity, which is a key potential marker of metabolic health in CGS. This is not surprising given that visceral adipose and central obesity is a significant risk factor for insulin resistance in aging humans. The inverse association between increasing adipose tissue and decreasing insulin sensitivity in the dog is well-documented (83, 84). Evidence for a direct causative link between visceral adipose and insulin sensitivity has also been demonstrated in dogs after omentectomy (85). Studies in companion dogs have shown a positive correlation between increasing visceral to subcutaneous fat ratio and fasting insulin levels and a possible association with surrogate indices of insulin sensitivity (86). Further work is needed to assess the validity and utility of such indices in larger samples and trends with age (83, 87, 88).
Insulin sensitivity, as measured by blood glucose clearance, deteriorates with age in both humans and dogs. This has been documented in both cross-sectional (89) as well as longitudinal studies in dogs (67). Although dogs exhibit greater ability to maintain glycemic control, and therefore develop insulin-dependent diabetes mellitus far less commonly than humans, there is substantial evidence that dogs experience hyperinsulinemia in metabolically dysregulated states, such as overweight and obesity (88, 90, 91). Dogs also display increasing basal insulin levels with age (92, 93).
In laboratory dogs, there is extensive literature documenting mechanisms of insulin resistance. Increasing adiposity due to high fat diet, for example, leads to impaired glucose clearance and increased hepatic gluconeogenesis despite hyperinsulinemia (83, 94–97). A 12-year dietary restriction study performed in Labrador Retrievers demonstrated that improved insulin sensitivity (measured by IV glucose tolerance tests) was associated with increased healthspan and lifespan in calorically restricted dogs (67, 93). Dogs with higher insulin sensitivity were at lower risk of dying and needing treatment for chronic disease (93).
A current challenge in incorporating insulin sensitivity as a marker of metabolic health in the CGS assessment is the lack of sufficiently validated tools for assessing insulin sensitivity in companion dogs (87). Due to their simplicity and ease of use, surrogate index approaches based on steady state fasting insulin and glucose levels have been used extensively in human clinical studies (98), and these have also been used as a proxy measurement of insulin resistance in dogs (99–102). However, it is unclear what these surrogate indices represent physiologically when applied to dogs. There is conflicting evidence concerning the correlation of these indices with dynamic measures of glucose tolerance (83, 103), and the model assumptions for these surrogate indices have not been fully evaluated in dogs (98). While using surrogate indices to assess insulin resistance is a potentially powerful approach, further work is needed to determine their reliability and clinical utility in companion dogs.
In addition, further work needs to be done to understand the contribution of decreased insulin sensitivity to age-associated clinical disease. While dogs are not as susceptible to diabetes mellitus as humans, there are many other negative health consequences associated with insulin resistance in humans and animal models, such as cancer, cardiovascular and neurodegenerative disease, osteoarthritis, and kidney disease (74–77, 104). These may also impact aging dogs, and making those connections is key to establishing the utility of this metabolic biomarker and enable earlier intervention to address age-related metabolic dysfunction.
Serum levels of triglycerides, cholesterol, lipoproteins, and free fatty acids change with age in dogs (105). A recent lipidomics study in companion dogs found associations between lipid profiles and body weight (a proxy for estimated lifespan in this species) as well as age (106). Multiple studies have also demonstrated that overweight/obese companion dogs exhibit perturbed lipid profiles (88, 107–109). This is relevant as increased age is a risk factor for overweight and obesity in dogs (110, 111).
Dyslipidemia is a hallmark feature of metabolic dysregulation and a functional consequence of the age-related redistribution of subcutaneous to visceral fat in humans; visceral fat is more lipolytically active and contributes to elevated free fatty acids (FFA), while subcutaneous fat has been shown to act as a sink to decrease circulating FFA (112). Central adiposity is linked with increased lipolysis and hepatic exposure to circulating free fatty acids (FFA) in multiple species, including in the dog, and this is hypothesized to produce hepatic insulin resistance (94, 113, 114).
Another pathophysiological consequence of chronically elevated serum FFA seen in humans and preclinical research studies is the ectopic deposition of lipid in non-adipose tissues, including liver, muscle, pancreas and heart. This lipotoxicity leads to induction of cellular stress and inflammation, immune cell infiltration, impaired tissue function, and cellular senescence (81) and is associated with the pathogenesis of several age-related diseases in humans, including sarcopenia, type 2 diabetes mellitus and fatty liver disease, and cardiac disease (115, 116). A study investigating differences in epaxial musculature in a small sample of young and aged Labrador Retrievers found a negative correlation between degree of CT attenuation and age (117). The authors suggested this may be due to greater muscle fat content, based on the correlation between skeletal muscle CT attenuation and skeletal muscle lipid content found in humans (118). However, there remains a paucity of literature directly examining the prevalence of ectopic lipid deposition with age and the contribution of this phenomenon to tissue dysfunction in the dog; this is an area in which further investigation is needed.
Adipose tissue secretes inflammatory cytokines, such as TNF-alpha and IL-6, as well as adipose-specific factors called adipokines, key signaling peptides that influence metabolic function and the pathophysiology of many obesity-associated diseases (119). Adiponectin, for example, is an anti-inflammatory adipokine that directly promotes insulin sensitivity and has been associated with improved metabolic health outcomes and longevity in humans and rodent models (120).
A recent meta-analysis of 20 canine studies found that serum adiponectin is lower in dogs with poor metabolic health (e.g., obese and overweight) (111). There is limited evidence suggesting adiponectin decreases with age in dogs, paralleling the case in humans (121, 122), but additional studies are needed to confirm this relationship. However, clinical studies have demonstrated preliminary associations between adiponectin and obesity-related cardiac dysfunction and MMVD, and adiponectin is promising as a biomarker for metabolic health and age-associated disease in dogs (123, 124).
Adipokines influence both local and systemic inflammation by activating receptors on immune cells, and alterations of adipokines are associated with metabolic dysregulation and increased inflammatory cytokines (125). The state of aging-associated, low grade systemic inflammation has been labeled “inflammaging,” and this has been shown to be predictive of cardiovascular disease, multimorbidity, and a decline in physical and cognitive function in humans (126).
While dogs experience increased innate immune system activity and decreasing adaptive immune responses with increasing age, as do humans, there has been scant research investigating the role of chronic inflammation as a risk factor for canine age-associated disease (127–129). Preliminary evidence supports a link between cardiac dysfunction and markers of chronic inflammation in dogs (123). An ongoing, longitudinal research project in aging sled dogs is investigating relationships between steady state circulating cytokines and several functional parameters related to general health, physical fitness, and cognition (130). This and future research should help elucidate the links between aging, chronic inflammation, and age-associated disease in dogs. Once these links are established, biomarkers of inflammation may be useful components of the CGS assessment tool.
Frailty is a critical concept in human geriatric medicine. Though there are a variety of definitions, the consensus is that frailty involves the loss of strength and function, diminished resistance to stressors, and increased risk of disease, disability, and death (8). Frailty, along with the development of specific diseases, is a function of the diminished robustness and resilience due to aging. This relationship with diminished robustness and resilience has been demonstrated in human clinical studies by linking measures of frailty to risk of adverse health outcomes (morbidity or mortality) or time to recover function from an adverse event (such as hospitalization) (131). Frailty in humans is recognized as a manifestation of aging that interacts with other age-related changes to influence functional and health outcomes in geriatric people (132). Limited research has shown that frailty is associated with aging in dogs, and measurement of frailty would likely constitute a major component of the CGS assessment (133).
There are a number of commonly used instruments to detect and quantify frailty in humans. These generally fall into two categories: the frailty phenotype (FP) (134) and the frailty index (FI) (135). The frailty phenotype is based on the presence or absence of specific clinical measurements, such as strength and walking speed, energy and activity level, and weight loss. The frailty index is based on a cumulative deficit model and consists of a list of diseases and clinical or laboratory abnormalities converted into a continuous frailty score.
Both types of instruments have been shown to predict the risk of disability and other negative health outcomes in humans, including death. There is controversy over the best measurement approach and choice of component variables, but there is consensus that frailty assessment should include measures of function (136, 137). Assessment of frailty is used to guide clinical interventions, and there is some evidence that this approach can improve patient function and reduce the risk of negative outcomes (8, 9).
Because dogs age more rapidly but in a similar manner to humans, it is expected that the concept of frailty will be useful in this species for predicting risk and guiding clinical interventions. Components of frailty, such as decreased mobility, sarcopenia, increased disease incidence, increased need for acute or chronic medical care, and others are associated with chronological age in dogs as they are in humans. Preliminary studies have been conducted to validate both a FP approach and a Canine Frailty Index (CFI) for dogs (133, 138).
A key research need in canine geroscience is the further development and validation of a pragmatic instrument for clinical assessment of frailty in primary care practice. Having such an instrument that can signal an increased risk of morbidity and mortality in individual patients and be used to assess the impact of interventions to reduce this risk would greatly enhance the efficacy of preventative healthcare for dogs.
Assessment of frailty is itself a composite measure of the impact of aging on health and function. However, current approaches to measure canine frailty focus only on a subset of the critical domains of CGS; physical function and disease incidence. Incorporating a measure of frailty into diagnosis of the CGS along with other components measuring physical, functional, behavioral and metabolic manifestations of aging not directly assessed by the FP or CFI would result in a more robust measure of the impact of aging on health and quality of life.
Quality of life (QoL) is considered a critical subject for assessment in elderly humans. It incorporates the impact of aging in multiple domains, including physical health and function, cognition and mood, and psychosocial aspects of life. Health-related quality of life (HRQL) is also predictive of mortality in elderly humans (57, 139).
Just as dogs experience physical and functional decline with aging, they likely experience changes in quality of life similar to those seen in elderly humans. Limited assessment of health-related quality of life in dogs has demonstrated that several dimensions of HRQL decline with age (140). Quality of life assessment is a key factor in decision-making about euthanasia for dogs (141–144), so there is clearly a relationship between perceived QoL and mortality risk.
While there are a number of studies that have developed and demonstrated the usefulness of HRQL instruments in dogs (145–151), there is no consensus on the most appropriate way to measure changes in QoL with aging, and there are limited tools available for doing so. Dog owners commonly cite loss of appetite and mobility, perceived pain, and incontinence as key variables (144). However, less quantifiable or observable factors, such as loss of energy and apparent enjoyment of normal activities, may also be of importance. The specific changes most salient to the perception of quality of life as acceptable or unacceptable may also vary with context or individual owner values.
Given that QoL often appears to decline with age and is associated with the risk of euthanasia in dogs, establishing standards and robust instruments for assessment of QoL in aging dogs is a critical research need. Quality of life is a crucial component of the CGS instrument, and improving our understanding of this variable and how to measure it is necessary for the clinical implementation of the CGS framework.
The age-related decline in health and function of companion dogs has significant impact on their human caregivers. There are financial and psychological costs to caring for dogs with chronic illness, so the negative consequences of aging on canine health inevitably also affects the wellbeing of dog owners. Measures of caregiver burden show that caring for a pet with chronic or terminal illness is associated with higher levels of stress, anxiety, and depression and with lower quality of life compared with having a healthy pet (3, 4).
The end of life for most dogs is not due to natural disease but to the deliberate choice by owners to request euthanasia (152–154). This directly links the caregiver burden of age-associated disease and dysfunction in dogs to canine longevity patterns. Large and giant breed dogs, for example, are more likely than smaller breeds to die from chronic, age-related musculoskeletal disease (155, 156). This may well be a function of the greater burden caring for large dogs with impaired mobility places on owners, leading to earlier euthanasia.
A better ability to assess and mitigate the effects of aging on health, function, and quality of life in dogs through implementation of the CGS framework will have indirect benefits for humans by reducing the burden of age-associated disease. This, in turn, will further benefit dogs by reducing some of the drivers of euthanasia.
Aging is a key risk factor for many specific diseases in dogs, including most of the leading causes of canine mortality: neoplasia, cardiovascular disease, and degenerative neurologic or musculoskeletal diseases. The molecular and cellular mechanisms of aging that occur in all individuals are the root cause of many diseases that may seem unrelated because they occur in different organ systems or have differing proximate pathophysiologic causes. Aging can be understood as a collection of common root causes of age-related disease with variable, extended latency to clinical manifestation. This results in a heterogeneous pattern of aging phenotypes among individuals influenced by a complex interaction of many factors.
An important component of CGS is the increased incidence of age-associated disease. Despite significant differences between individuals, breeds, and populations in the specific diseases that manifest with increasing age, the overall increased incidence of age-related disease should serve as both a sign of the health effects of age and as a trigger for interventions to prevent, and even reverse, the influence of aging pathways on morbidity. Some measure of this increased risk should be incorporated into any CGS assessment tool.
Accomplishing this will require a more sophisticated and quantitative understanding of the impact of age on the incidence of specific diseases in specific canine populations. Research linking the core mechanisms of aging and the diseases that companion dogs of various breeds experience will strengthen the predictive value of a disease-incidence component to the CGS.
The purpose of the Canine Geriatric Syndrome framework is to facilitate recognition of the significance of aging as a risk factor for negative health outcomes in dogs and to begin development of a clinical assessment tool for veterinarians to identify and characterize the impact of aging on health and mortality risk in individual canine patients. To achieve this goal, specific components for such a CGS diagnostic tool must be identified and then tested to determine their clinical utility and predictive value. Future research is needed to elucidate the relationships between individual morbidity and mortality risk and the components of the CGS assessment, including specific physical and functional characteristics of aging, laboratory markers of aging pathways and metabolic dysfunction, frailty, quality of life, and caregiver burden. Figure 1 illustrates the key components of a CGS tool and the next steps needed to further develop these.
Once this tool is fully developed and validated, it will have multiple uses. Primarily, the diagnosis of CGS will signal the presence of an age-related increase in the risk of negative health outcomes for individual dogs, which can then trigger preventative and palliative interventions. However, CGS assessment will also be invaluable in evaluating the impact of interventions targeting aging pathways. Improvements in a validated measure of canine aging will be a key goal for studies assessing pharmaceutical, dietary, and other interventions to mitigate or lessen the negative impact of aging on health status.
This article has identified some potential components of the CGS and a CGS assessment instrument as well as knowledge gaps that need to be filled in order to operationalize this framework. We hope this serves as a stimulus for a robust, collaborative effort within the veterinary research community to advance both this framework and the larger field of veterinary geroscience.
The original contributions presented in the study are included in the article/supplementary material, further inquiries can be directed to the corresponding author.
FC developed the core concept of the CGS. BM wrote the first draft of the manuscript. FC and BM wrote sections of the manuscript. All authors contributed to manuscript revision and have read and approved the submitted version.
BM and FC are employed by Loyal, a biotechnology company developing drug therapies to extend lifespan and healthspan in dogs.
The remaining authors declare that the research was conducted in the absence of any commercial or financial relationships that could be construed as a potential conflict of interest.
All claims expressed in this article are solely those of the authors and do not necessarily represent those of their affiliated organizations, or those of the publisher, the editors and the reviewers. Any product that may be evaluated in this article, or claim that may be made by its manufacturer, is not guaranteed or endorsed by the publisher.
1. Harman D. The aging process. Proc Natl Acad Sci USA. (1981) 78:7124–8. doi: 10.1073/pnas.78.11.7124
2. Franceschi C, Garagnani P, Morsiani C, Conte M, Santoro A, Grignolio A, et al. The continuum of aging and age-related diseases: common mechanisms but different rates. Front Med. (2018) 5:61. doi: 10.3389/fmed.2018.00061
3. Christiansen SB, Kristensen AT, Sandøe P, Lassen J. Looking after chronically iii dogs: impacts on the caregiver's life. Anthrozoös. (2013) 26:519–33. doi: 10.2752/175303713X13795775536174
4. Spitznagel MB, Jacobson DM, Cox MD, Carlson MD. Caregiver burden in owners of a sick companion animal: a cross-sectional observational study. Vet Rec. (2017) 181:321. doi: 10.1136/vr.104295
5. Karasik D, Demissie S, Cupples LA, Kiel DP. Disentangling the genetic determinants of human aging: biological age as an alternative to the use of survival measures. J Gerontol A Biol Sci Med Sci. (2005) 60:574–87. doi: 10.1093/GERONA/60.5.574
6. Jylhävä J, Pedersen NL, Hägg S. Biological age predictors. EBioMedicine. (2017) 21:29–36. doi: 10.1016/J.EBIOM.2017.03.046
7. Diebel LWM, Rockwood K. Determination of biological age: geriatric assessment vs. biological biomarkers. Curr Oncol Rep. (2021) 23:104. doi: 10.1007/S11912-021-01097-9
8. Hoogendijk EO, Afilalo J, Ensrud KE, Kowal P, Onder G, Fried LP. Frailty: implications for clinical practice and public health. Lancet. (2019) 394:1365–75. doi: 10.1016/S0140-6736(19)31786-6
9. Walston J, Buta B, Xue Q-L. Frailty screening and interventions: considerations for clinical practice. Clin Geriatr Med. (2018) 34:25–38. doi: 10.1016/j.cger.2017.09.004
10. Kennedy BK, Berger SL, Brunet A, Campisi J, Cuervo AM, Epel ES. Geroscience: linking aging to chronic disease. Cell. (2014) 159:709–13. doi: 10.1016/J.CELL.2014.10.039
11. Sierra F. The emergence of geroscience as an interdisciplinary approach to the enhancement of health span and life span. Cold Spring Harb Perspect Med. (2016) 6:a025163. doi: 10.1101/cshperspect.a025163
12. Kaeberlein M. Translational geroscience: a new paradigm for 21st century medicine. Transl Med Aging. (2017) 1:1–4. doi: 10.1016/j.tma.2017.09.004
13. Rowe JW, Kahn RL. Human aging: usual and successful. Science. (1987) 237:143–50. Available online at: https://go.gale.com/ps/i.do?p=HRCA&sw=w&issn=00368075&v=2.1&it=r&id=GALE%7CA5086264&sid=googleScholar&linkaccess=fulltext (accessed November 30, 2021).
14. Kaeberlein M. How healthy is the healthspan concept? GeroScience. (2018) 40:361–4. doi: 10.1007/s11357-018-0036-9
15. Ukraintseva S, Yashin AI, Arbeev KG. Resilience versus robustness in aging. J Gerontol A Biol Sci Med Sci. (2016) 71:1533–4. doi: 10.1093/gerona/glw083
16. Gilmore KM, Greer KA. Why is the dog an ideal model for aging research? Exp Gerontol. (2015) 71:14–20. doi: 10.1016/j.exger.2015.08.008
17. Hoffman JM, Creevy KE, Franks A, O'Neill DG, Promislow DEL. The companion dog as a model for human aging and mortality. Aging Cell. (2018) 17:e12737. doi: 10.1111/acel.12737
18. Jones A, Head E, Greer KA. The dog as a model for aging research. In: Conn's Handbook of Models for Human Aging. Elsevier. (2018) p. 167–76. doi: 10.1016/B978-0-12-811353-0.00014-2
19. Ruple A, MacLean E, Snyder-Mackler N, Creevy KE, Promislow D. Dog models of aging. Annu Rev Anim Biosci. (2021) 10:419–39. doi: 10.1146/annurev-animal-051021-080937
20. Arbeev KG, Ukraintseva SV, Yashin AI. Dynamics of biomarkers in relation to aging and mortality. Mech Ageing Dev. (2016) 156:42–54. doi: 10.1016/j.mad.2016.04.010
21. Sándor S, Kubinyi E. Genetic pathways of aging and their relevance in the dog as a natural model of human aging. Front Genet. (2019) 10:948. doi: 10.3389/fgene.2019.00948
22. López-Otín C, Blasco MA, Partridge L, Serrano M, Kroemer G. The hallmarks of aging. Cell. (2013) 153:1194–217. doi: 10.1016/j.cell.2013.05.039
23. Bartke A, Brannan S, Hascup E, Hascup K, Darcy J. Energy metabolism and aging. World J Mens Health. (2021) 39:222–32. doi: 10.5534/wjmh.200112
24. Keene BW, Atkins CE, Bonagura JD, Fox PR, Häggström J, Fuentes VL, et al. ACVIM consensus guidelines for the diagnosis and treatment of myxomatous mitral valve disease in dogs. J Vet Intern Med. (2019) 33:1127–40. doi: 10.1111/jvim.15488
25. Boswood A, Häggström J, Gordon SG, Wess G, Stepien RL, Oyama MA, et al. Effect of pimobendan in dogs with preclinical myxomatous mitral valve disease and cardiomegaly: The EPIC study—A randomized clinical trial. J Vet Intern Med. (2016) 30:1765–79. doi: 10.1111/jvim.14586
26. Kaur J. A comprehensive review on metabolic syndrome. Cardiol Res Pract. (2014) 2014:943162. doi: 10.1155/2014/943162
27. Alberti KG, Eckel RH, Grundy SM, Zimmet PZ, Cleeman JI, Donato KA, et al. Harmonizing the metabolic syndrome: a joint interim statement of the International Diabetes Federation Task Forceon Epidemiology and Prevention; National Heart, Lung, and Blood Institute; American Heart Association; World HeartFederation; International Atherosclerosis Society; and International Association for the Study of Obesity [Internet]. Obesity Metab. (2010) 7:63–5. doi: 10.14341/2071-8713-5281
28. Hildrum B, Mykletun A, Hole T, Midthjell K, Dahl AA. Age-specific prevalence of the metabolic syndrome defined by the International Diabetes Federation and the National Cholesterol Education Program: the Norwegian HUNT 2 study. BMC Public Health. (2007) 7:220. doi: 10.1186/1471-2458-7-220
29. Jiang B, Zheng Y, Chen Y, Chen Y, Li Q, Zhu C, et al. Age and gender-specific distribution of metabolic syndrome components in East China: role of hypertriglyceridemia in the SPECT-China study. Lipids Health Dis. (2018) 17:92. doi: 10.1186/s12944-018-0747-z
30. Aguilar M, Bhuket T, Torres S, Liu B, Wong RJ. Prevalence of the metabolic syndrome in the United States, 2003-2012. JAMA. (2015) 313:1973–4. doi: 10.1001/jama.2015.4260
31. Medina G, Vera-Lastra O, Peralta-Amaro AL, Jiménez-Arellano MP, Saavedra MA, Cruz-Domínguez MP, et al. Metabolic syndrome, autoimmunity and rheumatic diseases. Pharmacol Res. (2018) 133:277–88. doi: 10.1016/j.phrs.2018.01.009
32. Clini E, Crisafulli E, Radaeli A, Malerba M. COPD and the metabolic syndrome: an intriguing association. Intern Emerg Med. (2013) 8:283–9. doi: 10.1007/s11739-011-0700-x
33. McKenzie BA, Lacroix-Fralish ML, Chen F. The phenotype of aging in the dog: how aging impacts the health and wellbeing of dogs and their caregivers. J Amer Vet Med Assoc. (in Press).
34. Gesierich K, Failing K, Neiger R. Age determination in dogs using ocular light reflection, dental abrasion and tartar. Tierarztl Prax Ausg K Kleintiere Heimtiere. (2015) 43:317–22. doi: 10.15654/TPK-140974
35. Salt C, Morris PJ, Wilson D, Lund EM, German AJ. Association between life span and body condition in neutered client-owned dogs. J Vet Intern Med. (2019) 33:89–99. doi: 10.1111/jvim.15367
36. Kuk JL, Katzmarzyk PT, Nichaman MZ, Church TS, Blair SN, Ross R. Visceral fat is an independent predictor of all-cause mortality in men. Obesity. (2006) 14:336–41. doi: 10.1038/oby.2006.43
37. Xu J, Wan CS, Ktoris K, Reijnierse EM, Maier AB. Sarcopenia is associated with mortality in adults: a systematic review and meta-analysis. Gerontology. (2021) 2021:1–16. doi: 10.1159/000517099
38. ElFaramawy AAA, Hanna IS, Darweesh RM, Ismail AS, Kandil HI. The degree of hair graying as an independent risk marker for coronary artery disease, a CT coronary angiography study. Egypt Heart J. (2018) 70:15–9. doi: 10.1016/j.ehj.2017.07.001
39. Schnohr P, Nyboe J, Lange P, Jensen G. Longevity and gray hair, baldness, facial wrinkles, and arcus senilis in 13,000 men and women: the Copenhagen City Heart Study. J Gerontol A Biol Sci Med Sci. (1998) 53:M347–50. doi: 10.1093/gerona/53A.5.M347
40. Tobias G, Tobias TA, Abood SK. Estimating age in dogs and cats using ocular lens examination. Compendium Continuing Education Practising Veterinarian-North American Edition. (2000) 22:1085–93.
41. Zamansky A, Sinitca AM, Kaplun DI, Dutra LML, Young RJ. Automatic estimation of dog age: the dogage dataset and challenge [Internet]. Lecture Notes in Computer Science. (2019) 2019:421–6. doi: 10.1007/978-3-030-30508-6_34
42. Harvey CE, Shofer FS, Laster L. Association of age and body weight with periodontal disease in North American dogs. J Vet Dent. (1994) 11:94–105. doi: 10.1177/089875649401100301
43. Stella JL, Bauer AE, Croney CC. A cross-sectional study to estimate prevalence of periodontal disease in a population of dogs (Canis familiaris) in commercial breeding facilities in Indiana and Illinois. PLoS ONE. (2018) 13:e0191395. doi: 10.1371/journal.pone.0191395
44. Gunn DA, Larsen LA, Lall JS, Rexbye H, Christensen K. Mortality is Written on the Face. J Gerontol A Biol Sci Med Sci. (2016) 71:72–7. doi: 10.1093/gerona/glv090
45. Pavasini R, Guralnik J, Brown JC, di Bari M, Cesari M, Landi F, et al. Short Physical Performance Battery and all-cause mortality: systematic review and meta-analysis. BMC Med. (2016) 14:215. doi: 10.1186/s12916-016-0763-7
46. Guralnik JM, Simonsick EM, Ferrucci L, Glynn RJ, Berkman LF, Blazer DG, et al. A short physical performance battery assessing lower extremity function: association with self-reported disability and prediction of mortality and nursing home admission. J Gerontol. (1994) 49:M85–94. doi: 10.1093/geronj/49.2.M85
47. Nakazawa A, Nakamura K, Kitamura K, Yoshizawa Y. Association between activities of daily living and mortality among institutionalized elderly adults in Japan. J Epidemiol. (2012) 22:501–7. doi: 10.2188/jea.JE20110153
48. Belshaw Z, Dean R, Asher L. Slower, shorter, sadder: a qualitative study exploring how dog walks change when the canine participant develops osteoarthritis. BMC Vet Res. (2020) 16:85. doi: 10.1186/s12917-020-02293-8
49. Brown DC, Michel KE, Love M, Dow C. Evaluation of the effect of signalment and body conformation on activity monitoring in companion dogs. Am J Vet Res. (2010) 71:322–5. doi: 10.2460/ajvr.71.3.322
50. Siwak CT, Murphey HL, Muggenburg BA, Milgram NW. Age-dependent decline in locomotor activity in dogs is environment specific. Physiol Behav. (2002) 75:65–70. doi: 10.1016/S0031-9384(01)00632-1
51. Siwak CT, Tapp PD, Zicker SC, Murphey HL, Muggenburg BA, Head E, et al. Locomotor activity rhythms in dogs vary with age and cognitive status. Behav Neurosci. (2003) 117:813–24. doi: 10.1037/0735-7044.117.4.813
52. Morrison R, Penpraze V, Greening R, Underwood T, Reilly JJ, Yam PS. Correlates of objectively measured physical activity in dogs. Vet J. (2014) 199:263–7. doi: 10.1016/j.tvjl.2013.11.023
53. Michel KE, Brown DC. Association of signalment parameters with activity of pet dogs. J Nutr Sci. (2014) 3:e28. doi: 10.1017/jns.2014.49
54. Griss S, Riemer S, Warembourg C, Sousa FM, Wera E, Berger-Gonzalez M, et al. If they could choose: How would dogs spend their days? Activity patterns in four populations of domestic dogs. Appl Anim Behav Sci. (2021) 243:105449. doi: 10.1016/j.applanim.2021.105449
55. Salvin HE, McGreevy PD, Sachdev PS, Valenzuela MJ. Growing old gracefully—Behavioral changes associated with “successful aging” in the dog, Canis familiaris. J Vet Behav. (2011) 6:313–20. doi: 10.1016/j.jveb.2011.04.004
56. Szabó D, Miklósi Á, Kubinyi E. Owner reported sensory impairments affect behavioural signs associated with cognitive decline in dogs. Behav Processes. (2018) 157:354–60. doi: 10.1016/j.beproc.2018.07.013
57. Brown DS, Thompson WW, Zack MM, Arnold SE, Barile JP. Associations between health-related quality of life and mortality in older adults. Prev Sci. (2015) 16:21–30. doi: 10.1007/s11121-013-0437-z
58. Boggatz T. Quality of life in old age - a concept analysis. Int J Older People Nurs. (2016) 11:55–69. doi: 10.1111/opn.12089
59. Tsai S-Y, Chi L-Y, Lee C-H, Chou P. Health-related quality of life as a predictor of mortality among community-dwelling older persons. Eur J Epidemiol. (2007) 22:19–26. doi: 10.1007/s10654-006-9092-z
60. Madari A, Farbakova J, Katina S, Smolek T, Novak P, Weissova T, et al. Assessment of severity and progression of canine cognitive dysfunction syndrome using the CAnine DEmentia Scale (CADES). Appl Anim Behav Sci. (2015) 171:138–45. doi: 10.1016/j.applanim.2015.08.034
61. Ruehl WW, DePaoli AC, Bruyette DS. L-deprenyl for treatment of behavioral and cognitive problems in dogs: preliminary report of an open label trial. Appl Anim Behav Sci. (1994) 39:191. doi: 10.1016/0168-1591(94)90146-5
62. Fast R, Schütt T, Toft N, Møller A, Berendt M. An observational study with long-term follow-up of canine cognitive dysfunction: clinical characteristics, survival, and risk factors. J Vet Intern Med. (2013) 27:822–9. doi: 10.1111/jvim.12109
63. Schütt T, Toft N, Berendt M. Cognitive function, progression of age-related behavioral changes, biomarkers, and survival in dogs more than 8 years old. J Vet Intern Med. (2015) 29:1569–77. doi: 10.1111/jvim.13633
64. Cummings NE, Lamming DW. Regulation of metabolic health and aging by nutrient-sensitive signaling pathways. Mol Cell Endocrinol. (2017) 455:13–22. doi: 10.1016/j.mce.2016.11.014
65. Barzilai N, Huffman DM, Muzumdar RH, Bartke A. The critical role of metabolic pathways in aging. Diabetes. (2012) 61:1315–22. doi: 10.2337/db11-1300
66. Musselman LP, Kühnlein RP. Drosophila as a model to study obesity and metabolic disease. J Exp Biol. (2018) 221(Pt Suppl 1). doi: 10.1242/jeb.163881
67. Lawler DF, Larson BT, Ballam JM, Smith GK, Biery DN, Evans RH, et al. Diet restriction and ageing in the dog: major observations over two decades. Br J Nutr. (2008) 99:793–805. doi: 10.1017/S0007114507871686
68. Yue Y, Li S, Shen P, Park Y. Caenorhabditis elegans as a model for obesity research. Curr Res Food Sci. (2021) 4:692–7. doi: 10.1016/j.crfs.2021.09.008
69. Houthoofd K, Vanfleteren JR. The longevity effect of dietary restriction in Caenorhabditis elegans. Exp Gerontol. (2006) 41:1026–31. doi: 10.1016/j.exger.2006.05.007
70. Partridge L, Piper MDW, Mair W. Dietary restriction in Drosophila. Mech Ageing Dev. (2005) 126:938–50. doi: 10.1016/j.mad.2005.03.023
71. Osborne TB, Mendel LB, Ferry EL. The effect of retardation of growth upon the breeding period and duration of life of rats. Science. (1917) 45:294–5. doi: 10.1126/science.45.1160.294
72. McCay CM, Maynard LA, Sperling G, Barnes LL. Retarded growth, life span, ultimate body size and age changes in the albino rat after feeding diets restricted in calories [internet]. J Nutr. (1939) 18:1–13. doi: 10.1093/jn/18.1.1
73. Mattison JA, Colman RJ, Beasley TM, Allison DB, Kemnitz JW, Roth GS, et al. Caloric restriction improves health and survival of rhesus monkeys. Nat Commun. (2017) 8:14063. doi: 10.1038/ncomms14063
74. Ormazabal V, Nair S, Elfeky O, Aguayo C, Salomon C, Zuñiga FA. Association between insulin resistance and the development of cardiovascular disease. Cardiovasc Diabetol. (2018) 17:122. doi: 10.1186/s12933-018-0762-4
75. Arcidiacono B, Iiritano S, Nocera A, Possidente K, Nevolo MT, Ventura V, et al. Insulin resistance and cancer risk: an overview of the pathogenetic mechanisms. Exp Diabetes Res. (2012) 2012:789174. doi: 10.1155/2012/789174
76. Artunc F, Schleicher E, Weigert C, Fritsche A, Stefan N, Häring H-U. The impact of insulin resistance on the kidney and vasculature. Nat Rev Nephrol. (2016) 12:721–37. doi: 10.1038/nrneph.2016.145
77. Tchetina EV, Markova GA, Sharapova EP. Insulin resistance in osteoarthritis: similar mechanisms to type 2 diabetes mellitus. J Nutr Metab. (2020) 2020:4143802. doi: 10.1155/2020/4143802
78. Craft S. Insulin resistance and Alzheimer's disease pathogenesis: potential mechanisms and implications for treatment. Curr Alzheimer Res. (2007) 4:147–52. doi: 10.2174/156720507780362137
79. Kealy RD, Lawler DF, Ballam JM, Mantz SL, Biery DN, Greeley EH, et al. Effects of diet restriction on life span and age-related changes in dogs. J Am Vet Med Assoc. (2002) 220:1315–20. doi: 10.2460/javma.2002.220.1315
80. Palmer AK, Kirkland JL. Aging and adipose tissue: potential interventions for diabetes and regenerative medicine. Exp Gerontol. (2016) 86:97–105. doi: 10.1016/j.exger.2016.02.013
81. Tchkonia T, Morbeck DE, Von Zglinicki T, Van Deursen J, Lustgarten J, Scrable H, et al. Fat tissue, aging, and cellular senescence. Aging Cell. (2010) 9:667–84. doi: 10.1111/j.1474-9726.2010.00608.x
82. Turner RBS, Tyrrell D, Hepworth G, Dunshea FR, Mansfield CS. Compartmental fat distribution in the abdomen of dogs relative to overall body fat composition. BMC Vet Res. (2020) 16:104. doi: 10.1186/s12917-020-02327-1
83. Ader M, Stefanovski D, Richey JM, Kim SP, Kolka CM, Ionut V, et al. Failure of homeostatic model assessment of insulin resistance to detect marked diet-induced insulin resistance in dogs. Diabetes. (2014) 63:1914–9. doi: 10.2337/db13-1215
84. German AJ, Hervera M, Hunter L, Holden SL, Morris PJ, Biourge V, et al. Improvement in insulin resistance and reduction in plasma inflammatory adipokines after weight loss in obese dogs. Domest Anim Endocrinol. (2009) 37:214–26. doi: 10.1016/j.domaniend.2009.07.001
85. Lottati M, Kolka CM, Stefanovski D, Kirkman EL, Bergman RN. Greater omentectomy improves insulin sensitivity in nonobese dogs. Obesity. (2009) 17:674–80. doi: 10.1038/oby.2008.642
86. Müller L, Kollár E, Balogh L, Pöstényi Z, Márián T, Garai I, et al. Body fat distribution and metabolic consequences - Examination opportunities in dogs. Acta Vet Hung. (2014) 62:169–79. doi: 10.1556/avet.2013.057
87. Irvine AJ, Butterwick R, Watson T, Millward DJ, Morgan LM. Determination of insulin sensitivity in the dog: an assessment of three methods. J Nutr. (2002) 132(6 Suppl 2):1706S–8. doi: 10.1093/jn/132.6.1706S
88. Verkest KR, Rand JS, Fleeman LM, Morton JM. Spontaneously obese dogs exhibit greater postprandial glucose, triglyceride, and insulin concentrations than lean dogs. Domest Anim Endocrinol. (2012) 42:103–12. doi: 10.1016/j.domaniend.2011.10.002
89. Strasser A, Niedermüller H, Hofecker G, Laber G. The effect of aging on laboratory values in dogs. Zentralbl Veterinarmed A. (1993) 40:720–30. doi: 10.1111/j.1439-0442.1993.tb00689.x
90. Gilor C, Niessen SJM, Furrow E, DiBartola SP. What's in a name? Classification of diabetes mellitus in veterinary medicine and why it matters. J Vet Intern Med. (2016) 30:927–40. doi: 10.1111/jvim.14357
91. Van Citters GW, Kabir M, Kim SP, Mittelman SD, Dea MK, Brubaker PL, et al. Elevated glucagon-like peptide-1-(7–36)-amide, but not glucose, associated with hyperinsulinemic compensation for fat feeding. J Clin Endocrinol Metab. (2002) 87:5191–8. doi: 10.1210/jc.2002-020002
92. Bhashyam S, Parikh P, Bolukoglu H, Shannon AH, Porter JH, Shen Y-T, et al. Aging is associated with myocardial insulin resistance and mitochondrial dysfunction. Am J Physiol Heart Circulatory Physiol. (2007) 293:H3063–71. doi: 10.1152/ajpheart.00163.2007
93. Larson BT, Lawler DF, Spitznagel EL, Kealy RD. Improved glucose tolerance with lifetime diet restriction favorably affects disease and survival in dogs. J Nutr. (2003) 133:2887–92. doi: 10.1093/jn/133.9.2887
94. Kim SP, Ellmerer M, Van Citters GW, Bergman RN. Primacy of hepatic insulin resistance in the development of the metabolic syndrome induced by an isocaloric moderate-fat diet in the dog. Diabetes. (2003) 52:2453–60. doi: 10.2337/diabetes.52.10.2453
95. Rebrin K, Steil GM, Mittelman SD, Bergman RN. Causal linkage between insulin suppression of lipolysis and suppression of liver glucose output in dogs. J Clin Invest. (1996) 98:741–9. doi: 10.1172/JCI118846
96. Finegood DT, Pacini G, Bergman RN. The insulin sensitivity index. Correlation in dogs between values determined from the intravenous glucose tolerance test and the euglycemic glucose clamp. Diabetes. (1984) 33:362–8. doi: 10.2337/diabetes.33.4.362
97. Kabir M, Catalano KJ, Ananthnarayan S, Kim SP, Van Citters GW, Dea MK, et al. Molecular evidence supporting the portal theory: a causative link between visceral adiposity and hepatic insulin resistance. Am J Physiol Endocrinol Metab. (2005) 288:E454–61. doi: 10.1152/ajpendo.00203.2004
98. Wallace TM, Levy JC, Matthews DR. Use and abuse of HOMA modeling. Diabetes Care. (2004) 27:1487–95. doi: 10.2337/diacare.27.6.1487
99. Schnurr TM, Reynolds AJ, Gustafson SJ, Duffy LK, Dunlap KL. Conditioning causes an increase in glucose transporter-4 levels in mononuclear cells in sled dogs. Int J Biochem Cell Biol. (2014) 55:227–31. doi: 10.1016/j.biocel.2014.09.009
100. Strage EM, Lewitt MS, Hanson JM, Olsson U, Norrvik F, Lilliehöök I, et al. Relationship among insulin resistance, growth hormone, and insulin-like growth factor I concentrations in diestrous Swedish Elkhounds. J Vet Intern Med. (2014) 28:419–28. doi: 10.1111/jvim.12307
101. de Marchi PN, Machado LH de A, Holsback L, Calesso JR, Fagnani R, Zacarias Junior A, et al. Metabolic profile and adipokine levels in overweight and obese dogs. Turk J Vet Anim Sci. (2020) 44:1093–9. doi: 10.3906/vet-2004-44
102. Vitger AD, Stallknecht BM, Miles JE, Hansen SL, Vegge A, Bjørnvad CR. Immunometabolic parameters in overweight dogs during weight loss with or without an exercise program. Domest Anim Endocrinol. (2017) 59:58–66. doi: 10.1016/j.domaniend.2016.10.007
103. Verkest KR, Fleeman LM, Rand JS, Morton JM. Basal measures of insulin sensitivity and insulin secretion and simplified glucose tolerance tests in dogs. Domest Anim Endocrinol. (2010) 39:194–204. doi: 10.1016/j.domaniend.2010.06.001
104. de la Monte SM. Insulin resistance and Alzheimer's disease. BMB Rep. (2009) 42:475–81. doi: 10.5483/BMBRep.2009.42.8.475
105. Kawasumi K, Kashiwado N, Okada Y, Sawamura M, Sasaki Y, Iwazaki E, et al. Age effects on plasma cholesterol and triglyceride profiles and metabolite concentrations in dogs. BMC Vet Res. (2014) 10:57. doi: 10.1186/1746-6148-10-57
106. Hoffman JM, Kiklevich JV, Klavins K, Valencak TG, Austad SN. Alterations of lipid metabolism with age and weight in companion dogs. J Gerontol A Biol Sci Med Sci. (2021) 76:400–5. doi: 10.1093/gerona/glaa186
107. Bailhache E, Nguyen P, Krempf M, Siliart B, Magot T, Ouguerram K. Lipoproteins abnormalities in obese insulin-resistant dogs. Metabolism. (2003) 52:559–64. doi: 10.1053/meta.2003.50110
108. Rafaj R, Kuleš J, Turković V, Rebselj B, Mrljak V, Kučer N. Prospective Hematologic and Biochemical Evaluation of Spontaneously Overweight and Obese dogs. (2016). Available online at: https://www.semanticscholar.org/paper/d8830695afb7addb07e64df81963af28f0f1dd00
109. Tvarijonaviciute A, Ceron JJ, de Torre C, Ljubić BB, Holden SL, Queau Y, et al. Obese dogs with and without obesity-related metabolic dysfunction - a proteomic approach. BMC Vet Res. (2016) 12:211. doi: 10.1186/s12917-016-0839-9
110. Usui S, Yasuda H, Koketsu Y. Characteristics of obese or overweight dogs visiting private Japanese veterinary clinics. Asian Pac J Trop Biomed. (2016) 6:338–43. doi: 10.1016/j.apjtb.2016.01.011
111. Muñoz-Prieto A, Cerón JJ, Martínez-Subiela S, Mrljak V, Tvarijonaviciute A. A systematic review and meta-analysis of serum adiponectin measurements in the framework of dog obesity. Animals. (2020) 10:91650. doi: 10.3390/ani10091650
112. Ibrahim MM. Subcutaneous and visceral adipose tissue: structural and functional differences. Obes Rev. (2010) 11:11–8. doi: 10.1111/j.1467-789X.2009.00623.x
113. Gayet C, Bailhache E, Dumon H, Martin L, Siliart B, Nguyen P. Insulin resistance and changes in plasma concentration of TNFalpha, IGF1, and NEFA in dogs during weight gain and obesity. J Anim Physiol Anim Nutr. (2004) 88:157–65. doi: 10.1111/j.1439-0396.2003.00473.x
114. Broussard JL, Kolka CM, Castro AVB, Asare Bediako I, Paszkiewicz RL, Szczepaniak EW, et al. Elevated nocturnal NEFA are an early signal for hyperinsulinaemic compensation during diet-induced insulin resistance in dogs. Diabetologia. (2015) 58:2663–70. doi: 10.1007/s00125-015-3721-6
115. van Herpen NA, Schrauwen-Hinderling VB. Lipid accumulation in non-adipose tissue and lipotoxicity. Physiol Behav. (2008) 94:231–41. doi: 10.1016/j.physbeh.2007.11.049
116. Britton KA, Fox CS. Ectopic fat depots and cardiovascular disease. Circulation. (2011) 124:e837–41. doi: 10.1161/CIRCULATIONAHA.111.077602
117. Sutherland-Smith J, Hutchinson D, Freeman LM. Comparison of computed tomographic attenuation values for epaxial muscles in old and young dogs. Am J Vet Res. (2019) 80:174–7. doi: 10.2460/ajvr.80.2.174
118. Goodpaster BH, Kelley DE, Thaete FL, He J, Ross R. Skeletal muscle attenuation determined by computed tomography is associated with skeletal muscle lipid content. J Appl Physiol. (2000) 89:104–10. doi: 10.1152/jappl.2000.89.1.104
119. Fasshauer M, Blüher M. Adipokines in health and disease. Trends Pharmacol Sci. (2015) 36:461–70. doi: 10.1016/j.tips.2015.04.014
120. Iwabu M, Okada-Iwabu M, Yamauchi T, Kadowaki T. Adiponectin/adiponectin receptor in disease and aging. NPJ Aging Mech Dis. (2015) 1:15013. doi: 10.1038/npjamd.2015.13
121. Mori A, Lee P, Takemitsu H, Sako T, Arai T. Comparison of insulin signaling gene expression in insulin sensitive tissues between cats and dogs. Vet Res Commun. (2009) 33:211–26. doi: 10.1007/s11259-008-9168-1
122. Damoiseaux C, Merveille A-C, Krafft E, Da Costa AM, Gomart S, Jespers P, et al. Effect of physiological determinants and cardiac disease on plasma adiponectin concentrations in dogs. J Vet Intern Med. (2014) 28:1738–45. doi: 10.1111/jvim.12433
123. Tropf M, Nelson OL, Lee PM, Weng HY. Cardiac and metabolic variables in obese dogs. J Vet Intern Med. (2017) 31:1000–7. doi: 10.1111/jvim.14775
124. Kim H-S, Kang J-H, Jeung E-B, Yang M-P. Serum concentrations of Leptin and adiponectin in dogs with myxomatous mitral valve disease. J Vet Intern Med. (2016) 30:1589–600. doi: 10.1111/jvim.14570
125. Ouchi N, Parker JL, Lugus JJ, Walsh K. Adipokines in inflammation and metabolic disease. Nat Rev Immunol. (2011) 11:85–97. doi: 10.1038/nri2921
126. Ferrucci L, Fabbri E. Inflammageing: chronic inflammation in ageing, cardiovascular disease, and frailty. Nat Rev Cardiol. (2018) 15:505–22. doi: 10.1038/s41569-018-0064-2
127. Day MJ. Ageing, immunosenescence and inflammageing in the dog and cat. J Comp Pathol. (2010) 142 Suppl 1:S60–9. doi: 10.1016/j.jcpa.2009.10.011
128. Jimenez AG, Downs CJ, Lalwani S, Cipolli W. Cellular metabolism and IL-6 concentrations during stimulated inflammation in small and large dog breeds' primary fibroblasts cells, as they age. J Exp Biol. (2020) 224:jeb233734. doi: 10.1242/jeb.233734
129. Alexander JE, Colyer A, Haydock RM, Hayek MG, Park J. Understanding how dogs age: longitudinal analysis of markers of inflammation, immune function, and oxidative stress. J Gerontol A Biol Sci Med Sci. (2018) 73:720–8. doi: 10.1093/gerona/glx182
130. Fleyshman DI, Wakshlag JJ, Huson HJ, Loftus JP, Olby NJ, Brodsky L, et al. Development of infrastructure for a systemic multidisciplinary approach to study aging in retired sled dogs. Aging. (2021) 13:21814–37. doi: 10.18632/aging.203600
131. Hatheway OL, Mitnitski A, Rockwood K. Frailty affects the initial treatment response and time to recovery of mobility in acutely ill older adults admitted to hospital. Age Ageing. (2017) 46:920–5. doi: 10.1093/ageing/afw257
132. Gemikonakli G, Mach J, Hilmer SN. Interactions between the aging gut microbiome and common geriatric giants: polypharmacy, frailty, and dementia. J Gerontol A Biol Sci Med Sci. (2021) 76:1019–28. doi: 10.1093/gerona/glaa047
133. Banzato T, Franzo G, Di Maggio R, Nicoletto E, Burti S, Cesari M, et al. A Frailty Index based on clinical data to quantify mortality risk in dogs. Sci Rep. (2019) 9:16749. doi: 10.1038/s41598-019-52585-9
134. Fried LP, Tangen CM, Walston J, Newman AB, Hirsch C, Gottdiener J, et al. Frailty in older adults: evidence for a phenotype. J Gerontol A Biol Sci Med Sci. (2001) 56:M146–56. doi: 10.1093/gerona/56.3.M146
135. Rockwood K, Mitnitski A. Frailty in relation to the accumulation of deficits. J Gerontol A Biol Sci Med Sci. (2007) 62:722–7. doi: 10.1093/gerona/62.7.722
136. Howlett SE, Rutenberg AD, Rockwood K. The degree of frailty as a translational measure of health in aging. Nature Aging. (2021) 1:651–65. doi: 10.1038/s43587-021-00099-3
137. Fried LP, Cohen AA, Xue Q-L, Walston J, Bandeen-Roche K, Varadhan R. The physical frailty syndrome as a transition from homeostatic symphony to cacophony. Nat Aging. (2021) 1:36–46. doi: 10.1038/s43587-020-00017-z
138. Hua J, Hoummady S, Muller C, Pouchelon J-L, Blondot M, Gilbert C, et al. Assessment of frailty in aged dogs. Am J Vet Res. (2016) 77:1357–65. doi: 10.2460/ajvr.77.12.1357
139. Dominick KL, Ahern FM, Gold CH, Heller DA. Relationship of health-related quality of life to health care utilization and mortality among older adults. Aging Clin Exp Res. (2002) 14:499–508. doi: 10.1007/BF03327351
140. Rodger S, Scott EM, Nolan A, Wright AK, Reid J. Effect of age, breed, and sex on the health-related quality of life of owner assessed healthy dogs. Front Vet Sci. (2021) 8:603139. doi: 10.3389/fvets.2021.603139
141. Mallery KF, Freeman LM, Harpster NK, Rush JE. Factors contributing to the decision for euthanasia of dogs with congestive heart failure. J Am Vet Med Assoc. (1999) 214:1201–4.
142. McMullen SL, Clark WT, Robertson ID. Reasons for the euthanasia of dogs and cats in veterinary practices. Austr Vet Practitioner. (2001) 31:80–4.
143. Bussolari CJ, Habarth J, Katz R, Phillips S, Carmack B, Packman W. The euthanasia decision-making process: A qualitative exploration of bereaved companion animal owners. Bereave Care. (2018) 37:101–8. doi: 10.1080/02682621.2018.1542571
144. Marchitelli B, Shearer T, Cook N. Factors contributing to the decision to euthanize: diagnosis, clinical signs, and triggers. Vet Clin North Am Small Anim Pract. (2020) 50:573–89. doi: 10.1016/j.cvsm.2019.12.007
145. Yam PS, Butowski CF, Chitty JL, Naughton G, Wiseman-Orr ML, Parkin T, et al. Impact of canine overweight and obesity on health-related quality of life. Prev Vet Med. (2016) 127:64–9. doi: 10.1016/j.prevetmed.2016.03.013
146. Davies V, Reid J, Wiseman-Orr ML, Scott EM. Optimising outputs from a validated online instrument to measure health-related quality of life (HRQL) in dogs. PLoS ONE. (2019) 14:e0221869. doi: 10.1371/journal.pone.0221869
147. Belshaw Z, Asher L, Harvey ND, Dean RS. Quality of life assessment in domestic dogs: An evidence-based rapid review. Vet J. (2015) 206:203–12. doi: 10.1016/j.tvjl.2015.07.016
148. Freeman LM, Rush JE, Clark MA, Bulmer BJ. Validation and preliminary data from a health-related quality of life questionnaire for owners of dogs with cardiac disease. J Vet Intern Med. (2020) 34:1119–26. doi: 10.1111/jvim.15791
149. Reid J, Wiseman-Orr L, Scott M. Shortening of an existing generic online health-related quality of life instrument for dogs. J Small Anim Pract. (2018) 59:334–42. doi: 10.1111/jsap.12772
150. Lynch S, Savary-Bataille K, Leeuw B, Argyle DJ. Development of a questionnaire assessing health-related quality-of-life in dogs and cats with cancer. Vet Comp Oncol. (2011) 9:172–82. doi: 10.1111/j.1476-5829.2010.00244.x
151. Schofield I, O'Neill DG, Brodbelt DC, Church DB, Geddes RF, Niessen SJM. Development and evaluation of a health-related quality-of-life tool for dogs with Cushing's syndrome. J Vet Intern Med. (2019) 33:2595–604. doi: 10.1111/jvim.15639
152. O'Neill DG, Church DB, McGreevy PD, Thomson PC, Brodbelt DC. Longevity and mortality of owned dogs in England. Vet J. (2013) 198:638–43. doi: 10.1016/j.tvjl.2013.09.020
153. Michell AR. Longevit of British breeds of dog and its relationships with-sex, size, cardiovascular variables and disease. Vet Rec. (1999) 145:625–9. doi: 10.1136/vr.145.22.625
154. Pegram C, Gray C, Packer RMA, Richards Y, Church DB, Brodbelt DC, et al. Proportion and risk factors for death by euthanasia in dogs in the UK. Sci Rep. (2021) 11:9145. doi: 10.1038/s41598-021-88342-0
155. Fleming JM, Creevy KE, Promislow DEL. Mortality in north American dogs from 1984 to 2004: an investigation into age-, size-, and breed-related causes of death. J Vet Intern Med. (2011) 25:187–98. doi: 10.1111/j.1939-1676.2011.0695.x
Keywords: aging, lifespan, healthspan, quality of life, caregiver burden
Citation: McKenzie BA, Chen FL, Gruen ME and Olby NJ (2022) Canine Geriatric Syndrome: A Framework for Advancing Research in Veterinary Geroscience. Front. Vet. Sci. 9:853743. doi: 10.3389/fvets.2022.853743
Received: 13 January 2022; Accepted: 23 March 2022;
Published: 21 April 2022.
Edited by:
Anna Katharine Shoveller, University of Guelph, CanadaReviewed by:
Karyn L. Hamilton, Colorado State University, United StatesCopyright © 2022 McKenzie, Chen, Gruen and Olby. This is an open-access article distributed under the terms of the Creative Commons Attribution License (CC BY). The use, distribution or reproduction in other forums is permitted, provided the original author(s) and the copyright owner(s) are credited and that the original publication in this journal is cited, in accordance with accepted academic practice. No use, distribution or reproduction is permitted which does not comply with these terms.
*Correspondence: Brennen A. McKenzie, YnJlbm5lbkBsb3lhbGZvcmRvZ3MuY29t
†These authors have contributed equally to this work and share first authorship
Disclaimer: All claims expressed in this article are solely those of the authors and do not necessarily represent those of their affiliated organizations, or those of the publisher, the editors and the reviewers. Any product that may be evaluated in this article or claim that may be made by its manufacturer is not guaranteed or endorsed by the publisher.
Research integrity at Frontiers
Learn more about the work of our research integrity team to safeguard the quality of each article we publish.