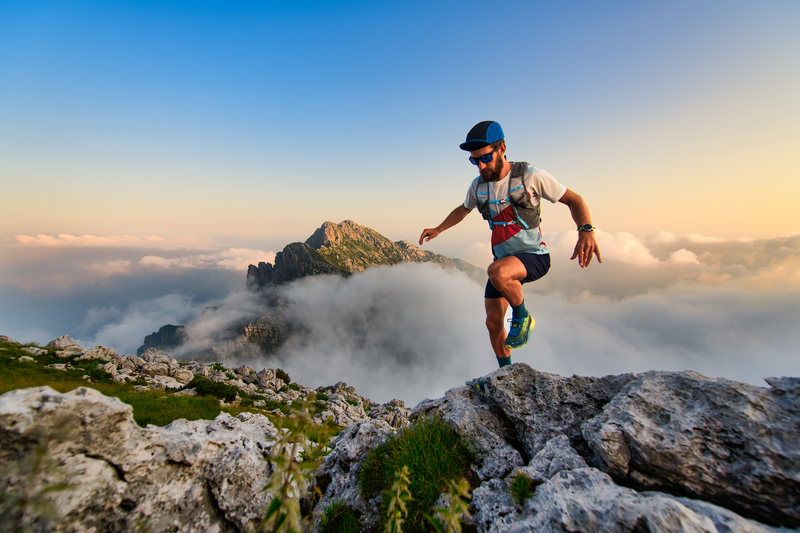
94% of researchers rate our articles as excellent or good
Learn more about the work of our research integrity team to safeguard the quality of each article we publish.
Find out more
ORIGINAL RESEARCH article
Front. Vet. Sci. , 01 December 2022
Sec. Animal Behavior and Welfare
Volume 9 - 2022 | https://doi.org/10.3389/fvets.2022.1027020
Introduction: Sheep have heterogenous social connections that influence transmission of some infectious diseases. Footrot is one of the top five globally important diseases of sheep, it is caused by Dichelobacter nodosus and transmits between sheep when infectious feet contaminate surfaces, e.g., pasture. Surfaces remain infectious for a few minutes to a few days, depending on surface moisture levels. Susceptible sheep in close social contact with infectious sheep might be at risk of becoming infected because they are likely to step onto infectious footprints, particularly dams and lambs, as they cluster together.
Methods: High resolution proximity sensors were deployed on 40 ewes and their 54 lambs aged 5–27 days, in a flock with endemic footrot in Devon, UK for 13 days. Sheep locomotion was scored daily by using a 0–6 integer scale. Sheep were defined lame when their locomotion score (LS) was ≥2, and a case of lameness was defined as LS ≥2 for ≥2 days.
Results: Thirty-two sheep (19 ewes, 9 single, and 4 twin lambs) became lame during the study, while 14 (5 ewes, 5 single, and 4 twin lambs) were lame initially. These 46 sheep were from 29 family groups, 14 families had >1 lame sheep, and transmission from ewes to lambs was bidirectional. At least 15% of new cases of footrot were from within family transmission; the occurrence of lameness was higher in single than twin lambs. At least 4% of transmission was due to close contact across the flock. Most close contact occurred within families. Single and twin lambs spent 1.5 and 0.9 hours/day with their dams, respectively, and twin lambs spent 3.7 hours/day together. Non-family sheep spent only 0.03 hours/day in contact. Lame single lambs and ewes spent less time with non-family sheep, and lame twin lambs spent less time with family sheep.
Discussion: We conclude that most transmission of lameness is not attributable to close contact. However, in ewes with young lambs, some transmission occurs within families and is likely due to time spent in close contact, since single lambs spent more time with their dam than twin lambs and were more likely to become lame.
Footrot is one of the top five globally important diseases of sheep and causes lameness, poor welfare and production losses (1–4). Footrot is caused by Dichelobacter nodosus. Diseased feet are infectious for 3 days to > 10 weeks, depending on the time to recovery (5). Diseased feet deposit D. nodosus on surfaces such as pasture where it is detected in only some samples of soil from areas where sheep spend considerable time, e.g., around feed and water points, and from areas where sheep are only transiently present (5). This distribution of detection in both high and low use areas of a pasture is explained by the nature of D. nodosus—D. nodosus cannot replicate off-host (5, 6) and only survives in the environment for a few minutes to a few days; survival time increases in moist environments and pasture can be persistently contaminated in wet weather (5, 7).
The incidence of footrot increases in flocks of ewes with young lambs. This has been attributed to high stocking densities, moist weather conditions, and susceptible newborn lambs (8). The incidence of footrot also clusters within families [dam and lamb(s)] (9). Within family clusters could be explained by genetic susceptibility to footrot, however, heritable resistance is low (h = 0.1) (10) and it is possible that close proximity explains at least some transmission (indirect via pasture) of footrot within families (11–15). Dams and their lambs spend considerable time together and the majority of physical contacts within family are close and prolonged, e.g., suckling, whilst contact between non-family ewes and lambs tend to be more distant and brief, e.g., sniffing (14).
Since social affinity in sheep is highly correlated with spatial proximity (16), proximity sensors are a suitable tool to assess their social behavior and have been used previously in studies assessing contact patterns in sheep (17–19). Proximity sensors collect high-resolution, continuous data of spatial co-location between two animals, and these types of biologging technologies are particularly useful to investigate transmission of disease because large volumes of data are collected continuously for each animal without the need for continuous observation and without disturbing the animals.
Analysis of data on disease transmission between pairs of animals cannot be carried out using traditional statistical tools because paired data are inherently related and defy the rules of independence, i.e., sheep A and B in contact at time t is the same data as sheep B and A in contact at time t (20). Network-based diffusion analysis (NBDA) can use association probabilities that account for the behavior of both sheep A and sheep B directly, to investigate whether transmission of a disease occurs through a social process (21), by determining if the spread of disease follows the connections in a social network (22). So for footrot, the connection of a lame sheep with a non-lame sheep could increase the risk of transmission of disease.
The aim of our study was to use proximity sensors and NBDA to determine whether family spatial co-location contributes to the spread of footrot between ewes and lambs. We used data from Ozella et al. (15) together with visual observations of lameness from the same flock at the same time.
Ethical approval was granted by the University of Exeter (eCLESPsy000541).
The study took place from 1st−15th October 2019 on a farm with permanent grass pasture in the Blackdown Hills, Devon, United Kingdom. The study population was a commercial flock of 50 pedigree Poll Dorset ewes with 68 lambs, sired by 5 rams. The breeding cycle on the study farm [described fully in (19)] was typical for Poll Dorsets, with mating in mid-April and parturition from September to mid-October. Poll Dorset ewes are sexually active for most of the year and produce lambs from August–May in the Northern Hemisphere.
Each ewe was identified with a unique number with livestock marker paint, and her lambs were marked with that same number, with the larger twin in a litter differentiated by a paint dot on the head. On day 0 (October 1st), all sheep were moved onto a field which had not been grazed by sheep for at least 4 weeks to ensure that the pasture was free from D. nodosus and so not a source of infection of D. nodosus (5–7). The field was surrounded by large hedgerows on all sides, with the water trough positioned at one side, and was managed by strip grazing using an electric fence. Initially the flock had access to 0.69 hectares (ha) of pasture, this was increased to 1.34 ha after 4 days, then to 1.98 ha after a further 4 days. Meteorological data were collected daily using a Davis Vantage Pro2 Plus weather station (Supplementary Table 1) and summarized into two climatic indexes—the mean daily temperature-humidity index (THI, °C), which combines temperature and humidity (23) and the mean daily wind-chill index (WCI, °C) which combines wind speed and temperature (24).
The proximity sensing platform was designed by the SocioPatterns collaboration consortium (http://www.sociopatterns.org/). The sensors have a bidirectional radio interface and transmit packets carrying a unique identifier as a data payload, which is received by nearby sensors. The exchange of packets is used to measure spatial proximity by attenuation, i.e., the difference between the received and transmitted power (25). An attenuation threshold of −75 dBm was used to detect sheep co-located within 1–1.5 m. Information on previous calibration and validation of the sensing system are in Fielding et al. (26). Based on the calibration and validation work of Fielding et al. (26) and a number of studies with sheep (15, 19), we have confidence that an attenuation threshold of −75 dBm characterizes proximity between on-sheep sensors of within 1–1.5 m (approximately the body length of an adult sheep). Because the maximum distance detected between two sensors is 5 m (26), we can state that the above mentioned attenuation threshold characterizes proximity never exceeding 5 m. A co-location occurred when at least one radio packet was exchanged which exceeded the attenuation threshold. The duration of a co-location was the number of consecutive 20 s time intervals in which radio packets were exchanged.
The proximity sensors used in this study have been used in other social network studies on animals (15, 19, 26, 27). The sensors weigh ~6 g (sensor ~ 2.7 g, lithium coin battery ~ 3 g), and have a memory of ~1,000 h of contact events and a battery life of ~25 days. Sensors were put on the ewes on freely rotating neck collars and on lambs on adjustable body harnesses that were checked daily and enlarged as they grew. The total weight of the equipment was ~100 g, < 5% of an animal's body weight as recommended (28, 29).
Proximity sensors were fitted to all 50 ewes and 65/68 lambs; three were considered too small to wear the device. Not all devices collected data for the whole study. We excluded whole family groups if one family member was not tagged, or experienced device issues [see (15)], leaving 40 ewes and 54 lambs with complete data.
Most sheep with footrot are lame (30), consequently lameness was used as a proxy for footrot and it was assumed that only lame sheep were infectious. Ewes and lambs had been acclimatized to locomotion scoring by KL walking through the flock and scoring locomotion each week throughout September 2019. For the study period (1st−15th October), the locomotion of all ewes and lambs was assessed once each day by KL using a validated locomotion scoring system (31) (Supplementary Table 2). Scores were recorded on paper and entered manually into Microsoft Excel (32). An episode of lameness was defined as a sheep with a locomotion score ≥2 on ≥2 days. The daily and cumulative prevalence of lameness in ewes, single and twin lambs were calculated.
Interdigital dermatitis (ID), a presentation of footrot (33) was observed in sheep during the study. Nine ewes and 10 lambs, identified by the farmer, were treated by spraying the interdigital skin of all four feet with topical antibiotic. No other infectious causes of lameness were observed. The feet of all ewes and lambs were inspected on the last day of the study and footrot lesions were scored (34) (Supplementary Table 3) by EN, who was blind to the locomotion scores of the sheep. Other foot lesions (white line disease, fibroma, and granuloma) were identified and recorded using classical definitions (35).
All analysis was done using RStudio v4.0.3. The social network was visualized using igraph (36) and ggnetwork (37). Sheep have clear and consistent diurnal patterns with periods of inactivity during the night (38) but sleep in short bouts (39) therefore the start of each 24-h period can be chosen arbitrarily and was selected as midnight for the study because that provided 13, 24-h periods. The day of deployment and removal of sensors was not included in the analysis of data. The time sheep spent with other sheep per day was calculated by summing the 20-s contact periods over a 24-h period for within family, and out of family contacts.
The probability of association between pairs of sheep was measured using dyadic association indexes to estimate the proportion of time pairs spent in close contact. The index was calculated as in Ozella et al. (15) with the formula:
where the association index (AI) equals the number of 20-s sampling periods x, with individuals a and b in contact, divided by the number of sampling periods where individuals a and b were in contact, plus the number of sampling periods where a was detected without b, and the number of sampling periods where b was detected without a. The index ranges from 0 (two individuals were never observed together) to 1 (two individuals were always observed together) consequently, the higher the value of the index, the greater the contact between a pair of sheep.
Multi-network NBDA was carried out using the NBDA R package (40) to quantify the relative importance of different social networks in transmission of D. nodosus. Within a multi-network NBDA, social networks are used as predictor variables which represent hypotheses about possible transmission pathways of a trait. In our analysis social transmission of footrot occurred if a sheep became lame following contact with a lame sheep, and asocial transmission of footrot occurred if a sheep became lame without contact with a lame sheep. The Akaike weight was used to rank models, using the probability that the model was the best Kullback-Leibler model in the set, given the dataset and candidate models (41).
The basic NBDA model (40) is fitted by maximum likelihood and is expressed as:
where λi(t) is the rate at which individual i acquires the disease as a function of time t, λo(t) is the baseline rate of disease acquisition, zi(t) is the “status” of individual i at time t (1 = diseased; 0 = naive), N is the number of individuals in the population and aij is a non-negative value indicating the connection strength from j to i: connection strength takes values from 0 = never associated to 1 = always associated in a social network, s is the key output parameter, it is the relative strength of social transmission of lameness to asocial transmission of lameness.
Multinetwork NBDA (42) expands this to:
where an, ij(t) is the connection strength from j to i in network n at time t (day of the study), and sn is the transmission rate per unit connection in network n relative to the rate of asocial transmission, and z is the status (0 or 1) of the individual. Bi is the sum of the coefficients of the effect of variables 1:k on asocial transmission multiplied by the value for individual i, and Γi is the sum of the coefficients of the effects of variables 1:k on social transmission multiplied by the value of variable k for individual i.
A summary of all candidate models tested are in Table 1. We detail below which predictors were used and why.
Table 1. Reference table for each model with the combination of individual-level variables and baseline rates of acquisition of lameness.
There were four hypotheses on transmission pathways of D. nodosus through social networks in the flock tested, these were:
Hypothesis 1: there is an increased risk of transmission of footrot within families. To test this the connection strength between sheep i and j was set to 1 if there was a family relationship, and 0 for a non-family relationship. This hypothesis did not investigate spatial proximity, rather family networks.
Hypothesis 2: the time sheep are in close proximity to each other increases the risk of transmission of footrot. To test this, the connection strength between sheep i and j was set to their association probability from the dyadic association network over the whole study period.
Hypothesis 3: there is transmission of footrot only outside families. To test this, the model was set up as for hypothesis 2, but with connection strength set to 0 for family members, excluding contacts between sheep in the same family.
Hypothesis 4: all sheep have equal risk of becoming lame; footrot is not spread by close contact between sheep. To test this, the connection strength between all pairs of sheep was set to 1. If this homogenous network is favored over the network hypotheses 1–3, it would imply that all sheep have an equal risk of becoming lame.
Visualizations of the connection strength for each of these four hypotheses for example family groups are shown in Supplementary Figure 1.
Models were also run without inclusion of a social network predictor in order to determine if inclusion of a social network (hypothesis 1–4) improved model fit compared to use of individual-level predictors alone. Since it is plausible that the likelihood of whether a sheep becomes lame as a result of social or asocial transmission could be affected by whether the sheep is a ewe, single or twin, individual-level predictors (dummy coded predictors for whether a sheep was a lamb or not, or a twin or not) were investigated to see if they affected the baseline rate of acquisition or the social rate of acquisition of lameness, or both, as well as models without individual-level predictors. The exponential of the coefficient for an individual-level predictor gives a rate, where <1 is a decrease, and >1 is an increase.
In addition, three assumptions on the baseline rate of acquisition of lameness were tested (43), these were:
• (A) Constant: the rate of acquisition of lameness remained constant over time
• (B) Gamma distribution: a systematic increase or decrease in the rate of asocial acquisition of lameness over time
• (C) Weibull distribution: a varying rate of acquisition of lameness with an additional shape parameter κ, when x >1 increasing baseline rate, a constant baseline where κ =1 and a decreasing baseline where κ < 1.
Multi-model inferencing across the model sets for NBDA was used to calculate the median estimates of the parameters to provide robust inference about the strength of transmission through the different networks and the effects of predictor variables (40, 41). A lower limit calculation for the s parameter was performed for relevant models, using the profile likelihood function to search between 0 and the maximum likelihood estimate for s in each model. This is because NBDA can have more certainty about the lower limit of s than the upper limit because the profile likelihood is highly asymmetrical.
The proportion of cases solved by social transmission corresponding to the lower limit of s were calculated as in Hoppitt et al. (40):
Where i is the sheep that became lame during event e (the case of lameness), and te is the day of the study at which event e occurred. This is the predicted relative social transmission rate divided by the predicted total relative transmission rate for i at the time of transmission. The mean of psocial, e across all events is the estimated proportion of events that occurred by social transmission. All other parameters are as defined in Equations (2) and (3).
The time per day each sheep spent with family and non-family sheep was used as the outcome variable in linear mixed effects models to investigate the relationship between time spent in contact with sheep and potential drivers of social contact using lme4 (44). Six models were run with ewes, single and twin lambs investigated separately because their contact patterns varied [Supplementary Table 4 and Ozella et al. (15)]. Random effects were included for day and sheep. Fixed effects were ewe age (years), lamb age (days) and lamb sex, and measured factors that could affect sheep behavior: whether a sheep was lame, whether sheep were gathered, pasture size, mean daily THI (°C) and mean daily WCI (°C).
The models took the form:
where y was the continuous outcome variable time per day with family, or time with non-family, β0 was the intercept, βxj were the explanatory variables that varied by sheep and βxxij were the explanatory variables that varied by day. Residual variance estimates were included at sheep (uij) and day (ei).
Multi-model inferencing (41) using rank by Akaike's Corrected Information Criterion (AICc) was used to account for model selection uncertainty and to calculate model-averaged coefficients and confidence intervals for fixed effects. Variable importance was calculated as the sum of the Akaike weights over all models that included the variable.
A total of 46 sheep from 29 families [dam and lamb(s)] were lame during the study; 5 ewes, 5 single, and 4 twin lambs were lame at the start of the study and a further 19 ewes, 9 single, and 4 twin lambs became lame (Table 2), giving a total of 24 lame ewes, 14 singles, and 8 twins. In 14 families with >1 lame sheep, transmission of lameness between ewes and lambs was bidirectional. Individual daily locomotion scores are in Supplementary Figures 2, 3.
Table 2. Descriptive characteristics for the 40 ewes and their 54 lambs with 13 days of midnight-midnight contact data and complete locomotion records.
Lameness was primarily caused by interdigital dermatitis (ID) (Table 2, Supplementary Table 5). On day 14 ID lesions were observed on 12 (30%) ewes, 9 (34.6%) single lambs, and 11 (39.3%) twin lambs, some lesions were very mild and not all sheep with ID were lame (Supplementary Table 5).
All sheep contacted almost all other sheep at some point in the study period (Figure 1). There were 216,054 contacts over the 13 days, there were 1,338–3,754 contact pairs per day, with 4,358 unique pairings over the 13 days, out of a possible 4,371. Overall, sheep spent an average of 5.27 hours/day [standard deviation (sd) = 3.26] with other sheep (Table 3). Twin lambs spent most time with other sheep (mean 8.55 hours/day), followed by single lambs (mean 5.43 hours/day), while ewes spent less time with other sheep (mean 2.87 hours/day): 64.2% of all contact time was within families. Single lambs spent 1.50 hours/day (sd = 1.05) with their dam, while twins spent 0.92 hours/day (sd = 0.78) with their dam and 3.69 hours/day (sd = 2.31) with each other (Table 3). The dyadic association indexes (AI) over the whole study period were strongest between twins (mean AI = 0.282), followed by single lambs and their dams (mean AI = 0.233), with weaker bonds between twin lambs and their dams (mean AI = 0.088) (Supplementary Table 4). The association between non-family sheep was extremely low (mean AI = 0.003), for single and twin lambs and 0.002 for ewes (Supplementary Table 4), and on average, sheep spent 0.03 hours/day with each non-family sheep.
Figure 1. Visualization of the network over the entire study period with 94 nodes (sheep) and 4,358 edges (contacts). Ewe and lamb family groups have the same number, letter A denotes a lamb and B a twin to lamb A.
Table 3. Descriptive statistics of hours/day over 13 days that sheep spent with other sheep: overall, within family and out of family for ewes, single and twin lambs.
Hypothesis 1 (lameness is transmitted within families) was the most favored transmission pathway (Figure 2) and models where the family network was the social network predictor had ∑ Akaike Weightoverall = 0.78 (Table 4). Within the family network models, lambs became lame at 0.59 times the rate of ewes, and twin lambs became lame at a much slower rate, 2.70 × 10−9 times, than single lambs and ewes (Table 5). Models fitted best when the sheep predictor (whether a sheep was a ewe or lamb, and a twin or single) affected the rate of asocial transmission of lameness only (Table 4), which indicated ewes and lambs had different baseline rates for acquisition of asocially derived lameness. When this is accounted for (models 3, 19 and 35), at least 15% of cases were attributed to social transmission (Table 4).
Figure 2. Akaike Weight for models with different social network predictors and combinations of variables affected social and asocial transmission. Models are ordered by Akaike Weight and are shown if the Akaike Weight was >0.00. 1. Full descriptions of the models are in Table 1 and the full set of Akaike Weights are in Table 4. Akaike Weight is the probability the model is the best Kullback-Leibler model in the set.
Table 4. Lower confidence interval for the s parameter, the corresponding proportion of cases estimated to be by social transmission, and Akaike Weights for each model, ranked by overall Akaike Weight.
Table 5. Median model-averaged terms from the multi-network network-based diffusion analysis, for all social networks combined, and individually for the kinship network of family groups, spatial proximity network of all sheep, spatial proximity of non-family sheep, and the homogenous network.
Hypothesis 2 (lameness is transmitted through spatial co-location with any sheep) received some support with ∑ Akaike Weightoverall = 0.15 (Table 4, Figure 2). As in the family network, models fitted best when ewes and lambs had different baseline rates for acquisition of lameness (models 7, 23 and 39), and when this was accounted for, at least 4% of cases came from social transmission across the flock. The 4% estimate for the role of contact in disease transmission from the proximity sensors is different from the 15% estimate for the family network because these estimates are from two different network assumptions: family and close proximity estimated from the sensors.
Hypothesis 3 (lameness was transmitted through spatial co-location with non-family sheep) and hypothesis 4 (all sheep had equal risk of acquisition of lameness) received little support (Table 4, Figure 2), indicating it was unlikely that lameness was transmitted via spatial co-location with non-family sheep, or that all sheep had equal risk of acquisition of lameness. Models without a social network predictor (models 16, 32, and 48) all received little support (Table 4, Figure 2).
A constant baseline asocial rate of acquisition of lameness had most support (Table 4, Supplementary Table 6), indicating that the risk of acquisition of disease from the environment remained constant over the study period.
The two outcome variables used in the six linear mixed effect models for ewes, single and twin lambs were time per day in contact with family and time per day in contact with non-family sheep (Tables 6, 7). Lame single lambs and ewes spent less time in contact with non-family sheep than non-lame counterparts, a reduction of 0.70 (95% CI = 0.26–1.15) hours/day for single lambs and 0.20 (95% CI = 0.10–0.29) hours/day for ewes. Within family contact time did not change in single lambs and ewes but lame twins spent 0.49 (95% CI = 0.02–1.33) hours/day less with their family than non-lame twin lambs (Table 6).
Table 6. Linear mixed effects models for factors associated with daily time ewes and lambs spent with their family and other sheep.
Table 7. Variable importance for variables in Table 6: factors associated with time ewes and lambs spent with their family and other sheep.
Ewes spent 0.11 (95% CI = 0.01–0.21) fewer hours/day with non-family sheep for each year of age; ewe age was not associated with time spent with their lambs. Twin lambs spent 0.22 (95% CI = 0.11–0.34) hours/day less with their family for each day of life (Table 6).
As pasture size increased ewes spent less time with family and non-family sheep (Table 6), and twin lambs spent less time with non-family sheep (Table 6), but there was no effect of pasture size on the time single lambs spent with non-family sheep. As the THI increased, twin lambs spent 0.17 (95% CI = 0.02–0.32) hours/day less with their family (Table 6).
This is the first study to investigate the role of social contact in the transmission of footrot in sheep. We used high-resolution contact data combined with phenotypic observations to investigate the role of the family network and spatial co-location networks in the acquisition of footrot. We demonstrate that social behavior within families is associated with acquisition of a proportion cases of lameness but that most transmission of footrot is asocial, that is, not associated with infection from specific sheep.
Asocial transmission of footrot has been reported previously in lambs born on straw bedding in pens with groups of ewes. Lambs were negative for D. nodosus at birth but within a few hours they had strains of D. nodosus on their interdigital skin not entirely consistent with those of their dam, indicating that even their first exposure to D. nodosus came from floor surfaces contaminated by several ewes rather than just their dam (45). Models fitted best when the underlying risk of acquisition of lameness from asocial transmission was constant over the 13 days (Table 4, Supplementary Table 6), this is consistent with pasture persistently contaminated with D. nodosus. Despite this, sheep did not have a homogenous risk of acquisition of lameness from the environment (Table 4). This is probably because pasture is not homogenously infectious, all areas of a field, whether frequently used by sheep or not, are heterogeneously contaminated with D. nodosus (5) and so it is “chance” whether a sheep “steps” into a contaminated area of the field. Further heterogeneity in acquisition of lameness might have come from sheep clustering by hedges for considerable time during wet weather (17, 19, 46, 47) and because sheep social behavior changed when they were given access to more (uncontaminated) pasture twice during the study (15). These managements are specific to this flock, but all flocks will have management actions that alter their behavior and might alter the risk of disease transmission.
Sheep with footrot contaminate the ground as they stand and walk and we hypothesize that sheep which spend considerable time spatially co-located with lame sheep are more likely to contact that contaminated ground and become diseased. Models using the family network (hypothesis 1) received more support than hypothesis 2 which considered spatial co-location between sheep (Table 4, Figure 2). It might be that it is the nature of very close contact only made within families, e.g., suckling, that brings sheep into sufficiently close contact for sufficient time for sheep to acquire infection from ground contaminated by a family member. Diseases with low infectiousness are particularly sensitive to the definition of a contact (48, 49) and the definition of contact we investigated (1–1.5 m and 20 s packets of time) in hypothesis 2, probably includes contacts that were never going to lead to transmission of footrot and so underestimated the role of close contact.
Even within families the total contact time influenced acquisition of lameness. Single lambs were more likely to become lame than twin lambs; they spent more time with their dam, possibly because they suckle for longer per day than twin lambs (50) and did not reduce contact time with their family with increasing age as did twin lambs, as in this study (Table 6) and Morgan and Arnold (13). Family networks might also be a risk for transmission of lameness because lame sheep changed their behavior and spent less time with non-family members (Table 6) which would contribute to more within family, social, spread of footrot.
Risks from family networks could also be explained by other factors such as genetic susceptibility to footrot and all sheep in the current study were related to greater or lesser extent. Heritability to footrot is low (0.1) (10) and it is unlikely that heritability explains all the transmission of footrot within families. Families also group together and it might be hypothesized that certain families spent more time in “high risk” areas of the field, however, as Clifton et al. (5) report, D. nodosus is found across pasture—there are no “high risk” areas in fields. Overall, we conclude that it is the time that families spend together that increases the risk of transmission of footrot.
The risk of transmission of D. nodosus within families was bidirectional, sometimes ewes were lame before their lambs, and vice versa, which was also reported in another longitudinal study (30). In both that study and the current study, lambs were not observed from birth and so social behavior and the first occurrence of footrot might have been missed, since the incubation period for footrot is 7–14 days (51, 52). One might hypothesize that since lambs are born without D. nodosus infection (45), the first lamb in a flock infected must have been infected from the ewes, but after that one lamb is infected, infection is bi-directional between ewes and lambs. A longer study observing lambs from birth would be needed to investigate the time to first infection and how changes in lamb behavior with age change the risk of routes of transmission.
Whilst a small proportion of all transmission was social, the finding is important because disease control depends on understanding all routes of transmission. In a flock where ewes and lambs are penned separately all transmission would be within family (52). However, it is useful to know that even in a field where ewes and lambs mix freely some spread of disease arises within families. Isolation of lame sheep reduces onward spread of disease so from our study we can conclude that separation of whole families once one is lame with footrot could protect the rest of the flock since it is likely that another family member is incubating disease. There was little evidence of social transmission of footrot outside families (Table 4). Our study suggests that short contact times and many combinations of pairs of contacts between non-family sheep are not sufficient for transmission. In addition, lame sheep spent less time with non-family sheep than non-lame sheep (Table 6), which could also be why lameness was not transmitted as a result of social contact with non-family sheep.
A limitation of the study is that we used lameness to define infectiousness and so sheep with mild ID that were not lame would have been categorized as non-infectious: we do not know if these sheep are infectious, but they have a lower load of D. nodosus than diseased sheep (33). There were sheep with ID at the end of our study that were not lame (Supplementary Figures 1, 2, Supplementary Table 5). If those sheep were infectious then they are missing from the order of acquisition, which would reduce the power of NBDA to detect a social effect (53). However, it would have perturbed sheep behavior and so affected our hypotheses to examine sheep daily for signs of mild lesions. In addition, sheep would have been incubating footrot, but not lame, at the start of the study, and so the origin of their infection was not known and would have been “asocial” in our analyses. We removed families from the analysis if one member of the family had incomplete data to avoid inflated probabilities of association with non-family sheep. However, data on as few as 30% of individuals in a social network can give an accurate representation of their position in the network (54).
Our study illustrates that high-resolution contact data together with phenotypic observation provides insights into the role of social networks in transmission of D. nodosus within a sheep flock. While most transmission of D. nodosus occurs asocially in a flock of ewes with young lambs, at least 15% of cases occur from within family transmission. We conclude that this is because sheep spend more time with their family than with non-family sheep, and because lame sheep reduce the time they spend with non-family sheep. Further evidence that duration of close contact is important is that single lambs were more likely to become lame than twin lambs, and spent more time with their dam than did twin lambs.
The raw data supporting the conclusions of this article will be made available by the authors, without undue reservation.
The animal study was reviewed and approved by University of Exeter-eCLESPsy000541.
KL analyzed the data and wrote the manuscript draft. EP, JL, and KL collected the farm data. LO and CC processed the proximity sensor data. LG, DC, and JL conceived the study design. All authors contributed to the article, reviewed, and approved the submitted version.
KL was supported by a BBSRC MIBTP iCase Studentship (training grant number BB/M01116X/1) with the Agriculture and Horticulture Development Board. EP was supported by a BBSRC Studentship (training grant number BB/M009122/1).
Thanks go to Graham and Anne Langford of the Blackdown Flock and the Centurian Group of Poll Dorset Breeders and Sam Boon of AHDB Signet. Thanks also go to Elizabeth Nabb (EN) and Naomi Prosser for their help with collection of the foot lesion data.
Author JL is a director of ActivInsights Ltd.
The remaining authors declare that the research was conducted in the absence of any commercial or financial relationships that could be construed as a potential conflict of interest.
All claims expressed in this article are solely those of the authors and do not necessarily represent those of their affiliated organizations, or those of the publisher, the editors and the reviewers. Any product that may be evaluated in this article, or claim that may be made by its manufacturer, is not guaranteed or endorsed by the publisher.
The Supplementary Material for this article can be found online at: https://www.frontiersin.org/articles/10.3389/fvets.2022.1027020/full#supplementary-material
1. Nieuwhof GJ, Bishop SC. Costs of the major endemic diseases of sheep in Great Britain and the potential benefits of reduction in disease impact. Anim Sci. (2005) 81:23–9. doi: 10.1079/ASC41010023
2. Rather M, Wani S, Hussain I, Bhat M, Kabli Z, Magray S. Determination of prevalence and economic impact of ovine footrot in central Kashmir India with isolation and molecular characterization of Dichelobacter nodosus. Anaerobe. (2011) 17:73–7. doi: 10.1016/j.anaerobe.2011.02.003
3. Wassink GJ, King EM, Grogono-Thomas R, Brown JC, Moore LJ, Green LE. A within farm clinical trial to compare two treatments (parenteral antibacterials and hoof trimming) for sheep lame with footrot. Prev Vet Med. (2010) 96:93–103. doi: 10.1016/j.prevetmed.2010.05.006
4. Hickford J, Davies S, Zhou H, Gudex B. A survey of the control and financial impact of footrot in the New Zealand Merino industry. In: Proceedings-New Zealand Society of Animal Production, 2005. Christchurch: New Zealand Society of Animal Production (1999). p. 117.
5. Clifton R, Giebel K, Liu N, Purdy KJ, Green LE. Sites of persistence of Fusobacterium necrophorum and Dichelobacter nodosus: a paradigm shift in understanding the epidemiology of footrot in sheep. Sci Rep. (2019) 9:14429. doi: 10.1038/s41598-019-50822-9
6. Beveridge WIB. Foot-rot in sheep: a transmissible disease due to infection with Fusiformis nodosus (n. sp) studies on its cause, epidemiology, and control. CSIRO Austral Bull. (1941) 140:1–56.
7. Whittington R. Observations on the indirect transmission of virulent ovine footrot in sheep yards and its spread in sheep on unimproved pasture. Aust Vet J. (1995) 72:132–4. doi: 10.1111/j.1751-0813.1995.tb15032.x
8. Russell VNL, Green LE, Bishop SC, Medley GF. The interaction of host genetics and disease processes in chronic livestock disease: a simulation model of ovine footrot. Prev Vet Med. (2013) 108:294–303. doi: 10.1016/j.prevetmed.2012.11.006
9. Kaler J, Medley GF, Grogono-Thomas R, Wellington EMH, Calvo-Bado LA, Wassink GJ, et al. Factors associated with changes of state of foot conformation and lameness in a flock of sheep. Prev Vet Med. (2010) 97:237–44. doi: 10.1016/j.prevetmed.2010.09.019
10. Skerman TM, Johnson D, Kane DW, Clarke JN. Clinical footscald and footrot in a New Zealand Romney flock: phenotypic and genetic parameters. Aust J Agric Res. (1988) 39:907–16. doi: 10.1071/AR9880907
11. Galeana L, Orihuela A, Aguirre V, Vázquez R. Mother-young spatial association and its relation with proximity to a fence separating ewes and lambs during enforced weaning in hair sheep (Ovis aries). Appl Anim Behav Sci. (2007) 108:81–8. doi: 10.1016/j.applanim.2006.10.016
12. Hinch G, Lecrivain E, Lynch J, Elwin R. Changes in maternal-young associations with increasing age of lambs. Appl Anim Behav Sci. (1987) 17:305–18. doi: 10.1016/0168-1591(87)90154-7
13. Morgan P, Arnold G. Behavioural relationships between Merino ewes and lambs during the four weeks after birth. Anim Sci. (1974) 19:169–76. doi: 10.1017/S000335610002273X
14. Norton E, Benaben S, Mbotha D, Schley D. Seasonal variations in physical contact amongst domestic sheep and the implications for disease transmission. Livest Sci. (2012) 145:34–43. doi: 10.1016/j.livsci.2011.12.017
15. Ozella L, Price E, Langford J, Lewis KE, Cattuto C, Croft DP. Association networks and social temporal dynamics in ewes and lambs. Appl Anim Behav Sci. (2022) 246:105515. doi: 10.1016/j.applanim.2021.105515
16. Le Pendu Y, Maublanc M-L, Briedermann L, Dubois M. Spatial structure and activity in groups of Mediterranean mouflon (Ovis gmelini): a comparative study. Appl Anim Behav Sci. (1996) 46:201–16. doi: 10.1016/0168-1591(95)00660-5
17. Doyle RE, Broster JC, Barnes K, Browne WJ. Temperament, age and weather predict social interaction in the sheep flock. Behav Processes. (2016) 131:53–8. doi: 10.1016/j.beproc.2016.08.004
18. Paganoni B, Macleay C, Van Burgel A, Thompson A. Proximity sensors fitted to ewes and rams during joining can indicate the birth date of lambs. Comput Electron Agric. (2020) 170:105249. doi: 10.1016/j.compag.2020.105249
19. Ozella L, Langford J, Gauvin L, Price E, Cattuto C, Croft DP. The effect of age, environment and management on social contact patterns in sheep. Appl Anim Behav Sci. (2020) 225:104964. doi: 10.1016/j.applanim.2020.104964
20. Sosa S, Sueur C, Puga-Gonzalez I. Network measures in animal social network analysis: their strengths, limits, interpretations and uses. Method Ecol Evolut. (2020) 12:10–21. doi: 10.1111/2041-210X.13366
21. Silk MJ, Croft DP, Delahay RJ, Hodgson DJ, Weber N, Boots M, et al. The application of statistical network models in disease research. Methods Ecol Evolut. (2017) 8:1026–41. doi: 10.1111/2041-210X.12770
22. Hoppitt W, Laland KN. Detecting social learning using networks: a users guide. Am J Primatol. (2011) 73:834–44. doi: 10.1002/ajp.20920
24. Tucker CB, Rogers AR, Verkerk GA, Kendall PE, Webster JR, Matthews LR. Effects of shelter and body condition on the behaviour and physiology of dairy cattle in winter. Appl Anim Behav Sci. (2007) 105:1–13. doi: 10.1016/j.applanim.2006.06.009
25. Cattuto C, Van Den Broeck W, Barrat A, Colizza V, Pinton J-F, Vespignani A. Dynamics of person-to-person interactions from distributed RFID sensor networks. PLoS ONE. (2010) 5:e11596. doi: 10.1371/journal.pone.0011596
26. Fielding HR, Silk MJ, Mckinley TJ, Delahay RJ, Wilson-Aggarwal JK, Gauvin L, et al. Spatial and temporal variation in proximity networks of commercial dairy cattle in Great Britain. Prev Vet Med. (2021) 194:105443. doi: 10.1016/j.prevetmed.2021.105443
27. Wilson-Aggarwal JK, Ozella L, Tizzoni M, Cattuto C, Swan GJ, Moundai T, et al. High-resolution contact networks of free-ranging domestic dogs Canis familiaris and implications for transmission of infection. PLoS Negl Trop Dis. (2019) 13:e0007565. doi: 10.1371/journal.pntd.0007565
28. Sikes RS, Care A, Mammalogists UCOTASO. 2016 Guidelines of the American Society of Mammalogists for the use of wild mammals in research and education. J Mammal. (2016) 97:663–88. doi: 10.1093/jmammal/gyw078
29. Wilson DE, Cole RF, Nichols JD, Foster MS. Measuring and Monitoring Biological Diversity Standard Methods for Mammals. Washington, DC: Sminthsonian Books (1996).
30. Kaler J, George TR, Green LE. Why are sheep lame? Temporal associations between severity of foot lesions and severity of lameness in 60 sheep. Anim Welfare. (2011) 20:433–8.
31. Kaler J, Wassink GJ, Green LE. The inter- and intra-observer reliability of a locomotion scoring scale for sheep. Vet J. (2009) 180:189–94. doi: 10.1016/j.tvjl.2007.12.028
33. Witcomb LA, Green LE, Kaler J, Ul-Hassan A, Calvo-Bado LA, Medley GF, et al. A longitudinal study of the role of Dichelobacter nodosus and Fusobacterium necrophorum load in initiation and severity of footrot in sheep. Prev Vet Med. (2014) 115:48–55. doi: 10.1016/j.prevetmed.2014.03.004
34. Moore LJ, Wassink GJ, Green LE, Grogono-Thomas R. The detection and characterisation of Dichelobacter nodosus from cases of ovine footrot in England and Wales. Vet Microbiol. (2005) 108:57–67. doi: 10.1016/j.vetmic.2005.01.029
36. Csardi G, Nepusz T. The igraph software package for complex network research. InterJ Complex Syst. (2006) 1695:1–9.
37. Briatte F. ggnetwork: Geometries to Plot Networks with ‘ggplot2'. R package version 0.5.10 (2021).
38. Sarout BNM, Waterhouse A, Duthie C-A, Poli CHEC, Haskell MJ, Berger A, et al. Assessment of circadian rhythm of activity combined with random regression model as a novel approach to monitoring sheep in an extensive system. Appl Anim Behav Sci. (2018) 207:26–38. doi: 10.1016/j.applanim.2018.06.007
39. Munro J. Sleep in sheep. In: Proceedings of the British Society of Animal Production. (1957). p. 71–5. doi: 10.1017/S0369852100001810
40. Hoppitt W, Photopoulou T, Hasenjager MJ, Leadbeater E. NBDA: A Package For Implementing Network-Based Diffusion Analysis. R package version 0.9.6 (2020). Available online at: https://github.com/whoppitt/NBDA
41. Burnham KP, Anderson DR. A practical information-theoretic approach. Model select Multimodel Inference. (2002) 2:149–205.
42. Farine DR, Aplin LM, Sheldon BC, Hoppitt W. Interspecific social networks promote information transmission in wild songbirds. Proc R Soc B Biol Sci. (2015) 282:20142804. doi: 10.1098/rspb.2014.2804
43. Hoppitt W, Laland K. Social Learning: An Introduction to Mechanisms, Methods and Models. Princeton, NJ; Oxford: Princeton University Press (2013).
44. Bates D, Maechler M, Bolker B, Walker S. Fitting linear mixed-effects models using lme4. J Stat Softw. (2015) 67:1–48. doi: 10.18637/jss.v067.i01
45. Muzafar M, Calvo-Bado LA, Green LE, Smith EM, Russell CL, Grogono-Thomas R, et al. The role of the environment in transmission of Dichelobacter nodosus between ewes and their lambs. Vet Microbiol. (2015) 179:53–9. doi: 10.1016/j.vetmic.2015.04.010
46. Broster J, Doyle RE. Measuring resource use in sheep with proximity logger technology. In: Proceedings of the 4th Australian and New Zealand Spatially Enabled Livestock Management Symposium (Sydney, NSW) (2013).
47. Taylor D, Schneider D, Brown W, Price I, Trotter M, Lamb D, et al. GPS tracking: use of shelter and shade by Merino ewes. In: 2010 Proceedings of the Sheep CRC Conference (Adelaide, SA) (2010).
48. Chen S, White BJ, Sanderson MW, Amrine DE, Ilany A, Lanzas C. Highly dynamic animal contact network and implications on disease transmission. Sci Rep. (2014) 4:4472. doi: 10.1038/srep04472
49. Dawson DE, Farthing TS, Sanderson MW, Lanzas C. Transmission on empirical dynamic contact networks is influenced by data processing decisions. Epidemics. (2019) 26:32–42. doi: 10.1016/j.epidem.2018.08.003
50. Teke B, Akdag F. The effects of age of lamb and parity of dam and sex and birth type of lamb on suckling behaviours of Karayaka lambs. Small Ruminant Res. (2012) 103:176–81. doi: 10.1016/j.smallrumres.2011.08.012
51. Egerton JR, Roberts DS, Parsonson IM. The aetiology and pathogenesis of ovine foot-rot. I A histological study of the bacterial invasion. J Comparative Pathol. (1969) 79:207–15. doi: 10.1016/0021-9975(69)90007-3
52. Kuhnert P, Cippa V, Hardi-Landerer MC, Schmicke M, Ulbrich S, Locher I, et al. Early infection dynamics of Dichelobacter nodosus during an ovine experimental footrot in contact infection. Schweiz Arch Tierheilkd. (2019) 161:465–72. doi: 10.17236/sat00215
53. Wild S, Allen SJ, Krützen M, King SL, Gerber L, Hoppitt WJ. Multi-network-based diffusion analysis reveals vertical cultural transmission of sponge tool use within dolphin matrilines. Biol Lett. (2019) 15:20190227. doi: 10.1098/rsbl.2019.0227
Keywords: sheep, footrot, transmission, proximity sensor, social network, network based diffusion analysis
Citation: Lewis KE, Price E, Croft DP, Langford J, Ozella L, Cattuto C and Green LE (2022) Social behaviour and transmission of lameness in a flock of ewes and lambs. Front. Vet. Sci. 9:1027020. doi: 10.3389/fvets.2022.1027020
Received: 24 August 2022; Accepted: 17 November 2022;
Published: 01 December 2022.
Edited by:
Tony Waterhouse, Scotland's Rural College, United KingdomReviewed by:
Carrie S. Wilson, Agricultural Research Service (USDA), United StatesCopyright © 2022 Lewis, Price, Croft, Langford, Ozella, Cattuto and Green. This is an open-access article distributed under the terms of the Creative Commons Attribution License (CC BY). The use, distribution or reproduction in other forums is permitted, provided the original author(s) and the copyright owner(s) are credited and that the original publication in this journal is cited, in accordance with accepted academic practice. No use, distribution or reproduction is permitted which does not comply with these terms.
*Correspondence: Katharine E. Lewis, a2F0ZS5sZXdpc0Bub3R0aW5naGFtLmFjLnVr
†Present address: Katharine E. Lewis, Department of Veterinary Medicine and Science, University of Nottingham, Nottingham, United Kingdom
Disclaimer: All claims expressed in this article are solely those of the authors and do not necessarily represent those of their affiliated organizations, or those of the publisher, the editors and the reviewers. Any product that may be evaluated in this article or claim that may be made by its manufacturer is not guaranteed or endorsed by the publisher.
Research integrity at Frontiers
Learn more about the work of our research integrity team to safeguard the quality of each article we publish.