- 1Department of Animal Science, Faculty of Agriculture, Tarbiat Modares University, Tehran, Iran
- 2Department of Animal Science, Faculty of Agriculture, Yasouj University, Yasouj, Iran
- 3Department of Animal Science, Faculty of Agriculture, University of Kurdistan, Sanandaj, Iran
- 4Department of Agriculture, Payam Noor University Tehran, Tehran, Iran
- 5The Roslin Institute and Royal (Dick) School of Veterinary Studies R(D)SVS, University of Edinburgh, Edinburgh, United Kingdom
Introduction: The uncoupling proteins (UCPs) are involved in lipid metabolism and belong to a family of mitochondrial anionic transporters. In poultry, only one UCP homologue has been identified and experimentally shown to be associated with growth, feed conversion ratio, and abdominal fat according to its predominant expression in bird muscles. In endotherm birds, cell metabolic efficiency can be tuned by the rate of mitochondrial coupling. Thus, avUCP may be a key contributor to controlling metabolic rate during particular environmental changes.
Methods: This study aimed to perform a set of in-silico investigations primarily focused on the structural, biological, and biomimetic functions of avUCP. Thereby, using in silico genome analyses among 8 avian species (chicken, turkey, swallow, manakin, sparrow, wagtail, pigeon, and mallard) and a series of bioinformatic approaches, we provide phylogenetic inference and comparative genomics of avUCPs and investigate whether sequence variation can alter coding sequence characteristics, the protein structure, and its biological features. Complementarily, a combination of literature mining and prediction approaches was also applied to predict the gene networks of avUCP to identify genes, pathways, and biological crosstalk associated with avUCP function.
Results: The results showed the evolutionary alteration of UCP proteins in different avian species. Uncoupling proteins in avian species are highly conserved trans membrane proteins as seen by sequence alignment, physio-chemical parameters, and predicted protein structures. Taken together, avUCP has the potential to be considered a functional marker for the identification of cell metabolic state, thermogenesis, and oxidative stress caused by cold, heat, fasting, transfer, and other chemical stimuli stresses in birds. It can also be deduced that avUCP, in migrant or domestic birds, may increase heat stress resistance by reducing fatty acid transport/b-oxidation and thermoregulation alongside antioxidant defense mechanisms. The predicted gene network for avUCP highlighted a cluster of 21 genes involved in response to stress and 28 genes related to lipid metabolism and the proton buffering system. Finally, among 11 enriched pathways, crosstalk of 5 signaling pathways including MAPK, adipocytokine, mTOR, insulin, ErbB, and GnRH was predicted, indicating a possible combination of positive or negative feedback among pathways to regulate avUCP functions.
Discussion: Genetic selection for fast-growing commercial poultry has unintentionally increased susceptibility to many kinds of oxidative stress, and so avUCP could be considered as a potential candidate gene for balancing energy expenditure and reactive oxygen species production, especially in breeding programs. In conclusion, avUCP can be introduced as a pleiotropic gene that requires the contribution of regulatory genes, hormones, pathways, and genetic crosstalk to allow its finely-tuned function.
1. Introduction
Generalized homeostasis of energy expenditure and energy intake is essential for the best selection criteria in poultry, however, the regulatory mechanisms connecting feed intake, growth, and energy balance are still confusing. A growing body of literature implies avian Uncoupling Protein (avUCP) plays a key role in cell metabolism and adaptive thermogenesis. It thus may have the potential to be considered the missing link in the chain of whole-body energy homeostasis in chickens. The avUCP is a homolog with more than 70% protein sequence similarity with mammalian UCP3 and UCP2, harboring two conserved regions of the mitochondrial carrier and ADP/ATP transporter translocase (1, 2). The avUCP gene was first identified in 2001 after screening a hummingbird skeletal muscle cDNA library (3). Subsequent studies in different avian species revealed a predominant expression of avUCP mRNA in skeletal muscle in chicken (Gallus gallus), king penguin (Aptenodytes patagonicus), and hummingbird (Eupetomena macroura) (1, 4–7). The interconnection of growth, oxidative stress, reproductive state, immunity, and feather coloration processes and their efficacy on thermo-regulation have been suggested by several studies (8–12).
For a long time, shivering thermogenesis has been known to be the main thermogenic mechanism in avian species (13, 14), although the evidence for the existence of adaptive mechanisms of heat production and non-shivering thermogenesis are currently growing (1). This thermogenesis mechanism can be boosted by increasing oxidative metabolic capacity along with the uncoupling of aerobic metabolism from ATP production. Also, previous studies support the involvement of avUCP in avian energy expenditure and adaptive thermogenesis (1, 15–18). Taouis et al. showed that early thermal conditioning in broiler chicks can instantly reduce body temperature and avUCP expression in the pectoral muscle, which may potentially improve the resistance to heat stress in broilers (15). In contrast, another study showed that diet-induced thermogenesis had no control over feed intake in layers and broilers and the expression of avUCP was not influenced by layer and broiler genotypes. Consequently, these findings led to the rejection of the hypothesis of the involvement of avUCP in diet-induced thermogenesis (19). A study in ducklings has verified that, in proportion to the degree of cold, the increase in metabolic heat production occurs in parallel with the upregulation of avUCP and higher mitochondrial oxidative phosphorylation, while no change in mitochondrial membrane conductance capacity occurred (20).
avUCP has also been suggested to be involved in cell metabolism. Several studies have identified polymorphism of the avUCP gene that is associated with fat metabolism, growth, feed intake, and exposure to abiotic stress conditions (2, 21, 22). Additionally, it was also revealed that the upregulation of avUCP can result in the down-regulation of reactive oxygen species (ROS) production in the skeletal muscle of fasted chickens (23). On the other hand, the avUCP expressed in glycolytic muscle fibers may be a passive transporter of pyruvate for ensuring a sustained balance between glycolysis and oxidative phosphorylation (24). Conversely, it was demonstrated that heat stress stimulates mitochondrial superoxide production in broiler skeletal muscle through the downregulation of uncoupling protein (25, 26). Another study showed that the expression of members of the beta-oxidation pathway and mitochondrial fatty acid transport were upregulated upon heat stress. However, the expression of avUCP did not control ROS production in heat-stressed chickens (18). Heat stress, by causing oxidative stress, impairs mitochondrial function by decreasing avUCP expression (27) which can further impair meat quality and increases glycolysis and intramuscular fat deposition (2, 28, 29). Additionally, the upregulation of avUCP and avPGC-1α together can help to reduce ROS accumulation and lipid oxidation in the skeletal muscles of birds (28, 30). Several distinct studies of avUCP, have demonstrated the regulation of avUCP expression and regulation of its putative function. Accordingly, thyroid hormones were reported to increase thermogenic capacity in the avian muscle and liver (31, 32). Moreover, uncoupling of sarco- endoplasmic reticulum calcium ATPase pump activity in muscle (33) and regulation of glycolysis are involved in controlling thermogenic processes in avian species. Some studies have implied that thermogenesis is controlled by thyroid hormone affecting PPARGC1A and SLC25A4 gene expression in chickens (34, 35). However, triiodothyronine (T3) is reported to have a biphasic effect on avUCP expression (32). Another study investigating variations in avUCP expression, thyroid hormone metabolism, and heat production during cold exposure has reported a significant increase in body temperature, avUCP expression, T3 level, renal outer-ring deiodination activity, and also increased thyroxine (T4) level, and hepatic inner-ring deiodination activity. Meanwhile, no significant differences in body weight and feed intake were reported in comparison with chickens reared in normal temperatures (36).
Moreover, it has been implied that avUCP gene expression is down-regulated by leptin hormone and up-regulated by pro-inflammatory cytokines IL-6 and TNFα through modulation of avUCP-related transcription factors (PPARs and PGC-1α) (32). Two-fold over-expression in gastrocnemius muscle, significant down-regulation, and no significant change were reported in avUCP mRNA expression through injection of thyroid hormone, methimazole, and insulin respectively (16). Additionally, selenium deficiency in broilers can cause a reduction in avUCP mRNA levels that results in oxidative stress, inflammation, and glyco-metabolism disorders (37).
Furthermore, in fat chickens with a higher fat diet, avUCP was significantly up-regulated, which could be correlated with the particular need for antioxidant pathways in muscle (38). Previous research has provided some evidence for the involvement of the beta-adrenergic system, PPAR transcription factors, and the AMP-activated protein kinase (AMPK) to control the expression of avUCP (39). Furthermore, oral use of D-aspartate resulted in a reduction in body temperature through the decline in avUCP mRNA expression in the breast muscle, which may be involved in reduced mitochondrial proton leaks and heat production (40).
There is also some evidence Oleuropein can also affect avUCP expression as well as genes related to mitochondrial oxidative phosphorylation and induce mitochondrial biogenesis in avian muscle cells. Oleuropeins can suppress mitochondrial superoxide production, through up-regulation of avUCP and manganese superoxide dismutase (41, 42). Therefore, the orexin system in avian muscle cells can regulate mitochondrial dynamics without affecting ATP synthesis (43). Evidence has also been presented that retinoic acid can activate the thermogenic function of avUCP in birds (44). Interestingly, “avian” is reported to be the only vertebrate lineage having just one UCP gene (45). Thus, the avian uncoupling protein seems to provide a unique opportunity to explore the functional activity and regulation patterns of UCP. We aimed, therefore, to investigate avUCPs in eight different avian species (chicken, turkey, swallow, manakin, sparrow, wagtail, pigeon, and mallard) through a wide range of comparative bioinformatics analyses to better understand the details of avUCPs, from their coding sequences to their functional consequences.
2. Methods
2.1. Coding sequence analysis
The nucleotide coding sequences and amino acid sequences of avian uncoupling proteins were downloaded from a dataset contained at https://figshare.com/. Sequence alignment of coding sequences (CDS) for eight avian species including chicken, turkey, swallow, manakin, sparrow, wagtail, pigeon, and mallard was conducted for determining the number of conserved, variable, parsimonious, and singleton sites in avUCPs. Moreover, nucleotide composition, GC content, codon frequency, and relative synonymous codon usage were obtained using MEGA11 software (46). The Codon Adaptation Index (CAI) for each of the studied avian species was estimated using the Markov model with 500 replications over the avUCP DNA sequence. As the reference set to calculate the CAI is important for interpretation, the codon usage table for each species (if it exists), from the codon usage database on the CAIcal server (http://www.kazusa.or.jp/codon/) was therefore utilized (47). The Relative Synonymous Codon Usage (RSCU) was calculated as follows:
Where xi is the number of times the ith codon has been favored to be used for an amino acid, and n represents the number of synonymous codons for that amino acid.
2.2. Protein sequence analysis
Amino acid (a.a) composition, physio-chemical parameters and phylogenetic analysis of eight avian protein sequences were performed in QIAGEN CLC Genomics Workbench (RRID: SCR_011853) (48). Physio-chemical parameters including molecular weight, isoelectric point, extinction coefficient, instability index, aliphatic index, and grand average of hydropathicity, were determined for each avUCP protein sequence. The phylogenetic analyses were performed using the Neighbor-Joining method, Jukes-Cantor protein distance measure, and bootstrapping over 307 a.a of protein sequences of eight avian species in CLC Genomics Workbench (48). Entropy analysis was then carried out using BioEdit (49) to further determine variable and conserved sites and finally, by using the Skylign online tool, a positional logo of amino acid variability was constructed (50).
2.3. Protein structure prediction
The secondary structures of avUCPs were predicted by the SOPMA predictor (51). All avUCP sequences were submitted to the Phyre2 web portal (http://www.sbg.bio.ic.ac.uk/~phyre2/html/page.cgi?id=index) as a batch file for tertiary structure prediction. After that, the avUCPs were modeled through four stages including homology detection, fold library scanning to predict secondary structure, loop modeling, and sidechain fitting (52). Structural evaluation and qualification were then performed using the Swiss-Model online tool (53).
2.4. Sequence-based gene ontology prediction
PredictProtein was used to predict Gene Ontology (GO) terms of cellular components, molecular function, and biological process for avUCP protein sequences (54). In this process, the distance between the input protein sequence and the closest annotated protein represents the reliability of GO prediction (54).
2.5. Interactive network prediction and gene-based enrichment analysis
By identifying genes related to avUCP from the literature, a list of genes was extracted according to Davoodi and Ehsani (55) for protein-protein network prediction. The list of most related-genes was provided through the retrospective review of previous studies on avUCP (5, 42, 56–71). Biomolecular network prediction and gene set enrichment analysis of networked genes were performed in Cytoscape (72) using STRING v11.5 (73, 74).
2.6. Pathway crosstalk prediction
Crosstalk prediction was applied using XtalkDB by querying pathways enriched for avUCP to predict which pairs of signaling pathways may interact to reach a conclusive understanding of biological pathways involved in the regulation of avUCP functions from a global view (75).
2.7. Datasets
The nucleotide coding sequences (CDS) and protein sequences of avian uncoupling protein (avUCP) from eight different avian species (chicken, turkey, swallow, manakin, sparrow, wagtail, pigeon, and mallard) were retrieved in FASTA format from the NCBI database and used for in silico analyses (Table 1).
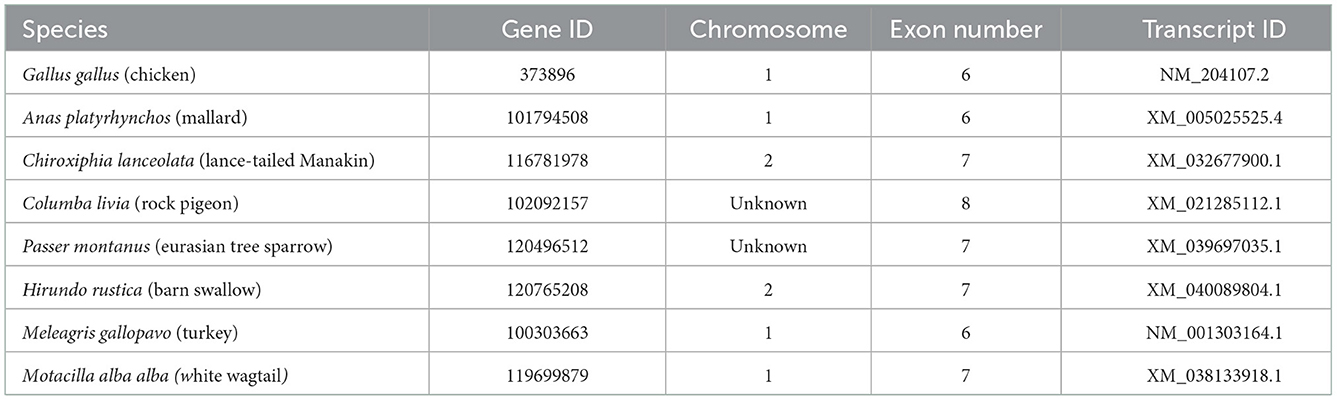
Table 1. General information for avUCP genes in eight avian species extracted from the NCBI database.
2.8. Model of analysis
This research had an integrative pipeline but no unique statistical model. All parts of the pipeline are explained previously in each section.
3. Results
3.1. Coding sequence analysis
3.1.1. Nucleotide composition
The CDS sequences were analyzed for nucleotide composition, GC content, conserved, variable, parsimony informative, and singleton sites. The CDS length in all selected birds consisted of 924 nucleotides. The number of conserved, variable, parsimony informative, and singleton sites were revealed as 684, 240, 145, and 95, respectively. Divergence details of GC content among the eight avian species are shown in Table 2. The “C” content in the coding sequences of avUCP in wagtail (2.5), mallard (1.0), sparrow (0.8), and manakin (0.1) was higher than that of “G,” however, the “G” content in turkey (1.8), chicken (1.2), swallow (0.6), and pigeon (0.3) was higher than that of the “C” content.
3.1.2. Codon usage analysis
The codon usage (CU) and relative synonymous codon usage (RSCU) values of avUCP coding sequence were calculated, then CU and RSCU patterns were obtained from the eight avian avUCPs. Generally, 64 combinations of 3-letter codons encode 20 different amino acids, thus showing codon redundancy. After excluding the three stop codons, 25 codons in pigeon, sparrow, turkey, wagtail, 24 codons in chicken, mallard, and swallow, and 23 codons in manakin avUCP were observed with an RSCU value higher than 1. Moreover, the numbers of unused codons (RSCU = 0) were as follows: chicken−13, mallard−14, manakin−8, pigeon−16, swallow−13, sparrow−15, turkey−11, and wagtail−18. The RSCU value of the codon CUG, which encodes leucine, was the highest in all selected avian species. By looking at codons with an RSCU >1 and examining their final bases, it was found that they ended, on average, with C (15), G (9), and roughly one U and A in the selected species. The highly preferred codons within avUCP, with their corresponding CU and RSCU values are presented in Table 3. As can be seen, all highly preferred codons, except the CAU (only in manakin), which encodes for histidine, end with a “C” or a “G.” In addition, these 25 highly preferred codons are responsible for encoding around 76% (in manakin) to 89% (in wagtail) of the total protein sequences of UCP. Moreover, the expected codon adaptation index (CAI) for retrieved CDSs of chicken, mallard, manakin, pigeon, sparrow, swallow, turkey, and wagtail were 0.707, 0.691, 0.712, 0.697, 0.695, 0.692, 0.709, and 0.696, respectively.
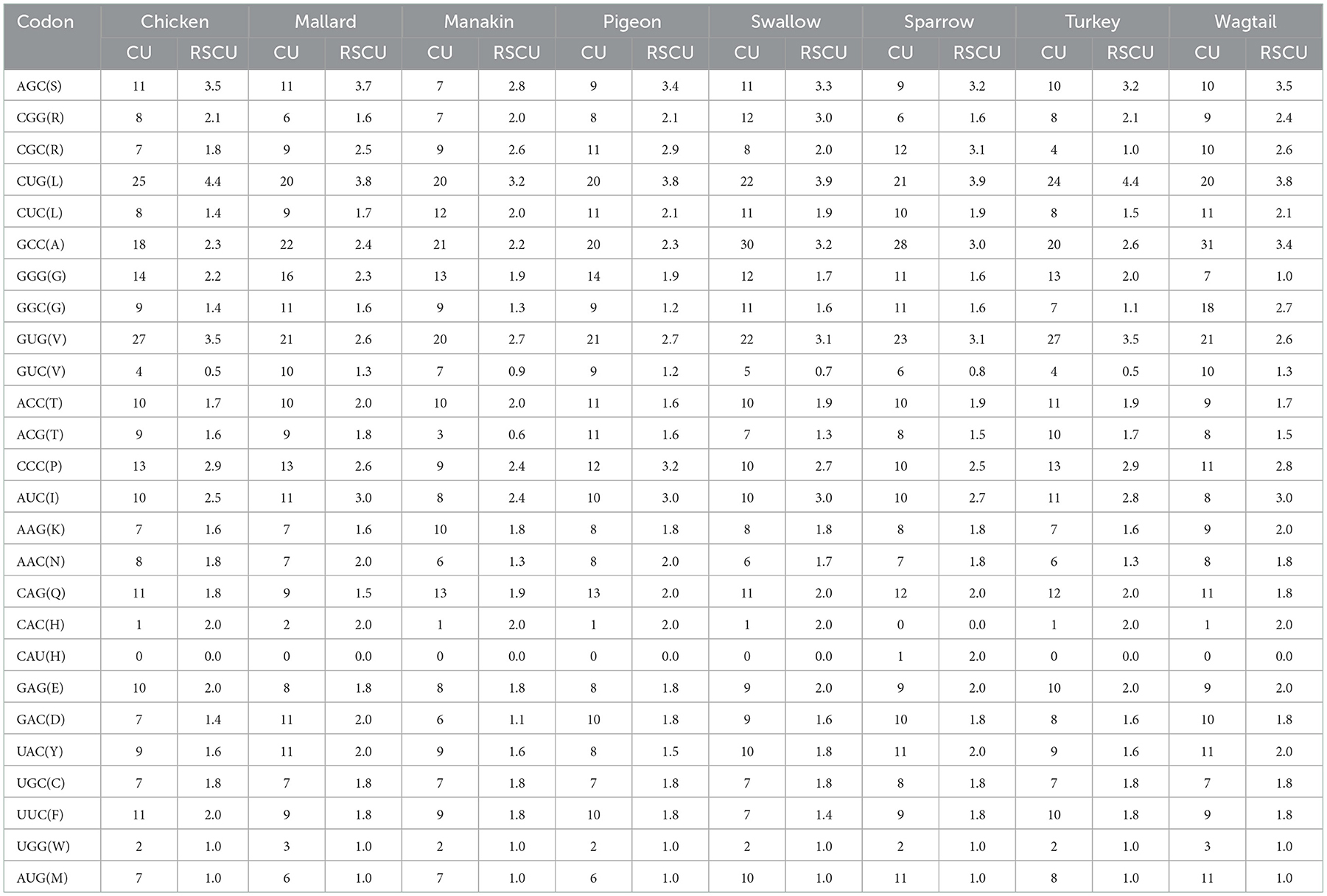
Table 3. The codon usage and relative synonymous codon usage of highly preferred codons for avUCP in eight avian species.
3.2. Protein sequence analysis
3.2.1. Amino acid compositions
The results of the protein sequence analysis of the eight avUCPs revealed a sequence length of 307 amino acids and the amino acid compositions in different birds are represented in Figure 1. Alanine, leucine, valine, and glycine have been observed at high frequency in all avUCPs, while histidine—a positively charged, and tryptophan—an aromatic amino acid, have been detected at the lowest frequency in all avUCPs. In contrast to the slight variation in amino acid usage among the eight studied avUCP sequences, tyrosine was the only completely constant amino acid among the avUCPs in all eight birds.
3.2.2. Physio-chemical analysis
Physio-chemical parameters of uncoupling proteins including molecular weight, isoelectric point, aliphatic index, number of sulfur atoms, hydrophobicity, hydrophilicity, the percentage of negatively and positively charged amino acids, instability index, and grand average hydropathy of avian uncoupling protein in eight avian species are summarized in Table 4. The isoelectric point of UCPs ranged from 9.51 to 9.66. The lowest instability index (II) and the highest aliphatic index (AI) were observed in UCP of manakin. The molecular weight and the overall negatively/positively charged amino acids were nearly similar in all avUCPs in the current study.
The atomic sulfur count varied from 14 (mallard, pigeon) to 20 (sparrow). Sulfur can be found in cysteine and methionine amino acids. Eight cysteine residues were observed in seven of the avUCPs but the protein sequence of sparrow contained nine cysteine residues. Furthermore, the hydrophobic methionine was variable among avUCPs, which could be a source of variability in atomic sulfur count among avUCP sequences in the studied species.
A protein with an II smaller than 40 is considered stable, and proteins with an II above 40 can be considered somehow unstable (76, 77) and in the current study, the highest II was observed in UCP of chicken. Also, protein sequences with a GRAVY index above 0 are more likely to be hydrophobic and all studied proteins were revealed to have more hydrophobic regions.
3.2.3. Entropy analysis
For more evaluation of the status of amino acids in the avUCP protein sequences, entropy measures for each position were estimated using BioEdit (49) and visualized by the Shannon entropy plot, as shown in Figure 2. The estimated entropies of aligned sequences ranged from 0 to 1.56, the average entropy was estimated as 0.133 and a total of 274 positions displayed an entropy of 0. Seven positions revealed entropy values higher than 1 (147, 151, 267, 306, 299, 150, and 307). Among these, 147th and 151st positions showed the highest entropy of 1.56 and 1.39, respectively. Also, eight regions with a length of more than 10 amino acids (1→ 11, 30→ 50, 56→ 70, 83→ 107, 133→ 146, 157→ 172, 174→ 187, 216→ 246) have represented entropy of zero, indicating totally conserved regions in the examined avUCP protein sequences. Moreover, any region with more than 10 consecutive amino acids with an average entropy of >1 was not observed. To provide a compact representation of the most variable sites with the highest entropy in avUCP sequences among these eight species, the Skylign online tool (50) was used to make a positional logo showing amino acid variability (Figure 3).
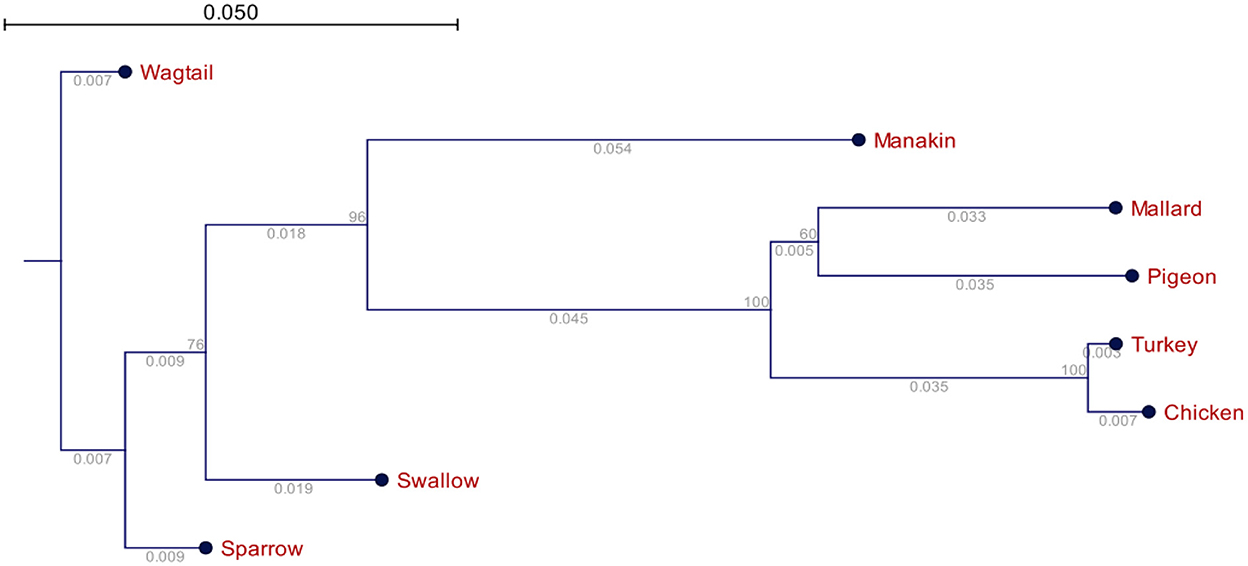
Figure 3. Phylogenetic relationships of the avUCPs in eight avian species (the numbers below the lines represent the branch size, and the numbers above the lines represent the bootstrap value).
3.2.3.1. Phylogeny analysis
The phylogenetic relationship of the avUCPs in the eight examined avian species is depicted in Figure 4. A neighbor-joining tree was derived from the multiple protein sequence alignment. Phylogenetically, the longest distance was detected between chicken and wagtail, in contrast chicken and turkey showed the closest phylogenetic relationship which is in agreement with comparative results of amino acid component, codon usage pattern and physio-chemical parameters among avUCPs of mentioned birds.
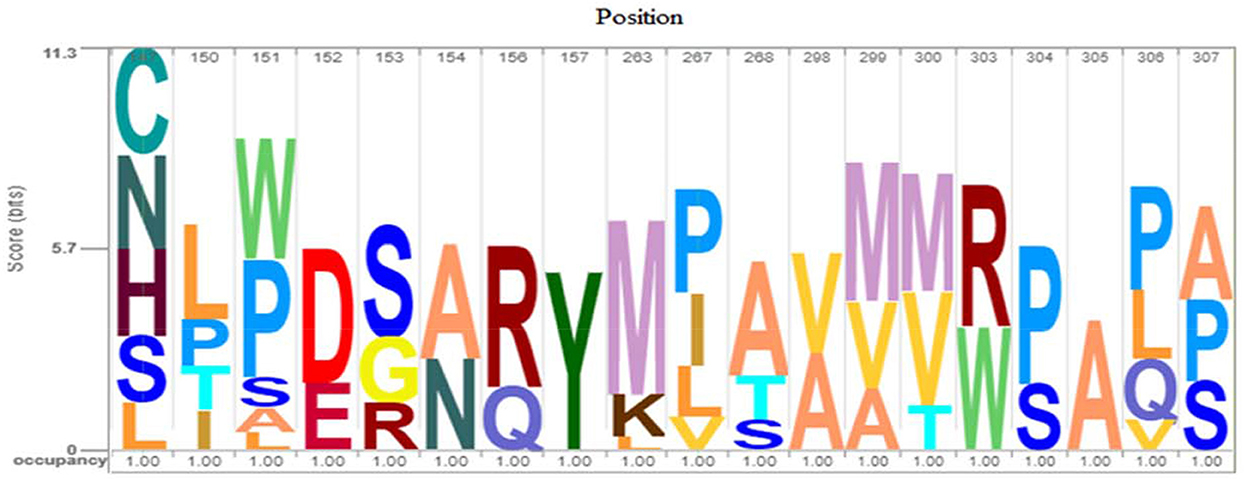
Figure 4. Logo of avUCP protein alignments (most variable sites with high entropy) among eight avian species. The height of the bar of letters (amino acids) displays the conservation at that position and the height of each letter within a bar is determined based on the frequency of that letter in that position.
3.3. Protein structure prediction
SOPMA was used to determine the percentage of α-helix, β-sheets, turns, and random coils to predict the secondary structure of the selected avUCP sequences through a neural network approach (51). The schematic predicted secondary structures of avUCPs in the eight avian species are shown in Figure 5. Because the secondary and tertiary structures of the protein are completely influenced by its primary structure, any differences in amino acid sequences can potentially modify the secondary and tertiary structures. Within the eight avUCP proteins, slightly different percentages of α-helical and β-turn formations were derived. The average contribution of alpha-helices, extended-strands, beta-turns, and random coils were calculated as 46.60 ± 1.04, 15.87 ± 0.58, 7.42 ± 0.65, and 30.08 ± 0.95% among the eight avUCP proteins, respectively. The level of alpha-helical structure was determined higher than other secondary structures.
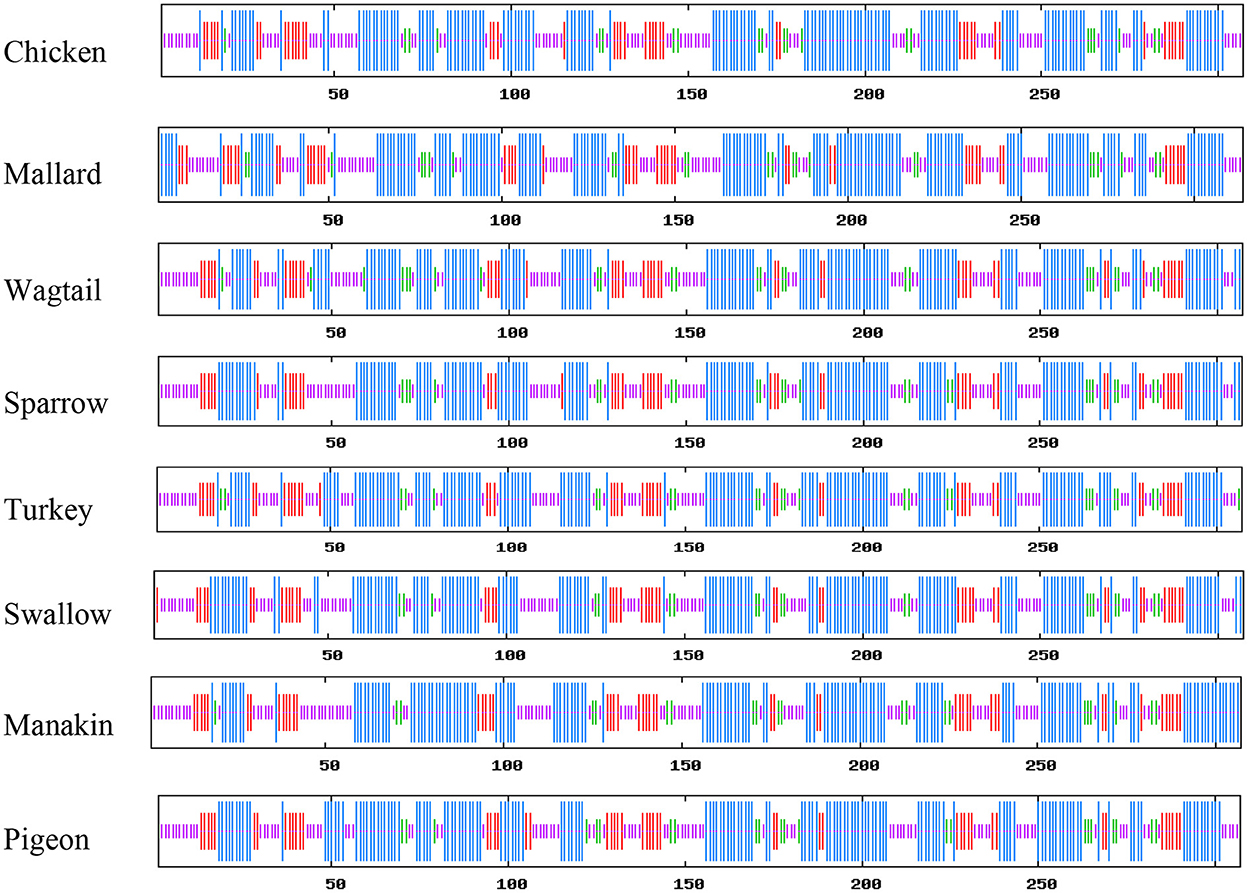
Figure 5. Comparative view of the secondary structure of avUCPs in eight avian species (Blue, Alpha helix; Purple, Random coil; Red, Extended-strand; Green, Beta turn).
The protein structure was predicted by three different software programs: phyre2, Predict Protein and SOPMA, with the final structural evaluation performed by SWISS_MODEL for checking the clashing score, favoured Ramachandran residues, and rotamer outliers. By using the Phyre2web portal (52), through the homology detection method, three-dimensional structures of the 8 avUCPs alongside potential extracellular, cytoplasmic, and trans membrane helices were predicted. In the protein structure, 6 trans membrane, 4 extracellular, and 3 cytosolic regions were predicted for the avUCPs. The two C-terminal and N-terminal segments were considered extracellular regions. Moreover, both hydrophobic and hydrophilic regions can be seen throughout the avUCP sequence. Accordingly, six areas with positive hydropathy are likely to represent transmembrane helices, which indicates avUCP can function as a trans membrane protein (Figure 6A). Meanwhile, areas with negative hydropathy show that these regions can form the extracellular part of avUCP (Figure 6A).
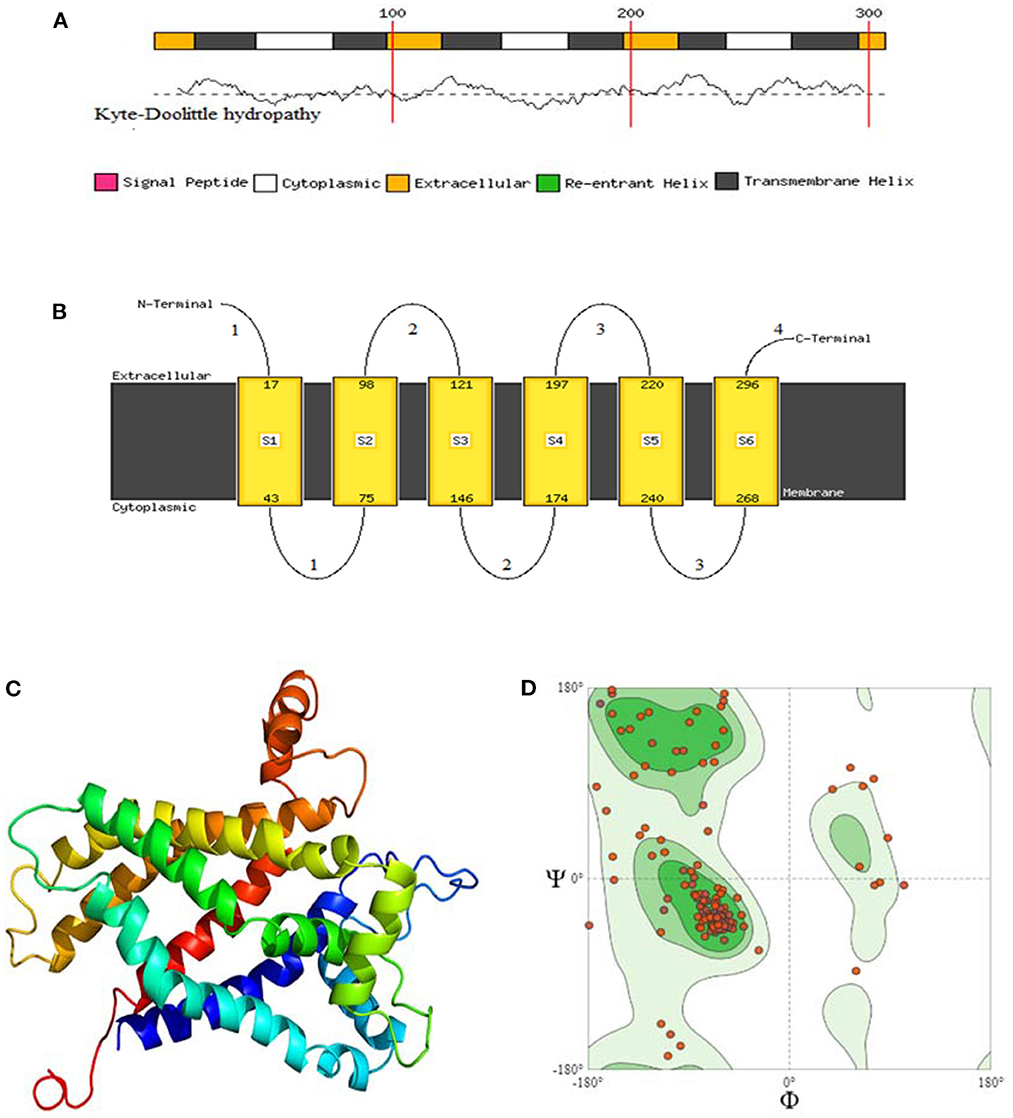
Figure 6. (A) Membrane helix prediction with support vector machines with Kyte-Doolittle hydropathy plot. (B) Schematic figure of predicted extracellular, cytoplasmic, and transmembrane helices. (C) Top 3D model of avian UCP [Model dimensions (Å): X: 53.347, Y: 74.255, Z: 50.565, Image colored by the rainbow N → C terminus]. (D) Ramachandran plot with 88.46% favored. A dihedral angle of a protein is the internal angle of polypeptide backbone at which two adjacent planes meet. The conformation of the backbone can be described by two dihedral angles per residue, because the backbone residing between two juxtaposing Cα atoms are all in a single plane. These angles are called ϕ (phi) which involves the backbone atoms C-N-Cα-C, and ψ (psi) which involves the backbone atoms N-Cα-C-N.
Ultimately, among the eight avUCPs, the predicted structure with the highest sequence identity (71%), alignment coverage (94%), interface similarity (51%), and confidence (100%) is illustrated in Figures 6A–C. The nuclear magnetic resonance molecular fragment replacement approach was applied to re-specify protein structure using the Swiss-Model web tool. The local and global model quality was then specified from Ramachandran analysis for the predicted tertiary structure of avUCP (Figure 6D). The final structure evaluation resulted in a clash score (the number of serious clashes per 1,000 atoms) of 90%, Ramachandran residues favored−88.46%, Ramachandran outliers−3.50%, rotamer outliers−8.09%, 0.01 bad angles, and two C-beta deviations.
3.4. Sequence-based gene ontology prediction
Gene Ontology (GO) terms associated with avUCP were predicted using deep learning embedding through the PredictProtein online tool (54). For this reason, the distance between the input avUCP protein and the closest annotated protein was represented as the reliability of GO prediction. The GO trees of avUCP are depicted in Figure 7. Consequently, four biological processes including “mitochondrial transmembrane transport,” “proton transmembrane transport,” “adaptive thermogenesis,” and “response to cold” were predicted with 57% reliability. Also, three cellular components including “mitochondrion,” “mitochondrial inner membrane,” and “integral component of the membrane,” along with one molecular function of “oxidative phosphorylation uncoupler activity” were also anticipated with 57% confidence.
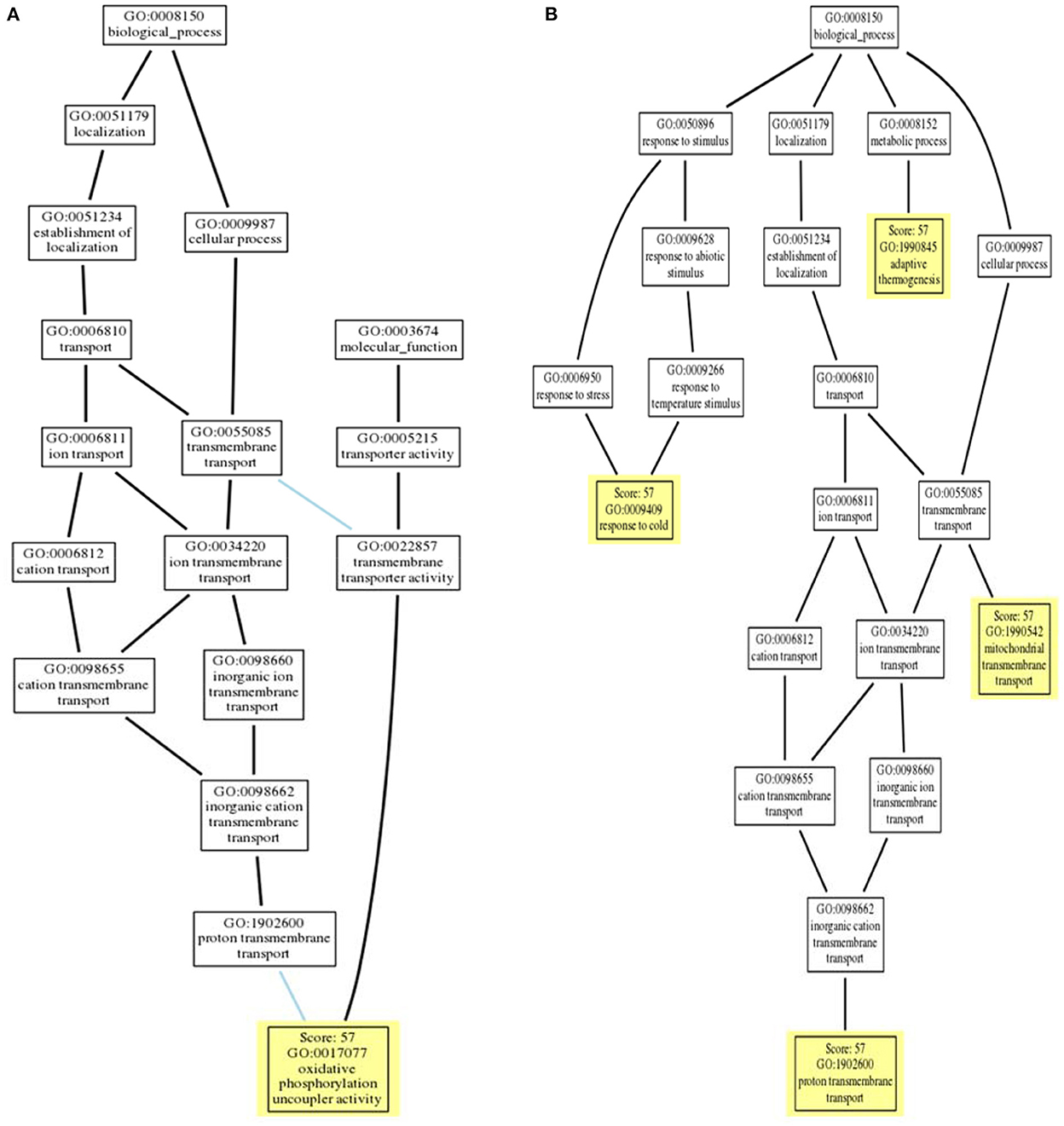
Figure 7. (A) Molecular function ontology tree and (B) biological process ontology tree (locations of PREDICTED terms are highlighted in yellow with respect to inferred terms).
3.5. Interactive network prediction and gene set enrichment analysis
Gene network was predicted in Cytoscape software (3.9.1) using the embedded STRING app, and clustering was performed by the K-means method (72). A total of 49 published avUCP related-genes were used as input for network prediction and resulted in highly orchestrated interactions among genes. This network is divided into two clusters with the highest confidence: cluster one containing 21 genes involved in response to stress, and cluster two containing 28 genes involved in lipid metabolism and proton buffering system. The predicted network is shown in Figure 8. GO analysis on these networked genes highlighted several biological processes including the fatty acid metabolic process (GO:0006631), response to chemical (GO:0042221), cellular response to chemical stimulus (GO:0070887), oxidation-reduction process (GO: 0055114), fatty acid beta-oxidation (GO: 0006635), and regulation of fatty acid metabolic process (GO:0019217) as being enriched (FDR < 0.01). Furthermore, three cellular components including “mitochondrion,” “TOR complex1,” and “mitochondrial membrane,” were enriched (FDR < 0.01). The PPAR signaling pathway, adipocytokine signaling, FoXO signaling, mTOR signaling pathway, insulin signaling pathway, MAPK signaling pathway, fatty acid degradation, fatty acid metabolism, GnRH signaling pathway, ErbB signaling pathway, and oxidative phosphorylation were also indicated as enriched KEGG pathways for the avUCP gene network (FDR < 0.05).
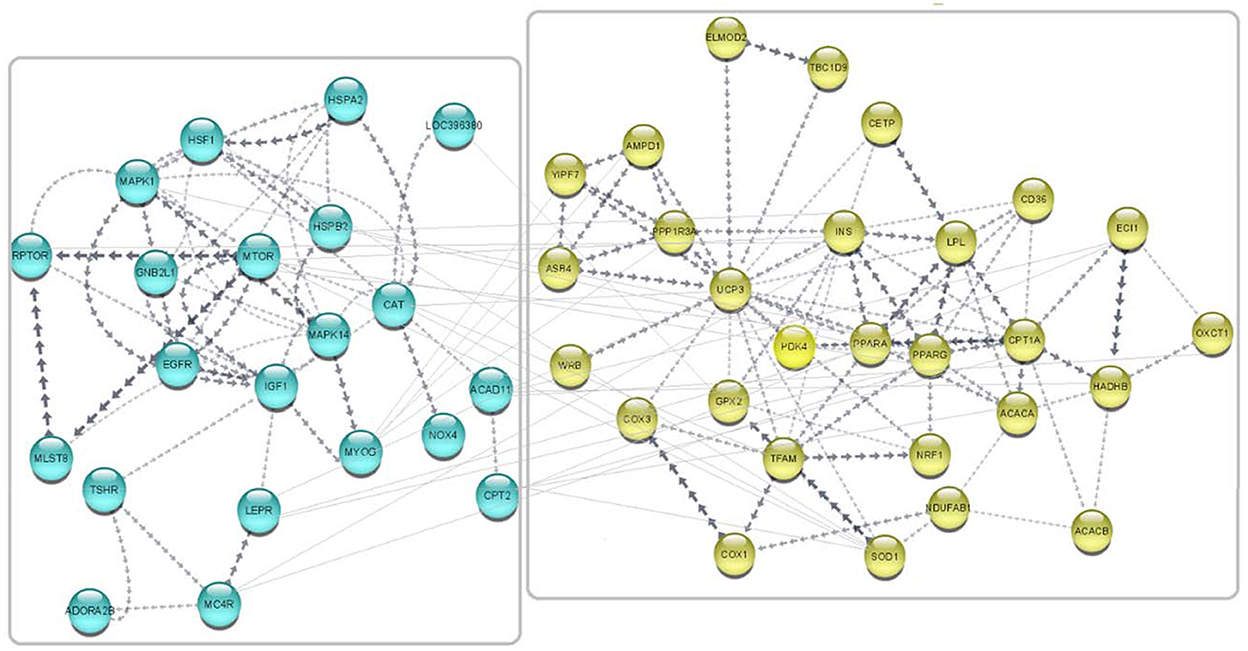
Figure 8. Predicted network for the regulatory and collaborative genes with avUCP. Separate boxes show clusters. Blue nodes represent genes involved in response to stress (cold and free radicals) (cluster 1). Yellow nodes represent genes involved in lipid metabolism and proton buffering system (cluster 2).
3.6. Pathway crosstalk
After a query of enriched pathways, we used XtalkDB (75) to predict which pairs of signaling pathways may crosstalk with each other. The six enriched pathways were predicted to be involved in a network of crosstalk which is depicted in Figure 9. In detail, adipocytokine signaling was revealed to have an activation effect on MAPK, insulin, and ErbB signaling pathways, and both activation and inhibition effects on the mTOR signaling pathway.
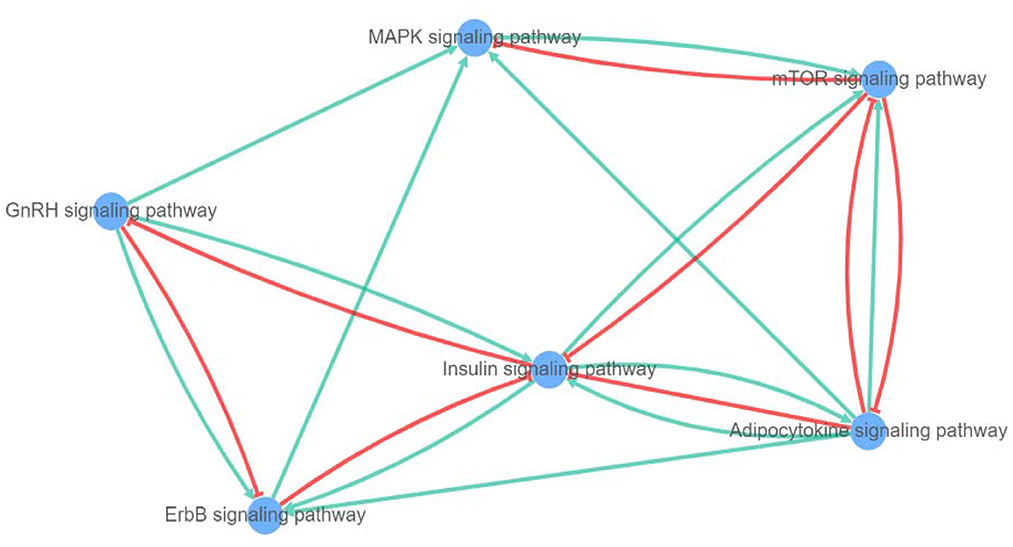
Figure 9. Network of crosstalk among enriched pathways (blue lines show activation and red lines represent inhibition effect).
The mTOR signaling pathway is predicted to act as an inhibitor for both MAPK and insulin signaling pathways. Among these, the MAPK showed only an activation effect on the mTOR pathway. Moreover, the insulin signaling pathway is anticipated to induce adipocytokine, mTOR, and ErbB signaling pathways, alongside inhibited GnRH signaling pathway. The GnRH signaling pathway is shown to be involved in activation of adipocytokine and MAPK signaling pathways and both negative and positive crosstalk with ErbB signaling pathway. In addition, the ErbB signaling pathway can activate and silence MAPK and insulin signaling pathways, respectively.
4. Discussion
4.1. Coding sequence analysis
4.1.1. Codon usage analysis
In the investigation of codon usage, the RSCU value is a ratio between the occurrence frequency of a certain codon and the expected usage frequency for codons (78). Codons encoding amino acids of avUCP with RSCU values higher than 1.0 represent positive codon usage bias and codons with RSCU values lower than 1 display negative codon usage bias. Moreover, codons with RSCU = 0 display unfavorable codons. According to the results, the avUCP gene obviously prefers codons with “C” and “G” in the third position over the other bases. It can be concluded that almost all highly preferred codons, except the CAU codon (only in manakin), which encodes for histidine, end with a “C” or a “G.”
However, the mechanisms of inducing codon biases remained an open question. It can be attributed to the expression level of genes, selective pressure, evolutionary trend, phylogenetic relations of organisms, and genetic drift (79–81).
Moreover, the CAI values of 1 and 0 refer to the species in which only the most frequent codons are used, and species using the least frequent codons. Although manakin had the lowest GC ratio among the studied birds, it revealed the highest CAI, indicating that it uses 71.2% of the frequently used codons. Manakin uses most of the synonymous codons (53/61 codons) to encode avUCP so that it has the lowest number of unused codons (8). Interestingly, manakin is the only species among the selected birds which breeds in tropical forests. Therefore, the observed differences in manakin may be a result of adaptation to tropical conditions. Furthermore, the use of a comparatively wide range of synonymous codons alongside the use of frequently-used codons can be regarded as an evolutionary variation to achieve efficient translation in relatively important functional genes.
4.2. Protein sequence analysis
In protein sequence and physio-chemical analysis, a slight variation in amino acids and physio-chemical parameters among the eight studied avUCP proteins was detected. Tyrosine was the only amino acid showing a constant level in all avian species. The range of isoelectric points of UCPs implies that UCPs can be membrane proteins. As the pH of the intermembrane space and the mitochondrial matrix is about 7.0 and 8.0, respectively, thus UCPs carry an electrical charge in that region. Additionally, since aliphatic side chains like alanine, leucine, and valine determine the aliphatic index (AI) of a protein, avUCP, which shows a high content of these amino acids, could be considered thermostable. The highest AI of 100.42 was observed in manakin avUCP which may illustrate the importance of avUCP stability specifically in manakin.
The variation of atomic sulfur count from 14 to 20 among the eight avUCP proteins illustrates another significant difference among avian species. Sulfur can be found in cysteine and methionine amino acids. Nine cysteine residues were observed in avUCP in sparrow but the protein sequence of the others contained eight cysteine residues. Furthermore, the hydrophobic methionine was variable among avUCPs, which could be the second source of variability in atomic sulfur count among avUCP sequences in the studied species. Sulfur-containing amino acids are responsible for stronger connections than aliphatic and aromatic amino acids. Thus, they can provide a more sustainable 3D structure representing functional specificity in membrane proteins by creating a disulfide bond (77, 82). The percentage of hydrophobic and hydrophilic amino acids in the studied sequences were very close to each other indicating that these chemical characteristics play important roles in the encoded avUCP protein. The higher potential of hydrophobicity according to GRAVY indices above 0 is another appropriate state for transmembrane proteins. If a protein plays an important functional role, the state of its hydrophobicity and hydrophilicity will remain stable as much as possible, for the conservation of its function. Also, the stability of positively and negatively charged amino acids among all studied avUCPs may imply the effectiveness of charged regions of this protein which needs to be conserved among birds.
4.2.1. Entropy analysis
The positions of aligned sequences in the entropy plot can correlate with the structural and chemical characteristics of certain amino acids and their influence on the function of avUCPs. Therefore, regions that contain residue positions with low entropy are more likely to be involved in the functional sites of avUCP (83, 84). Moreover, the absence of any region with more than 10 consecutive amino acids with an average entropy of >1 can be concluded as evidence of conservation in avUCPs.
4.3. Protein structure prediction
Because of the high frequency of alanine, leucine, valine, and glycine, and the low frequency of histidine and tryptophan in all avUCPs, the level of alpha-helical structure was determined higher than in other secondary structures. In agreement with our result, it is already known that transmembrane regions of proteins contain a high level of alpha-helices devoid of polar amino acids, while extracellular and cytoplasmic regions of the protein are usually enriched with polar amino acids like tyrosine and tryptophan (77).
The Ramachandran parameters of predicted protein can display the statistical distribution of the combinations of torsional Phi and Psi angles in the avUCP protein structure (85). Moreover, rotamers in protein structure imply conformational isomers of amino acid residues in the sidechain of avUCP, therefore, rotamer outliers display conformations that drop outside the reference (86). Also, C-beta deviation can reflect misfit conformation and inconsistency between sidechain and protein backbone that can be used for structure validation (87). In the current structural modeling for avUCP, the two C-beta deviations have resulted from valine and proline amino acids in positions 56 and 50, respectively, which were predicted to be in the cytoplasmic region of avUCP.
Hence, in addition to regulatory hormones and elements, different innate parameters can affect gene expression patterns of any pleiotropic genes like avUCP, with those parameters including, codon usage, GC content, CpG dinucleotide content, splicing sites, CpG islands, mRNA secondary structure, coding sequences (CDS), ribosomal binding sites, stimulators, the expression of other genes, along with environmental conditions (78, 88). For example, previous research has revealed cysteine residues of UCPs can be glutathionylated (89–92). They suggest that reactive oxygen species and glutathionylation can regulate non-phosphorylating respiration. Mailloux et al. (93) have identified Cys25 and Cys259 as the probable glutathionylation sites on UCPs (93). Interestingly, in the current study Cys25 and Cys257 were determined as conserved sites and predicted to be located in transmembrane and cytoplasmic regions of avUCP, respectively.
4.4. Sequence-based gene ontology prediction
The sequence-based predicted biological processes of “mitochondrial transmembrane transport,” “proton transmembrane transport,” “adaptive thermogenesis,” and “response to cold” for avUCP are congruent with results from previous studies. In this regard, some studies support the involvement of avUCP in avian energy expenditure and adaptive thermogenesis (1, 15–18). Additionally, it should be mentioned that cold acclimation can not only induce fatty acid-mediated uncoupling of oxidative phosphorylation processes but also increases the rate of ADP and Pi concentrations, along with ATP synthesis in the mitochondria of chicken skeletal muscle, which seems to be a counterproductive occurrence in response to cold stress condition in birds (34). Moreover, Ueda et al. reported a correlation between uncoupling and both exogenous and endogenous fatty acids in the mitochondria of chicken skeletal muscle during cold temperatures (94). Another study conducted on king penguins showed that superoxide activates the proton transport of mitochondria and GDP inhibits the transport of the superoxide-activated-proton, demonstrating that avUCP mediates mitochondrial proton transport but plays no role in the basal proton leak (6). Because thermogenic hormones have an induction effect on avUCP expression, the involvement of avUCP in avian thermogenesis can be concluded (16).
4.5. Interactive network prediction and gene-based enrichment analysis
Through the network of gene-gene interactions, centralized by avUCP and using previously recognized avUCP- related genes (5, 34, 42, 56–71, 94), we have found two major clusters of genes pointing to the overall functionality of response to stress and lipid metabolism/proton buffering. Our well-categorized findings are in agreement with the outputs of other studies. Previously conducted studies revealed the predominant presence of avUCP protein in skeletal muscles (pectoral, glycolytic fibers) (90) alongside recognizing the alteration in expression pattern during different physiological states [cold stress (10, 12, 34, 58, 90), heat stress (12, 29, 92, 95, 96), transfer stress (97), high fat diet, and fat (16, 38, 66, 95)], that clearly reflect the involvement of avUCP in fatty acid β-oxidation and cell metabolism. Moreover, one study reported that a high concentration of chemical stimulus like ammonia can be effective in the expression of 12 energy metabolism-related genes (avUCP, HK1, HK2, PK, PFK, PDHX, CS, LDHA, LDHB, SDHA, SDHB, and AMPK), in chicken liver. Otherwise, it was reported that ammonia gas resulted in mitochondrial damage, ATPase reduction, and ultimately reduction of energy release in the chicken liver (98).
4.6. Pathway crosstalk
Finally, among 11 enriched pathways, interaction of five signaling pathways including MAPK, adipocytokine, mTOR, insulin, ErbB, and GnRH was predicted, indicating a possible combination of positive and negative feedback among pathways to regulate avUCP functions. In general, biological pathway crosstalk refers to the different feedback in seemingly distinct pathways (99). Consequently, it seems that maintaining a delicate balance of avUCP functions such as lipid metabolism, thermogenesis, response to cold, and response to ROS can occur by crosstalk between involved pathways. Moreover, when a single gene is considered in depth, within a network of genes, all potential regulatory interferences will emerge. Additionally, it is also known that the interaction between pathways can regulate specific gene expression (100, 101).
A panoply of changes in the primary sequence of avUCPs can potentially be involved in changes to protein function and expression through alteration of the final structure of the avUCP molecule. Accumulation of findings represents avUCP as an essential gene for whole-body energy balance, adaptive thermogenesis, and antioxidant defense in birds. This study contributes to a better understanding of avUCP characterization, function, and critical signaling pathways for evaluating how it is regulated in avian species exposed to different conditions. Additionally, the present study provides putative functions for avUCPs, and indicates some of the genes, pathways, and mechanisms that are involved in fine-tuning mitochondrial oxidative phosphorylation.
In conclusion, we have compared the sequence, structure and physio-chemical properties of avUCP in 8 bird species and determine the functional pathways and networks in which avUCP is involved. Oxidative stress in birds is known as one of the most energy-demanding events influencing energy expenditure, the balance of detoxification of free radicals, and oxidative phosphorylation, so avUCP could be viewed as a significant marker for developing heat-stress-resistant breeds in future genomic selection programmes.
Data availability statement
The data presented in the study (nucleotide coding sequences and amino acid sequences of avian uncoupling proteins in FASTA format) are deposited at https://figshare.com/ with the accession number https://figshare.com/search?q=10.6084%2Fm9.figshare.20518086 and are also presented in Table 1.
Author contributions
PD and MG-Z conceived the overall design, undertook the project management, contributed to the interpretation of results, and critically revised the manuscript. PD carried out the analyses and drafted the manuscript. MD, MR, SK, and EE performed the review part of this research, collecting a set of genes used for gene network analysis and provided support in the analyses. JS revised the entire manuscript, contributed to the interpretation of results, and critically reviewed the manuscript. All authors read and approved the final manuscript.
Funding
This work was funded in part by Biotechnology and Biological Sciences Research Council (BBSRC) grant number BBS/E/D/10002071.
Acknowledgments
The authors are grateful for the insightful comments offered by the peer reviewers at the Journal of Frontiers in Veterinary Science. The authors would also wish to acknowledge Dr. Helene Hayes and Jack Dekkers for their support and encouragement. For the purpose of open access, the author has applied a Creative Commons Attribution (CC BY) licence to any Author Accepted Manuscript version arising from this submission.
Conflict of interest
The authors declare that the research was conducted in the absence of any commercial or financial relationships that could be construed as a potential conflict of interest.
Publisher's note
All claims expressed in this article are solely those of the authors and do not necessarily represent those of their affiliated organizations, or those of the publisher, the editors and the reviewers. Any product that may be evaluated in this article, or claim that may be made by its manufacturer, is not guaranteed or endorsed by the publisher.
Abbreviations
ADP, Adenosine diphosphate; AI, Aliphatic index; AMPK, AMP-activated protein kinase; ATP, Adenosine triphosphate; CAI, Codon Adaptation Index; cDNA, Complementary DNA; CDS, Coding sequence; CS, Citrate synthase; CU, Codon usage; FDR, False discovery rate; GDP, Guanosine diphosphate; GO, Gene ontology; HK1, Hexokinase 1; HK2, Hexokinase 2; II, Instability index; IL-6, Interleukin 6; LDHA, Lactate dehydrogenase A; LDHB, Lactate dehydrogenase B; mRNA, Messenger RNA; PDHX, Pyruvate dehydrogenase complex component X; PFK, Phosphofructokinase; PGC-1α, Peroxisome proliferator-activated receptor γ coactivator-1α; Pi, Inorganic phosphate; PK, Protein kinase; PPARGC1A, PPARG coactivator 1 alpha; PPARs, Peroxisome proliferator-activated receptors; ROS, Reactive oxygen species; RSCU, Relative Synonymous Codon Usage; SDHA, Succinate dehydrogenase complex flavoprotein subunit A; SDHB, Succinate dehydrogenase complex iron sulfur subunit B; SLC25A4, Solute Carrier Family 25 Member 4; TNFα, Tumor necrosis factor alpha; TORC1, TOR complex 1; UCP, Uncoupling Protein.
References
1. Raimbault S, Dridi S, Denjean F, Lachuer J, Couplan E, Bouillaud F, et al. An uncoupling protein homologue putatively involved in facultative muscle thermogenesis in birds. Biochem J. (2001) 353:441–5. doi: 10.1042/bj3530441
2. Darzi Niarami M, Masoudi AA, Vaez Torshizi R, Davoodi P. A novel mutation in the promoter region of avian uncoupling protein3 associated with feed efficiency and body composition traits in broiler chicken. J Worlds Poult Res. (2020) 10:87–95. doi: 10.36380/jwpr.2020.12
3. Beltran DF, Shultz AJ, Parra JL. Speciation rates are positively correlated with the rate of plumage color evolution in hummingbirds. Evolution. (2021) 75:1665–80. doi: 10.1111/evo.14277
4. Vianna C, Hagen T, Zhang C-Y, Bachman E, Boss O, Gereben B, et al. Cloning and functional characterization of an uncoupling protein homolog in hummingbirds. Physiol Genomics. (2001) 5:137–45. doi: 10.1152/physiolgenomics.2001.5.3.137
5. Evock-Clover CM, Poch SM, Richards MP, Ashwell CM, Mcmurtry JP. Expression of an uncoupling protein gene homolog in chickens. Comp Biochem Physiol B Biochem. (2002) 133:345–58. doi: 10.1016/S1095-6433(02)00113-7
6. Talbot DA, Hanuise N, Rey B, Rouanet JL, Duchamp C, Brand MD. Superoxide activates a GDP-sensitive proton conductance in skeletal muscle mitochondria from king penguin (Aptenodytes patagonicus). Biochem Biophys Res Commun. (2003) 312:983–8. doi: 10.1016/j.bbrc.2003.11.022
7. Dridi S, Onagbesan O, Swennen Q, Buyse J, Decuypere E, Taouis M. Gene expression, tissue distribution and potential physiological role of uncoupling protein in avian species. Comp Biochem Physiol A Mol Integr Physiol. (2004) 139:273–83. doi: 10.1016/j.cbpb.2004.09.010
8. Duval E. Age-based plumage changes in the Lance-tailed Manakin: a two-year delay in plumage maturation. Condor. (2009) 107:915–20. doi: 10.1093/condor/107.4.915
9. Garratt M, Brooks RC. Oxidative stress and condition-dependent sexual signals: more than just seeing red. Proc Biol Sci. (2012) 279:3121–30. doi: 10.1098/rspb.2012.0568
10. Wollenberg Valero KC, Pathak R, Prajapati I, Bankston S, Thompson A, Usher J, et al. A candidate multimodal functional genetic network for thermal adaptation. Peer J. (2014) 2:e578. doi: 10.7717/peerj.578
11. Davoodi P, Ehsani A, Vaez Torshizi R, Masoudi AA. New insights into genetics underlying of plumage color. Anim Genet. (2021) 53:80–93. doi: 10.1111/age.13156
12. Mota-Rojas D, Titto CG, De Mira Geraldo A, Martínez-Burnes J, Gómez J, Hernández-Ávalos I, et al. Efficacy and function of feathers, hair, and glabrous skin in the thermoregulation strategies of domestic animals. Animals. (2021) 11:3472. doi: 10.3390/ani11123472
13. Hohtola E, Henderson RP, Rashotte ME. Shivering thermogenesis in the pigeon: the effects of activity, diurnal factors, and feeding state. Am J Physiol Regul Integr Comp Physiol AM. (1998) 275:R1553–62. doi: 10.1152/ajpregu.1998.275.5.R1553
14. Bicudo JE, Vianna CR, Chaui-Berlinck JG. Thermogenesis in birds. Biosci Rep. (2001) 21:181–8. doi: 10.1023/A:1013648208428
15. Taouis M, De Basilio V, Mignon-Grasteau S, Crochet S, Bouchot C, Bigot K, et al. Early-age thermal conditioning reduces uncoupling protein messenger RNA expression in pectoral muscle of broiler chicks at seven days of age. Poult Sci. (2002) 81:1640–3. doi: 10.1093/ps/81.11.1640
16. Collin A, Taouis M, Buyse J, Ifuta NB, Darras VM, Van As P, et al. Thyroid status, but not insulin status, affects expression of avian uncoupling protein mRNA in chicken. Am J Physiol Endocrinol. (2003) 284:E771–8. doi: 10.1152/ajpendo.00478.2002
17. Collin A, Cassy S, Buyse J, Decuypere E, Damon M. Potential involvement of mammalian and avian uncoupling proteins in the thermogenic effect of thyroid hormones. Domest Anim Endocrinol. (2005) 29:78–87. doi: 10.1016/j.domaniend.2005.02.007
18. Mujahid A. Acute cold-induced thermogenesis in neonatal chicks (Gallus gallus). Comp Biochem Physiol Part A Mol Integr Physiol. (2010) 156:34–41. doi: 10.1016/j.cbpa.2009.12.004
19. Swennen Q, Delezie E, Collin A, Decuypere E, Buyse J. Further investigations on the role of diet-induced thermogenesis in the regulation of feed intake in chickens: comparison of age-matched broiler versus layer cockerels. Poult Sci. (2007) 86:895–903. doi: 10.1093/ps/86.5.895
20. Teulier L, Rouanet JL, Letexier D, Romestaing C, Belouze M, Rey B, et al. Cold-acclimation-induced non-shivering thermogenesis in birds is associated with upregulation of avian UCP but not with innate uncoupling or altered ATP efficiency. J Exp Biol. (2010) 213:2476–82. doi: 10.1242/jeb.043489
21. Rey B, Halsey LG, Dolmazon V, Rouanet J-L, Roussel D, Handrich Y, et al. Long-term fasting decreases mitochondrial avian UCP-mediated oxygen consumption in hypometabolic king penguins. Am J Physiol Regul Integr Comp Physiol. (2008) 295:92–100. doi: 10.1152/ajpregu.00271.2007
22. Jin S, Yang L, He T, Fan X, Wang Y, Ge K, et al. Polymorphisms in the uncoupling protein 3 gene and their associations with feed efficiency in chickens. Asian Australas J Anim Sci. (2018) 31:1401–6. doi: 10.5713/ajas.18.0217
23. Abe T, Mujahid A, Sato K, Akiba Y, Toyomizu M. Possible role of avian uncoupling protein in down-regulating mitochondrial superoxide production in skeletal muscle of fasted chickens. FEBS Lett. (2006) 580:4815–22. doi: 10.1016/j.febslet.2006.07.070
24. Criscuolo F, Mozo J, Hurtaud C, Nübel T, Bouillaud F. UCP2, UCP3, avUCP, what do they do when proton transport is not stimulated? Possible relevance to pyruvate and glutamine metabolism. Biochim Biophys Acta. (2006) 1757:1284–91. doi: 10.1016/j.bbabio.2006.06.002
25. Mujahid A, Sato K, Akiba Y, Toyomizu M. Acute heat stress stimulates mitochondrial superoxide production in broiler skeletal muscle, possibly via downregulation of uncoupling protein content. Poult Sci. (2006) 85:1259–65. doi: 10.1093/ps/85.7.1259
26. Mujahid A, Akiba Y, Toyomizu M. Acute heat stress induces oxidative stress and decreases adaptation in young white leghorn cockerels by downregulation of avian uncoupling protein. Poult Sci. (2007) 86:364–71. doi: 10.1093/ps/86.2.364
27. Del Vesco AP, Gasparino E. Production of reactive oxygen species, gene expression, and enzymatic activity in quail subjected to acute heat stress. J Anim Sci. (2013) 91:582–7. doi: 10.2527/jas.2012-5498
28. Kikusato M, Toyomizu M. Crucial role of membrane potential in heat stress-induced overproduction of reactive oxygen species in avian skeletal muscle mitochondria. PLoS ONE. (2013) 8:e64412. doi: 10.1371/journal.pone.0064412
29. Lu Z, He X, Ma B, Zhang L, Li J, Jiang Y, et al. Chronic heat stress impairs the quality of breast-muscle meat in broilers by affecting redox status and energy-substance metabolism. J Agric Food Chem. (2017) 65:11251–8. doi: 10.1021/acs.jafc.7b04428
30. Xu L, Wu SG, Zhang HJ, Zhang L, Yue HY, Ji F, et al. Comparison of lipid oxidation, messenger ribonucleic acid levels of avian uncoupling protein, avian adenine nucleotide translocator, and avian peroxisome proliferator-activated receptor-γ coactivator-1α in skeletal muscles from electrical- and gas-stunned broilers. Poult Sci. (2011) 90:2069–75. doi: 10.3382/ps.2011-01348
31. Liu J-S, Chen Y-Q, Li M. Thyroid hormones increase liver and muscle thermogenic capacity in the little buntings (Emberiza pusilla). J Therm Biol. (2006) 31:386–93. doi: 10.1016/j.jtherbio.2006.01.002
32. Ferver A, Dridi S. Regulation of avian uncoupling protein (av-UCP), expression by cytokines and hormonal signals in quail myoblast cells. Comp Biochem Physiol Part A Mol Integr Physiol. (2020) 248:110747. doi: 10.1016/j.cbpa.2020.110747
33. Bal NC, Periasamy M. Uncoupling of sarcoendoplasmic reticulum calcium ATPase pump activity by sarcolipin as the basis for muscle non-shivering thermogenesis. Philos Trans R Soc Lond, B, Biol Sci. (2020) 375:20190135. doi: 10.1098/rstb.2019.0135
34. Toyomizu M, Ueda M, Sato S, Seki Y, Sato K, Akiba Y. Cold-induced mitochondrial uncoupling and expression of chicken UCP and ANT mRNA in chicken skeletal muscle. FEBS Lett. (2002) 529:313–8. doi: 10.1016/S0014-5793(02)03395-1
35. Walter I, Seebacher F. Endothermy in birds: underlying molecular mechanisms. J Exp Biol. (2009) 212:2328–36. doi: 10.1242/jeb.029009
36. Collin A, Buyse J, Van As P, Darras VM, Malheiros RD, Moraes VM, et al. Cold-induced enhancement of avian uncoupling protein expression, heat production, and triiodothyronine concentrations in broiler chicks. Gen Comp Endocrinol. (2003) 130:70–7. doi: 10.1016/S0016-6480(02)00571-3
37. Zheng S, Zhao J, Xing H, Xu S. Oxidative stress, inflammation, and glycometabolism disorder-induced erythrocyte hemolysis in selenium-deficient exudative diathesis broilers. J Cell Physiol. (2019). doi: 10.1002/jcp.28298
38. Collin A, Swennen Q, Skiba-Cassy S, Buyse J, Chartrin P, Le Bihan-Duval E, et al. Regulation of fatty acid oxidation in chicken (Gallus gallus): interactions between genotype and diet composition. Comp Biochem Physiol B. (2009) 153:171–7. doi: 10.1016/j.cbpb.2009.02.012
39. Joubert R, Métayer Coustard S, Swennen Q, Sibut V, Crochet S, Cailleau-Audouin E, et al. The beta-adrenergic system is involved in the regulation of the expression of avian uncoupling protein in the chicken. Domest Anim Endocrinol. (2010) 38:115–25. doi: 10.1016/j.domaniend.2009.08.002
40. Do PH, Tran PV, Bahry MA, Yang H, Han G, Tsuchiya A, et al. Oral administration of a medium containing both D-aspartate-producing live bacteria and D-aspartate reduces rectal temperature in chicks. Br Poult Sci. (2017) 58:569–77. doi: 10.1080/00071668.2017.1335858
41. Kikusato M, Muroi H, Uwabe Y, Furukawa K, Toyomizu M. Oleuropein induces mitochondrial biogenesis and decreases reactive oxygen species generation in cultured avian muscle cells, possibly via an up-regulation of peroxisome proliferator-activated receptor γ coactivator-1α. Anim Sci J. (2016) 87:1371–8. doi: 10.1111/asj.12559
42. Muroi H, Hori K, Tokutake Y, Hakamata Y, Kawabata F, Toyomizu M, et al. Oleuropein suppresses mitochondrial reactive oxygen species generation possibly via an activation of transient receptor potential V1 and sirtuin-1 in cultured chicken muscle cells. Anim Sci J. (2022) 93:e13677. doi: 10.1111/asj.13677
43. Lassiter K, Greene E, Piekarski A, Faulkner OB, Hargis BM, Bottje W, et al. Orexin system is expressed in avian muscle cells and regulates mitochondrial dynamics. Am J Physiol Regul Integr Comp Physiol. (2015) 308:173–87. doi: 10.1152/ajpregu.00394.2014
44. Criscuolo F, Gonzalez-Barroso MDM, Maho YL, Ricquier D, Bouillaud F. Avian uncoupling protein expressed in yeast mitochondria prevents endogenous free radical damage. Proc Royal Soc B. (2005) 272:803–10. doi: 10.1098/rspb.2004.3044
45. Yalin E, Hurtaud C, Ricquier D, Bouillaud F, Hughes J, Criscuolo F. Avian UCP: the killjoy in the evolution of the mitochondrial uncoupling proteins. J Mol Evol. (2007) 65:392–402. doi: 10.1007/s00239-007-9020-1
46. Tamura K, Stecher G, Kumar S. MEGA11: molecular evolutionary genetics analysis version 11. Mol Biol Evol. (2021) 38:3022–7. doi: 10.1093/molbev/msab120
47. Puigbò P, Bravo IG, Garcia-Vallvé S. E-CAI: a novel server to estimate an expected value of Codon Adaptation Index (eCAI). BMC Bioinform. (2008) 9:65. doi: 10.1186/1471-2105-9-65
48. Qiagen. CLC Genomics Workbench 20.0 (QIAGEN). (2016). Available online at: www.qiagenbioinformatics.com (accessed June 14, 2022).
49. Alzohairy A. BioEdit: an important software for molecular biology. GERF Bull Biosci. (2011) 2:60–1.
50. Wheeler TJ, Clements J, Finn RD. Skylign: a tool for creating informative, interactive logos representing sequence alignments and profile hidden Markov models. BMC Bioinformatics. (2014) 15:7. doi: 10.1186/1471-2105-15-7
51. Geourjon C, Deléage G. SOPMA: significant improvements in protein secondary structure prediction by consensus prediction from multiple alignments. Comput Appl Biosci. (1995) 11:681–4. doi: 10.1093/bioinformatics/11.6.681
52. Kelley LA, Mezulis S, Yates CM, Wass MN, Sternberg MJE. The Phyre2 web portal for protein modeling, prediction and analysis. Nat Protoc. (2015) 10:845–58. doi: 10.1038/nprot.2015.053
53. Waterhouse A, Bertoni M, Bienert S, Studer G, Tauriello G, Gumienny R, et al. SWISS-MODEL: homology modelling of protein structures and complexes. Nucleic Acids Res. (2018) 46:296–303. doi: 10.1093/nar/gky427
54. Bernhofer M, Dallago C, Karl T, Satagopam V, Heinzinger M, Littmann M, et al. Predict protein – predicting protein structure and function for 29 years. BioRxiv. (2021). doi: 10.1093/nar/gkab354
55. Davoodi P, Ehsani A. In-silico investigation of genomic regions related to ascites and identifying their pathways in broilers. World's Poult Sci J. (2019) 75:193–206. doi: 10.1017/S0043933919000035
56. Fromme T, Reichwald K, Platzer M, Li X-S, Klingenspor M. Chicken ovalbumin upstream promoter transcription factor II regulates uncoupling protein 3 gene transcription in Phodopus sungorus. BMC Mol Biol. (2007) 8:1. doi: 10.1186/1471-2199-8-1
57. Dridi S, Temim S, Derouet M, Tesseraud S, Taouis M. Acute cold- and chronic heat-exposure upregulate hepatic leptin and muscle uncoupling protein (UCP), gene expression in broiler chickens. J Exp Zool A Ecol Genet Physiol. (2008) 309:381–8. doi: 10.1002/jez.461
58. Wang JT, Zhang XJ, Xu SW. Effects of cold stress on energy metabolism in the chicken. J Appl Physiol. (2009) 25:172–6.
59. Cai Y, Song Z, Wang X, Jiao H, Lin H. Dexamethasone-induced hepatic lipogenesis is insulin dependent in chickens (Gallus gallus domesticus). Stress. (2011) 14:273–81. doi: 10.3109/10253890.2010.543444
60. Sibut V, Hennequet-Antier C, Le Bihan-Duval E, Marthey S, Duclos MJ, Berri C. Identification of differentially expressed genes in chickens differing in muscle glycogen content and meat quality. BMC Genom. (2011) 12:112. doi: 10.1186/1471-2164-12-112
61. Cui HX, Liu RR, Zhao GP, Zheng MQ, Chen JL, Wen J. Identification of differentially expressed genes and pathways for intramuscular fat deposition in pectoralis major tissues of fast-and slow-growing chickens. BMC Genom. (2012) 13:213. doi: 10.1186/1471-2164-13-213
62. Ji B, Ernest B, Gooding JR, Das S, Saxton AM, Simon J, et al. Transcriptomic and metabolomic profiling of chicken adipose tissue in response to insulin neutralization and fasting. BMC Genom. (2012) 13:441. doi: 10.1186/1471-2164-13-441
63. Li Q, Xu Z, Liu L, Yu H, Rong H, Tao L, et al. Effects of breeds and dietary protein levels on the growth performance, energy expenditure and expression of avUCP mRNA in chickens. Mol Biol Rep. (2013) 40:2769–79. doi: 10.1007/s11033-012-2030-0
64. Saneyasu T, Shiragaki M, Nakanishi K, Kamisoyama H, Honda K. Effects of short term fasting on the expression of genes involved in lipid metabolism in chicks. Comp Biochem Physiol B, Biochem Mol Biol. (2013) 165:114–8. doi: 10.1016/j.cbpb.2013.03.005
65. Hicks JA, Porter TE, Liu HC. Identification of microRNAs controlling hepatic mRNA levels for metabolic genes during the metabolic transition from embryonic to posthatch development in the chicken. BMC Genom. (2017) 18:687. doi: 10.1186/s12864-017-4096-5
66. Desert C, Baéza E, Aite M, Boutin M, Le Cam A, Montfort J, et al. Multi-tissue transcriptomic study reveals the main role of liver in the chicken adaptive response to a switch in dietary energy source through the transcriptional regulation of lipogenesis. BMC Genom. (2018) 19:187. doi: 10.1186/s12864-018-4520-5
67. Chen Y, Zhao Y, Jin W, Li Y, Zhang Y, Ma X, et al. MicroRNAs and their regulatory networks in Chinese Gushi chicken abdominal adipose tissue during postnatal late development. BMC Genom. (2019) 20:778. doi: 10.1186/s12864-019-6094-2
68. Suzuki S, Kobayashi M, Murai A, Tsudzuki M, Ishikawa A. Characterization of growth, fat deposition, and lipid metabolism-related gene expression in lean and obese meat-type chickens. J Poult Sci. (2019) 56:101–11. doi: 10.2141/jpsa.0180064
69. Cogburn LA, Trakooljul N, Wang X, Ellestad LE, Porter TE. Transcriptome analyses of liver in newly-hatched chicks during the metabolic perturbation of fasting and re-feeding reveals THRSPA as the key lipogenic transcription factor. BMC Genom. (2020) 21:109. doi: 10.1186/s12864-020-6525-0
70. Pirany N, Bakrani Balani A, Hassanpour H, Mehraban H. Differential expression of genes implicated in liver lipid metabolism in broiler chickens differing in weight. Br Poult Sci. (2020) 61:10–6. doi: 10.1080/00071668.2019.1680802
71. Dhamad A, Zampiga M, Greene ES, Sirri F, Dridi S. Neuropeptide Y and its receptors are expressed in chicken skeletal muscle and regulate mitochondrial function. Gen Comp Endocrinol. (2021) 310:113798. doi: 10.1016/j.ygcen.2021.113798
72. Shannon P, Markiel A, Ozier O, Baliga NS, Wang JT, Ramage D, et al. Cytoscape: a software environment for integrated models of biomolecular interaction networks. Genome Res. (2003) 13:2498–504. doi: 10.1101/gr.1239303
73. Szklarczyk D, Gable AL, Lyon D, Junge A, Wyder S, Huerta-Cepas J, et al. STRING v11: protein–protein association networks with increased coverage, supporting functional discovery in genome-wide experimental datasets. Nucleic Acids Res. (2019) 47:607–13. doi: 10.1093/nar/gky1131
74. Szklarczyk D, Gable AL, Nastou KC, Lyon D, Kirsch R, Pyysalo S, et al. The STRING database in 2021: customizable protein–protein networks, and functional characterization of user-uploaded gene/measurement sets. Nucleic Acids Res. (2021) 49:605–12. doi: 10.1093/nar/gkaa1074
75. Sam SA, Teel J, Tegge AN, Bharadwaj A, Murali TM. XTalkDB: a database of signaling pathway crosstalk. Nucleic Acids Res. (2017) 45:432–9. doi: 10.1093/nar/gkw1037
76. Gamage DG, Gunaratne A, Periyannan GR, Russell TG. Applicability of instability index for in vitro protein stability prediction. Protein Pept Lett. (2019) 26:339–47. doi: 10.2174/0929866526666190228144219
77. Mbaye MN, Hou Q, Basu S, Teheux F, Pucci F, Rooman M. A comprehensive computational study of amino acid interactions in membrane proteins. Sci Rep. (2019) 9:12043. doi: 10.1038/s41598-019-48541-2
78. Gun L, Yumiao R, Haixian P, Liang Z. Comprehensive analysis and comparison on the codon usage pattern of whole Mycobacterium tuberculosis coding genome from different area. Biomed Res Int. (2018) 2018:3574976. doi: 10.1155/2018/3574976
79. Suzuki H, Morton BR. Codon adaptation of plastid genes. PLoS ONE. (2016) 11:e0154306. doi: 10.1371/journal.pone.0154306
80. Athey J, Alexaki A, Osipova E, Rostovtsev A, Santana-Quintero LV, Katneni U, et al. A new and updated resource for codon usage tables. BMC Bioinform. (2017) 18:391. doi: 10.1186/s12859-017-1793-7
81. Bahiri-Elitzur S, Tuller T. Codon-based indices for modeling gene expression and transcript evolution. Comput Struct Biotechnol J. (2021) 19:2646–63. doi: 10.1016/j.csbj.2021.04.042
82. Gómez-Tamayo JC, Cordomí A, Olivella M, Mayol E, Fourmy D, Pardo L. Analysis of the interactions of sulfur-containing amino acids in membrane proteins. Protein Sci. (2016) 25:1517–24. doi: 10.1002/pro.2955
83. Reva B, Antipin Y, Sander C. Determinants of protein function revealed by combinatorial entropy optimization. Genome Biol. (2007) 8:R232. doi: 10.1186/gb-2007-8-11-r232
84. Anashkina AA, Petrushanko IY, Ziganshin RH, Orlov YL, Nekrasov AN. Entropy analysis of protein sequences reveals a hierarchical organization. Entropy. (2021) 23:1647. doi: 10.3390/e23121647
85. Hollingsworth SA, Karplus PA. A fresh look at the Ramachandran plot and the occurrence of standard structures in proteins. Biomol Concepts. (2010) 1:271–83. doi: 10.1515/bmc.2010.022
86. Haddad Y, Adam V, Heger Z. Rotamer dynamics: analysis of rotamers in molecular dynamics simulations of proteins. Biophys J. (2019) 116:2062–72. doi: 10.1016/j.bpj.2019.04.017
87. Lovell SC, Davis IW, Arendall WB III, De Bakker PI, Word JM, et al. Structure validation by Calpha geometry: phi, psi and Cbeta deviation. Proteins. (2003) 50:437–50. doi: 10.1002/prot.10286
88. Zhou Z, Dang Y, Zhou M, Li L, Yu CH, Fu J, et al. Codon usage is an important determinant of gene expression levels largely through its effects on transcription. Proc Natl Acad Sci USA. (2016) 113:6117–25. doi: 10.1073/pnas.1606724113
89. Rey B, Spée M, Belouze M, Girard A, Prost J, Roussel D, et al. Oxygen recovery up-regulates avian UCP and ANT in newly hatched ducklings. J Comp Physiol B Biochem Syst Environ Physiol. (2009) 180:239–46. doi: 10.1007/s00360-009-0409-6
90. Rey B, Roussel D, Romestaing C, Belouze M, Rouanet JL, Desplanches D, et al. Up-regulation of avian uncoupling protein in cold-acclimated and hyperthyroid ducklings prevents reactive oxygen species production by skeletal muscle mitochondria. BMC Physiol. (2010) 10:5. doi: 10.1186/1472-6793-10-5
91. Jenni-Eiermann S, Jenni L, Smith S, Costantini D. oxidative stress in endurance flight: an unconsidered factor in bird migration. PLoS ONE. (2014) 9:e97650. doi: 10.1371/journal.pone.0097650
92. Akbarian A, Michiels J, Degroote J, Majdeddin M, Golian A, De Smet S. Association between heat stress and oxidative stress in poultry; mitochondrial dysfunction and dietary interventions with phytochemicals. J Anim Sci Biotechnol. (2016) 7:37. doi: 10.1186/s40104-016-0097-5
93. Mailloux RJ, Seifert EL, Bouillaud F, Aguer C, Collins S, Harper M-E. Glutathionylation acts as a control switch for uncoupling proteins UCP2 and UCP3. JBC. (2011) 286:21865–75. doi: 10.1074/jbc.M111.240242
94. Ueda M, Watanabe K, Sato K, Akiba Y, Toyomizu M. Possible role for avPGC-1alpha in the control of expression of fiber type, along with avUCP and avANT mRNAs in the skeletal muscles of cold-exposed chickens. FEBS Lett. (2005) 579:11–7. doi: 10.1016/j.febslet.2004.11.039
95. Brannan KE, Helfrich KK, Flentke GR, Smith SM, Livingston KA, Jansen Van Rensburg C. Influence of incubation, diet, and sex on avian uncoupling protein expression and oxidative stress in market age broilers following exposure to acute heat stress. Poult Sci. (2022) 101:101748. doi: 10.1016/j.psj.2022.101748
96. Xu R, Yu C, Mao L, Jiang M, Gao L, Li M, et al. Antioxidant defense mechanisms and fatty acid catabolism in Red-billed Leiothrix (Leiothrix lutea) exposed to high temperatures. Avian Re. (2022) 13:100013. doi: 10.1016/j.avrs.2022.100013
97. Zhang L, Yue HY, Wu SG, Xu L, Zhang HJ, Yan HJ, et al. Transport stress in broilers. II. Superoxide production, adenosine phosphate concentrations, and mRNA levels of avian uncoupling protein, avian adenine nucleotide translocator, and avian peroxisome proliferator-activated receptor-gamma coactivator-1alpha in skeletal muscles. Poult Sci. (2010) 89:393–400. doi: 10.3382/ps.2009-00281
98. Li Z, Miao Z, Ding L, Teng X, Bao J. Energy metabolism disorder mediated ammonia gas-induced autophagy via AMPK/mTOR/ULK1-Beclin1 pathway in chicken livers. Ecotoxicol Environ Saf. (2021) 217:112219. doi: 10.1016/j.ecoenv.2021.112219
99. Vert G, Chory J. Crosstalk in cellular signaling: background noise or the real thing? Dev Cell. (2011) 21:985–91. doi: 10.1016/j.devcel.2011.11.006
100. Müller IE, Rubens JR, Jun T, Graham D, Xavier R, Lu TK. Gene networks that compensate for crosstalk with crosstalk. Nat Commun. (2019) 10:4028. doi: 10.1038/s41467-019-12021-y
Keywords: avian uncoupling protein, nucleotide coding sequence, protein structure prediction, codon usage, pathway crosstalk
Citation: Davoodi P, Ghaderi-Zefrehei M, Dolatabady MM, Razmkabir M, Kianpour S, Esfahani EN and Smith J (2023) In silico investigation of uncoupling protein function in avian genomes. Front. Vet. Sci. 9:1085112. doi: 10.3389/fvets.2022.1085112
Received: 31 October 2022; Accepted: 28 December 2022;
Published: 19 January 2023.
Edited by:
Rajesh Kumar Pathak, Chung-Ang University, Republic of KoreaReviewed by:
Joycee Jogi, Nanaji Deshmukh Veterinary Science University, IndiaRohit Shukla, Jaypee University of Information Technology, India
Ravendra Chauhan, Rhodes University, South Africa
Copyright © 2023 Davoodi, Ghaderi-Zefrehei, Dolatabady, Razmkabir, Kianpour, Esfahani and Smith. This is an open-access article distributed under the terms of the Creative Commons Attribution License (CC BY). The use, distribution or reproduction in other forums is permitted, provided the original author(s) and the copyright owner(s) are credited and that the original publication in this journal is cited, in accordance with accepted academic practice. No use, distribution or reproduction is permitted which does not comply with these terms.
*Correspondence: Mostafa Ghaderi-Zefrehei, mosmos741@yahoo.com;
mghaderi@yu.ac.ir; Jacqueline Smith,
jacqueline.smith@roslin.ed.ac.uk
†ORCID: Peymaneh Davoodi orcid.org/0000-0002-7037-2956
Mostafa Ghaderi-Zefrehei orcid.org/0000-0002-9710-883X
Mustafa Muhaghegh Dolatabady orcid.org/0000-0003-4876-1514
Mohammad Razmkabir orcid.org/0000-0003-2445-2899
Somayeh Kianpour orcid.org/0000-0002-3990-6585
Effat Nasre Esfahani orcid.org/0000-0002-5445-4759
Jacqueline Smith orcid.org/0000-0002-2813-7872