- 1College of Animal Science and Technology, Yangzhou University, Yangzhou, China
- 2International Centre for Agricultural Research in the Dry Areas, Addis Ababa, Ethiopia
- 3Joint International Research Laboratory of Agriculture and Agri-Product Safety of Ministry of Education of China, Yangzhou University, Yangzhou, China
It has long been recognized that enterotoxigenic Escherichia coli (ETEC) is the major pathogen responsible for vomiting and diarrhea. E. coli F17, a main subtype of ETEC, is characterized by high morbidity and mortality in young livestock. However, the transcriptomic basis underlying E. coli F17 infection has not been fully understood. In the present study, RNA sequencing was conducted to explore the expression profiles of mRNAs and long non-coding RNAs (lncRNAs) in the jejunum of lambs who were identified as resistant or sensitive to E. coli F17 that was obtained in a challenge experiment. A total of 772 differentially expressed (DE) mRNAs and 190 DE lncRNAs were detected between the E. coli F17—resistance and E. coli F17-sensitive lambs (i.e., TFF2, LOC105606142, OLFM4, LYPD8, REG4, APOA4, TCONS_00223467, and TCONS_00241897). Then, a two-step machine learning approach (RX) combination Random Forest and Extreme Gradient Boosting were performed, which identified 16 mRNAs and 17 lncRNAs as potential biomarkers, within which PPP2R3A and TCONS_00182693 were prioritized as key biomarkers involved in E. coli F17 infection. Furthermore, functional enrichment analysis showed that peroxisome proliferator-activated receptor (PPAR) pathway was significantly enriched in response to E. coli F17 infection. Our finding will help to improve the knowledge of the mechanisms underlying E. coli F17 infection and may provide novel targets for future treatment of E. coli F17 infection.
Introduction
Diarrhea is the most commonly reported disease associated with infection by a complex mixture of bacteria in young animals. Among them, Escherichia coli (E. coli) is the major pathogenic bacterium responsible for diarrhea (1). Pathogenic E. coli have been divided into five pathotypes based on the virulence properties and clinical signs of the host: enterotoxigenic E. coli (ETEC), enterohemorrhagic E. coli (EHEC), enteropathogenic E. coli (EPEC), enteroinvasive E. coli (EIEC), and diffusely enteroadherent E. coli [DAEC, (2)].
Among these pathotypes, ETEC has been identified as the major agent of E. coli-related diarrhea (3–6). Mechanistically, ETEC adheres to intestinal epithelial cells (IECs), leading to the production and replication of enterotoxins (7). Clinical reports revealed that ETEC infection exhibits enteropathogenicity and enterotoxigenicity, causing increased mortality and clinical signs such as severe vomiting and diarrhea (8). The fimbrial adhesins, F5 (9), F17 (10), F18 (11), and F41 (12) are associated with ETEC, mainly in young animals. E. coli F17, one of the main subtypes of ETEC, has been reported as the major pathogen associated with ETEC-related diarrhea worldwide and is responsible for high morbidity and mortality (13–15). The growing prevalence of E. coli F17 has renewed the sense of urgency for E. coli F17 research.
Over 100,000 long non-coding RNAs (lncRNAs) have now been identified, and although the roles of most of them are still unknown, lncRNAs have been shown to play key roles in gene regulation and cellular functions (16). Increasing evidence has shown that lncRNAs contribute to immune activity at multiple levels during E. coli infection. For example, lncRNA-TUB was shown to mediate E. coli-induced inflammatory factor secretion and Staphylococcus aureus adhesion to epithelial cells (17), and lncRNA-XIST was found to mediate E. col-induced inflammatory response in bovine mastitis (18). These data indicate that how specific lncRNAs can regulate E. coli infection. However, remarkably few comparative studies of the roles of lncRNAs and mRNAs in E. coli infections, especially E. coli F17, have been conducted.
For this study, lambs that were resistant or sensitive to E. coli F17 were obtained in a challenge experiment. RNA sequencing (RNA-Seq) was performed to obtain the transcriptomic profiles. Then, differential expression analysis, machine learning analysis, integrative network, and functional enrichment analyses were performed for a deep insight into lncRNA and mRNA in response to E. coli F17 infection. Our results will help to improve the knowledge of the mechanisms underlying E. coli F17 infection and may provide novel targets for future treatment of E. coli F17 infection.
Materials and Methods
Ethics Approval
All the lamb experimental procedures used in the study were reviewed and approved by the Experimental Animal Welfare and Ethical of Institute of Animal Science, Yangzhou University (No: NFNC2020-NFY-6), and were performed in accordance with the Regulations for the Administration of Affairs Concerning Experimental Animals approved by the State Council of the People's Republic of China.
Sample Collection
All experimental lambs were supplied by the Xilaiyuan Agriculture Co., Ltd. (Jiangsu Providence, China). E. coli F17-resistant and E. coli F17-sensitive lambs were detected from a challenge experiment of E. coli F17 (DN1401, fimbrial structural subunit: F17b, fimbrial adhesin subunit: Subfamily II adhesins, originally isolated from diarrheic calves) as described in our previous report (19).
Briefly, 50 healthy newborn lambs were randomly selected and reared on lamb milk replacer free of antimicrobial additives and free of probiotics from when they were 1 day old to 3 days old. At 3 days after birth, lambs were divided into high-dose and low-dose challenge groups. Lambs in the high-dose and low-dose challenge groups were orally gavaged with 50.0 and 1.0 ml of actively growing culture of E. coli F17 (1 × 109 CFU/ ml) for 4 days, respectively. Then, 10 healthy lambs in the high-dose challenge group (antagonism candidate group) and 10 lambs with severe diarrhea in low-dose challenge group (sensitive candidate group, evaluate via stool consistency scoring) were euthanized by administering pentobarbital overdose. Histopathological examination and bacteria plate counting of the intestinal contents were conducted to evaluate the severity of the diarrhea. Results showed that severe pathological intestinal tissues were observed in E. coli F17-sensitive candidate lambs, while relatively healthy intestinal tissue were observed in E. coli F17-antagonism candidate lambs. Intestinal contents bacteria plate counting demonstrated that bacteria in the intestinal contents of E. coli F17-sensitive candidate lambs (1.22*109 on average) were significantly higher than that of E. coli F17-antagonism candidate lambs (3.37*107 on average). Detailed results of intestinal histopathological detection and bacterial counting can be found in in our previous report (19).
Finally, six healthy lambs with mild intestinal pathology in the high-dose challenge group (antagonism group, AN) and six lambs with severe diarrhea in the low-dose challenge group (sensitive group, SE) with severe intestinal pathology were selected. Proximal jejunum tissue was collected and snap-frozen in liquid nitrogen for RNA isolation.
RNA Extraction and Sequencing
Ribonucleic acid was extracted from the jejunum tissue using TRIzol® per the manufacturer's instructions (Invitrogen, Carlsbad, CA, USA). The quality of the extracted RNA was determined using an RNA Nano 6000 Assay Kit, and RNA integrity number (RIN) was obtained using an Agilent 2100 Bioanalyzer with RIN ≥ 8.0 as the threshold.
The mRNA and lncRNA libraries were constructed using a NEB Next® Ultra™ RNA Library Prep Kit for Illumina® per the manufacturer's instructions (NEB, Ipswich, MA, USA). The RNA libraries were sequenced on an Illumina HiSeqTM 2500 platform with PE150 strategy (paired-end 150 bp) by Beijing Novogene Technology Co., Ltd (Beijing, China).
Sequencing Data Analysis
The raw reads were obtained in FASTQ format. Low-quality reads, namely, reads with adapters, reads that contained N (wherein the proportion of unidentified bases > 0.2%), and low-quality reads (quality scores < Q20; i.e., bases with sQ ≤ 5 more than 50% of all reads) were removed. Clean reads were generated and then mapped to the Ovis aries reference genome (Oar_v4.0) using Hisat2 (20). StringTie (21) was used to assemble the mRNA transcripts. Then, coding and non-coding RNA candidates from the transcripts were distinguished using Coding-Non-Coding-Index [CNCI, (22)], Coded Potential Calculator-2 [CPC2, (23)], and Pfam-scan [PFAM, (24)] software. Non-coding RNA candidates with lengths > 200 nt, and with exon numbers ≥ 2 were identified as candidate lncRNAs.
The expected number of Fragments Per Kilobase of transcript sequence per Million fragments sequenced [FPKM, (25)] was used to estimate the expression levels of candidate lncRNA and mRNA transcripts. Differentially expressed (DE) lncRNAs and DE mRNAs were identified between AN and SE groups using edgeR R library (26). lncRNAs and mRNAs were considered significantly DE as the threshold of padj (p-values adjusted by Benjamini and Hochberg's approach) < 0.01.
Identification of mRNA/lncRNA Biomarkers Using Machine Learning Method
To identify potential lncRNA and mRNA biomarkers for E. coli F17 infection, one two-step machine learning approach (RX) combination Random Forest [RF, (27)] and Extreme Gradient Boosting [XGBoost, (28)] were performed. The randomForest library and XGBoost library in R software was applied for the analysis. The detailed strategy for RX were described in our previous research (29).
Briefly, we systematically examined a range of parameters (Ntree and mtry values for RF, colsample and eta for XGBoost), and out-of-bag (OOB) error rate was used for determining the derive minimum hyperparameter values required for final analysis. For biomarkers identification, firstly, RF was applied to select the subset of lncRNAs and mRNAs with positive values of variable important measures (VIMs). Then, these selected lncRNAs and mRNAs from RF were further assessed by XGBoost. Similarly, XGBoost produces a VIM rank for the genes named “Gain.” In the current study, the VIM value of individual variable (mRNA and lncRNA) denotes the relative contribution of the variable for each tree in the model. The higher the “Gain” value, the more important the variable is for generating a classification between lambs AN and SE lambs. Hence, variable with a high “Gain” were therefore prioritized as potential mRNA/lncRNA biomarkers for E. coli F17 infection.
Integrative Network Analysis
To elucidate the interaction between the mRNAs and lncRNAs, cis- and trans-target genes of lncRNA were predicted. Coding genes located 100 kb upstream or downstream of the corresponding lncRNAs were considered cis-target genes. To identify candidate trans-target genes, Pearson correlation coefficients were calculated between the expression level of coding genes and corresponding lncRNAs. Coding genes were considered trans-target genes for |correlation| ≥ 0.95.
Based on the target gene prediction, the interactions between DE lncRNAs and DE mRNAs were used to construct the lncRNA-mRNA integrative network using Cytoscape v3.7.2 software (30).
Functional Analysis
Gene Ontology (GO) and Kyoto Encyclopedia of Genes and Genomes (KEGG) enrichment analyses were performed for the DE mRNAs and target genes of the DE lncRNAs GOseq R library (31) and KO-Based Annotation System (KOBAS) programs (32), followed by a Fisher's exact test with a false discovery rate (FDR) multiple test correction to assess the statistical significance (p < 0.05).
Real-Time QPCR
To validate the RNA-Seq data, five mRNAs and five lncRNAs were randomly selected. The house-keeping gene GAPDH was selected as the reference gene, and the primers were designed using Primer Premier 5 software. The sequences of the selected mRNAs and lncRNAs were shown in Supplementary Table S1.
Total RNA was extracted from the jejunum tissue of 12 lambs (6 AN and 6 SE) processed for sequencing using TRIzol® per the manufacturer's instructions (Invitrogen, Carlsbad, CA, USA). The first strand of cDNA was prepared using FastKing gDNA Dispelling RT per the manufacturer's instructions (Vazyme Biotech, Nanjing, Jiangsu, China).
The PCR thermocycler program procedure was as follows: 37°C for 15 min, followed by 85°C for 5 s. The reaction mixture contained 4.0 μl 5× FastKing-RT SuperMix 2.0 μl, 2.0 μg Total RNA, and RNase-free ddH2O to a total volume to 20 μl. The quality of the cDNA was evaluated by housekeeping gene amplification and stored at −20°C until use.
Real-time qPCR was performed in triplicate with cDNA to validate the reliability of RNA-Seq data following the SYBR Green I method with 1 cycle at 95°C for 15 min, followed by 40 cycles at 95°C for 10 s, and 60°C for 30 s. The dissociation curve was analyzed after amplification.
The 2−ΔΔCt method (33) was used to calculate expression level of selected lncRNAs and mRNAs. The results were shown as relative expression level (log2FoldChange mean ± standard error) using GraphPad Prism 6 software.
Results
Global RNA-Seq Data
The average numbers of raw reads were 85,523,999 (AN) and 84,450,970 (SE); the average numbers of clean reads were 84,384,636 (AN), and 83,112,267 (SE); and the average mapping rates for the AN and SE were 98.67 and 98.41%, respectively. Detailed characteristics of the two libraries are shown in Table 1.
We identified a total of 20,601 mRNAs and 12,426 lncRNAs, of which 9,148 of the lncRNAs were novel and 3,278 were annotated lncRNAs (Figure 1A). Among the novel lncRNAs, 53.2, 27.6, and 19.1% were identified as lncRNAs, sense-overlapping lncRNAs, and antisense lncRNAs, respectively (Figure 1B). The 20,601 mRNAs and 12,426 lncRNAs were screened for in-depth analyses.
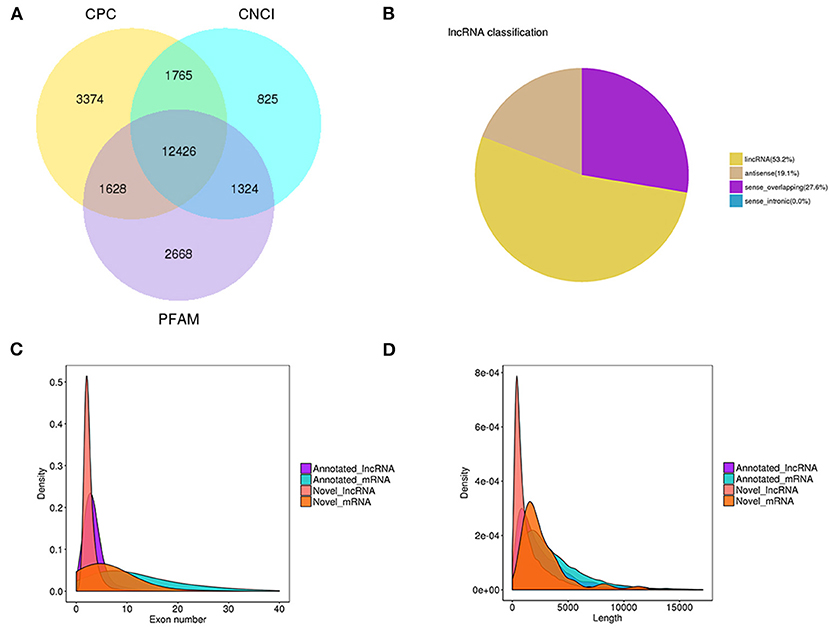
Figure 1. lncRNA filter/classification and exon/length distribution of identified lncRNAs and mRNAs. (A) Filter of identified lncRNAs. (B) Classification of identified lncRNAs. (C) Exon number distribution of identified lncRNAs and mRNAs. (D) Length distribution of identified lncRNAs and mRNAs.
The mRNAs had an average of 5.76 exons, whereas most of the lncRNAs had an average of 2.71 exons (Figure 1C). Most of the mRNAs were 500-3,000 bp long with an average length of 2285.65 bp, whereas most of the lncRNAs were 200-1,000 nt long with an average length of 1713.33 nt (Figure 1D). The detailed information of mRNAs and lncRNAs can be found in Supplementary Table S2.
Differentially Expressed mRNAs and lncRNAs
We identified 772 DE mRNAs between the AN and SE libraries, within which 367 were upregulated and 405 downregulated (Figure 2A). One hundred ninety DE lncRNAs were identified between the AN and SE libraries, within which 95 were upregulated and 95 were downregulated (Figure 2B). Details are provided in Supplementary Table S3.
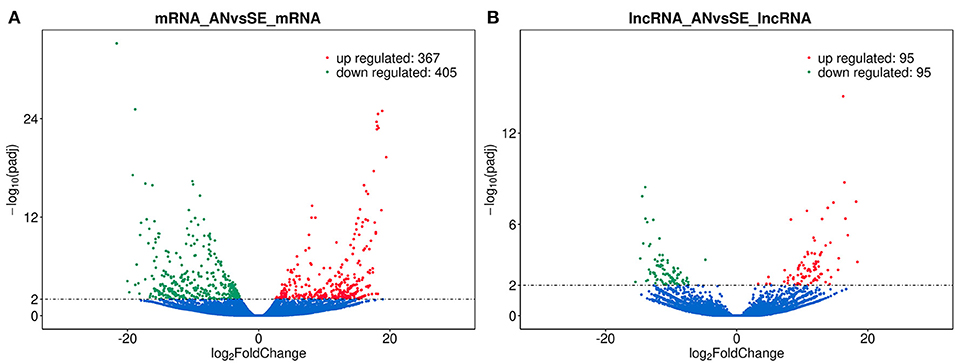
Figure 2. Volcano plot of differentially expressed (DE) mRNAs (A) and lncRNAs (B) in antagonism group (AN) vs. sensitive group (SE), where red and green represent up or downregulation, respectively.
Cluster analysis was performed and heat maps of the DE lncRNAs (Figure 3A) and DE mRNAs (Figure 3B) revealed a clear different expression pattern clearly between AN and SE.
Identification of Potential mRNA and lncRNA Biomarkers for E. Coli F17 Infection
The final parameters used for RF and XGBoost analyses of mRNA and lncRNA datasets were chosen based on a systematic evaluation of a range of values. Details can be seen in Supplementary Table S3.
For mRNA biomarkers identification, 4,424 mRNAs with positive VIM value were identified by RF and 16 mRNAs were further selected by XGBoost, of which the top three mRNAs with highest Gain value were PPP2R3A (0.81), GPR158 (0.14), and RAB44 (0.01). For lncRNA biomarkers identification, 2,700 lncRNAs with positive VIM value were identified by RF and 17 lncRNAs were further selected by XGBoost, of which the top three lncRNAs with highest Gain value were TCONS_00182693 (0.63), TCONS_00223088 (0.29), and XR_001434061.1 (0.04). Figure 4 illustrates the mRNAs (Figure 4A) and lncRNAs (Figure 4B) selected by RX. The detailed results of RX can be found in Supplementary Table S4.
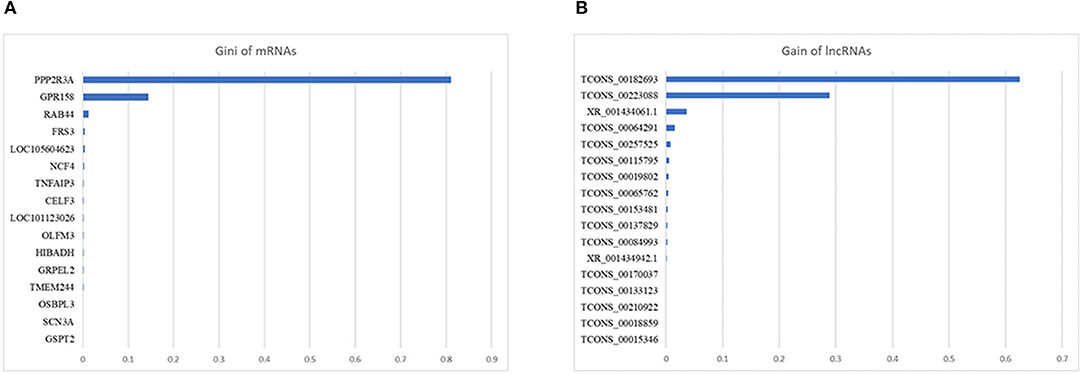
Figure 4. Gain value of the mRNAs (A) and lncRNAs (B) selected by the two-step machine learning approach (RX).
Target Gene Prediction and Integrative Network Analysis
Overall, 15,379 cis-target genes of 12,481 corresponding lncRNAs and 5,756 trans-target genes of 5,171 corresponding lncRNAs were predicted. Detailed prediction results are provided in Supplementary Tables S5, S6. Based on the DE analysis, 48 DE lncRNAs were found to cis-regulate 38 DE mRNAs, and 115 DE lncRNAs were found to trans-regulate 264 DE mRNAs. A total of 950 DE lncRNA-mRNA pairs were used for the subsequent integrative network analysis.
The connection number of each candidate node in the integrative network was calculated. The top three most connected DE lncRNAs were TCONS_00133120 (61), TCONS_00070741 (36), and TCONS_00009486 (36); and the top three most connected DE mRNAs were CES3 (33), SLC5A12 (28), and SOAT2 (20). The interaction network is in shown in Supplementary Figure S1 and detailed information is provided in Supplementary Table S7.
GO and KEGG Enrichment Analysis
To explore the mechanisms underlying E. coli F17 infection, GO and KEGG enrichment analysis were conducted on the up and downregulated DE mRNAs and DE lncRNAs identified in AN vs. SE.
The upregulated DE mRNAs were significantly enriched in 159 GO terms and 14 KEGG pathways. The top enriched GO terms Figure 5A were multi-organism cellular process (GO:0001071), extracellular region (GO:0005576), and binding (GO:0005488) under the biological process (BP), cellular component (CC), and molecular function (MF), respectively. The top enriched KEGG pathways Figure 5B were nitrogen metabolism (oas00910), amoebiasis (oas05146) and peroxisome proliferator-activated receptor (PPAR) signaling pathway (oas03320).
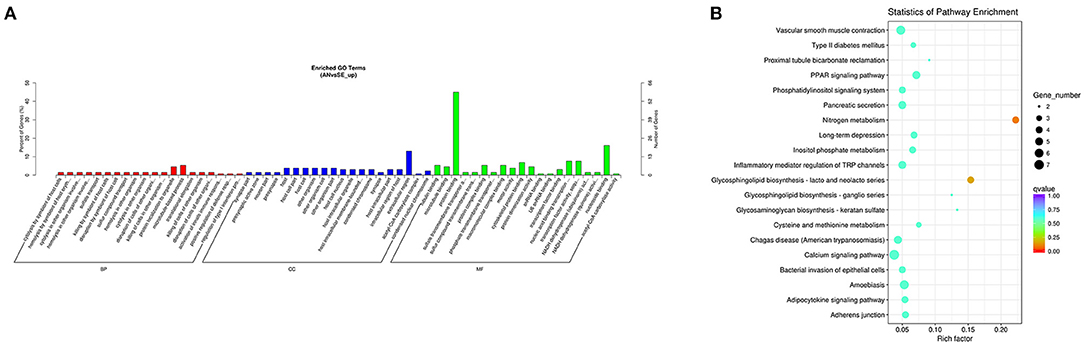
Figure 5. Top annotated gene ontology (GO) terms (A) and Kyoto Encyclopedia of Genes and Genomes (KEGG) pathways (B) of upregulated DE mRNAs.
The cis-target genes of upregulated DE lncRNAs were significantly enriched in 47 GO terms and 18 KEGG pathways. The top enriched GO terms Figure 6A were regulation of protein metabolic process (GO:0019538), ubiquitin ligase complex (GO:0000151), and nickel cation binding (GO:0016151) under the BP, CC, and MF categories, respectively. The top enriched KEGG pathways Figure 6B were metabolic pathways (oas01100), HTLV-I infection (oas05166), and steroid hormone biosynthesis (oas00140).
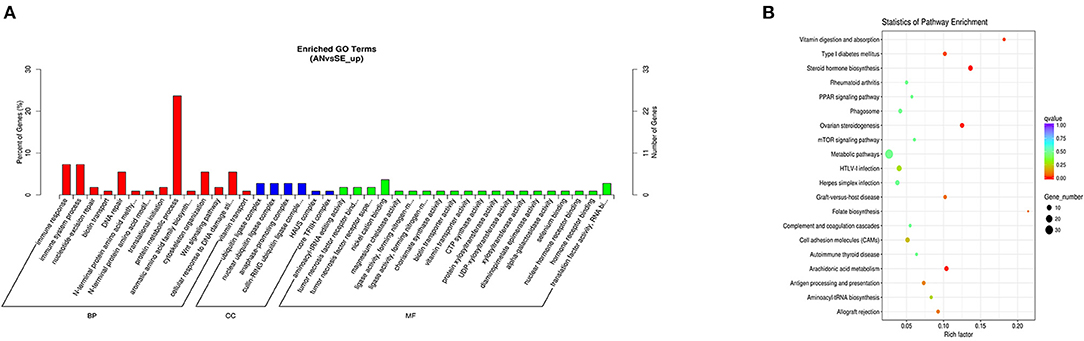
Figure 6. Top annotated GO terms (A) and KEGG pathways (B) of cis-target genes of upregulated DE lncRNAs.
The trans-target genes of the upregulated DE lncRNAs were significantly enriched in 103 GO terms and 49 KEGG pathways. The top enriched GO terms Figure 7A were single-organism metabolic process (GO:0044710), nuclear part (GO:0044428), and binding (GO:0005488) under the BP, CC, and MF categories, respectively. The top enriched KEGG pathways Figure 7B were metabolic pathways (oas01100), peroxisome (oas04146) and PPAR signaling pathway (oas03320).
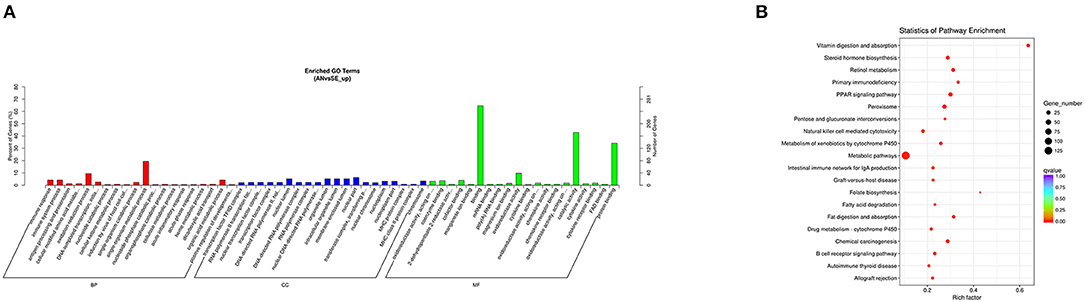
Figure 7. Top annotated GO terms (A) and KEGG pathways (B) of trans-target genes of upregulated DE lncRNAs.
The downregulated DE mRNAs were significantly enriched in 109 GO terms and 33 KEGG pathways. The top enriched GO terms Figure 8A were oxidation-reduction process (GO:0055114), nuclear chromosome (GO:0000228), and catalytic activity (GO:0003824) under the BP, CC, and MF categories, respectively. The top enriched KEGG pathways Figure 8B were metabolic pathways (oas01100), fat digestion and absorption (oas04975), and PPAR signaling pathway (oas03320).
The cis-target genes of the downregulated DE lncRNAs were significantly enriched in 78 GO terms and 6 KEGG pathways. The top enriched GO terms Figure 9A were intracellular transport (GO:0046907), chorion (GO:0042600), and binding (GO:0005488) under the BP, CC, and MF categories, respectively. The top enriched KEGG pathways Figure 9B were chemokine signaling pathway (oas04062), leukocyte transendothelial migration (oas04670), and glutathione metabolism (oas00480).
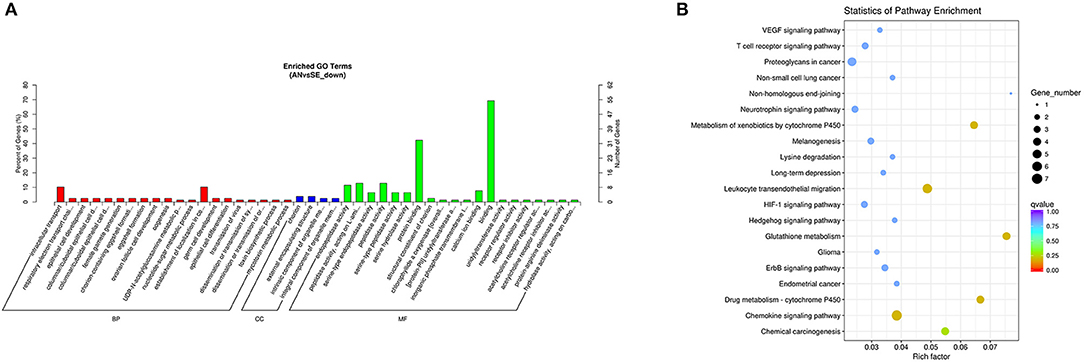
Figure 9. Top annotated GO terms (A) and KEGG pathways (B) of cis-target genes of the downregulated DE lncRNAs.
The trans-target genes of downregulated DE lncRNAs were significantly enriched in 109 GO terms and 12 KEGG pathways. The top enriched GO terms Figure 10A were biological adhesion (GO:0022610), extracellular region (GO:0005576), and binding (GO:0005488) under the BP, CC, and MF categories, respectively. The top enriched KEGG pathways Figure 10B were neuroactive ligand-receptor interaction (oas04080), calcium signaling pathway (oas04020), and adrenergic signaling in cardiomyocytes (oas04261).
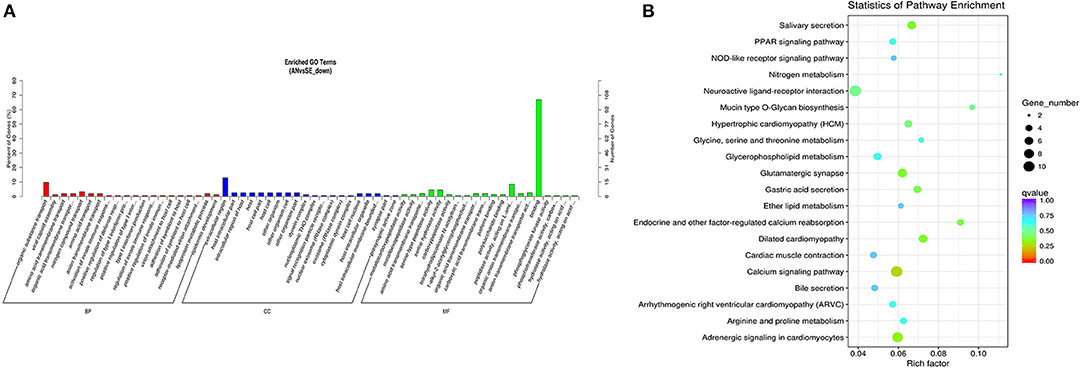
Figure 10. Top annotated GO terms (A) and KEGG pathways (B) of trans-target genes of the downregulated DE lncRNAs.
Detailed results of GO and KEGG enrichment analysis are provided in Supplementary Tables S8, S9.
Validation of the RNA-Seq Data
The expression level of selected lncRNAs and mRNAs obtained by RT-qPCR were compared with those obtained by RNA-Seq are shown in Figure 11. The expression patterns of selected lncRNAs and mRNAs were similar between RNA-Seq and RT-qPCR, indicating the reproducibility and reliability of our sequencing data.
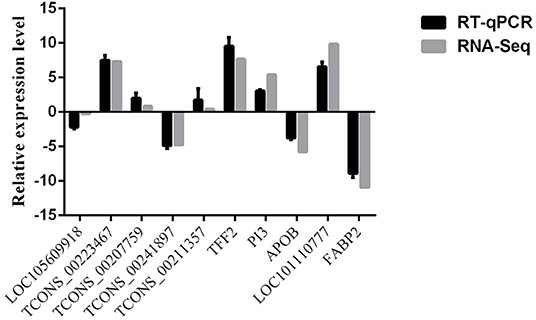
Figure 11. Comparisons of the results of the RNA-Seq and RT-qPCR analyses of selected lncRNAs and mRNAs in AN vs. SE.
Discussion
Considering the susceptibility of jejunum to E. coli F17 (19) and globally high prevalence of E. coli F17 in young livestock, we chose newborn lambs as the animal model of E. coli F17 infection for this study. Challenge experiments were conducted and E. coli F17-resistant (AN) and E. coli F17-sensitive (SE) lambs were identified based on histopathological examinations and bacteria plate counting of intestinal contents, and jejunum tissues were chosen for the final sequencing.
The average mapping rate of the clean reads was 98.54%, and 12,426 lncRNAs and 20,601 mRNAs were identified. According to the average FPKM values, the mRNA with the highest expression level was beta-2-microglobulin (B2M), a critical component of the major histocompatibility complex (MHC)-I antigen processing and presentation, which has important roles in immune control. Association was revealed between B2M and reaction to cancer immunotherapies in multiple organs (34, 35), however, investigations of the role of B2M in pathogenic E. coli infections are still limited. In our previous RNA-Seq research in spleen of diarrhea sheep, we found that B2M was an adjacent gene to DE circRNA (36). We consider that B2M may play various roles in the immune response to diarrhea in multiple organs. However, additional work is needed to confirm this possibility. The lncRNA with the highest expression level was TCONS_00211357, which was predicted to target several NF-κB pathway-related genes such as TRIM38 [NF-κB activator, (37)] and NFKBID [NF-κB inhibitor, (38)]. Numerous studies have shown that the NF-κB pathway is involved in the host inflammatory response to E. coli (39–41). Our results suggest that there is a certain probability that TCONS_00211357 act as a key regulator of NF-κB pathway in immune response to E. coli F17 infection.
As anticipated, expression profiles of lncRNAs and mRNAs vary between the AN and SE groups. Comparisons revealed 772 DE mRNAs and 190 DE lncRNAs, of which the number of upregulated DE mRNAs and DE lncRNAs were relatively lower than that of downregulated DE mRNAs and DE lncRNAs, similar to the results reported previously for a variety E. coli challenged experiments (42–44). Hence, an unstable transcriptional profile may be the main reason for the serve diarrhea seen in SE lambs.
Many of the top-ranked upregulated DE mRNAs (by padj) were reported to be involved in intestinal epithelial barrier restitution, such as the trefoil factors (TFFs), LOC105606142 (mucin-2-like), OLFM4, REG4, and LYPD8. The intestine has a double-layer physical barrier (mucus layer and IEC layer) that separates intestinal bacteria from the underlying lamina propria and deeper intestinal layers (45). Mucus, which is composed of TFFs and mucin glycoprotein (46), separates the pathogenic bacteria from direct contact with the IECs (47). The TFFs are known to be involved in mucosal restitution, protection, and proliferation, and are important stabilizers of the intestinal mucus. Here, we concentrate on two members of TFF family: TFF2 and TFF3. As previously documented, TFF3, rather than TFF2, is more involved in mucosal restitution and protection, especially in intestinal immunity (48). However, in the present study, TFF2 expression was notably higher in AN lambs than SE lambs (fold change = 204). TFF3 was highly expressed level in all lambs, but no significant changes in its expression were detected between the AN and SE lambs. Hence, we speculated that although TFF3 functions are important in mucosal restitution, the activation of TFF2 may be the key antagonist for E. coli F17 infection. Another component of mucus, mucin glycoprotein, is formed of densely glycosylated MUC2 mucin (49). Similar to TFF2, LOC105606142 (mucin-2-like) expression was significantly higher in AN lambs than it was in the SE lambs. On the basis of these results, we hypothesized that these two genes, separately or together, accelerate mucosal restitution to protect the host against E. coli F17. Products of E. coli F17 (lipopolysaccharides and enterotoxin) can lead to the massive apoptosis of IECs, which forms another physical barrier below the mucus layer, inducing IECs to proliferate. In response to injury, intestinal stem cells give rise to daughter cells with the potential to proliferate to prevent IEC damage (50). In our present study, we identified 3 genes (OLFM4, LYPD8, and REG4) that regulate the immune response of IECs, and these genes were notably more highly expressed in AN lambs than they were in SE lambs. Olfactomedin 4 (OLFM4) is generally thought to be involved in the regulation of several important signaling pathways underlying a number of imperative cellular functions (51–53). For example, in individuals infected with H. pylori, OLFM4 upregulation has been demonstrated as a marker of the immune response through reducing or eliminating H. pylori colonization (54). Given the likely cellular immune function of OLFM4, OLFM4 upregulation in the AN lambs may suggest a potential role for OLFM4 in the host immune response against E. coli F17 infection by reducing E. coli F17 colonization. Plaur domain-containing 8 (LYPD8) is a highly N-glycosylated glycosylphosphatidylinositol-anchored protein that is highly expressed on IECs. Recent studies reported that LYPD8 mediates segregation of pathogenic bacteria (including pathogenic E. coli) and epithelial cells in the intestine to preserve intestinal homeostasis (55). Considering the above evidence and our result, we infer that upregulation of LYPD8 may contribute to intestinal defense against E. coli F17 by reducing attachment on IECs. However, whether LYPD8 prevents infection with E. coli F17 still needs to be determined. REG4 was found to be strongly upregulated during intestinal inflammation, and may be involved in enhancing intestinal metaplasia and growth of organoids (8). We also found that REG4 strongly upregulated in the AN lambs, implying that REG4 may be essential to intestinal metaplasia of E. coli F17-infected hosts. Taken together, our data highlight several potential mechanisms that prevent E. coli F17 infection in AN lambs, namely, protection from mucosal restitution (TFF2 and LOC105606142) and cellular immune response (OLFM4, LYPD8, and REG4).
Based on top-ranked downregulated DE mRNAs, SE lambs had higher expression of metabolism-related genes, such as fatty acid-binding protein 2 (FABP2) and apolipoprotein genes (APOA4, APOC3, and APOB). FABP2 encodes lipid chaperones that mediates multiple lipid-mediated intestinal biological function (56, 57). FABP2 can also serve as a biomarker of intestinal inflammation, such as acute intestinal ischemia and active ulcerative colitis (58). In the present study, we found that the high expression level of FABP2 was positively corrected with the severity of diarrhea in E. coli F17 hosts, indicating that it may serve as a candidate biomarker for E. coli F17 infection. Apolipoprotein genes has been implicated in the major functions of high-density lipoprotein, including lipid binding and dissolution, and activation of lecithin (59). Our data show that apolipoprotein genes were significantly more highly expressed in SE lambs than AN lambs, especially APOA4 (fold change > 1,800). Given the critical roles of these genes in lipid metabolism, it is likely that the metabolic homeostasis of SE lambs was severely disrupted. To our great interest, in addition to lipid metabolism, APOA4 also plays a role in intestinal anti-inflammatory processes, and over-expression of APOA4 in IECs has been shown to promote differentiation and increase junctional strength (60). Thus, we speculate that in SE lambs, the proliferation and restitution of IECs were severely dysregulated, resulting in activation of APOA4 for maintenance of junction and interaction between IECs.
Advances in lncRNAs have led to their potential in acting as gene silencers by interfering with the transcription machinery to suppress gene expression (16). The top upregulated lncRNA TCONS_00223467 was predicted to target APOA4 and APOC3, two top-ranked downregulated DE mRNAs. Therefore, in the AN lambs, TCONS_00223467 may function as APOA4/APOC3-silencing factors, thereby maintaining the stability of IECs differentiation. The top downregulated lncRNA were TCONS_00241897. Interestingly, TCONS_00241897 was also predicted to target LOC101110777 (WAP four-disulfide core domain protein 18-like, WFDC18-like), one of the top-ranked upregulated DE mRNAs. WFDC genes have putative roles in immunity, such as anti-HIV, anti-microbial, and cell migration activities (61). TCONS_00241897 may function as a silencer of LOC101110777 and may be therapeutically relevant in E. coli F17 infection, especially the intestinal anti-inflammatory response. Taken together, our data suggests that TCONS_00223467 and TCONS_00241897 may play important roles in E. coli F17 infection as a gene silencer, making the prime candidates for future research.
Machine learning (ML) methods have shown promising results in identifying potential biomarkers when applied to transcriptomic datasets (62–64). In our previous study, we compared the performance of different ML methods and differential gene expression analysis methods [RF, XGBoost, RX, t-test, and edgeR; (29)]. Given that our previous results demonstrated that RX identified the smallest subsets of genes with the highest classification accuracy, RX was performed in the present study to identify potential mRNAs and lncRNAs biomarkers for E. coli F17 infection. Sixteen mRNAs and 17 lncRNAs were finally selected by RX, within which the mRNA and lncRNA with highest Gain value were PPP2R3A and TCONS_00182693. The protein phosphatase 2 regulatory subunit B”α (PPP2R3A) gene is a regulatory subunit of protein phosphatase 2A (PP2A) which regulates diverse cellular processes (65). TCONS_00182693 is the lncRNA with highest Gain value. However, not much is known about its roles in E. coli infection. The high Gain value of PPP2R3A and TCONS_00182693 demonstrated that they achieved a good performance in distinguishing AN and SE lambs in our transcriptomic datasets. Furthermore, the decision tree-based methods underlying RX (29) also indicated that certain interactivity existing between them and other mRNAs/lncRNAs was picked up by RX. Taken together, these results demonstrated that PPP2R3A and TCONS_00182693 may serve as reliable biomarkers for detection of E. coli F17 infection and reflect an important regulatory role for the phenotype under study.
The functional enrichment analyses of the DE mRNAs and target genes of the DE lncRNAs showed that immune-related terms were enriched for the upregulated DE mRNAs and lncRNAs, and that metabolic-related terms were enriched for the downregulated DE mRNAs and DE lncRNAs. Similar results have been reported previously (66, 67), further verifying our hypothesis that that the activity of immune-related genes was increased in the AN lambs and that the metabolic homeostasis was severely disrupted in the SE lambs during E. coli F17 infection. Notably, the PPAR signaling pathway was found enriched in KEGG enrichment analysis based on both DE lncRNAs and DE mRNAs. Mechanistically, the internalization of E. coli leads to the activation of intestine and liver immune system through the PPAR signaling pathway (68, 69), which support our result that the PPAR signaling pathway was linked to intestine inflammation. However, several known E. coli infection pathways, such as the TLR4 and NF-κB pathways, were not enriched in our study, which is inconsistent with the results of the previous studies. One potential explanation for these inconsistencies is that all experimental lambs were challenged with E. coli F17 in our study, while these genes were initially revealed between challenged and unchallenged individuals.
To further understand the interactions between the identified DE lncRNAs/mRNAs and the underlying intestinal immune mechanism, we constructed a DE lncRNA-mRNA integrative network that contained 950 DE lncRNA-mRNA pairs. The DE lncRNAs with the most connections were TCONS_00133120 (61), TCONS_00070741 (36), and TCONS_00009486 (36), and they were predicted to be target members of SLC family (e.g., SLC2A5, SLC5A1, and SLC15A1). The solute carrier (SLCs) family regulates the transport of molecules and have been overwhelmingly confirmed to function in cell proliferation, migration, and apoptosis (70–73). Therefore, we hypothesized that these lncRNAs may similarly regulate certain biological progress in the IECs. However, further in-depth studies are clearly needed to prove this hypothesis. The DE mRNAs with the most connections were CES3 (33), SLC5A12 (28), and SOAT2 (20). Carboxylesterase 3 (CES3) encodes an enzyme that has a wide range of activities associated with the lipid-metabolism, has a possible preventive role in cancer (74, 75). SLC5A12, (SMCT2), and was initially reported to mediate sodium-dependent transport (76, 77), but the significance of its role in the immune response is still unclear. Sterol O-acyltransferases subtype 2 (SOAT2) encodes a microsomal protein and is especially expressed in intestine and liver. SOAT2 was shown to play a critical role in delaying the development of atherosclerosis (78). It is uncertain whether these top connected lncRNAs and mRNAs are sufficient to prevent E. coli F17 infection, but there is a high probability that they interact closely and act as key regulators of the host's response to E. coli F17 infection.
Conclusion
Ribonucleic acid sequencing analysis identified 772 DE mRNAs and 190 DE lncRNAs between E. coli F17-resistant and E. coli F17-sensitive lambs. Several potential candidate mRNAs (TFF2, LOC105606142, OLFM4, LYPD8, REG4, and APOA4) and lncRNAs (TCONS_00223467 and TCONS_00241897) involved in intestinal immunity were identified. The functional enrichment analysis showed that the PPAR signaling pathway was significantly enriched in response to E. coli F17 infection. Together, our findings will increase the knowledge of the regulation modalities of lncRNAs and mRNAs against E. coli F17 infection.
Data Availability Statement
The datasets presented in this study can be found in online repositories. The names of the repository/repositories and accession number(s) can be found at: https://www.ncbi.nlm.nih.gov/, PRJNA759095.
Ethics Statement
The animal study was reviewed and approved by Experimental Animal Welfare and Ethical of Institute of Animal Science, Yangzhou University.
Author Contributions
WC, XL, XC, TG, JM, AH, and WS: conceptualization. WC, XL, WZ, TH, XC, and ZR: data curation. WC, XL, and ZR: formal analysis. XC and WS: supervision. WC: writing—original draft. WC and WS: writing—review and editing. WS: funding acquisition. All authors read and approved the final manuscript.
Funding
This work was supported by the National Natural Science Foundation of China-CGIAR (32061143036), National Natural Science Foundation of China (31872333, 32172689), Major New Varieties of Agricultural Projects in Jiangsu Province (PZCZ201739), The Projects of Domesticated Animals Platform of the Ministry of Science, Key Research and Development Plan (modern agriculture) in Jiangsu Province (BE2018354), Jiangsu Agricultural Science and Technology Innovation Fund [CX(18)2003], and Jiangsu Postgraduate Research and Innovation Program (KYCX21_3260).
Conflict of Interest
The authors declare that the research was conducted in the absence of any commercial or financial relationships that could be construed as a potential conflict of interest.
Publisher's Note
All claims expressed in this article are solely those of the authors and do not necessarily represent those of their affiliated organizations, or those of the publisher, the editors and the reviewers. Any product that may be evaluated in this article, or claim that may be made by its manufacturer, is not guaranteed or endorsed by the publisher.
Acknowledgments
We thank Margaret Biswas, Ph.D., from Liwen Bianji (Edanz) (www.liwenbianji.cn/) for editing the English text of a draft of this manuscript.
Supplementary Material
The Supplementary Material for this article can be found online at: https://www.frontiersin.org/articles/10.3389/fvets.2022.819917/full#supplementary-material
Supplementary Figure S1. The differentially expressed interaction network.
Supplementary Table S1. Primers and sequences of the selected mRNAs and lncRNAs for Real-time qPCR.
Supplementary Table S2. Detailed information of all mRNAs and lncRNAs.
Supplementary Table S3. Differentially expressed lncRNAs and mRNAs.
Supplementary Table S4. Details results of the two-step machine learning approach (RX).
Supplementary Table S5. Results of cis-target genes prediction of lncRNAs.
Supplementary Table S6. Results of trans-target genes prediction of lncRNAs.
Supplementary Table S7. Details of the differentially expressed (DE) interaction network.
Supplementary Table S8. Gene ontology (GO) enrichment results of differentially expressed mRNAs and target genes of differentially expressed lncRNAs.
Supplementary Table S9. Kyoto Encyclopedia of Genes and Genomes (KEGG) enrichment results of differentially expressed mRNAs and target genes of differentially expressed lncRNAs.
References
1. Kaper JB, Nataro JP, Mobley HLT. Pathogenic Escherichia coli. Nat Rev Microbiol. (2004) 2:123–40. doi: 10.1038/nrmicro818
2. Levine MM. Escherichia coli that cause diarrhea: enterotoxigenic, enteropathogenic, enteroinvasive, enterohemorrhagic, and enteroadherent. J Infect Dis. (1987) 155:377–89. doi: 10.1093/infdis/155.3.377
3. Subekti DS, Lesmana M, Tjaniadi P, Machpud N, Sriwati S, Daniel JC, et al. Prevalence of enterotoxigenic Escherichia coli (ETEC) in hospitalized acute diarrhea patients in Denpasar, Bali, Indonesia. Diagn Microbiol Infect Dis. (2003) 47:399–405. doi: 10.1016/S0732-8893(03)00120-2
4. Cheng DR, Zhu SY, Su ZR, Zuo WY, Lu H. Prevalence of the E. coli type three secretion system 2 (ETT2) locus among enterotoxigenic E. coli (ETEC) Shigatoxin-producing E coli (STEC) from weaned piglets. African J Microbiol Res. (2011) 5:4697–701. doi: 10.5897/AJMR11.768
5. Bandyopadhyay S, Mahanti A, Lodh C, Samanta I, Biswas TK, Dutta TK, et al. The prevalence and drug resistance profile of Shiga-toxin producing (STEC), enteropathogenic (EPEC) and enterotoxigenic (ETEC) Escherichia coli in free ranging diarrheic and non-diarrheic yaks of West Kameng, Arunachal Pradesh, India. Vet Arh. (2015) 85:501–10.
6. Ogundare ST, Fasanmi OG, Fasina FO. Risk factors for prevalence of enterotoxigenic Escherichia coli (ETEC) in diarrheic and non-diarrheic neonatal and weaner pigs, South Africa. Biomed Environ Sci. (2018) 31:149. doi: 10.3967/bes2018.018
7. Isidean SD, Riddle MS, Savarino SJ, Porter CK. A systematic review of ETEC epidemiology focusing on colonization factor and toxin expression. Vaccine. (2011) 29:6167–78. doi: 10.1016/j.vaccine.2011.06.084
8. Xiao YT, Lu Y, Wang Y, Yan WH, Cai W. Deficiency in intestinal epithelial Reg4 ameliorates intestinal inflammation and alters the colonic bacterial composition. Mucosal Immunol. (2019) 12:919–29. doi: 10.1038/s41385-019-0161-5
9. Weiner M, Dacko J, Osek J. Correlation between the presence of F5, F6, F17, F18, F41 fimbriae and the toxicity profile in Escherichia coli strains isolated from piglets with diarrhea. Medycyna Weterynaryjna-Veterinary. Med Sci Pract. (2004) 60:1342–6.
10. Cid D, Sanz R, Marin I, De Greve H, Ruiz-Santa-Quiteria JA, Amils R, et al. Characterization of nonenterotoxigenic Escherichia coli strains producing F17 fimbriae isolated from diarrheic lambs and goat kids. J Clin Microbiol. (1999) 37:1370–5. doi: 10.1128/JCM.37.5.1370-1375.1999
11. Bertagna F, Treglia G, Orlando E, Dognini L, Giovanella L, Sadeghi R, et al. Prevalence and clinical significance of incidental F18-FDG breast uptake: a systematic review and meta-analysis. Jpn J Radiol. (2014) 32:59–68. doi: 10.1007/s11604-013-0270-0
12. Kwon D, Choi C, Jung T, Chung HK, Kim JP, Bae SS, et al. Genotypic prevalence of the fimbrial adhesins (F4 F5, F6, F41 and F18) and toxins (LT, STa. STb and Stx2e) in Escherichia coli isolated from postweaning pigs with diarrhoea or oedema disease in Korea. Vet Record. (2002) 150:35–7. doi: 10.1136/vr.150.2.35
13. Bihannic M, Ghanbarpour R, Auvray F, Cavalie L, Chatre P, Boury M, et al. Identification and detection of three new F17 fimbrial variants in Escherichia coli strains isolated from cattle. Vet Res. (2014) 45:76. doi: 10.1186/s13567-014-0076-9
14. Ryu JH, Kim S, Park J, Choi KS. Characterization of virulence genes in Escherichia coli strains isolated from pre-weaned calves in the Republic of Korea. Acta Vet Scand. (2020) 62:45. doi: 10.1186/s13028-020-00543-1
15. Siuce J, Maturrano L, Wheeler JC, Rosadio R. Diarrheagenic Escherichia coli isolates from neonatal alpacas mainly display F17 fimbriae adhesion gene. Trop Anim Health Prod. (2020) 52:3917–21. doi: 10.1007/s11250-020-02415-2
16. Statello L, Guo CJ, Chen LL, Huarte M. Gene regulation by long non-coding RNAs and its biological functions. Nat Rev Mol Cell Biol. (2021) 22:96–118. doi: 10.1038/s41580-020-00315-9
17. Wang H, Wang XX, Li XR, Wang QW, Qing SZ, Zhang Y, et al. A novel long non-coding RNA regulates the immune response in MAC-T cells and contributes to bovine mastitis. FEBS J. (2019) 286:1780–95. doi: 10.1111/febs.14783
18. Ma M, Pei Y, Wang X, Feng J, Zhang Y, Gao MQ. LncRNA XIST mediates bovine mammary epithelial cell inflammatory response via NF-kappaB/NLRP3 inflammasome pathway. Cell Prolif. (2019) 52:e12525. doi: 10.1111/cpr.12525
19. Jin CY, Bao JJ, Wang Y, Chen WH, Wu TY, Wang LH, et al. Changes in long non-coding RNA expression profiles related to the antagonistic effects of Escherichia coli F17 on lamb spleens. Sci Rep. (2018) 8:16514. doi: 10.1038/s41598-018-34291-0
20. Kim D, Paggi JM, Park C, Bennett C, Salzberg SL. Graph-based genome alignment and genotyping with HISAT2 and HISAT-genotype. Nat Biotechnol. (2019) 37:907. doi: 10.1038/s41587-019-0201-4
21. Pertea M, Pertea GM, Antonescu CM, Chang TC, Mendell JT, Salzberg SL. StringTie enables improved reconstruction of a transcriptome from RNA-seq reads. Nat Biotechnol. (2015) 33:290. doi: 10.1038/nbt.3122
22. Sun L, Luo HT, Bu DC, Zhao GG, Yu KT, Zhang CH, et al. Utilizing sequence intrinsic composition to classify protein-coding and long non-coding transcripts. Nucleic Acids Res. (2013) 41:e166. doi: 10.1093/nar/gkt646
23. Kang YJ, Yang DC, Kong L, Hou M, Meng YQ, Wei LP, et al. CPC2: a fast and accurate coding potential calculator based on sequence intrinsic features. Nucleic Acids Res. (2017) 45:12–6. doi: 10.1093/nar/gkx428
24. El-Gebali S, Mistry J, Bateman A, Eddy SR, Luciani A, Potter SC, et al. The Pfam protein families database in 2019. Nucleic Acids Res. (2019) 47:427–32. doi: 10.1093/nar/gky995
25. Trapnell C, Williams BA, Pertea G, Mortazavi A, Kwan G, Van Baren MJ, et al. Transcript assembly and quantification by RNA-Seq reveals unannotated transcripts and isoform switching during cell differentiation. Nat Biotechnol. (2010) 28:511–U174. doi: 10.1038/nbt.1621
26. Robinson MD, Mccarthy DJ, Smyth GK. edgeR: a Bioconductor package for differential expression analysis of digital gene expression data. Bioinformatics. (2010) 26:139–40. doi: 10.1093/bioinformatics/btp616
28. Chen T, He T, Michael B, Vadim K, Tang Y, Hyunsu C, et al. xgboost: Extreme Gradient Boosting. R package version 1.5.0.1. 2016. https://CRAN.R-project.org/package=xgboost
29. Chen W, Alexandre PA, Ribeiro G, Fukumasu H, Sun W, Reverter A, et al. Identification of predictor genes for feed efficiency in beef cattle by applying machine learning methods to multi-tissue transcriptome data. Front Genet. (2021) 12:619857. doi: 10.3389/fgene.2021.619857
30. Shannon P, Markiel A, Ozier O, Baliga NS, Wang JT, Ramage D, et al. Cytoscape: a software environment for integrated models of biomolecular interaction networks. Genome Res. (2003) 13:2498–504. doi: 10.1101/gr.1239303
31. Young MD, Wakefield MJ, Smyth GK, Oshlack A. Gene ontology analysis for RNA-seq: accounting for selection bias. Genome Biol. (2010) 11:R14. doi: 10.1186/gb-2010-11-2-r14
32. Xie C, Mao XZ, Huang JJ, Ding Y, Wu JM, Dong S, et al. KOBAS 2.0: a web server for annotation and identification of enriched pathways and diseases. Nucleic Acids Res. (2011) 39:316–22. doi: 10.1093/nar/gkr483
33. Livak KJ, Schmittgen TD. Analysis of relative gene expression data using real-time quantitative PCR and the 2(T)(-Delta Delta C) method. Methods. (2001) 25:402–8. doi: 10.1006/meth.2001.1262
34. Sanchez-Cespedes M. B2M, JAK2 and MET in the genetic landscape of immunotolerance in lung cancer. Oncotarget. (2018) 9:35603–4. doi: 10.18632/oncotarget.26277
35. Wang HB, Liu BR, Wei J. Beta2-microglobulin(B2M) in cancer immunotherapies: biological function, resistance and remedy. Cancer Lett. (2021) 517:96–104. doi: 10.1016/j.canlet.2021.06.008
36. Jin CY, Bao JJ, Wang Y, Chen WH, Zou SX, Wu TY, et al. Changes in circRNA expression profiles related to the antagonistic effects of Escherichia coli F17 in lamb spleens. Sci Rep. (2018) 8:14524. doi: 10.1038/s41598-018-31719-5
37. Hu S, Li Y, Wang B, Peng K. TRIM38 protects chondrocytes from IL-1beta-induced apoptosis and degeneration via negatively modulating nuclear factor (NF)-kappaB signaling. Int Immunopharmacol. (2021) 99:108048. doi: 10.1016/j.intimp.2021.108048
38. Arnold CN, Pirie E, Dosenovic P, Mcinerney GM, Xia Y, Wang N, et al. A forward genetic screen reveals roles for Nfkbid, Zeb1, and Ruvbl2 in humoral immunity. Proc Natl Acad Sci USA. (2012) 109:12286–93. doi: 10.1073/pnas.1209134109
39. Zhao J, Cao J, Yu L, Ma H. Dehydroepiandrosterone alleviates E. Coli O157:H7-induced inflammation by preventing the activation of p38 MAPK and NF-kappaB pathways in mice peritoneal macrophages. Mol Immunol 114. (2019) 114–22. doi: 10.1016/j.molimm.2019.07.013
40. Cui L, Wang H, Lin J, Wang Y, Dong J, Li J, et al. Progesterone inhibits inflammatory response in E.coli- or LPS-Stimulated bovine endometrial epithelial cells by NF-kappaB and MAPK pathways. Dev Comp Immunol. (2020) 105:103568. doi: 10.1016/j.dci.2019.103568
41. Sheng X, You Q, Zhu H, Li Q, Gao H, Wang H, et al. Enterohemorrhagic E. coli effector NleL disrupts host NF-kappaB signaling by targeting multiple host proteins. J Mol Cell Biol. (2020) 12:318–21. doi: 10.1093/jmcb/mjaa003
42. Zhou C, Liu Z, Jiang J, Yu Y, Zhang Q. Differential gene expression profiling of porcine epithelial cells infected with three enterotoxigenic Escherichia coli strains. BMC Genomics. (2012) 13:330. doi: 10.1186/1471-2164-13-330
43. Wu ZC, Liu Y, Dong WH, Zhu GQ, Wu SL, Bao WB. CD14 in the TLRs signaling pathway is associated with the resistance to E. coli F18 in Chinese domestic weaned piglets. Sci Rep. (2016) 6:24611. doi: 10.1038/srep24611
44. Augustino SMA, Xu Q, Liu X, Mi S, Shi L, Liu Y, et al. Integrated analysis of lncRNAs and mRNAs reveals key trans-target genes associated with ETEC-F4ac adhesion phenotype in porcine small intestine epithelial cells. BMC Genomics. (2020) 21:780. doi: 10.1186/s12864-020-07192-8
45. Abreu MT. Toll-like receptor signalling in the intestinal epithelium: how bacterial recognition shapes intestinal function. Nat Rev Immunol. (2010) 10:131–44. doi: 10.1038/nri2707
46. Busch M, Dunker N. Trefoil factor family peptides–friends or foes? Biomol Concepts. (2015) 6:343–59. doi: 10.1515/bmc-2015-0020
47. Sinha R, Sahoo NR, Shrivastava K, Kumar P, Qureshi S, Kumar D, et al. Resistance to ETEC F4/F18-mediated piglet diarrhoea: opening the gene black box. Trop Anim Health Prod. (2019) 51:1307–20. doi: 10.1007/s11250-019-01934-x
48. Siber-Hoogeboom R, Schicht M, Hoogeboom S, Paulsen F, Traxdorf M. Obstructive sleep apnea and rhonchopathy are associated with downregulation of trefoil factor family peptide 3 (TFF3)-Implications of changes in oral mucus composition. PLoS ONE. (2017) 12:e0185200. doi: 10.1371/journal.pone.0185200
49. Yamashita MSA, Melo EO. Mucin 2 (MUC2) promoter characterization: an overview. Cell Tissue Res. (2018) 374:455–63. doi: 10.1007/s00441-018-2916-9
50. Ghosh SS, Wang J, Yannie PJ, Ghosh S. Intestinal barrier dysfunction, LPS translocation, and disease development. J Endocr Soc. (2020) 4:039. doi: 10.1210/jendso/bvz039
51. Li J, Liu C, Li D, Wan M, Zhang H, Zheng X, et al. OLFM4 inhibits epithelial-mesenchymal transition and metastatic potential of cervical cancer cells. Oncol Res. (2019) 27:763–71. doi: 10.3727/096504018X15399955297355
52. Liu W, Li H, Aerbajinai W, Botos I, Rodgers GP. OLFM4-RET fusion is an oncogenic driver in small intestine adenocarcinoma. Oncogene. (2021) 41:72–82. doi: 10.1038/s41388-021-02072-1
53. Neyazi M, Bharadwaj SS, Bullers S, Varenyiova Z, Oxford IBDCSI, Travis S, et al. Overexpression of cancer-associated stem cell gene OLFM4 in the colonic epithelium of patients with primary sclerosing cholangitis. Inflamm Bowel Dis. (2021) 27:1316–27. doi: 10.1093/ibd/izab025
54. Liu W, Yan M, Liu Y, Wang R, Li C, Deng C, et al. Olfactomedin 4 downregulates innate immunity against Helicobacter pylori infection. Proc Natl Acad Sci USA. (2010) 107:11056–61. doi: 10.1073/pnas.1001269107
55. Okumura R, Kurakawa T, Nakano T, Kayama H, Kinoshita M, Motooka D, et al. Lypd8 promotes the segregation of flagellated microbiota and colonic epithelia. Nature. (2016) 532:117. doi: 10.1038/nature17406
56. Venold FF, Penn MH, Thorsen J, Gu J, Kortner TM, Krogdahl A, et al. Intestinal fatty acid binding protein (fabp2) in Atlantic salmon (Salmo salar): localization and alteration of expression during development of diet induced enteritis. Comp Biochem Physiol A Mol Integr Physiol. (2013) 164:229–40. doi: 10.1016/j.cbpa.2012.09.009
57. Stevens BR, Goel R, Seungbum K, Richards EM, Holbert RC, Pepine CJ, et al. Increased human intestinal barrier permeability plasma biomarkers zonulin and FABP2 correlated with plasma LPS and altered gut microbiome in anxiety or depression. Gut. (2018) 67:1555–7. doi: 10.1136/gutjnl-2017-314759
58. Kocsis D, Papp M, Tornai T, Tulassay Z, Herszenyi L, Toth M, et al. Intestinal fatty acid binding protein: marker of enterocyte damage in acute and chronic gastroenterological diseases. Orv Hetil. (2016) 157:59–64. doi: 10.1556/650.2016.30336
59. Yang YJ, Fu Q, Zhou T, Li Y, Liu SK, Zeng QF, et al. Analysis of apolipoprotein genes and their involvement in disease response of channel catfish after bacterial infection. Dev Comp Immunol. (2017) 67:464–70. doi: 10.1016/j.dci.2016.09.007
60. Orso E, Moehle C, Boettcher A, Szakszon K, Werner T, Langmann T, et al. The satiety factor apolipoprotein A-IV modulates intestinal epithelial permeability through its interaction with alpha-catenin: Implications for inflammatory bowel diseases. Hormone and Metabolic Research. (2007) 39:601–11. doi: 10.1055/s-2007-984466
61. Zhang C, Hu HY, Wang XY, Zhu YJ, Jiang M. WFDC Protein: A promising diagnosis biomarker of ovarian cancer. J Cancer. (2021) 12:5404–12. doi: 10.7150/jca.57880
62. Dimopoulos AC, Koukoutegos K, Psomopoulos FE, Moulos P. Combining multiple RNA-Seq data analysis algorithms using machine learning improves differential isoform expression analysis. Methods Protoc. (2021) 4:68. doi: 10.3390/mps4040068
63. Huang GH, Zhang YH, Chen L, Li Y, Huang T, Cai YD. Identifying lung cancer cell markers with machine learning methods and single-cell RNA-Seq data. Life. (2021) 11:40. doi: 10.3390/life11090940
64. Lee J, Geng S, Li S, Li L. Single cell RNA-Seq and machine learning reveal novel subpopulations in low-grade inflammatory monocytes with unique regulatory circuits. Front Immunol. (2021) 12:627036. doi: 10.3389/fimmu.2021.627036
65. Chen H, Xu J, Wang P, Shu Q, Huang L, Guo J, et al. Protein phosphatase 2 regulatory subunit B”Alpha silencing inhibits tumor cell proliferation in liver cancer. Cancer Med. (2019) 8:7741–53. doi: 10.1002/cam4.2620
66. Fu C, Luo J, Ye ST, Yuan ZG, Li SJ. Integrated lung and tracheal mRNA-Seq and miRNA-Seq analysis of dogs with an avian-like H5N1 canine influenza virus infection. Front Microbiol. (2018) 9:303. doi: 10.3389/fmicb.2018.00303
67. Zhou D, Zhi FJ, Fang JY, Zheng WF, Li JM, Zhang GD, et al. RNA-Seq analysis reveals the role of Omp16 in brucella-infected RAW264.7 cells. Front Vet Sci. (2021) 8:646839. doi: 10.3389/fvets.2021.646839
68. Zong X, Cao XX, Wang H, Xiao X, Wang YZ, Lu ZQ. Cathelicidin-WA facilitated intestinal fatty acid absorption through enhancing PPAR-gamma dependent barrier function. Front Immunol. (2019) 10:1674. doi: 10.3389/fimmu.2019.01674
69. De Brito TV, Dias GJ, Da Cruz JS, Silva RO, Monteiro CED, Franco AX, et al. Gabapentin attenuates intestinal inflammation: role of PPAR-gamma receptor. Eur J Pharmacol. (2020) 873:172974. doi: 10.1016/j.ejphar.2020.172974
70. Xue G, Cheng Y, Ran F, Li XH, Huang T, Yang Y, et al. SLC gene-modified dendritic cells mediate T cell-dependent anti-gastric cancer immune responses in vitro. Oncol Rep. (2013) 29:595–604. doi: 10.3892/or.2012.2154
71. Bien-Moller S, Arlt A, Kaiser S, Grober A, Jedlitschky G, Grube M, et al. Expression of transporter proteins of the ABC, SLC and SLCO family in glioblastoma stem-like cells. Arch Pharmacol. (2018) 391:71.
72. Kang WT, Zhang M, Wang Q, Gu D, Huang ZL, Wang HB, et al. The SLC family are candidate diagnostic and prognostic biomarkers in clear cell renal cell carcinoma. Biomed Res Int. (2020) 2020:17. doi: 10.1155/2020/1932948
73. Heaton B, Liptrott N, David C. Glucose uptake in immune cells is lower in the presence of HIV antiretrovirals due to interactions with SLC transporters-An in vitro/in silico investigation. Br J Pharmacol. (2021) 178:456.
74. Dominguez E, Galmozzi A, Chang JW, Hsu KL, Pawlak J, Li WW, et al. integrated phenotypic and activity-based profiling links Ces3 to obesity and diabetes. Nat Chem Biol. (2014) 10:113–21. doi: 10.1038/nchembio.1429
75. Quiroga AD, Ceballos MP, Parody JP, Comanzo CG, Lorenzetti F, Pisani GB, et al. Hepatic carboxylesterase 3 (Ces3/Tgh) is downregulated in the early stages of liver cancer development in the rat. Biochimica Et Biophysica Acta-Molecular Basis of Disease. (2016) 1862:2043–53. doi: 10.1016/j.bbadis.2016.08.006
76. Srinivas SR, Gopal E, Zhuang L, Itagaki S, Martin PM, Fei YJ, et al. Cloning and functional identification of slc5a12 as a sodium-coupled low-affinity transporter for monocarboxylates (SMCT2). Biochem J. (2005) 392:655–64. doi: 10.1042/BJ20050927
77. Srivastava S, Nakagawa K, He X, Kimura T, Fukutomi T, Miyauchi S, et al. Identification of the multivalent PDZ protein PDZK1 as a binding partner of sodium-coupled monocarboxylate transporter SMCT1 (SLC5A8) and SMCT2 (SLC5A12). J Physiol Sci. (2019) 69:399–408. doi: 10.1007/s12576-018-00658-1
Keywords: lamb, E. coli F17, lncRNA, mRNA, RNA-Seq, machine learning
Citation: Chen W, Lv X, Zhang W, Hu T, Cao X, Ren Z, Getachew T, Mwacharo JM, Haile A and Sun W (2022) Insights Into Long Non-Coding RNA and mRNA Expression in the Jejunum of Lambs Challenged With Escherichia coli F17. Front. Vet. Sci. 9:819917. doi: 10.3389/fvets.2022.819917
Received: 22 November 2021; Accepted: 11 March 2022;
Published: 12 April 2022.
Edited by:
Eveline M. Ibeagha-Awemu, Agriculture and Agri-Food Canada (AAFC), CanadaReviewed by:
Mingxing Chu, Institute of Animal Sciences (CAS), ChinaXiaoyun He, Institute of Animal Sciences (CAS), China
Copyright © 2022 Chen, Lv, Zhang, Hu, Cao, Ren, Getachew, Mwacharo, Haile and Sun. This is an open-access article distributed under the terms of the Creative Commons Attribution License (CC BY). The use, distribution or reproduction in other forums is permitted, provided the original author(s) and the copyright owner(s) are credited and that the original publication in this journal is cited, in accordance with accepted academic practice. No use, distribution or reproduction is permitted which does not comply with these terms.
*Correspondence: Wei Sun, ZGt4bXN1bndlaUAxNjMuY29t