- 1College of Veterinary Medicine, Sichuan Agricultural University, Chengdu, China
- 2Key Laboratory of Animal Diseases and Human Health of Sichuan Province, Chengdu, Sichuan, China
Background: Glässer's disease, caused by Haemophilus parasuis (HPS), is responsible for economic losses in the pig industry worldwide. However, the existing commercial vaccines offer poor protection and there are significant barriers to the development of effective vaccines.
Methods: In the current study, we aimed to identify potential vaccine candidates and design a multi-epitope vaccine against HPS by performing pan-genomic analysis of 121 strains and using a reverse vaccinology approach.
Results: The designed vaccine constructs consist of predicted epitopes of B and T cells derived from the outer membrane proteins of the HPS core genome. The vaccine was found to be highly immunogenic, non-toxic, and non-allergenic as well as have stable physicochemical properties. It has a high binding affinity to Toll-like receptor 2. In addition, in silico immune simulation results showed that the vaccine elicited an effective immune response. Moreover, the mouse polyclonal antibody obtained by immunizing the vaccine protein can be combined with different serotypes and non-typable Haemophilus parasuis in vitro.
Conclusion: The overall results of the study suggest that the designed multi-epitope vaccine is a promising candidate for pan-prophylaxis against different strains of HPS.
Introduction
The disease caused by Haemophilus parasuis (HPS), known as Glässer's disease, is characterized by fibrinous polyserositis and arthritis (Figures 1, 2). It is one of the main infectious diseases in the day-old isolated farming model of the global pig industry and causes significant economic losses (1). H. parasuis strains are heterogeneous in terms of phenotypic and genotypic traits. Strains have been classified into 15 serotypes, but a large proportion of isolates remain non-typable (2). Currently, vaccination is the main measure for preventing HPS infection. Commercially available inactivated bacterin vaccines are based on serovar 5, a combination of serovars 4 and 5, or a combination of serovars 1 and 6. However, all these vaccine products showed limited cross-protection against heterologous strains. There is often even failure to achieve the desired effect in protection against different isolates of the same serotype (3). Moreover, the protection against non-typable strains remains elusive. In addition, more than one strain of HPS is often present in a pig farm. For example, 4–5 strains can be isolated from a herd at a given time, and up to 16 different strains can be isolated in a single pig farm during one production cycle (4–6). This epidemiological feature also poses a great challenge for the selection of HPS vaccines in the breeding process.
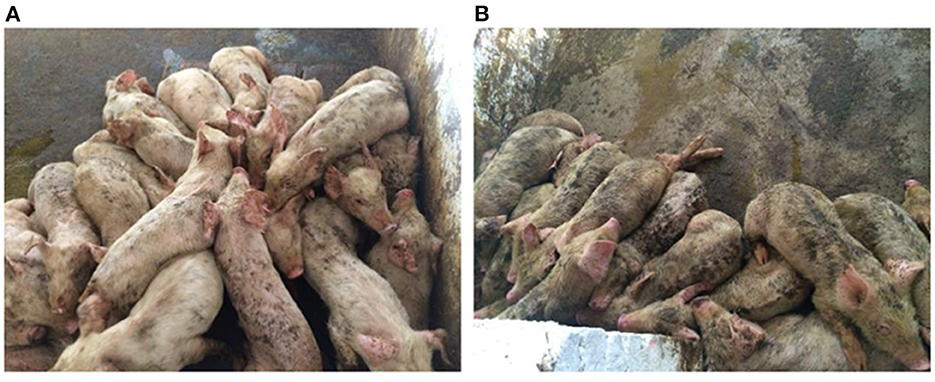
Figure 1. Group of nursery pigs diagnosed with Glässer's disease. (A,B) Pigs gather in the corner of the pen to protect themselves from the cold, their bodies are dirty, and their coats are ragged. Photographs taken by the author.
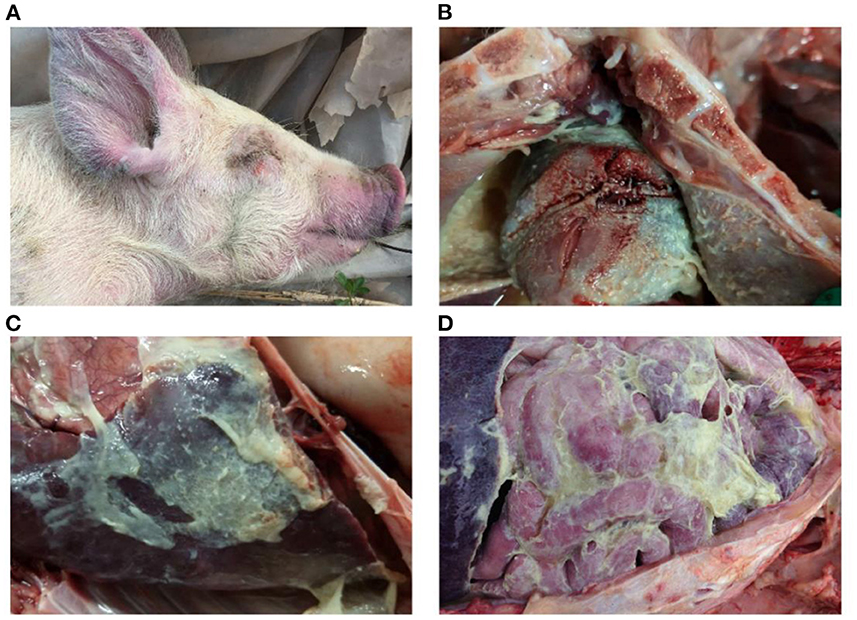
Figure 2. Gross lesions of Glässer's disease: (A) purple marks on the ears, skin around the eyes and tip of the nose of a pig that died of H. parasuis infection; (B) fibrinopurulent exudate on pericardial surface; and (C,D) fibrinopurulent exudate on serosal membranes in peritoneal and thoracic cavities. Photographs taken by the author.
Given the challenges faced by inactivated bacterial vaccines for treatment of HPS described above, the use of reverse vaccinology to develop protein vaccines against protective epitopes of the pathogen is a viable strategy. Reverse vaccinology involves computer programs to identify antigenic epitopes based on bacterial genome sequence information for vaccine development and design, avoiding the disadvantages of traditional vaccine design which is expensive and time consuming (7, 8). Moreover, with the constant updating of sequencing technologies, sequence information of bacterial genomes can be obtained at a low cost and in a short time, which also reduces the time required for vaccine design.
Hence, in this study, we used pan genome analysis to identify the core genome of HPS. Then, in silico prediction of B and T cell epitopes of outer membrane proteins in the core genome were performed to design a multi-epitope vaccine. An adjuvant was also ligated with to the vaccine to enhance the immunogenicity of the vaccine to obtain the final multi-epitope vaccine construct. Subsequently, the antigenicity and physicochemical properties of the vaccine construct were estimated. In addition, the secondary and tertiary structures of the construct were predicted and the interaction of the vaccine with Toll-like receptor 2 was assessed by molecular docking simulations. Finally, immune simulations were performed to confirm the immune potential of the vaccine construct and a vector was constructed for its expression in E. coli. The application potential of multi-epitope vaccine was preliminarily tested in mouse immunization test. Thus, in this work, a multi-epitope vaccine candidate was created using a novel vaccine design strategy based on pan-genomic analysis and reverse vaccinology techniques that will also help to accelerate the development of vaccines against other pathogens.
Materials and methods
Bacterial strains
In this study, we retrieved the complete genome of 105 HPS strains with rich geographical, virulent and serological diversity, which were available in March 2020 from NCBI (ftp://ftp.ncbi.nih.gov/genomes/all/). Information about the 105 strains is summarized in Supplementary Table 1.
In addition, 16 clinical strains isolated in Sichuan between 2015 and 2020 were sequenced. DNA was extracted from overnight culture using an E.Z.N.A Bacterial DNA Kit (OMEGA) following the manufacturer's guidelines and sequenced using the Min-ION MK1B platform. Raw ONT reads were corrected using Canu (9) (v1.5 https://github.com/marbl/canu, accessed on 15 October 2020) and SMARTdenovo (https://github.com/ruanjue/smartdenovo, accessed on 15 October 2020) was then used to assemble the error-corrected reads to obtain the assembled genome sequence. Lastly, Medaka (v1.0.1https://github.com/nanoporetech/medaka, accessed on 15 October 2020) and Homopolish (10) (v0.2.1 https://github.com/ythuang0522/homopolish, accessed on 15 May 2021) was used to perform multiple rounds of polishing for draft assemblies. One hundred and fifteen HPS strains were isolated from clinical cases with Glässer disease, and six strains were from the upper respiratory tract of healthy pigs.
Pan-genomic and phylogenetic analysis
To maintain the consistency and reliability of gene prediction and annotation, a standard software tool the Prokaryotic Genome Annotation System (Prokka) pipeline (11) (v1.14.5 https://github.com/tseemann/prokka, accessed on 5 September 2021) was uniformly applied to all the 121 HPS genomes. Based on the GFF3 files produced by Prokka, the Roary program (12) (https://github.com/sanger-pathogens/Roary, accessed on 6 October 2021) was used to carry out the pan-genomic analysis to identify core and accessory genes with a minimum percentage identity of 95% between each predicted protein homolog. Then, a NJ (neighbor-joining) tree was constructed according to the core genes of HPS strains using MEGA (13) with 1,000 bootstrap replications.
Selection of protein sequences for vaccine designing
The protein sequences of core genes and soft-core genes were extracted based on the results of the pan-genomic analysis. Then, the SignalP 5.0 server (14) was used to analyze for the presence of signal peptides (https://services.healthtech.dtu.dk/service.php?SignalP-5.0, accessed on 3 November 2021) and differentiate between secretory and non-secretory proteins. The subcellular localization of secreted proteins was further checked on Vaxign (http://www.violinet.org/vaxign/, accessed on 3 November 2021) to select outer membrane proteins as candidates for vaccine construction (15).
Prediction of B-cell epitopes
B cells are a central component of the adaptive immune system, and they provide long-term protection against infectious pathogens by producing antibodies. In this study, linear B-cell epitopes of the candidate proteins were predicted by BepiPred-2.0 web server (16) (https://services.healthtech.dtu.dk/service.php?BepiPred-2.0, accessed on 4 November 2021).
Prediction of cytotoxic T-lymphocyte (CTL) and helper T-lymphocyte (HTL) epitopes
The cytotoxic T-lymphocyte (CTL) epitopes from candidate protein sequences were predicted using the NetCTL 1.2 server (https://services.healthtech.dtu.dk/service.php?NetCTL-1.2, accessed on 4 November 2021). Default settings were used (threshold, 0.75) for the estimation of CTL epitopes (17). Then, the helper T cells 15-mer epitopes for candidate protein sequences were predicted by using the NetMHCII 2.3 server (https://services.healthtech.dtu.dk/service.php?NetMHCII-2.3, accessed on 4 November 2021). Seven mouse H2 class II alleles were evaluated. According to standards, the lowest consensus scores of the peptides were chosen to be the best binders and a lower percentile rank indicates higher affinity. The selection criterion was a cut-off of IC50 ≤ 50 and percentile rank <1 (18).
Vaccine construction
A putative vaccine candidate sequence was designed by combining B-cell epitopes with high-scoring CTL epitopes and high binding affinity HTL epitopes. TLR-2 agonist, phenol soluble modulin α4 (accession no. A9JX08) protein, was preferred as an adjuvant to enhance the immunogenicity of the vaccine (19). The adjuvant was linked to the first B-cell epitope through an EAAAK linker at the N terminal of the sequence, whereas the remaining B-cell and HTL epitopes were interlinked via GPGPG linkers. AAY linkers were used for joining the CTLs epitopes.
Evaluation of antigenicity, allergenicity, and physicochemical properties of the protein
In order to predict the antigenicity and allergenicity of the vaccine, VaxiJen v2.0 server (http://www.ddg-pharmfac.net/vaxijen/VaxiJen/VaxiJen.html, accessed on 5 November 2021) and AllerTOP v2.0 server (http://www.ddg-pharmfac.net/AllerTOP, accessed on 5 November 2021) were utilized, respectively (20, 21). The solubility of the designed vaccine was evaluated using the SOLpro server (22) (https://scratch.proteomics.ics.uci.edu, accessed on 5 November 2021). Furthermore, the designed vaccine was assessed for several physicochemical properties by using the ProtParam server (http://web.expasy.org/protparam/, accessed on 5 November 2021).
Extrapolation of secondary structure of the protein
The secondary structure of the multi-epitope vaccine was predicted using PSIPRED (http://bioinf.cs.ucl.ac.uk/psipred/, accessed on 6 November 2021) server and RaptorX (http://raptorx.uchicago.edu/StructurePropertyPred/predict/, accessed on 6 November 2021) with default parameters (23, 24).
Three-dimensional modeling and validation of the protein
Homology modeling of the final vaccine construct was performed using the Robetta server (25) (https://robetta.bakerlab.org/, accessed on 7 November 2021). Non-bond interactions between different types of atoms were analyzed using the ERRAT server (26) (http://services.mbi.ucla.edu/ERRAT/, accessed on 7 November 2021) to verify the tertiary structure. The Ramachandran plot was generated using the PROCHECK server (27) (https://servicesn.mbi.ucla.edu/PROCHECK/, accessed on 7 November 2021) to determine the relative proportion of amino acids in favored regions.
Prediction of discontinuous B-cell Epitopes
ElliPro (http://tools.iedb.org/ellipro/, accessed on 7 November 2021) was used for prediction of B-cell discontinuous epitopes in the protein model (28).
Molecular docking of the protein with TLR2
Toll-like receptors are sensors of the innate immune response, with TLR-2 recognizing the broadest range of PAMPs molecules and inducing significant antibacterial and antiviral responses. We used the ClusPro server (https://cluspro.bu.edu/login.php, accessed on 8 November 2021) to determine the docking of TLR-2 with the vaccine protein (29). The structural coordinates of the TLR-2 (PDB ID: 3A7C) were retrieved from the Protein Data Bank (30) (https://www.rcsb.org/, accessed on 8 November 2021). The docked structures were visualized via PyMOL (http://www.pymol.org, accessed on 8 November 2021) to analyze the interaction between the vaccine protein and TLR-2.
Characterization of the construct immune profile
For analyses of the immune responses of the vaccine construct in the mouse model, the online dynamic immune simulation C-lmmSim server (https://www.iac.cnr.it/~filippo/c-immsim/index.html, accessed on 9 November 2021) was employed (31). This online server functions based on a position specific scoring matrix (PSSM) for the prediction of immunogenic epitopes and immune interactions. All default simulation parameters were used with time steps specified at 1, 90, and 180.
In silico optimization and cloning of the protein
To ensure the efficient expression of the vaccine construct in Escherichia coli cells, we performed the reverse translation and codon optimization of the vaccine protein sequence using the Java Codon Adaptation Tool (32) (http://www.jcat.de/, accessed on 9 November 2021). E. coli strain K12 was selected as the expression host. During the run, the options were chosen to avoid rho-independent transcription terminators, bacterial ribosome binding sites, and restriction enzyme cleavage sites. Finally, the vaccine protein sequence was designed for cloning into a suitable host vector pET-28a(+)-MEV by employing the SnapGene software (https://www.snapgene.com/, accessed on 9 November 2021).
Inducible expression and purification of vaccine proteins
The synthetic expression vector named pET-28a(+)-MEV is available from Chengdu YouKang Jianxing Biotechnology Co. The pET-28a(+)-MEV was transformed into E. coli BL21 (DE3), and positive colonies were inoculated in LB liquid medium containing kanamycin and incubated overnight in a water bath shaker at 37°C. A 10 mL aliquot of overnight culture was inoculated into 1 L of LB medium containing kanamycin, and the culture was expanded to mid-log growth (OD600 ≈ 0.6) at 37°C in a water bath shaker. IPTG was added to the culture and incubated at 37°C for 5 h. The bacteria were collected by centrifugation and ultrasonically fragmented. After sonication, the vaccine protein was purified using a Ni2+-NTA resin column and concentrated by desalting using an Amicon® Ultra-4 10K centrifuge filter.
Mouse immunoassay and ELISA assay
Ten 6-week-old SPFKM grade Kunming mice were randomly divided into test and control groups of five mice each. The vaccine protein was diluted to 1 μg/μL and 50 μL was mixed with an equal volume of Quick Antibody immunoadjuvant. A 100 μL aliquot of the vaccine solution was intramuscularly injected into the hind legs of mice, which were immunized again with the same dose on day 14. The control group was injected with a mixture of saline and immune adjuvant. Blood was collected from the tail vein of the mice at 21 days post-immunization and the serum was isolated and preserved. Sixteen clinical isolates of HPS were coated onto ELISA plates and the absorbance was measured at OD450 and the data recorded. Statistical analysis and visualization of the data were carried out using R 4.1.
Results
Nanopore sequencing and genome assembly of 16 clinical isolates of HPS
To construct a more complete pan-genome of HPS, 16 clinical isolates of HPS (HPS-1–HPS-15) were sequenced using the ONT MinION MK1B platform. Reads of each strain with a depth of about 100 × were obtained. The raw ONT reads were corrected using Canu, and SMARTdenovo was then used to assemble the error-corrected reads to obtain the assembled genome sequence. Then, the raw reads were subjected to for multiple rounds of polishing using Medaka and Homopolish to improve the quality of the draft assemblies. The data that support the findings of this study have been deposited into the CNGB Sequence Archive (CNSA) of China National GeneBank DataBase (CNGBdb) with accession number CNP0002150 (33, 34). Information about the 16 strains is summarized in Supplementary Table 2.
Results of the pan-genomic and phylogenetic analysis
The observed pan-genome shared by the 121 HPS strains consists of 8,885 genes including 390 core genes and 8,495 accessory genes (Figure 3A). The non-linear regression analysis showed an obvious open pan-genome, and the size of core genes approached a constant value (Figure 3B). Based on concatenated core genes, we constructed a phylogenetic tree of the HPS, which showed the rich phylogenetic diversity of HPS (Figure 3C). These results indicate that the genome of HPS is highly variable and that it can continuously obtain foreign genes to adapt to different environments. This is the main reason why conventional vaccines are less protective.
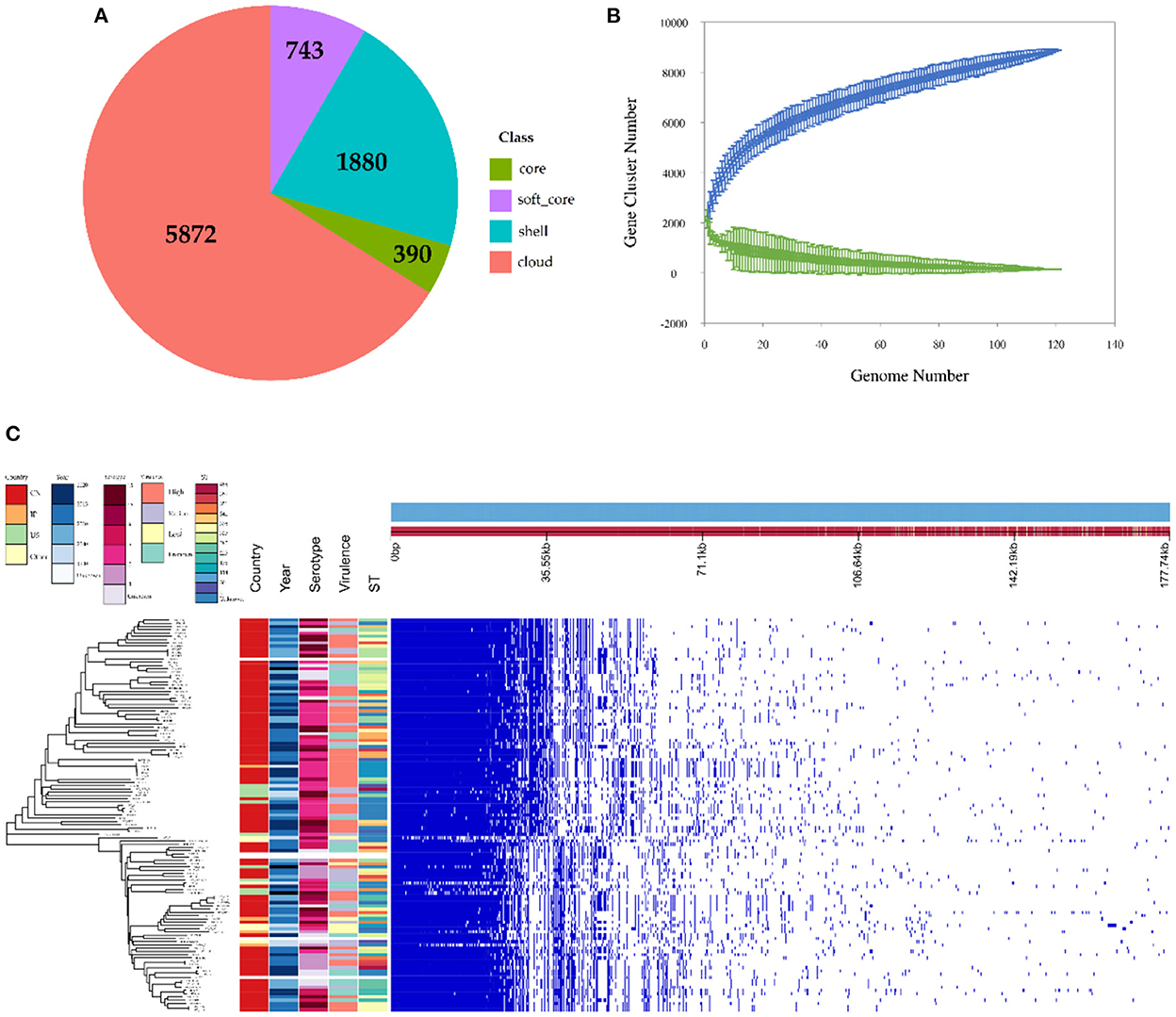
Figure 3. Pan-genomic and phylogenetic analysis of HPS based on 121 strains. (A) Core and accessory genes of HPS. Core: 119 ≤ strains ≤ 121; soft-core: 114≤ strains < 119; shell: 18 ≤ strains < 114; cloud: strains < 18. (B) Core and pan-genome curves for HPS. The blue dots and lines indicate the size of the pan-genome for each strain combination, and the relationship between pan-genome size and genome number, respectively. The green dots and lines indicate corresponding information for the core genome. (C) The left tree is a neighbor-joining phylogenetic tree constructed based on the core genes and annotated with the location of isolation, time of isolation, serotype, ST type, and virulence of each strain. The right matrix plot denotes the presence and absence of every gene over all strains by blue and white, respectively.
Protein sequences for vaccine designing
Eight proteins with secreted signal peptides localized to the outer membrane were screened from the core and soft-core genes using Signal 5.0 and Vaxign (Table 1). These protein sequences were then further subjected to epitope prediction in B, T, and helper cells.
Prediction of B-cell, cytotoxic T-lymphocyte (CTL), and helper T-lymphocyte (HTL) epitopes
BepiPred 2.0 server was used to select B cell epitopes with the default threshold > 0.6. Epitopes that are exposed and have a coiled structure were further selected for vaccine design (Table 2). The protein sequences were analyzed by NetCTL 1.2 server to identify the most immunodominant regions. Peptides with the highest binding affinity scores in each protein were identified as high-potential CTL epitope candidates and a total of three epitopes were screened (Table 2). The NetMHCII 2.3 web server predicted the MHC-II epitopes with the highest binding corresponding to the alleles based on the IC50 score. A total of 6 HTL epitopes were chosen for the final chimeric construct (Table 2).
Vaccine construction
A total of 5 B cell epitopes, six HTL epitopes and three HTL epitopes were used to construct multi-epitope vaccine chimeras. The B cell and HTL epitopes were linked together by GPGPG linkers, while AY linkers were used to link the CTL epitopes. Phenolic soluble modulin α4 (UniProt Id: A9JX08), a TLR-2 agonist, was added as an adjuvant at the N-terminal end with an EAAAK linker to enhance the immunogenicity of the vaccine. The final chimeric construct constituted 280 amino acids (Figure 4).
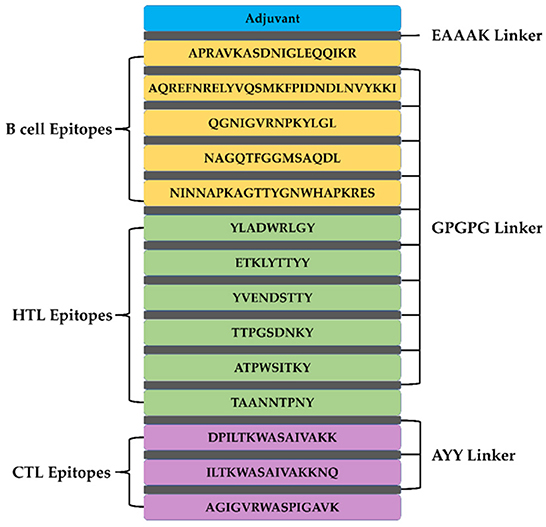
Figure 4. The structural arrangement of B- and T-cell epitopes along with linkers and adjuvant for the final multi-epitope vaccine construct.
Antigenicity, allergenicity, and physicochemical properties of the vaccine protein
VaxiJen 2.0 web server was used to predict the antigenicity of the vaccine design attached with an adjuvant, as 0.7756 with the bacterial model by opting for a threshold of 0.4. The antigenicity of the vaccine candidate was also checked without including the adjuvant part for which VaxiJen gave scores of 0.7114 in a model of bacteria. AllerTOP v.2 and AllergenFP online servers predicted the vaccine sequence to be non-allergenic in nature in the presence and absence of the adjuvant.
Solubility and physicochemical properties of the vaccine protein
The SOLpro server of the Scratch protein prediction tool predicted a solubility probability of 0.887834 for this vaccine protein. ExPASy ProtParam was used to predict the molecular weight (MW) of the vaccine protein as 29.4 kDa. The pI (theoretical isoelectric point value) of the protein was calculated as 9.65. The estimated half-life of the protein in mammals, yeast, and E. coli was estimated as 30 h, >20 h, and >10 h, respectively. In addition, the protein was predicted to have an instability index (II) of 24.99 by ProtParam, classifying it as stable since a value of >40 indicates instability. The values of 70.03 and −0.438 for the aliphatic index and GRAVY (grand average of hydropathicity) reflect the high thermostability and hydrophilic nature, respectively.
Secondary structure of the vaccine protein
Based on PSIPRED and RaptorX server results, the protein vaccine consists of 22% helix (H), 3% beta strand (E), and 73% coil (C) secondary structural elements (Figure 5A). Based on the accessibility of amino acid residues, 62% residues were predicted to be exposed, 18% medium exposed, and 18% were predicted to be buried (Figure 5B). In total, only 2% of residues were found to be localized in disordered structural domains.
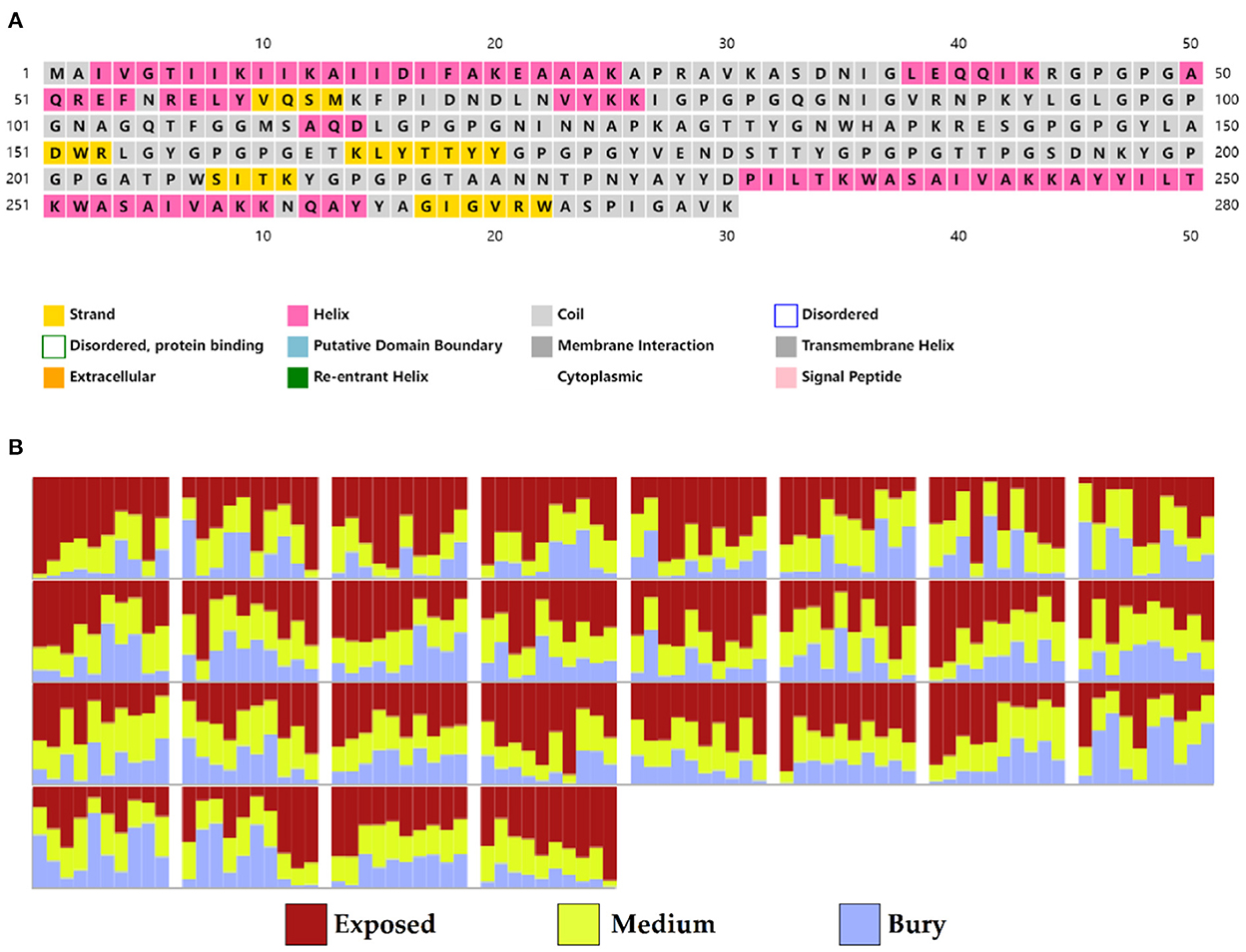
Figure 5. Secondary structure prediction of the vaccine protein sequence by using PSIPRED and RaptorX server showing (A) secondary structural elements and (B) solvent accessibility according to three states.
Three-dimensional modeling and validation of the vaccine protein
The 3D model of the vaccine protein was generated by Robetta server (Figure 6) and was visualized using PyMOL software. Ramachandran plot analysis showed that 100% of the residues were in the allowed regions and 91.1% of the residues were in the most favored regions. The overall quality factor score generated by the ERRAT2 was 92.647%. The above results for the 3D model demonstrate that the vaccine construct has a stable chemical structure.
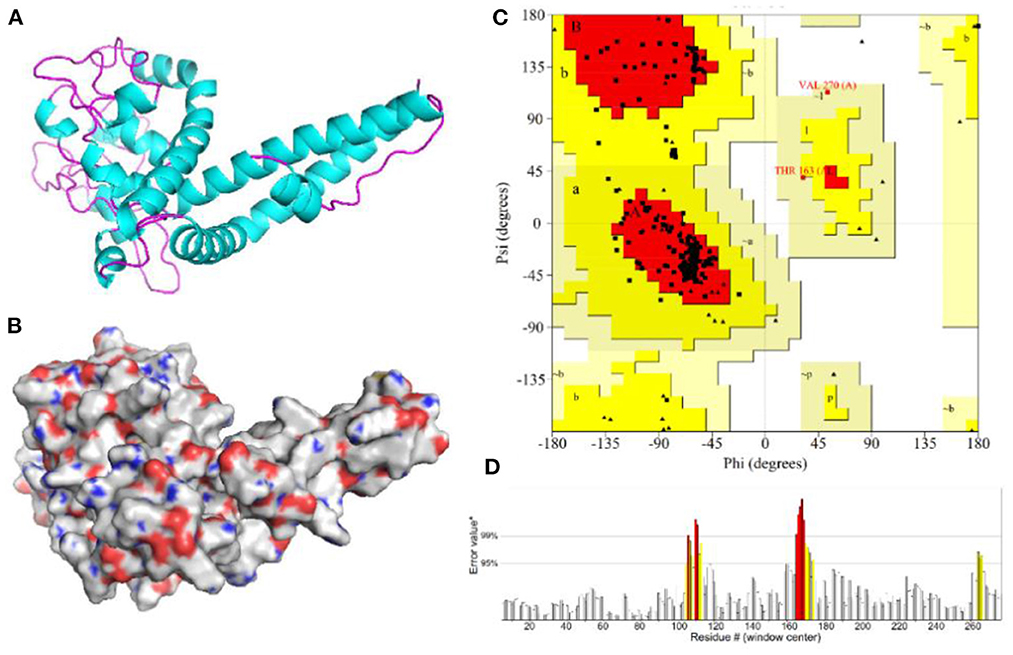
Figure 6. Homology modeling and validation of the three-dimensional structure of vaccine constructs. (A,B) 3D models of vaccine constructs generated by homology modeling on Robetta and visualized by PyMOL software. (C) Ramachandran plot analysis shows 100% of residues in the allowed region. (D) The overall quality factor score for the model was 92.647%.
Prediction of discontinuous B-cell epitopes
ElliPro server was used to predict discontinuous B-cell epitopes for the vaccine constructs. The results showed that the vaccine constructs had five discontinuous B-cell epitopes that ranged in size from 7 to 38 residues, with scores ranging from 0.588 to 0.822 (Figure 7 and Table 3).
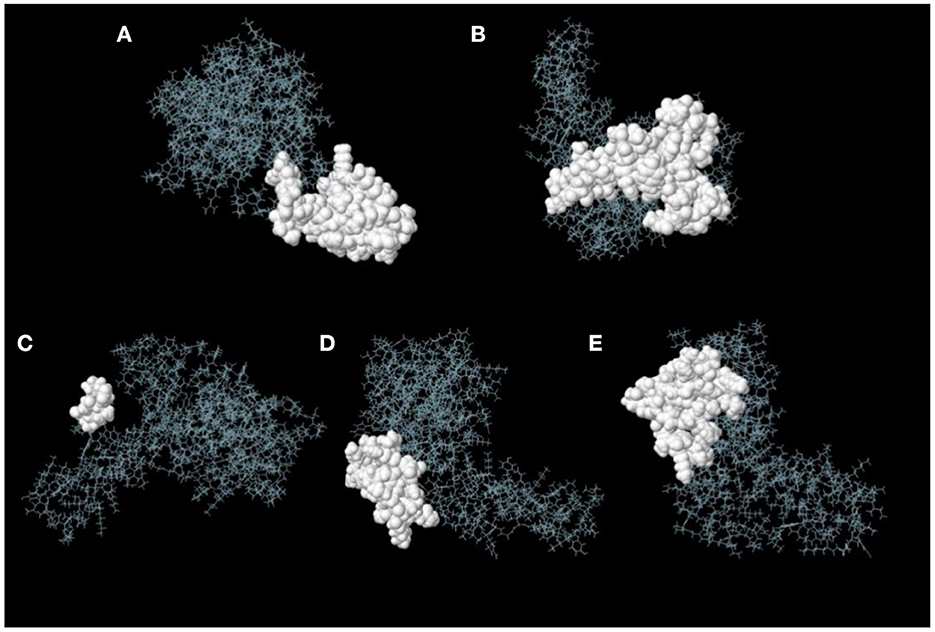
Figure 7. Discontinuous B-cell epitopes of vaccine constructs predicted by ElliPro. (A–E) The epitopes are represented by a white surface. The gray sticks correspond to the remainder of the protein.
Molecular docking of the vaccine protein with TLR2
Immune cells recognize evolutionarily conserved pathogen patterns in a targeted manner and respond by expressing Toll-like receptors. Within the TLR family, TLR2 has a broad recognition spectrum, recognizing lipoproteins, lipopolysaccharides, peptidoglycans and other signals indicating danger (35). Therefore, we used the ClusPro server to determine the docking of TLR 2 with the vaccine protein. The central energy between ligand receptor is −820.2, and the lowest energy of the docking complex is −857.6. The vaccine protein has multiple hydrogen-bonded interactions with TLR2 and exhibits a high binding affinity. The residues of the vaccine-TLR2 complex showing H-bond interactions are LYS25–ASP294, ARG28–GLY293, ARG28–PHE295, ARG271–LEU350, SER274–ASN379, ALA278–ASN379, LYS259–ARG400, LYS259–GLU374, and LYS259–GLU375 with a distance of 2.1, 1.8, 1.8, 2.2, 2.4, 1.6, 2.1, 1.9, and 1.9Å, respectively (Figure 8).
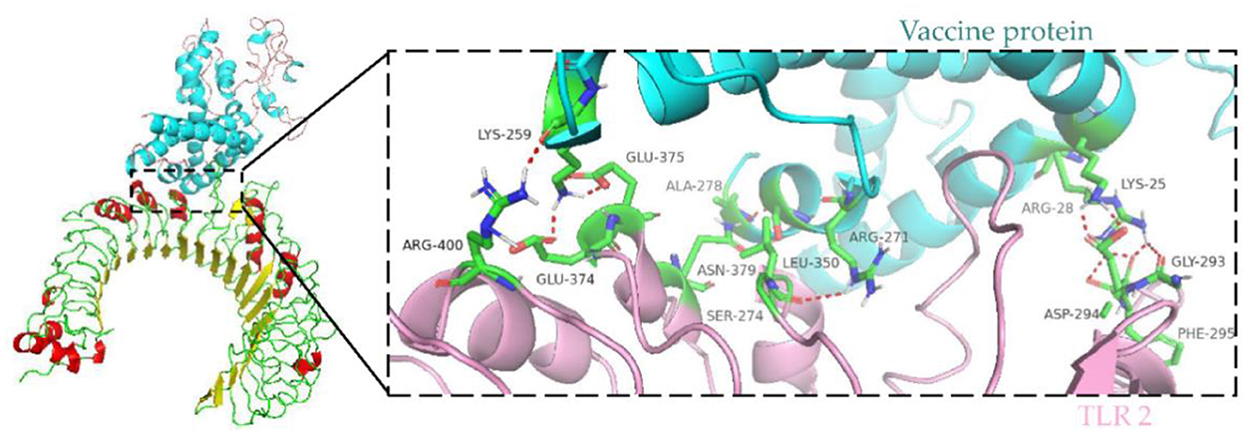
Figure 8. Molecular docking of subunit vaccine with TLR2. Docking complex of vaccine protein and TLR2, with the vaccine protein colored sky blue and the A chain of TLR2 colored light pink. Residues with H-bond interactions are represented in the sticks model and the remaining residues are represented in the cartoon model. Hydrogen bonds are represented as red dashed lines.
Immune simulation of the vaccine construct
Immunological characterization of the designed vaccine construct was analyzed using the C-ImmSim server. The C-ImmSim server immune simulation outcomes confirmed consistency with real immune reactions. As shown in Figure 9A, IgM production was recorded in the first injection of the vaccine construct and increased levels of IgM+IgG and IgG1+IgG2 were observed after the second and third immunizations. High levels of B-cell populations were observed after multiple injections of the vaccine constructs, indicating the formation of immune memory (Figure 9C). In addition, an increase in cytotoxicity and helper T-lymphocytes was observed following vaccination, thus indicating activation of cell-mediated immune responses (Figures 9B,D). The above results demonstrate the ability of the vaccine construct to induce an effective immune response to clear the antigen.
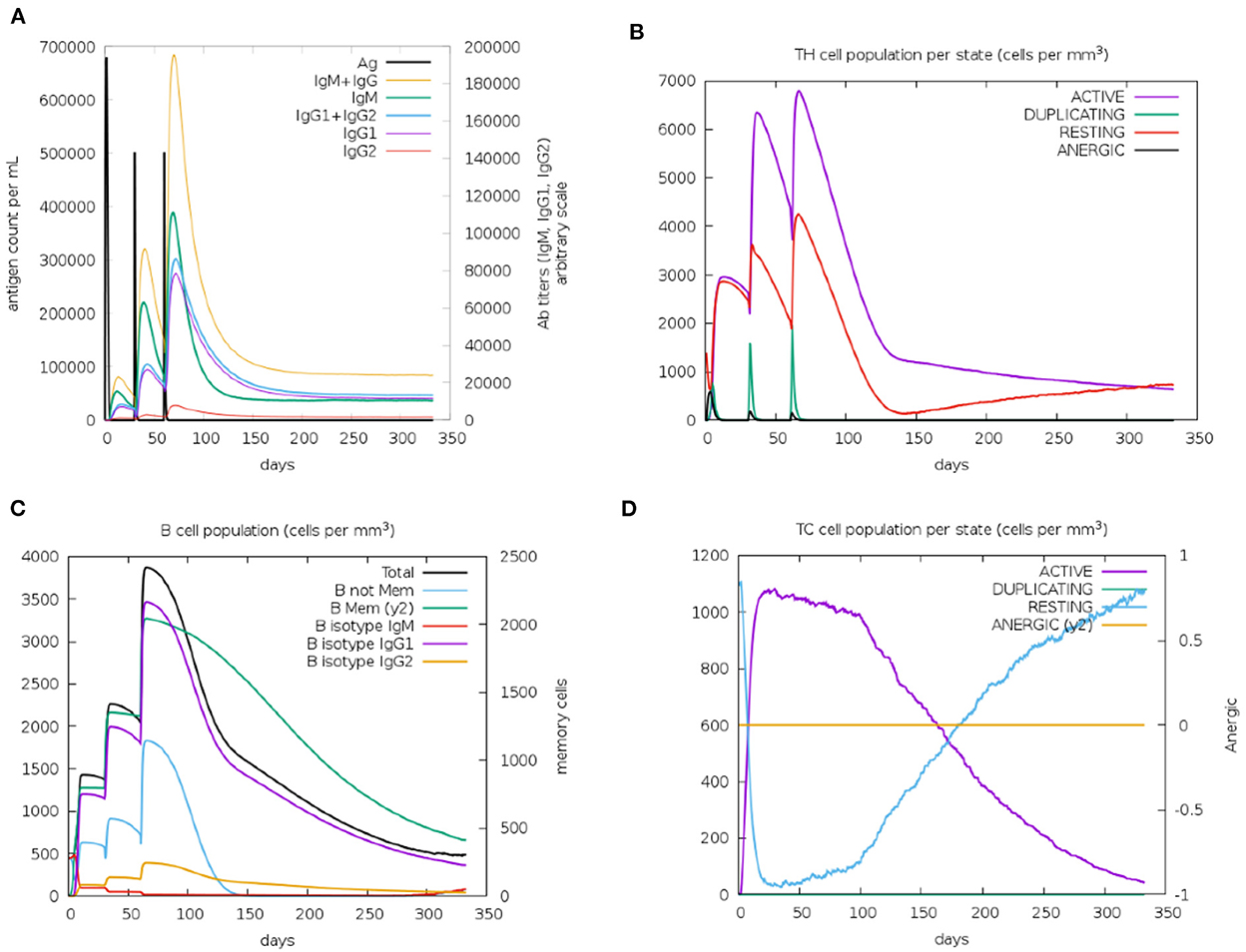
Figure 9. Simulated immunity of vaccine constructs using C-ImmSim server showing (A) an increase in immunoglobulins following vaccination (B,D), that the population of cytotoxic T-lymphocytes and helper T-lymphocytes increases after vaccination and remains higher during the entire exposure time, and (C) an increase in B-cell populations after vaccination, producing high levels of memory immunoglobulins.
Codon optimization and in silico cloning
The Java Codon Adaptation Tool was used for codon optimization. The vaccine protein sequence was reverse translated for optimal expression in E. coli (strain K12). The optimized DNA sequence had a codon adaptation index (CAI) value of 1 and a GC content of 54.05%, indicating that the designed vaccine is theoretically stably expressed in the selected microbial hosts. In addition, the DNA sequence for cloning into the E. coli vector pET-28a(+) was designed using the SnapGene software for recombinant plasmid construction (Figure 10).
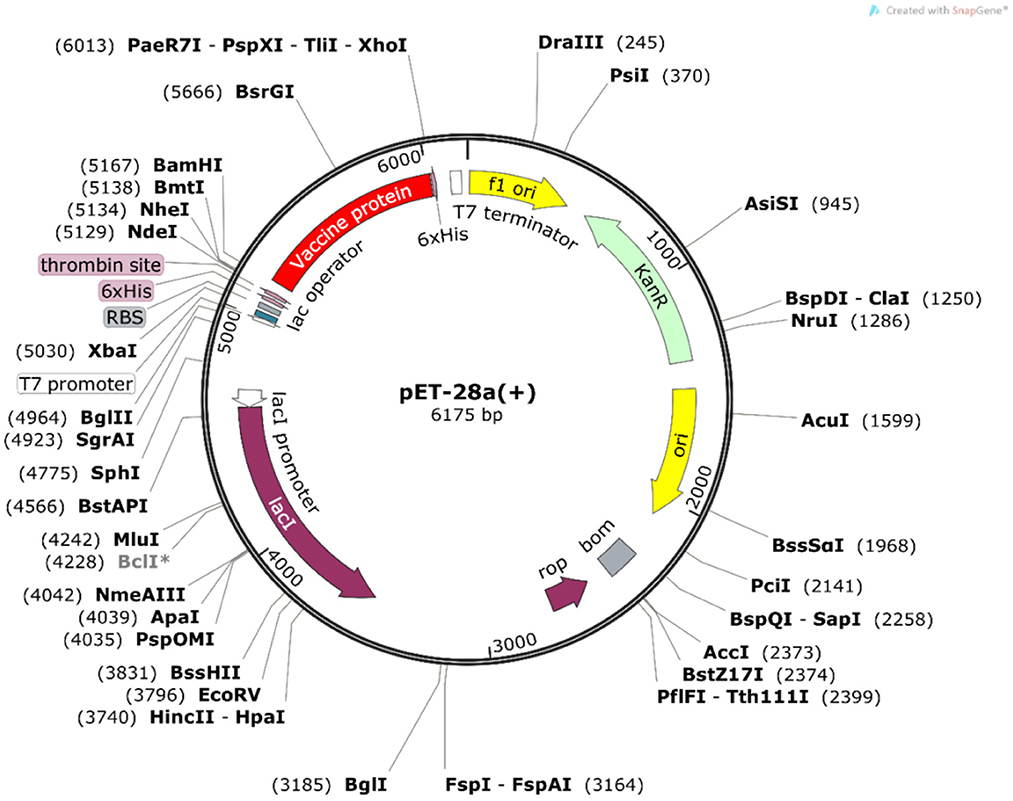
Figure 10. In silico cloning of the vaccine construct. The codon-optimized DNA sequence of the vaccine construct (in red) was cloned between the BamH I and Xho I restriction sites of the pET-28a(+) expression vector (shown in black circles).
Inducible expression and purification of vaccine proteins
IPTG was added to E. coli BL21 (DE3) to induce the expression of pET-28a(+)-MEV, and the product was examined by SDS-PAGE gel electrophoresis. The results showed that the induced pET-28a(+)-MEV recombinant produced a specific protein band around 33 kD as expected (Figure 11), whereas the uninduced sample did not show such a band. The sonicated lysates were purified using a Ni2+-NTA resin column, the eluate was collected and concentrated using an Amicon® Ultra-4 10K centrifuge filter, and the final vaccine protein was stored in 10% glycerol at −80°C.
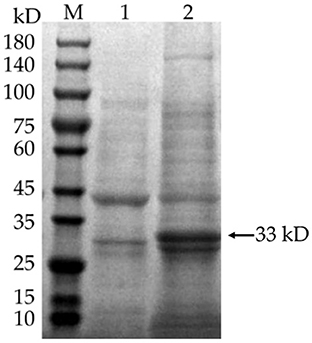
Figure 11. Induced expression of the vaccine protein. M: protein molecular quality standard (10–180 kD); 1: pET-28a(+)-MEV recombinant bacteria, uninduced; 2: pET-28a(+)-MEV recombinant bacteria, induced. Full-length gels are presented in Supplementary Image 1.
ELISA of 16 HPS clinical isolates
Pure cultures of each of the 16 HPS clinical isolates were used to coat enzyme-labeled plates at the same concentration and volume, and ELISA assays were performed using mouse polyclonal antibodies obtained by immunization with a multi-epitope vaccine, with a negative serum control. The results are shown in Table 4 and Figure 12: the mouse polyclonal antibodies obtained were able to bind to all 16 HPS clinical isolates, including seven serotype 5, two serotype 10, one serotype 1, two serotype 7, and four non-typable strains, and a one-tailed heteroscedasticity t-test was performed on the OD450 values of each isolate against the corresponding negative serum OD450 values, with all the p-values being less than the test level (α = 0.05), indicating the differences were significant. These results indicate that the antibodies obtained from mice immunized with the multi-epitope vaccine designed in this study were able to bind to different serotyped or non-typable HPS isolates.
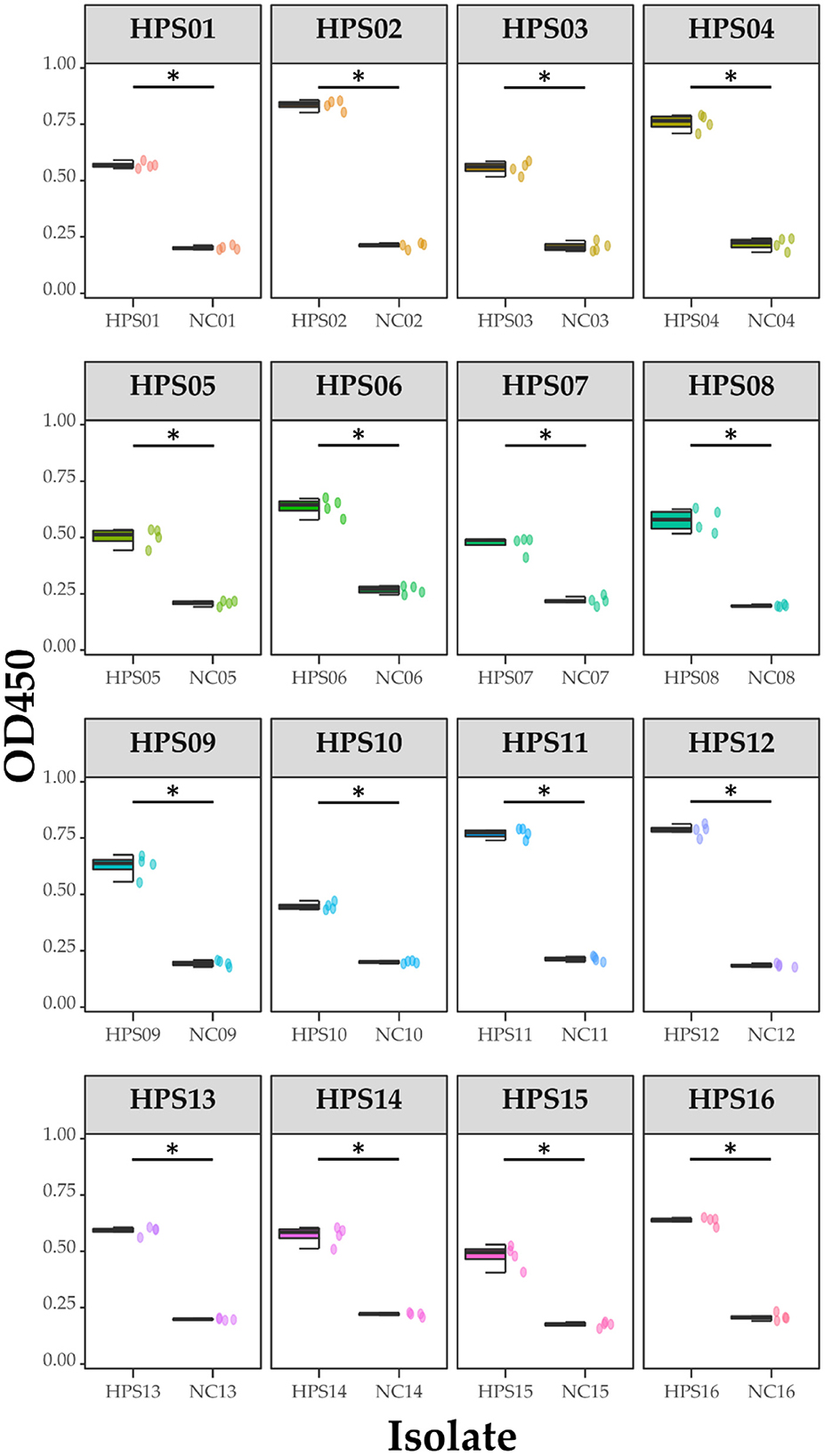
Figure 12. ELISA results for each isolate. Data are presented using box and scatter plots; NC is negative serum control; * indicates statistically significant difference.
Discussion
Haemophilus parasuis mainly causes Glässer's disease, characterized by fibrinous polyserositis and arthritis in nursery pigs and affecting growing pigs and sows. Vaccination is an effective measure to prevent mortality (36, 37). However, the available commercial vaccines only provide protection against a limited number of serotypes. Although autogenous vaccines are highly effective in protecting susceptible pigs, the strains used to prepare the vaccines are not isolated until after a disease outbreak (38). Furthermore, for the treatment of HPS infection, the timing of antibiotic administration is critical, and effective treatment should be administered before fibrinous inflammation develops. However, due to the difficulty of early diagnosis, the sick pigs have usually developed severe fibrinous inflammation by the time of diagnosis. Even if the pathogen has been killed by antibiotics, the pigs may still die due to the inflammation-induced cytokine storm and irreversible histopathological damage (39), and conventional antibiotic treatment is often ineffective. Therefore, this study aims to design a multi-epitope vaccine that is expected to provide broad protection against HPS infection in pig farms (all serotypes and non-typable strains). Pan-genomic analysis was used to identify the core genome of HPS, software programs and databases were used to predict the antigenic epitopes of outer membrane proteins in the core genome, and reverse vaccinology techniques were used to design the final multi-epitope vaccine, reducing traditional laboratory-based experimental practices.
Herein, we first sequenced the genomes of 16 HPS strains using Oxford nanopore sequencing technology (ONT) and obtained complete assemblies of high quality. Compared with second-generation sequencing platforms, the ONT platform has the advantages of lower cost, faster sequencing speed, longer read length, and greater ease of operation (40). Subsequently, the 16 HPS genomes obtained together with the 105 NCBI-indexed genomes were used to construct the HPS pangenome using Roary software. The analysis results showed that HPS has an open pan-genome. The number of core genes represents only 14% of the CDS of an individual genome, and the rest is highly variable. The large variation in the genomes of different isolates of HPS is the main reason for the lack of cross-protection of existing vaccines. It is also worth clarifying that not only virulent strains but also non-virulent strains were used to construct the pan-genome. The reason for this is that non-virulent strains have the potential to be converted into virulent strains. For example, serovar 7 strains were considered to all be non-virulent, but some serovar 7 strains have been isolated from systemic lesions of Glässer's disease, and disease has been reproduced with one of them (41, 42).
The following eight outer membrane proteins were identified from core and soft core genes by signal peptide prediction and subcellular localization: (1) Podoconjugate export protein kpsD—this protein is mainly involved in translocation of podoconjugates from the site of synthesis to the cell surface (43). (2) Outer membrane protein assembler bamA—bamA is mainly involved in catalyzing the assembly of bacterial transmembrane proteins and could be a potential vaccine candidate for the prevention of Escherichia coli and Salmonella infections (44, 45). (3) Lipopolysaccharide assembly protein lptD—this protein mediates lipopolysaccharide transport and lptD of Vibrio Parahemolyticus is highly immunogenic, providing 100% protection against Vibrio infection in mice and is a potential vaccine antigen (46). (4) Peptidoglycan-associated lipoprotein Pal—this protein interacts with Tol Pal is a natural TLR2 agonist and binds tightly to LPS, which is released into the bloodstream during infection causing sepsis (47, 48). In addition, Pal from Legionella pneumophila, Haemophilus influenzae, and Campylobacter jejuni was shown to be highly immunogenic and capable of inducing early innate and adaptive immune responses (49–51). (5) Lipoprotein mlaA—this protein, which belongs to the same class of lipoproteins as Pal, is also involved in maintaining the lipid asymmetry of the outer membrane of Gram-negative bacteria, forming an osmotic barrier to prevent the entry of toxic molecules (e.g., antibiotics, disinfectants, etc.). (6) The autotransport assembly complex protein TamA—studies suggest that this protein may be involved as a substrate for secretion to facilitate the secretion of autotransport proteins rather than in an autotransport system for pathogen colonization in the host (52). (7) Urea hydrolase-activating protein nlpD—this protein is also a lipoprotein, and in Cronobacter sakazakii, nlpD responds to acid stress to resist phagocytosis by maintaining membrane integrity. In addition, nlpD may also be involved in the regulation of iron uptake and the activity of the bis-arginine system (53, 54). (8) Pore protein gbp—pore proteins are abundantly present on the surface of bacteria as a sieving barrier and play an important role in host-bacteria interactions, making them potential vaccine candidate antigens and therapeutic targets (55). Then, B cell epitopes (n = 5), CTL epitopes (n = 3), and HTL epitopes (n = 6) were identified from these outer membrane proteins for use in the construction of the vaccine. To improve the stability of the vaccine structure, epitopes were linked together using the EAAAK linker and the GPGPG linker (56, 57). The multi-epitope vaccine was then linked to the phenol-soluble modulin α4 protein (a TLR 2 agonist) selected as an adjuvant using an AYY linker to enhance the immunogenicity of the vaccine (19). The vaccine construct was subsequently tested for antigenicity and allergenicity and was shown to be antigenic and non-allergenic with or without linking to the adjuvant; a higher antigenicity score was predicted for coupling with the adjuvant.
The final vaccine construct containing B-cell epitopes, CTL epitopes, and HTL epitopes as well as linkers and adjuvant is 280 amino acid residues and has a molecular weight of 29.38 kD. The theoretical pI of this vaccine protein was 9.65, indicating the basic nature of the protein. The instability index of the protein is 24.99, whereby a value of <40 indicates that the vaccine construct will be stable whenever expressed (58). In addition, other indicators demonstrated the high thermostability, hydrophilic nature and solubility of the vaccine construct.
The secondary structure prediction of the vaccine constructs showed that only 2% of the amino acid residues were disordered, confirming the stability of the constructs. The construct is mainly composed of coils (73%), which will facilitate antibody production. Moreover, the vaccine construct was modeled and validated, and the interaction of the vaccine construct with TLR2 was investigated using molecular docking simulations to elucidate an effective immune response. The validation of the 3D structure showed that all the residues were in the allowed regions, and over 90% were present in favorable regions, confirming that we obtained a high-quality structural model. Furthermore, the construct had a high binding affinity to TLR2, indicating that it has the potential to stimulate the generation of an immune response. According to the immune simulation results of the C-ImmSim server, a high level of memory B cell formation and antibody production, as well as an increased and prolonged maintenance of cytotoxic and helper T-lymphocytes could be observed after multiple immunizations, thus creating a humoral and cellular immune response that will help prevent infections. In addition, codon optimization was performed after reverse translation of the vaccine protein. The GC and CAI values predicted for the vaccine protein were 54.05% and 1 respectively, indicating that the protein can be expressed in large quantities in E. coli.
The vaccine protein was reverse-translated and codon-optimized, and the E. coli expression vector pET-28a(+)-MEV was constructed and transferred to E. coli BL21 (DE3) for induction of expression, and the target vaccine protein was purified. Polyclonal antibodies obtained from mice immunized with the vaccine protein were able to bind to different serotypes or non-typable HPS isolates, preliminary indicating that the vaccine protein is a promising vaccine candidate. The multi-epitope vaccine designed in this study also has multiple clinical use strategies based on the infection and epidemiological characteristics of HPS—For example, in the direct immunization of piglets against HPS infection. In addition, because the virulent strain of HPS can colonize the respiratory tract of piglets under the protection of maternal antibodies and thus stimulate the piglet organism to produce an immune response to prevent morbidity and mortality, this process is limited to the strain to which the sow has been exposed. Therefore, an alternative immunization strategy that may be more effective is the use of multi-epitope vaccines to immunize reserve or pregnant sows to stimulate the production of antibodies against various serotypes or non-typable HPS, with the piglets being protected by maternal antibodies exhibiting a wide range of reactivities and colonized by different strains of HPS virulence, thus producing an immune response to prevent infection.
Conclusion
The development of a new vaccine is necessary to address the complex epidemiological situation of Haemophilus parasuis and to solve the problems associated with existing vaccines. In this study, we utilized pan-genomic analysis with reverse vaccine technology to construct a vaccine with the potential to prevent infection by all serotypes as well as non-typable of Haemophilus parasuis, and this process that avoids the high cost and time-consuming drawbacks of traditional vaccine development. The vaccine construct had multiple B and T cell epitopes and exhibited high antigenicity, non-toxicity and non-allergenicity. In addition, immune simulation results showed that the vaccine activated high levels of humoral and cellular immune responses. The antibodies obtained from mice immunized with the multi-epitope vaccine were able to bind to different serotyped or untypable HPS isolates. In conclusion, the vaccine designed in this study is a promising candidate for the control of Haemophilus parasuis.
Data availability statement
The original contributions presented in the study are included in the article/Supplementary material, further inquiries can be directed to the corresponding author.
Ethics statement
The animal study was reviewed and approved by the Sichuan Provincial Laboratory Animal Management Committee.
Author contributions
MP and TT: methodology. MP and MR: software. PZ and TT: validation. PZ and YL: data curation. MP: writing-original draft preparation. TT and YW: writing-review and editing. XY and ZY: project administration. All authors have read and agreed to the published version of the manuscript.
Funding
This work was funded by the Sichuan Province Science and Technology Planning Project (Project Number: 2020YJ0345; Title: Molecular Regulation Mechanism of Haemophilus Parasuis Type II Toxin Antitoxin System in Bacterial Biofilm Formation); Sichuan Province Science and Technology Planning Project (Project Number: 2021YFSY0005; Title: Development of Synergist for Swine Disease Vaccine Based on Plant Polysaccharides and Cytokines); Sichuan Province Science and Technology Planning Project (Project Number: 2021YJ0270; Title: Establishment of Detection Method for Identification of African Swine Fever Virus Infection and Its Application in Biosafety Control System).
Acknowledgments
We thank YW for his insightful comments on the design of the study.
Conflict of interest
The authors declare that the research was conducted in the absence of any commercial or financial relationships that could be construed as a potential conflict of interest.
Publisher's note
All claims expressed in this article are solely those of the authors and do not necessarily represent those of their affiliated organizations, or those of the publisher, the editors and the reviewers. Any product that may be evaluated in this article, or claim that may be made by its manufacturer, is not guaranteed or endorsed by the publisher.
Supplementary material
The Supplementary Material for this article can be found online at: https://www.frontiersin.org/articles/10.3389/fvets.2022.1053198/full#supplementary-material
References
1. Brockmeier SL, Register KB, Kuehn JS, Nicholson TL, Loving CL, Bayles DO, et al. Virulence and draft genome sequence overview of multiple strains of the swine pathogen Haemophilus parasuis. PLoS ONE. (2014) 9:e103787. doi: 10.1371/journal.pone.0103787
2. Kielstein P, Rapp-Gabrielson VJ. Designation of 15 serovars of Haemophilus parasuis on the basis of immunodiffusion using heat-stable antigen extracts. J Clin Microbiol. (1992) 30:862–5. doi: 10.1128/jcm.30.4.862-865.1992
3. Blackall PJ, Trott DJ, Rapp-Gabrielson V, Hampson DJ. Analysis of Haemophilus parasuis by multilocus enzyme electrophoresis. Vet Microbiol. (1997) 56:125–34. doi: 10.1016/S0378-1135(96)01342-9
4. Cerdà-Cuéllar M, Naranjo JF, Verge A, Nofrarías M, Cortey M, Olvera A, et al. Sow vaccination modulates the colonization of piglets by Haemophilus parasuis. Vet Microbiol. (2010) 145:315–20. doi: 10.1016/j.vetmic.2010.04.002
5. Olvera A, Cerdà-Cuéllar M, Aragon V. Study of the population structure of Haemophilus parasuis by multilocus sequence typing. Microbiology. (2006) 152:3683–90. doi: 10.1099/mic.0.29254-0
6. Olvera A, Cerdà-Cuéllar M, Nofrarías M, Revilla E, Segalés J, Aragon V. Dynamics of Haemophilus parasuis genotypes in a farm recovered from an outbreak of Glässer's disease. Vet Microbiol. (2007) 123:230–7. doi: 10.1016/j.vetmic.2007.03.004
7. Jalal K, Khan K, Ahmad D, Hayat A, Basharat Z, Abbas MN, et al. Pan-genome reverse vaccinology approach for the design of multi-epitope vaccine construct against Escherichia albertii. Int J Mol Sci. (2021) 22:12814. doi: 10.3390/ijms222312814
8. D'Mello A, Ahearn CP, Murphy TF, Tettelin H. ReVac: a reverse vaccinology computational pipeline for prioritization of prokaryotic protein vaccine candidates. BMC Genomics. (2019) 20:981. doi: 10.1186/s12864-019-6195-y
9. Koren S, Walenz BP, Berlin K, Miller JR, Bergman NH, Phillippy AM. Canu: scalable and accurate long-read assembly via adaptive k-mer weighting and repeat separation. Genome Res. (2017) 27:722–36. doi: 10.1101/gr.215087.116
10. Huang Y, Liu P, Shih P. Homopolish: a method for the removal of systematic errors in nanopore sequencing by homologous polishing. Genome Biol. (2021) 22:95. doi: 10.1186/s13059-021-02282-6
11. Seemann T. Prokka: rapid prokaryotic genome annotation. Bioinformatics. (2014) 30:2068–9. doi: 10.1093/bioinformatics/btu153
12. Page AJ, Cummins CA, Hunt M, Wong VK, Reuter S, Holden MTG, et al. Roary: rapid large-scale prokaryote pan genome analysis. Bioinformatics. (2015) 31:3691–3. doi: 10.1093/bioinformatics/btv421
13. Kumar S, Stecher G, Li M, Knyaz C, Tamura K. MEGA X: molecular evolutionary genetics analysis across computing platforms. Mol Biol Evol. (2018) 35:1547–9. doi: 10.1093/molbev/msy096
14. Almagro Armenteros JJ, Tsirigos KD, Sønderby CK, Petersen TN, Winther O, Brunak S, et al. SignalP 50 improves signal peptide predictions using deep neural networks. Nat Biotechnol. (2019) 37:420–3. doi: 10.1038/s41587-019-0036-z
15. Ong E, Wang H, Wong MU, Seetharaman M, Valdez N, He Y. Vaxign-ML: supervised machine learning reverse vaccinology model for improved prediction of bacterial protective antigens. Bioinformatics. (2020) 36:3185–91. doi: 10.1093/bioinformatics/btaa119
16. Jespersen MC, Peters B, Nielsen M, Marcatili P. BepiPred-2.0: improving sequence-based B-cell epitope prediction using conformational epitopes. Nucleic Acids Res. (2017) 45:W24–9. doi: 10.1093/nar/gkx346
17. Peters B, Bulik S, Tampe R, Van Endert PM, Holzhütter H. Identifying MHC class I epitopes by predicting the TAP transport efficiency of epitope precursors. J Immunol. (2003) 171:1741–9. doi: 10.4049/jimmunol.171.4.1741
18. Nielsen M, Lund O. NN-align: an artificial neural network-based alignment algorithm for MHC class II peptide binding prediction. BMC Bioinformat. (2009) 10:296. doi: 10.1186/1471-2105-10-296
19. Chatterjee R, Sahoo P, Mahapatra SR, Dey J, Ghosh M, Kushwaha GS, et al. Development of a conserved chimeric vaccine for induction of strong immune response against Staphylococcus aureus using immunoinformatics approaches. Vaccines. (2021) 9:1038. doi: 10.3390/vaccines9091038
20. Doytchinova IA, Flower DR. VaxiJen: a server for prediction of protective antigens, tumour antigens and subunit vaccines. BMC Bioinformat. (2007) 8:4. doi: 10.1186/1471-2105-8-4
21. Dimitrov I, Bangov I, Flower DR, Doytchinova I. AllerTOP v.2–a server for in silico prediction of allergens. J Mol Model. (2014) 20:2278. doi: 10.1007/s00894-014-2278-5
22. Magnan CN, Randall A, Baldi P. SOLpro: accurate sequence-based prediction of protein solubility. Bioinformatics. (2009) 25:2200–7. doi: 10.1093/bioinformatics/btp386
23. McGuffin LJ, Bryson K, Jones DT. The PSIPRED protein structure prediction server. Bioinformatics. (2000) 16:404–5. doi: 10.1093/bioinformatics/16.4.404
24. Wang S, Li W, Liu S, Xu J. RaptorX-Property: a web server for protein structure property prediction. Nucleic Acids Res. (2016) 44:W430–5. doi: 10.1093/nar/gkw306
25. Kim DE, Chivian D, Baker D. Protein structure prediction and analysis using the Robetta server. Nucleic Acids Res. (2004) 32:W526–31. doi: 10.1093/nar/gkh468
26. Colovos C, Yeates TO. Verification of protein structures: patterns of nonbonded atomic interactions. Protein Sci. (1993) 2:1511–9. doi: 10.1002/pro.5560020916
27. Laskowski RA, MacArthur MW, Moss DS, Thornton JM. PROCHECK: a program to check the stereochemical quality of protein structures. J Appl Crystallogr. (1993) 26:283–91. doi: 10.1107/S0021889892009944
28. Ponomarenko J, Bui H, Li W, Fusseder N, Bourne PE, Sette A, et al. ElliPro: a new structure-based tool for the prediction of antibody epitopes. BMC Bioinformat. (2008) 9:514. doi: 10.1186/1471-2105-9-514
29. Kozakov D, Hall DR, Xia B, Porter KA, Padhorny D, Yueh C, et al. The ClusPro web server for protein-protein docking. Nat Protoc. (2017) 12:255–78. doi: 10.1038/nprot.2016.169
30. Berman HM, Westbrook J, Feng Z, Gilliland G, Bhat TN, Weissig H, et al. The protein data bank. Nucleic Acids Res. (2000) 28:235–42. doi: 10.1093/nar/28.1.235
31. Rapin N, Lund O, Bernaschi M, Castiglione F. Computational immunology meets bioinformatics: the use of prediction tools for molecular binding in the simulation of the immune system. PLoS ONE. (2010) 5:e9862. doi: 10.1371/journal.pone.0009862
32. Grote A, Hiller K, Scheer M, Münch R, Nörtemann B, Hempel DC, et al. JCat: a novel tool to adapt codon usage of a target gene to its potential expression host. Nucleic Acids Res. (2005) 33:W526–31. doi: 10.1093/nar/gki376
33. Chen FZ, You LJ, Yang F, Wang LN, Guo XQ, Gao F, et al. CNGBdb: China National GeneBank DataBase. Yi chuan Hereditas. (2020) 42:799–809.
34. Guo X, Chen F, Gao F, Li L, Liu K, You L, et al. CNSA: a data repository for archiving omics data. Database. (2020) 2020:baaa055. doi: 10.1093/database/baaa055
35. Majid M, Andleeb S. Designing a multi-epitopic vaccine against the enterotoxigenic Bacteroides fragilis based on immunoinformatics approach. Sci Rep. (2019) 9:19780. doi: 10.1038/s41598-019-55613-w
36. Martín de la Fuente AJ, Rodríguez-Ferri EF, Frandoloso R, Martínez S, Tejerina F, Gutiérrez-Martín CB. Systemic antibody response in colostrum-deprived pigs experimentally infected with Haemophilus parasuis. Res Vet Sci. (2009) 86:248–53. doi: 10.1016/j.rvsc.2008.07.017
37. Takahashi K, Naga S, Yagihashi T, Ikehata T, Nakano Y, Senna K, et al. A cross-protection experiment in pigs vaccinated with Haemophilus parasuis serovars 2 and 5 bacterins, and evaluation of a bivalent vaccine under laboratory and field conditions. J Vet Medical Sci. (2001) 63:487–91. doi: 10.1292/jvms.63.487
38. McOrist S, Bowles R, Blackall P. Autogenous sow vaccination for Glasser's disease in weaner pigs in two large swine farm systems. J Swine Health Prod. (2009) 17:90–6.
39. Costa-Hurtado M, Olvera A, Martinez-Moliner V, Galofré-Milà N, Martínez P, Dominguez J, et al. Changes in macrophage phenotype after infection of pigs with Haemophilus parasuis strains with different levels of virulence. Infect Immun. (2013) 81:2327–33. doi: 10.1128/IAI.00056-13
40. Zhang P, Jiang D, Wang Y, Yao X, Luo Y, Yang Z. Comparison of de novo assembly strategies for bacterial genomes. Int J Mol Sci. (2021) 22:14. doi: 10.3390/ijms22147668
41. Aragon V, Cerdà-Cuéllar M, Fraile L, Mombarg M, Nofrarías M, Olvera A, et al. Correlation between clinico-pathological outcome and typing of Haemophilus parasuis field strains. Vet Microbiol. (2010) 142:387–93. doi: 10.1016/j.vetmic.2009.10.025
42. Dazzi CC, Guizzo JA, Prigol SR, Kreutz LC, Driemeier D, Chaudhuri S, et al. New pathological lesions developed in pigs by a “non-virulent” strain of Glaesserella parasuis. Front Vet Sci. (2020) 7:98. doi: 10.3389/fvets.2020.00098
43. Arrecubieta C, Hammarton TC, Barrett B, Chareonsudjai S, Hodson N, Rainey D, et al. The transport of group 2 capsular polysaccharides across the periplasmic space in Escherichia coli. Roles for the KpsE and KpsD proteins. J Biol Chem. (2001) 276:4245–50. doi: 10.1074/jbc.M008183200
44. Guan Q, Wang X, Wang X, Teng D, Wang J. In silico analysis and recombinant expression of BamA protein as a universal vaccine against Escherichia coli in mice. Appl Microbiol Biot. (2016) 100:5089–98. doi: 10.1007/s00253-016-7467-y
45. Konovalova A, Kahne DE, Silhavy TJ. Outer membrane biogenesis. Annu Rev Microbiol. (2017) 71:539–56. doi: 10.1146/annurev-micro-090816-093754
46. Zha Z, Li C, Li W, Ye Z, Pan J. LptD is a promising vaccine antigen and potential immunotherapeutic target for protection against Vibrio species infection. Sci Rep. (2016) 6:38577. doi: 10.1038/srep38577
47. Godlewska R, Wiśniewska K, Pietras Z, Jagusztyn-Krynicka EK. Peptidoglycan-asso-ciated lipoprotein (Pal) of Gram-negative bacteria: function, structure, role in pathogenesis and potential application in immunoprophylaxis. FEMS Microbiol Lett. (2009) 298:1–11. doi: 10.1111/j.1574-6968.2009.01659.x
48. Liang MD, Bagchi A, Warren HS, Tehan MM, Trigilio JA, Beasley-Topliffe LK, et al. Bacterial peptidoglycan-associated lipoprotein: a naturally occurring toll-like receptor 2 agonist that is shed into serum and has synergy with lipopolysaccharide. J Infect Dis. (2005) 191:939–48. doi: 10.1086/427815
49. Mobarez AM, Rajabi RA, Salmanian AH, Khoramabadi N, Hosseini Doust SR. Induction of protective immunity by recombinant peptidoglycan associated lipoprotein (rPAL) protein of Legionella pneumophila in a BALB/c mouse model. Microb Pathogenesis. (2019) 128:100–5. doi: 10.1016/j.micpath.2018.12.014
50. Kodama S, Hirano T, Suenaga S, Abe N, Suzuki M. Eustachian tube possesses immunological characteristics as a mucosal effector site and responds to P6 outer membrane protein of nontypeable Haemophilus influenzae. Vaccine. (2006) 24:1016–27. doi: 10.1016/j.vaccine.2005.07.110
51. Cordwell SJ, Len ACL, Touma RG, Scott NE, Falconer L, Jones D, et al. Identification of membrane-associated proteins from Campylobacter jejuni strains using complementary proteomics technologies. Proteomics. (2008) 8:122–39. doi: 10.1002/pmic.200700561
52. Selkrig J, Mosbahi K, Webb CT, Belousoff MJ, Perry AJ, Wells TJ, et al. Discovery of an archetypal protein transport system in bacterial outer membranes. Nat Struct Mol Biol. (2012) 19:506–10. doi: 10.1038/nsmb.2261
53. Ji X, Lu P, Xue J, Zhao N, Zhang Y, Dong L, et al. The lipoprotein NlpD in Cronobacter sakazakii responds to acid stress and regulates macrophage resistance and virulence by maintaining membrane integrity. Virulence. (2021) 12:415–29. doi: 10.1080/21505594.2020.1870336
54. Tidhar A, Levy Y, Zauberman A, Vagima Y, Gur D, Aftalion M, et al. Disruption of the NlpD lipoprotein of the plague pathogen Yersinia pestis affects iron acquisition and the activity of the twin-arginine translocation system. Plos Neglect Trop D. (2019) 13:e0007449. doi: 10.1371/journal.pntd.0007449
55. Sharma A, Yadav SP, Sarma D, Mukhopadhaya A. Modulation of host cellular responses by gram-negative bacterial porins. Adv Protein Chem STR. (2022) 128:35–77. doi: 10.1016/bs.apcsb.2021.09.004
56. Chen X, Zaro JL, Shen W. Fusion protein linkers: property, design and functionality. Adv Drug Deliver Rev. (2013) 65:1357–69. doi: 10.1016/j.addr.2012.09.039
57. Arai R, Ueda H, Kitayama A, Kamiya N, Nagamune T. Design of the linkers which effectively separate domains of a bifunctional fusion protein. Protein Eng. (2001) 14:529–32. doi: 10.1093/protein/14.8.529
Keywords: Haemophilus parasuis, reverse vaccinology, pan-genome analysis, multi-epitope vaccine, immunoinformatics
Citation: Pang M, Tu T, Wang Y, Zhang P, Ren M, Yao X, Luo Y and Yang Z (2022) Design of a multi-epitope vaccine against Haemophilus parasuis based on pan-genome and immunoinformatics approaches. Front. Vet. Sci. 9:1053198. doi: 10.3389/fvets.2022.1053198
Received: 25 September 2022; Accepted: 30 November 2022;
Published: 29 December 2022.
Edited by:
Lian-Feng Li, Harbin Veterinary Research Institute (CAAS), ChinaReviewed by:
Yuexiu Zhang, The Ohio State University, United StatesFrancesco Ria, Catholic University of the Sacred Heart, Italy
Copyright © 2022 Pang, Tu, Wang, Zhang, Ren, Yao, Luo and Yang. This is an open-access article distributed under the terms of the Creative Commons Attribution License (CC BY). The use, distribution or reproduction in other forums is permitted, provided the original author(s) and the copyright owner(s) are credited and that the original publication in this journal is cited, in accordance with accepted academic practice. No use, distribution or reproduction is permitted which does not comply with these terms.
*Correspondence: Yin Wang, 10334@sicau.edu.cn
†These authors have contributed equally to this work and share first authorship