- 1Farm Technology Group, Wageningen University and Research, Wageningen, Netherlands
- 2Animal Production Systems Group, Wageningen University and Research, Wageningen, Netherlands
- 3Livestock Research, Research Centre, Wageningen University and Research, Wageningen, Netherlands
Infectious diseases, particularly bovine respiratory disease (BRD) and neonatal calf diarrhea (NCD), are prevalent in calves. Efficient health-monitoring tools to identify such diseases on time are lacking. Common practice (i.e., health checks) often identifies sick calves at a late stage of disease or not at all. Sensor technology enables the automatic and continuous monitoring of calf physiology or behavior, potentially offering timely and precise detection of sick calves. A systematic overview of automated disease detection in calves is still lacking. The objectives of this literature review were hence: to investigate previously applied sensor validation methods used in the context of calf health, to identify sensors used on calves, the parameters these sensors monitor, and the statistical tools applied to identify diseases, to explore potential research gaps and to point to future research opportunities. To achieve these objectives, systematic literature searches were conducted. We defined four stages in the development of health-monitoring systems: (1) sensor technique, (2) data interpretation, (3) information integration, and (4) decision support. Fifty-four articles were included (stage one: 26; stage two: 19; stage three: 9; and stage four: 0). Common parameters that assess the performance of these systems are sensitivity, specificity, accuracy, precision, and negative predictive value. Gold standards that typically assess these parameters include manual measurement and manual health-assessment protocols. At stage one, automatic feeding stations, accelerometers, infrared thermography cameras, microphones, and 3-D cameras are accurate in screening behavior and physiology in calves. At stage two, changes in feeding behaviors, lying, activity, or body temperature corresponded to changes in health status, and point to health issues earlier than manual health checks. At stage three, accelerometers, thermometers, and automatic feeding stations have been integrated into one system that was shown to be able to successfully detect diseases in calves, including BRD and NCD. We discuss these findings, look into potentials at stage four, and touch upon the topic of resilience, whereby health-monitoring system might be used to detect low resilience (i.e., prone to disease but clinically healthy calves), promoting further improvements in calf health and welfare.
Introduction
Diseases, in particular bovine respiratory disease (BRD) and neonatal calf diarrhea (NCD), are the most common causes of morbidity and mortality in veal calves (1), dairy calves (2), and beef youngstock (3). Despite slightly different prevalence rates (4), disease types affecting dairy and veal calves are similar (5–7). BRD symptoms include hampered respiration, nasal discharge, and coughing (5). A direct symptom of NCD is extremely watery feces (8). Potential risk factors for BRD include: inadequate passive transfer of immunity from colostrum (2, 9); low body weight at arrival in veal calves (10); poor indoor housing conditions compared to outdoor housing (10); and management practices such as weaning, comingling, and castration (11). Potential risk factors for NCD include: high exposure to pathogens causing NCD; factors related to host resistance or susceptibility to disease, e.g., low quality and quantity of colostrum; and factors about the environment that favor the host or agent, e.g., high stocking density and too high or too low ambient temperature and air humidity (12, 13).
Diseases in calves cause significant economic losses (14, 15), due to treatment (16), impaired growth and mortality (17). Diseases also impaired calf welfare (18). Moreover, antibiotic resistance, a major concern in human and veterinary medicine (19), is a serious problem in the veal (20, 21) and dairy industry (22). In addition, the overuse of antibiotics might result in the contamination of surface water near farms due to residues in the urine and feces of animals (23). Given the all-encompassing impact of calf health on sustainability aspects, it is essential that we develop accurate, timely, and practical systems to identify sick calves, in both the dairy and veal sectors.
The common practice for identifying diseases in calves is based on visual appraisal and clinical examinations performed by farmers and veterinarians (5). This practice is linked to a number of disadvantages: (1) Calves identified as sick already show clear clinical symptoms and may have already been sick for a while. For example, clinical signs of BRD might occur later than onset of fever (24), or even without the occurrence of fever (5), and clinical signs of NCD are visible when much of the associated tissue damage to the intestinal submucosa has already occurred (25). (2) Visual appraisal and clinical examinations are typically poor at identifying sick calves. For example, in a study diagnosing BRD in beef calves using clinical examination, the estimated sensitivity and specificity were 61.8 and 62.8%, respectively (26). Many sick calves, hence, go undetected, or require re-treatment due to delayed intervention and inappropriate antimicrobial dosage for the first case, which makes it difficult to promptly treat them, leading to greater chances of spread of disease, poorer animal welfare, and greater negative impacts on economy and environment, overall leading to poor sustainability of production systems involving calves.
Improved methods to detect health problems accurately and on a timely basis in individual calves are warranted. The decreasing cost and increasing implementation of electronic tools allows for the application of “sensing solutions” to animal farming. Behavioral and physiological parameters can nowadays be automatically recorded at individual animal level, continuously and over long periods of time (27, 28). During the past decade, various sensor data models have been proposed for automatic health-monitoring systems in dairy and veal calves. To date, however, there has been no systematic review presenting the associated gaps in research, while literature reviews have previously been done for pigs (29, 30), dairy cows (31, 32), dairy sheep (33), and dairy calves (27, 28). The objectives of this literature review were hence: to investigate previously applied sensor validation methods and gold standards; to identify sensors used on calves, the parameters these sensors monitor, and the statistical tools applied to identify diseases; and to explore potential research gaps to point to opportunities for future research.
Methods
Definitions
Animals included in this review were bovine animals aged <1 year; these include “calf” or “calves” (pre-weaned or weaned), heifers (weaning to 1 year of age), growing bulls (after arrival at the fattening farm up to 1 year of age), and beef cattle (early fattening period until 1 year of age). Precision livestock farming (PLF) is defined based on Berckmans (34) as “measuring variables on the animals, modeling these data to select information, and then using these models in real time for monitoring and control purposes”. We defined the following terms–SENSOR: an automatic tool capable of recording activities, behaviors, physiology, and body size of calves continuously; MODEL: a mathematical tool that describes the relations between the sensor output and the actual values of the measured parameters of the physical environment; VALIDATION: the process of determining the measurement ability of automatic tools relative to a gold standard using statistics. DISEASE: sickness status of an animal occurred naturally or induced by disease challenges.
We defined four stages of development of a particular sensor technique for disease detection based on Rutten et al. (31) (Figure 1):
• Stage one: SENSOR TECHNIQUE-applying sensor technology to automatically or manually record behavioral or physiological parameters in animals, visualizing these parameters.
• Stage two: DATA INTERPRETATION-changes in data are detected and connected to changes in behavior and physiology with an established link to the animal's health status;
• Stage three: INFORMATION INTEGRATION-multiple data resources, e.g., treatment records and sensor data, are integrated to direct the farmer to potential problems that need attention;
• Stage four: DECISION SUPPORT–a sensor system that aids to make a decision, e.g., whether to treat an animal or not; what to treat the animal for?
Inclusion and Exclusion Criteria
Peer-reviewed scientific articles describing applying sensors to calves were eligible for inclusion. Only articles based upon original data were included. Included articles were written in English, with complete, full-text documents available. To provide up-to-date review, only articles published between 2009 and 2021 were included. Manuscripts published after the completion of the literature search were not included (i.e., after May 10, 2021). Exclusion and inclusion criteria for the systematic review were based on an previous work by Beaver et al. (35) and agreed upon by all co-authors.
Search Strategy
Systematic searches were conducted using the Web of Science Core Collection database because it has high coverage rates of animal behavior and welfare and bio-system engineering journals with significant PLF contents.
The following search terms were applied: (calf OR calves OR dairy calf OR dairy heifer OR heifer calf OR heifers OR young cattle) AND (BRD OR bovine respiratory disease OR calf comfort OR calf health OR diarrhea OR group housing OR health OR precision livestock OR precision livestock farming OR proneness to disease OR welfare) AND (automatic OR automated measurement OR automated measures OR detection OR diagnosis OR disease monitoring OR evaluation OR modeling OR non-invasive detection OR prediction OR validation) AND (accelerometer OR activity sensor OR artificial intelligence OR automatic milk feeder OR bioacoustics OR computer vision OR electronic monitoring OR infrared thermography OR low-cost sensor OR non-invasive technology OR radio frequency identification OR reticulo-rumen bolus OR statistical process control OR sound analysis OR 3-D sensor). The selection of these search terms was based on initial screening of relevant articles to gain general background information and expert opinion.
Selection Process
The primary outcomes were selected based upon a four-step screening and appraisal process (Figure 2):
• Step one. Scanning the titles–filter out irrelevant results such as review articles in automatic detection, original articles of health monitoring in calves without applying sensor technology, or original articles of automatic health-monitoring systems in mature cattle or other species.
• Step two. Evaluating abstracts–identify and remove irrelevant articles.
• Step three. Snowballing–checking and selecting references within selected articles.
• Step four. Eligibility. Full texts of the remaining articles were read in detail. Original experimental studies were excluded if not aiming at health monitoring in calves aged up to 1 year using sensor technology.
Data Extraction
From each included article, where applicable, we recorded the objectives, animal category, parameters measured, sample size, gold standards for validation, sensors used, and measurements used to assess the performance of the sensors or algorithms. Missing information was noted down as “not available.” The results were pooled in the form of a table (Supplementary Material). The reliability for data extraction was tested by author 1 (DS) on a random subset of 20 articles, with a result of 100% agreement.
Data Management
Extracted data were entered into and managed in excel spreadsheets (version 2016, Microsoft Corp., Redmond, WA, RRID:SCR_016137).
Results and Discussion
Following the article-selection process described above, 54 articles were included in this review (Figure 2). As shown in Figure 3, 26 articles fell into stage one (sensor technique), 19 articles fell into stage two (data interpretation), and 9 articles fell into stage three (information integration). We found no articles at stage four (decision support).
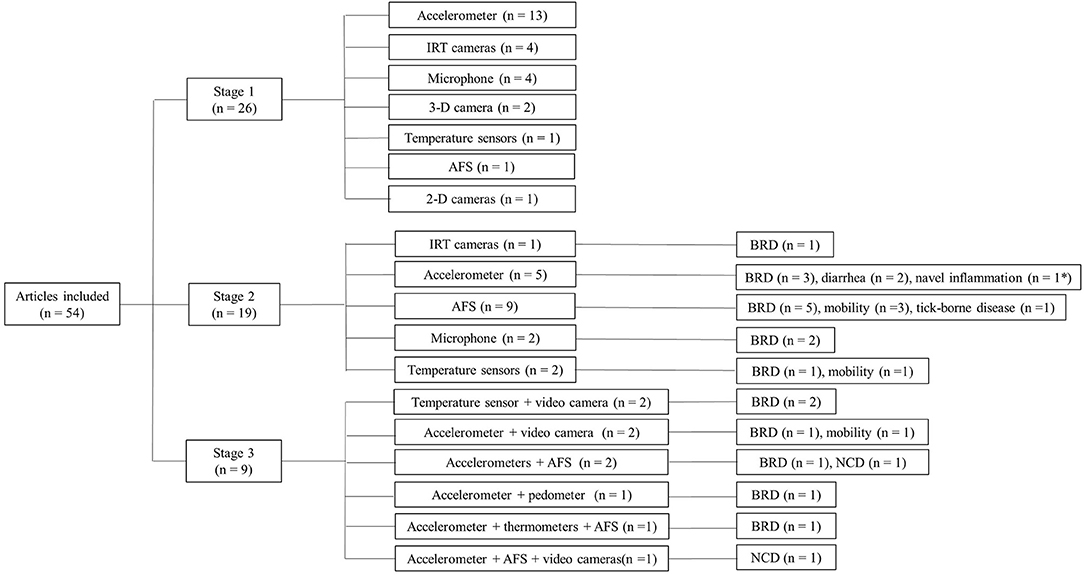
Figure 3. Distribution of stages of included articles. *In Studds et al. (56) both diarrhea and navel inflammation were studied.
Studies at these different stages use different validation methods and gold standards. Studies at stage one aim to check that a given sensor is accurately recording a particular behavioral or physiological parameter of interest. These studies typically use (a) manually collected parameter(s) as gold standard for their validation, e.g., video observations of lying bouts or rectal body temperature measurements using a thermometer. Stage two and stage three studies aim to identify sick calves as early as possible. Stage two and three studies develop and test algorithms applied to sensor data to accurately detect sick individuals. Manual health-assessment protocols are typically used as gold standards to develop and test these algorithms (Table 1).
We first define what is meant by “validation” in this review as well as define the terms used in this context, i.e., sensitivity, specificity, accuracy, and positive and negative predictive value. We follow up with a description of the different gold standards that have been used at the different stages of investigation. Next, we describe the various sensors that have been used in calf-health-monitoring research, the parameters these sensors record, and their accuracies in these recordings. We end by presenting the current research at stage two (data interpretation) and stage three (information integration), revealing important knowledge gaps between stage three and stage four (decision support), suggesting the direction for future study that will enable the bridging of these gaps, hence reaching automated health-related data interpretation and complete decision-support systems for calf production systems.
Validation
The validation assessments at different stages of studies share common principles. Validation assessments are typically calculated via so-called confusion matrices (Table 2) (36). Confusion matrices reveal relationships between the sensor of interest, the selected gold standard (see the below formulas for: sensitivity, specificity, accuracy, precision, and negative predictive values) and the underlying prevalence of the disease interest. “Positive and negative” show the sensor (or model) output (a response of “yes” or “no” to the disease detection), while “true and false” reflects whether the sensor (or model) output is in line with the gold standards in a pre-specified time window (i.e., whether the prediction matches the reality). When comparing article outcomes, it should be noted that sensitivity and specificity are affected by characteristics of the sensor, while accuracy, precision, and negative predictive values are affected by the prevalence of disease or behavior based on the dataset: the higher the prevalence, the better the accuracy, precision, and predictive values for the given dataset. Model developments are usually aimed to enhance the contrast in a sensor system output for the purpose of threshold evaluations (e.g., sensitivity, specificity, or accuracy) over a given range. Common methods used for model developments are correlation, area under curve (36), and receiver operating characteristic curves (36).
Gold Standard
To obtain a sound validation of a sensor or PLF system, an objective “gold standard” is needed. In this regard, studies at stage one to stage four require different gold standards. At stage one, gold standard means “variables of interest,” i.e., behavioral or physiological parameters; at stage two, three, and four, gold standard usually refers to the identification of disease, typically via a manual “clinical examination.”
Stage one studies, where sensors are checked directly for their ability to record behavioral or physiological parameters, tend to use manual sampling of these behavioral or physiological parameters. For sensors recording behavioral parameters, behavioral observations of videos, continuous or at regular intervals, are a commonly used reference for validation. Continuous sampling of focal animals will provide the most accurate data for calf behavior, but is a time-consuming exercise. For certain, long-term, so-called “state” behaviors, instantaneous scan sampling at regular intervals may provide an accurate enough gold standard and is less time consuming, for example, meal time and frequency over a 3 day period can be detected accurately with instantaneous scan sampling at short intervals of 30 s and 1 min (37). However, one main disadvantage of video observation is that it is labor intensive, and requires training to achieve appropriate observer reliability; and observer error might occur (38).
For sensors recording physiological parameters, manual measurements of these physiological parameters are also used as gold standards. Sensors recording body temperature, e.g., body surface temperature (39), eye temperature (40, 41), and rectal area temperature (40), typically use manually recorded rectal temperature as gold standard. When validating body dimensions in calves and heifers, manual measurements of body weight and dimensions are common, including body weight (42, 43), hip height (42), and wither height (42).
With increasing research into validating sensors in terms of how accurately they record behavior or physiological parameters, previously validated sensors may be used as automated gold standard to validate new sensors, which significantly reduces labor required for these types of stage one studies. The Hobo Pedant G Data Logger, for example, has been previously used as a gold standard to validate another accelerometer, the AfiTag II, for lying behavior and step count (44).
Stage two and three studies aim to identify sick calves. Here, a clinical examination is the most commonly used gold standard for disease diagnosis (Table 1) (45–50). Various protocols have been used in this type of study, such as the Wisconsin clinical respiratory score (51) and the Wisconsin calf health scoring chart (52). Further information can be added to these clinical examinations to complement the gold standard, including metadata such as management information (e.g., calf registration or enrollment data), morbidity and mortality data from the farm (53, 54), BW (55–57), post-mortem examination (58, 59), or blood parameters (16, 24, 51–53, 60–62). Of all the clinical examination protocols, the (modified) Wisconsin calf health score chart was the most commonly used protocol (47, 48, 52, 63, 64). Gold standards without clinical examination, e.g., from blood analysis (65), or a combination of BW, biochemical parameters from blood and fecal samples, and rectal temperature (66) have also previously been used. Clinical examination can be combined with clinical chemistry, for example, via blood sampling, to improve the accuracy of health assessment.
Visual appraisal of disease, e.g., BRD, relies on the experience of observers, and may have low specificity and be highly variable between observers based on their level of experience (67). Thus errors from the clinical examinations may transfer to the corresponding models (58). Improvements are necessary for the clinical examinations used as gold standards for the development of algorithms to detect diseases in calves. Firstly, training in clinical examination and high inter-observer consistency are required. Secondly, to better relate clinical examinations to model outcome, consistent and explicit definitions of diseases across the literature are needed.
When performing time-consuming clinical examinations for use as a gold standard, the frequency of these examinations needs to be carefully considered. As observed by the current authors, daily clinical examinations of calves can provide better timely reference, at the cost of disturbance to the group and high labor requirements. However, a low frequency of clinical examinations will result in late detection, making it difficult to develop an early disease detection algorithm (68). Previous research applied different frequencies–for clinical examinations–ranging from daily to weekly (Table 1). Clinical examinations combining two different frequencies applied at different life stages were also found, e.g., before (twice a week) and after the weaning period (once per week) of dairy calves (51, 52). To the author's knowledge, no study has yet compared the effect of different frequencies of clinical examinations on the accuracy of disease-detection models.
In summary, clinical examination is the most common gold standard used in the development of algorithms to identify sick calves. The Wisconsin calf health score chart was identified as a commonly used protocol for clinical examination in this context, with a sensitivity of 62.4, and specificity of 74.1% (69). Clinical references with high accuracy, consistent guidelines, and easy-to-follow protocols are needed for disease detection in calves. A standardized clinical scoring system will benefit the validation of the sensors and algorithms, making it easier to compare the performance of different algorithms. In addition, ultrasonographic assessment of the thorax could be a useful tool to assess BRD detection in calves (70, 71).
Stage One: Sensor Technology Used in Calves
Data sources used in calves include automatic feeding stations (AFS), accelerometers, microphones, infrared thermography (IRT) cameras, temperature sensors (i.e., boluses, thermometers), radiofrequency identification (RFID) chips, 3-D cameras, and 2-D cameras.
Automatic Feeding Stations
AFS, such as automated milk dispensers for pre-weaned calves and automatic concentrate bunks for post-weaned calves, and water bins, have been used in studies aimed at automated health monitoring in young calves, hence stage two research. These AFS can measure a wide range of parameters linked to feeding and drinking patterns, including daily feed intake (4, 45, 47, 52–55, 57, 72–74), frequency and duration of rewarded and unrewarded visits (4, 45, 47, 52–55, 57, 58, 61, 62, 72, 74), drinking speed (milk) (47, 52–54), water-drinking behavior (intake, time, and frequency) (62, 74), and other feeding behaviors (head-down duration at the AFS) (55, 72), time-to-bunk: time to approach feeding stations following feed-truck delivery (55, 72), and duration of unrewarded visit intervals (55). We did not find validation studies for common systems such as Förster-Technik GmbH (n = 5; Engen, Germany) or GrowSafe Systems (n = 3; GrowSafe Systems Ltd., Airdrie, AB, Canada). However, we found a study validating automatic feed and water bin (Intergado ® Ltd, Contagem, Minas Gerais, Brazil), and showed that this system seem to be able to measure feeding time, water drinking time, feed intake per visits, and water intake per visits with high correlation compared with the gold standards (r2 = 0.917, 0.963, 0.973, and 0.986, respectively) (74).
Accelerometers
Accelerometers are attached to the body of the calf, generally to one of the limbs, neck, or ear (tag). They are typically used to assess various activity-related behaviors. Accelerometers are accurate in recording calf behaviors, including lying time (44, 75–78), lying bouts (44, 75, 76, 78), standing time (75, 78), standing bouts (75), step counts (44, 79), locomotion time (78), gait scoring (79), feeding time (73, 78), sucking behavior from dams (38), and licking or sucking at objects, other calves' bodies, or own body (78). After more than 10 years of development, accelerometers are now used to record a broader variety of behaviors and more detailed behavioral patterns, e.g., recognizing between galloping, trotting, and walking (79), and recording behaviors such as eating, water drinking, chewing, positive social interactions, self-grooming, and inactivity (80). Step counts were originally measured by pedometers (60). This activity parameter was later integrated into accelerometers (44, 47, 66, 79, 81).
Temperature Sensors
Boluses, IRT cameras, and thermometers are used to measure body temperature. These temperature sensors have been developed to record body temperature at different anatomical areas, enabling the measurement of rectal temperature (50, 59) or temperature around the rectal area (40), reticulo-rumen temperature (24, 46), eye temperature (7, 16, 40, 41, 82), cheek temperature (7, 82), back, shoulder, and side temperature (7), and temperature at the base of the tail (39). These cameras have also shown high accuracy in measuring cheek temperatures (82), but have not been found to be highly accurate in measuring temperature around the rectal area (40) or core body temperature (41). In terms of eye temperature, IRT cameras seem to show varying levels of correlation between eye temperature and rectal temperature, e.g., high correlation (R2 ≥ 0.99) (82), low correlation (R2 ≤ 0.32) (40). This might be partly due to the use of an detection algorithm (82). A prototype thermometer provided by Nogami et al. (39) has been found to measure tail temperature with high correlation compared with rectal temperature in calves.
Other Sensors and Techniques
Microphones, when integrated into sound-acquisition systems, can detect abnormal cough sounds (51, 65, 83) and rumination sounds in calves (84–86). The performance of microphones varied in calves of different ages. Microphones accurately recorded rumination time in pre-weaned calves (85), but overestimated rumination time in weaning calves (86). RFID ear tags can be applied to monitor grooming behavior (measured via proximity to a brush) in heifers (87). IRT cameras have also been used to assess respiration rate in calves, at a high level of accuracy (88).
With the application of approaches such as computer vision or machine learning, an even broader range of parameters might be recorded with the available sensors. For example, Carslake et al. (89) applied machine-learning approaches to multi-class behavior identification (including locomotor play, self-grooming, ruminating, non-nutritive suckling, nutritive suckling, active lying, and non-active lying) as well as behavior quantification (i.e., behavior distribution) using a single sensor (comprised of an accelerometer and gyroscope) in calves. Computer vision allowed 2-D cameras to identify multiple behaviors, e.g., pen entering, pen leaving, standing or lying static behavior, turning, and feeding and drinking behaviors (90). 3-D cameras can monitor growth and morphology (i.e., BW, body mass, hip height, and wither height) in young calves and heifers (42, 43).
Knowing which parameters that sensors (or sensor combinations) can accurately measure can contribute to the development of an efficient sensor system at stage two and three. For example, accelerometers are not accurate in screening rumination time in calves (73), but this can instead be achieved by microphones (84, 85). Both accelerometers and AFS can record feeding and water-drinking behaviors, but AFS can record these behaviors directly without having to apply statistical models and are non-intrusive, i.e., not attached to the animal (80). In addition, no further hardware is needed when extracting data from AFS compared with accelerometers.
To sum up, available sensors (AFS, accelerometers, IRT cameras, microphones, and 3-D cameras) are accurate in measuring different behavioral or physiological parameters in calves, and approaches such as machine learning and computer vision broaden the range of behaviors that sensors can record. Future work should further develop behavior classification and quantification by applying computer-vision and machine-learning approaches.
Stage Two: Data Interpretation–Outcomes of Algorithms
In order to develop a sensor-based system that detects sick calves, i.e., sensor technology combined with algorithms, stage two studies must follow three steps: (1) identify how behavioral or physiological parameters change with disease, identified via a gold standard (this includes the selection of both parameters of interest and corresponding sensors); (2) investigating how these behavioral and physiological changes vary at which stage of disease they can first be detected; and (3) developing and testing the accuracy (or performance) of algorithms in detecting sick calves based on changes in these behavioral and physiological parameters. In this section, we highlight the algorithms that can detect diseases prior to clinical confirmation (Table 3), and summarize changes in behavioral and physiological parameters in response to disease as well as time course: disease states in animals typically lead to both behavioral and physiological changes over time.
Changes in Feeding Behaviors
Feeding behaviors and patterns, including intake, frequency, speed, and duration at various time ranges, are commonly used parameters for the early detection of disease in calves (91, 92). Note that most studies look at feeding behaviors aggregated at a daily level. With the application of RFID, individual calves are identified at AFS, whereby individual feeding behaviors can be recorded. For example, pre-weaned calves diagnosed with BRD drank less milk on the day of clinical examination (47) and on the first day of treatment (53), drank milk slower 4 days prior to the clinical examination (53), and performed fewer unrewarded visits to the milk dispenser 3 days prior to (52), and on the first day of treatment (53). Moreover, net daily energy intake (calculated for each calf by summing daily milk replacer and concentrate intake values) (52) and DMI (55), were reduced in BRD-infected calves in the time prior to the clinical examination, e.g., 3 days in Johnston et al. (52) and 6.8 days in Jackson et al. (55). In calves diagnosed with NCD, daily milk intake and time at water trough dropped 4 days prior to clinical examination (7).
Changes in Activity
Changes in activity parameters, such as step counts and lying behaviors, are used to detect sick calves. In calves diagnosed with BRD, for example, step counts (<6 days), lying bouts (<5 days), standing time (<1 day) were reduced (81). In calves diagnosed with NCD, results are inconclusive regarding activity: lying bouts were found to both decrease (<7 days) (7) and increase (<7 days to 3 days) (63), and lying durations were found to both decrease (<6 days to 3 days) (63) and increase (<7 days) (7). Finally, calves with inflamed navels show reduced lying time at day level after arrival at fattening farms compared with healthy calves (56).
Changes in Other Parameters
Coughing which is a typical symptom of BRD can be detected using microphones (83). Note that as opposed to activity and feeding behaviors, coughing has so far only been measured at group level. An increased coughing frequency was found to be correlated to BRD occurrence in group-housed calves (51, 65).
Changes in body temperature can be used to detected sick calves before clinical examination. BRD-diagnosed calves showed increases in orbital (eye plus 1 centimeter surrounding the eye) maximum temperature (16) and reticulo-ruminal temperature, e.g., −136 to −12 h (24) and −3.5 days (46) relative to diagnosis. One important methodological consideration with thermometers is that recorded temperatures differ based on the body area that is investigated. For example, skin temperature was consistently 2 to 3°C lower than the rectal temperature (39), while reticulo-rumen temperature was consistently 0.57°C higher than rectal temperature (24). As long as these differences between recorded temperature and body temperature are consistent, this should not affect the detection of temperature increases due to diseases in calves. In calves diagnosed with NCD, the temperature of the side flank and shoulder increased at least seven days prior to diagnosis (7).
Changes in social behaviors were also detected in sick calves. In a modeled disease challenge study (calves were infected with Mannheimia haemolytica), sick calves were found to show decreases in daily social grooming time and daily social lying time (lying within one body length of another calf) (48).
Some other behavioral parameters can be well recorded by sensors, but their potential in early disease detection is yet unknown. These include sucking behavior (38), rumination time (86), and play behavior (93–95). Further research into the link between these parameters and disease is warranted.
As explained above, behavior and physiology change with disease, and these changes can be detected using sensors and algorithms. Theoretically, “behaviors that are less critical for immediate survival and primarily support long-term fitness are most affected by disease” (96), such as play and exploratory behaviors (97). In practice, the type of diseases and the age of the animals also need to be taken into consideration as they might influence behavioral deviations. For example, in parasitized beef steers (aged between 4 and 11 months) and BRD-infected dairy calves, changes in activity (e.g., lying, standing, and step counts) enabled a better disease detection than feeding behaviors such as frequency and duration of feeding and drinking (66) and feed intake (47). In identifying NCD-infected calves and BRD-infected steers, however, feeding behaviors (e.g., the number of unrewarded visits to an automated milk dispenser, DMI, and bunk visit duration) permitted a more accurate detection of disease compared with activities such as lying and standing duration (4, 72). In addition, certain diseases result in behavioral changes that are easier to detect at an earlier stage. NCD-diagnosed calves, for example, displayed earlier and more consistent changes in feeding behaviors compared with BRD-diagnosed calves (53). Further research is hence needed into identifying the best, most sensitive behavioral and physiological parameters that can identify specific diseases or diseased state on a generic level.
Stage Three: Information Integration–Outcomes of Models
To date, sensor fusion (i.e., two or multiple sensors) was applied in a number of studies (n = 9, Figure 3), in which data from accelerometers, thermometers, and AFS are integrated into one model to identify diseases including BRD (64, 72) and NCD (4, 7). Information integration, however, means more than a multiple-sensor tool. First, “integration” does not mean accumulating all the data obtained from different sources. In the design of systems at stage three, redundancy needs to be reduced for a disease-detection model. To reach this, data mining (98), which allows for a more complete understanding of different parameters in relation to disease occurrence, is a prerequisite. Data mining allows for the selection of the key parameters, the variation of which reflects health status with high accuracy. In this way, by reducing the redundancy, the number of sensors used and possibly attached to a calf will be reduced. Second, multiple data sources mean that sensor data are not the only sources of data. Economic insights, for example, were also suggested to be considered for the treatment decisions (24, 57).
Given that many sensors and techniques are already commercially available, it is crucial to choose an appropriate sensor system when recoding certain parameters. For example, the combination of video cameras and sensors (including thermometers, accelerometers, or AFS), although popular for research (n = 5), seems impractical for on-farm settings. This might be due to the number of cameras required and the time-consuming process of analyzing the video footage. However, a recent study show that artificial intelligence is able to identify the physiology and behavior of animals using video footages with high accuracy (90), allowing for less labor.
Therefore, “information integration” means selecting as few meaningful parameters indicative of diseases as possible when developing models (thereby avoiding redundancy). The integrated systems will give an alert when the current status of a calf deviates from its earlier patterns, i.e., being sick. Ideally, models at this stage include a minimum number of sensors per animal, which is advantageous in terms of costs and maintenance labor but also in maintaining the integrity and freedom of movement of calves.
Stage Four: Decision Support–Automation
At stage four, decision support means that the integrated system can identify which disease is occurring based on the developed model. Farmers can refer to the decision made by the system as support. To the author's knowledge, no such systems are available for early disease detection in calves. An example of a stage four system in dairy cows is estrous detection and automatic identification of the best way to inseminate the cow (99). In the situation of early disease detection in calves, however, so far only alerts are available.
For the future, automation is crucial–a decision-support system with an easy-to-operate user interface is what farmers need for an easy identification of sick calves. Current models may give some form of alert, yet cannot give automatic decision support. Another important characteristic of such systems is the possibility for the farmers to enter feedback (e.g., whether the identified individual was truly ill with the suspected disease and whether the treatment was efficient) so that the system can continuously learn and adapt to the specific farming conditions. Knight (98) suggested a business model that bridges information integration and decision support. In the provided business model, farmers are buying a service from service providers. A service provider purchases the technologies from different developers, and provides the service of installation, maintenance, data collection, and data integration, thereby providing decision support to the farmers. However, at the same time we believe that technology should not replace but, rather, support management decisions made by the farmer.
Introducing “Resilience” Theory
Following the above four-stage approach, the decision-support system is regarded as a screening tool that aims to detect diseases at an early stage and provides a short list of “positive cases” of animals that have a sufficiently high chance of being prone to develop the targeted disease. Hence the farmer and the care taker is provided a window of opportunity to check for the clinical status. Additionally some preventive measures can be taken or a predefined health protocol can be applied. A negative outcome of a decision-support system predicts the likelihood of a clinically healthy animal of becoming diseased in the near future, i.e., its predisposition to diseases then is generally speaking a balance between environmental infection pressure and its immune system functioning. In a paper discussing sensor technologies in dairy farming, Knight argued that “the focus is on improving overall husbandry, rather than “solving” specific disease problems” (98). The same focus should apply to the dairy and veal industry as well. We therefore introduce “resilience” theory, through which the developed system might be able to quantify the resilience of individual animals, thereby identifying animals in a low-resilience state. This potentially allows for early intervention in the husbandry system, whereby the environment or management are specified in such a way that low-resilient individuals and the herd as a whole can maintain relatively healthy states.
Resilience in farm animals has been defined as “the capacity of the animal to be minimally affected by a disturbance or to rapidly return to the physiological, behavioral, cognitive, health, affective and production states that pertained before exposure to a disturbance” (100). Calves falling sick can be equaled to a complex system transiting from one stable state (healthy) to another (unhealthy), with the return to the original state being more difficult than the simple cancellation of factors that caused the change in state. Such shifts in complex systems have been termed “critical transitions” or “tipping points” (101). When such complex systems are close to tipping points, the recovery rate of that system from small perturbations becomes very slow, and this is known as “critical slowing down” (CSD) (102). For example, a cow showing “CSD” before parturition, in this case by using an accelerometer to assess activity (e.g., low average eating time, a disturbed circadian rhythm, and variance in ear temperature), is likely to develop periparturient disorders (103). CSD, which can be revealed through dynamic aspects of sensor data, is here seen as an increase in variance in the activity data, hence a loss of regularity. CSD, therefore, reflects a loss of resilience (101, 102). In still clinically healthy individuals, CSD reflects the animal's vulnerability to pathogens prior to the disease, and hence reflects a state of low resilience. Identifying CSD in sensor data patterns of “low-resilient” individual animals, would enable, for example, timely change of the environment of this animal in an attempt to increase its resilience (e.g., by removal of stressors, or the improvement of nutrition, etc.).
Current sensor tools focus on detecting the early stages of disease, while sensor technology already allows us to analyze the dynamics of physiology and behavior with high accuracy. Advanced analytical tools can estimate resilience status from the micro-recoveries in the data flow (104). These tools may eventually also be applicable to advanced and innovative calf health management systems.
Conclusions
This review summarized the literature on sensor systems so far studied in the context of health monitoring in calves between 2009 and 2021, and revealed the current phase of development by categorizing each study based on a four-stage system (sensor technology, data interpretation, information integration, decision support). Our literature search demonstrated that most studies up to now are at stage one (sensor technique) or stage two (data interpretation), and a few studies are at the beginning of stage three (information integration). Accelerometers, IRT cameras, microphones, and 3-D cameras can be accurate in measuring behavioral and physiological parameters in calves (at stage one). Deviations in behaviors (e.g., feeding, lying, and social behaviors), activity, and body temperature can be detected prior to the clinical examination (at stage two and three), and are promising for developing algorithms. To develop a health detection model with a minimal number of sensors, it is crucial to select appropriate sensor systems that can record the most relevant parameters that show clear changes in response to diseases in calves. Clear gaps in research include stage three (information integration) and stage four (decision support) systems, as well as forecasting methods via the identification of low-resilience animals.
Data Availability Statement
The original contributions presented in the study are included in the article/Supplementary Material, further inquiries can be directed to the corresponding authors.
Author Contributions
DS wrote the review (main body) and LW, PT and KR contributed during the discussion and reviewed the preliminary versions of the manuscript. All authors contributed to the article and approved the submitted version.
Funding
This study was financially supported by Stichting Brancheorganisatie Kalversector (SBK), the Dutch Ministry of Agriculture, Nature and Food Quality.
Conflict of Interest
The authors declare that the research was conducted in the absence of any commercial or financial relationships that could be construed as a potential conflict of interest.
Publisher's Note
All claims expressed in this article are solely those of the authors and do not necessarily represent those of their affiliated organizations, or those of the publisher, the editors and the reviewers. Any product that may be evaluated in this article, or claim that may be made by its manufacturer, is not guaranteed or endorsed by the publisher.
Supplementary Material
The Supplementary Material for this article can be found online at: https://www.frontiersin.org/articles/10.3389/fvets.2021.761468/full#supplementary-material
References
1. Pardon B. Longitudinal Study on Morbidity and Mortality in White Veal Calves in Belgium. BMC Vet Res. (2012) 8:26. doi: 10.1186/1746-6148-8-26
2. Windeyer MC, Leslie KE, Godden SM, Hodgins DC, Lissemore KD, LeBlanc SJ. Factors associated with morbidity, mortality, and growth of dairy heifer calves up to 3 months of age. Prev Vet Med. (2014) 113:231–40. doi: 10.1016/j.prevetmed.2013.10.019
3. Mötus K, Viltrop A, Emanuelson U. Reasons and risk factors for beef calf and youngstock on-farm mortality in extensive cow–calf herds. Animal. (2018) 12:1958–66. doi: 10.1017/S1751731117003548
4. Sutherland MA, Lowe GL, Huddart FJ, Waas JR, Stewart M. Measurement of dairy calf behavior prior to onset of clinical disease and in response to disbudding using automated calf feeders and accelerometers. J Dairy Sci. (2018) 101:8208–16. doi: 10.3168/jds.2017-14207
5. McGuirk SM, Peek SF. Timely diagnosis of dairy calf respiratory disease using a standardized scoring system. Anim Heal Res Rev. (2014) 15:145–7. doi: 10.1017/S1466252314000267
6. Marcato F, van den Brand H, Kemp B, van Reenen K. Evaluating potential biomarkers of health and performance in veal calves. Front Vet Sci. (2018) 5:133. doi: 10.3389/fvets.2018.00133
7. Lowe GL, Sutherland MA, Waas JR, Schaefer AL, Cox NR, Stewart M. Physiological and behavioral responses as indicators for early disease detection in dairy calves. J Dairy Sci. (2019) 102:5389–402. doi: 10.3168/jds.2018-15701
8. Renaud DL, Buss L, Wilms JN, Steele MA. Technical note: is fecal consistency scoring an accurate measure of fecal dry matter in dairy calves? J Dairy Sci. (2020) 103:10709–14. doi: 10.3168/jds.2020-18907
9. Edwards TA. Control methods for bovine respiratory disease for feedlot cattle. Vet Clin North Am Food Anim Pract. (2010) 26:273–84. doi: 10.1016/j.cvfa.2010.03.005
10. Brscic M, Leruste H, Heutinck LF, Bokkers EA, Wolthuis-Fillerup M, Stockhofe N, et al. Prevalence of respiratory disorders in veal calves and potential risk factors. J Dairy Sci. (2012) 95:2753–64. doi: 10.3168/jds.2011-4699
11. Smith RA, Step DL. Bovine respiratory disease looking back and looking forward, what do we see? Vet Clin Food Anim. (2020) 36:239–51. doi: 10.1016/j.cvfa.2020.03.009
12. Smith DR. Field disease diagnostic investigation of neonatal calf diarrhea. Vet Clin North Am Food Anim Pract. (2012) 28:465–81. doi: 10.1016/j.cvfa.2012.07.010
13. Pardon B, Alliet J, Boone R, Roelandt S, Valgaeren B, Deprez P. Prediction of respiratory disease and diarrhea in veal calves based on immunoglobulin levels and the serostatus for respiratory pathogens measured at arrival. Prev Vet Med. (2015) 120:169–76. doi: 10.1016/j.prevetmed.2015.04.009
14. Yazdanbakhsh O, Zhou Y, Dick S. An intelligent system for livestock disease surveillance. Inf Sci. (2017) 378:26–47. doi: 10.1016/j.ins.2016.10.026
15. Wang M, Schneider LG, Hubbard KJ, Smith DR. Cost of bovine respiratory disease in preweaned calves on us beef cow–calf operations (2011–2015). J Am Vet Med Assoc. (2018) 253:624–31. doi: 10.2460/javma.253.5.624
16. Schaefer AL, Cook NJ, Bench C, Chabot JB, Colyn J, Liu T, et al. The non-invasive and automated detection of bovine respiratory disease onset in receiver calves using infrared thermography. Res Vet Sci. (2012) 93:928–35. doi: 10.1016/j.rvsc.2011.09.021
17. Windeyer MC, Timsit E, Barkema H. Bovine respiratory disease in pre-weaned dairy calves: are current preventative strategies good enough? Vet J. (2017) 224:16–7. doi: 10.1016/j.tvjl.2017.05.003
18. Millman ST. Sickness behaviour and its relevance to animal welfare assessement at the group level. Anim Welf. (2007)16:123–5.
19. Awasthi A, Awasthi A, Riordan D, Walsh J. Non-invasive sensor technology for the development of a dairy cattle health monitoring system. Computers. (2016) 5:23. doi: 10.3390/computers5040023
20. Havelaar AH, Graveland H, Kassteele J. Van De, Zomer TP, Veldman K. A summary index for antimicrobial resistance in food animals in the Netherlands. BMC Vet Res. (2017) 13:305. doi: 10.1186/s12917-017-1216-z
21. Mitrenga S, Popp J, Sartison D, Deutsch S, Meemken D, Kreienbrock L, et al. Veterinary drug administration in german veal calves: an exploratory study on retrospective data. Prev Vet Med. (2020) 183:105–31. doi: 10.1016/j.prevetmed.2020.105131
22. Earley B, Arguello A, O'Riordan E, Crosson P, Cappelleri A, McGee M. Antimicrobial drug usage from birth to 180 days of age in irish dairy calves and in suckler beef calves. J Appl Anim Res. (2019) 47:474–85. doi: 10.1080/09712119.2019.1665525
23. Mostert P. The impact of diseases in dairy cows on greenhouse gas emissions and economic performance. (Ph.D. thesis). Wageningen University, Wageningen, Netherlands (2018)
24. Timsit E, Assie S, Quiniou R, Seegers H, Bareille N. Early Detection of bovine respiratory disease in young bulls using reticulo-rumen temperature boluses. Vet J. (2011) 190:136–42. doi: 10.1016/j.tvjl.2010.09.012
25. Schroeder ME, Bounpheng MA, Rodgers S, Baker RJ, Black W, Naikare H, et al. Development and performance evaluation of calf diarrhea pathogen nucleic acid purification and detection workflow. J Vet Diagnostic Investig. (2012) 24:945–53. doi: 10.1177/1040638712456976
26. White BJ, Renter DG. Bayesian estimation of the performance of using clinical observations and harvest lung lesions for diagnosing bovine respiratory disease in post-weaned beef calves. J Vet Diagnostic Investig. (2009) 21:446–53. doi: 10.1177/104063870902100405
27. Costa JHC, Cantor MC, Neave HW. Symposium review: precision technologies for dairy calves and management applications. J Dairy Sci. (2021) 104:1203–19. doi: 10.3168/jds.2019-17885
28. Morrison J, Renaud DL, Churchill KJ, Costa JHC, Steele MA, Winder CB. Predicting morbidity and mortality using automated milk feeders: a scoping review. J Dairy Sci. (2021) 104:7177–94. doi: 10.3168/jds.2020-19645
29. Matthews SG, Miller AL, Clapp J, Plotz T, Kyriazakis I. Early detection of health and welfare compromises through automated detection of behavioural changes in pigs. Vet J. (2016) 217:43–51. doi: 10.1016/j.tvjl.2016.09.005
30. Martinez-Aviles M, Fernandez-Carrion E, Lopez Garcia-Baones JM, Sanchez-Vizcaino JM. Early detection of infection in pigs through an online monitoring system. Transbound Emerg Dis. (2017) 64:364–73. doi: 10.1111/tbed.12372
31. Rutten CJ, Velthuis AGJ, Steeneveld W, Hogeveen H. Invited review: sensors to support health management on dairy farms. J Dairy Sci. (2013) 96:1928–52. doi: 10.3168/jds.2012-6107
32. Silva SR, Araujo JP, Guedes C, Silva F, Almeida M, Cerqueira JL. Precision technologies to address dairy cattle welfare: focus on lameness, mastitis and body condition. Animals. (2021) 11:1–17. doi: 10.3390/ani11082253
33. Vaintrub MO, Levit H, Chincarini M, Fusaro I, Giammarco M, Vignola G. Review: precision livestock farming, automats and new technologies: possible applications in extensive dairy sheep farming. Animal. (2021) 15:100143. doi: 10.1016/j.animal.2020.100143
34. Berckmans D. Precision livestock farming (PLF). Comput Electron Agric. (2008) 62:1. doi: 10.1016/j.compag.2007.09.002
35. Beaver A, Meagher RK, von Keyserlingk MAG, Weary DM. Invited review: a systematic review of the effects of early separation on dairy cow and calf health. J Dairy Sci. (2019) 102:5784–810. doi: 10.3168/jds.2018-15603
36. Leary NWO. Invited review: cattle lameness detection with accelerometers. J Dairy Sci. (2020) 103:3895–911. doi: 10.3168/jds.2019-17123
37. Miller-Cushon EK, DeVries TJ. Technical note: validation of methodology for characterization of feeding behavior in dairy calves. J Dairy Sci. (2011) 94:6103–10. doi: 10.3168/jds.2011-4589
38. Kour H, Patison KP, Corbet NJ, Swain DL. Validation of accelerometer use to measure suckling behaviour in Northern Australian beef calves. Appl Anim Behav Sci. (2018) 202:1–6. doi: 10.1016/j.applanim.2018.01.012
39. Nogami H, Okada H, Miyamoto T. Wearable and compact wireless sensor nodes for measuring the temperature of the base of a calf's tail. Sensors Mater. (2013) 25:577–82. doi: 10.18494/SAM.2013.907
40. Scoley GE, Gordon AW, Morrison SJ. Use of thermal imaging in dairy calves: exploring the repeatability and accuracy of measures taken from different anatomical regions. Transl Anim Sci. (2019) 3:564−76. doi: 10.1093/tas/txy126
41. Bell DJ, Macrae AI, Mitchell MA, Mason CS, Jennings A, Haskell MJ. Comparison of thermal imaging and rectal temperature in the diagnosis of pyrexia in pre-weaned calves using on farm conditions. Res Vet Sci. (2020) 131:259–65. doi: 10.1016/j.rvsc.2020.05.004
42. Nir O, Parmet Y, Werner D, Adin G, Halachmi I. 3D Computer-vision system for automatically estimating heifer height and body mass. Biosyst Eng. (2018) 173:4–10. doi: 10.1016/j.biosystemseng.2017.11.014
43. Pezzuolo A, Guarino M, Sartori L, Marinello FA. Feasibility study on the use of a structured light depth-camera for three-dimensional body measurements of dairy cows in free–stall barns. Sensors. (2018) 18:673. doi: 10.3390/s18020673
44. Swartz TH, McGilliard ML, Petersson-Wolfe CS. Technical note: the use of an accelerometer for measuring step activity and lying behaviors in dairy calves. J Dairy Sci. (2016) 99:9109–13. doi: 10.3168/jds.2016-11297
45. Borderas TF, Rushen J, von Keyserlingk MA, de Passille AM. Automated measurement of changes in feeding behavior of milk-fed calves associated with illness. J Dairy Sci. (2009) 92:4549–54. doi: 10.3168/jds.2009-2109
46. Voss B, Laue H, Hoedemaker M. Field-trial evaluation of an automatic temperature measurement device placed in the reticulo-rumen of pre-weaned male calves. Livest Sci. (2016) 189:78–81. doi: 10.1016/j.livsci.2016.05.005
47. Swartz TH, Findlay AN. Short communication: automated detection of behavioral changes from respiratory disease in pre-weaned calves. J Dairy Sci. (2017) 100:9273–8. doi: 10.3168/jds.2016-12280
48. Hixson CL, Krawczel PD, Caldwell JM, Miller-Cushon EK. Behavioral changes in group-housed dairy calves infected with mannheimia haemolytica. J Dairy Sci. (2018) 101:10351–60. doi: 10.3168/jds.2018-14832
49. Shane DD, McLellan JG, White BJ, Larson RL, Amrine DE, Sanderson MW, et al. Evaluation of animal-to-animal and community contact structures determined by a real-time location system for correlation with and prediction of new bovine respiratory disease diagnoses in beef cattle during the first 28 days after feedlot entry. AJVR. (2018) 79:1277–86. doi: 10.2460/ajvr.79.12.1277
50. Toaff-Rosenstein RL, Tucker CB. The sickness response at and before clinical diagnosis of spontaneous bovine respiratory disease. Appl Anim Behav Sci. (2018) 201:85–92. doi: 10.1016/j.applanim.2018.01.002
51. Vandermeulen J, Bahr C, Johnston D, Earley B, Tullo E, Fontana I, et al. Early recognition of bovine respiratory disease in calves using automated continuous monitoring of cough sounds. Comput Electron Agric. (2016) 129:15–26. doi: 10.1016/j.compag.2016.07.014
52. Johnston D, Kenny DA, Mcgee M, Waters SM, Kelly AK, Earley B. Electronic feeding behavioural data as indicators of health status in dairy calves. Irish J Agric Food Res. (2016) 55:159–68. doi: 10.1515/ijafr-2016-0016
53. Knauer WA, Godden SM, Dietrich A, James RE. The association between daily average feeding behaviors and morbidity in automatically fed group-housed preweaned dairy calves. J Dairy Sci. (2017) 100:5642–52. doi: 10.3168/jds.2016-12372
54. Knauer WA, Godden SM, Dietrich A, Hawkins DM, James RE. Evaluation of applying statistical process control techniques to daily average feeding behaviors to detect disease in automatically fed group-housed preweaned dairy calves. J Dairy Sci. (2018) 101:8135–45. doi: 10.3168/jds.2017-13947
55. Jackson KS, Carstens GE, Tedeschi LO, Pinchak WE. Changes in feeding behavior patterns and dry matter intake before clinical symptoms associated with bovine respiratory disease in growing bulls. J Anim Sci. (2016) 94:1644–52. doi: 10.2527/jas.2015-9993
56. Studds MJ, Deikun LL, Sorter DE, Pempek JA, Proudfoot KL. Short communication: the effect of diarrhea and navel inflammation on the lying behavior of veal calves. J Dairy Sci. (2018) 101:11251–5. doi: 10.3168/jds.2018-15003
57. Kayser WC, Carstens GE, Jackson KS, Pinchak WE, Amarnath B, Fu Y. Evaluation of statistical process control procedures to monitor feeding behavior patterns and detect onset of bovine respiratory disease in growing bulls. J Anim Sci. (2019) 97:1158–70. doi: 10.1093/jas/sky486
58. Moya D, Silasi R, McAllister TA, Genswein B, Crowe T, Marti S, et al. Use of pattern recognition techniques for early detection of morbidity in receiving feedlot cattle. J Anim Sci. (2015) 93:3623–38. doi: 10.2527/jas.2015-8907
59. Toaff-Rosenstein RL, Gershwin LJ, Tucker CB. Fever, feeding, and grooming behavior around peak clinical signs in bovine respiratory disease. J Anim Sci. (2016) 94:3918–32. doi: 10.2527/jas.2016-0346
60. Hanzlicek GA, White BJ, Mosier D, Renter DG, Anderson DE. Serial evaluation of physiologic, pathological, and behavioral changes related to disease progression of experimentally induced mannheimia haemolytica pneumonia in postweaned calves. Am J Vet Res. (2010) 71:359–69. doi: 10.2460/ajvr.71.3.359
61. Wolfger B, Schwartzkopf-Genswein KS, Barkema HW, Pajor EA, Levy M, Orsel K. Feeding behavior as an early predictor of bovine respiratory disease in north american feedlot systems. J Anim Sci. (2015) 93:377–85. doi: 10.2527/jas.2013-8030
62. Oliveira Júnior BR, Silper BF, Ribas MN, Machado FS, Lima JAM, Cavalcanti LFL, et al. Short communication: tick-borne disease is associated with changes in feeding behavior in automatically fed weaned dairy calves. J Dairy Sci. (2018) 101:11256–61. doi: 10.3168/jds.2018-14637
63. Swartz TH, Schramm HH. Petersson-wolfe CS. Short communication: association between neonatal calf diarrhea and lying behaviors. Vet Anim Sci. (2020) 9:100111. doi: 10.1016/j.vas.2020.100111
64. Duthie CA, Bowen JM, Bell DJ, Miller GA, Mason C, Haskell MJ. Feeding behaviour and activity as early indicators of disease in pre-weaned dairy calves. Animal. (2021) 15:100150. doi: 10.1016/j.animal.2020.100150
65. Carpentier L, Berckmans D, Youssef A, Berckmans D, van Waterschoot T, Johnston D, et al. Automatic cough detection for bovine respiratory disease in a calf house. Biosyst Eng. (2018) 173:45–56. doi: 10.1016/j.biosystemseng.2018.06.018
66. Szyszka O, Tolkamp BJ, Edwards SA, Kyriazakis I. The effects of acute versus chronic health challenges on the behavior of beef cattle. J Anim Sci. (2012) 90:4308–18. doi: 10.2527/jas.2011-4765
67. Amrine DE, White BJ, Larson R, Anderson DE, Mosier DA, Cernicchiaro N. Precision and accuracy of clinical illness scores, compared with pulmonary consolidation scores, in holstein calves with experimentally induced mycoplasma bovis pneumonia. Am J Vet Res. (2013) 74:310–5. doi: 10.2460/ajvr.74.2.310
68. Hogeveen H, Kamphuis C, Steeneveld W, Mollenhorst H. Sensors and clinical mastitis—the quest for the perfect alert. Sensors. (2010) 10:7991–8009. doi: 10.3390/s100907991
69. Buczinski S. T LO, Dendukuri N. Bayesian estimation of the accuracy of the calf respiratory scoring chart and ultrasonography for the diagnosis of bovine respiratory disease in pre-weaned dairy calves. Prev Vet Med. (2015) 119:227–31. doi: 10.1016/j.prevetmed.2015.02.018
70. Buczinski S, Forte G, Francoz D, Belanger A-M. Comparison of thoracic auscultation, clinical score, and ultrasonography as indicators of bovine respiratory disease in preweaned dairy calves. J Vet Intern Med. (2014) 28:234–42. doi: 10.1111/jvim.12251
71. Berman J, Francoz D, Dufour S, Buczinski S. Bayesian estimation of sensitivity and specificity of systematic thoracic ultrasound exam for diagnosis of bovine respiratory disease in pre-weaned calves. Prev Vet Med. (2019) 162:38–45. doi: 10.1016/j.prevetmed.2018.10.025
72. Kayser WC, Carstens GE, Parsons IL, Washburn KE, Lawhon SD, Pinchak WE, et al. Efficacy of statistical process control procedures to identify deviations in continuously measured physiologic and behavioral variables in beef steers experimentally challenged with mannheimia haemolytica. J Anim Sci. (2020) 98:1–11. doi: 10.1093/jas/skaa009
73. Wolfger B, Timsit E, Pajor EA, Cook N, Barkema HW, Orsel K. Technical note: accuracy of an ear tag-attached accelerometer to monitor rumination and feeding behavior in feedlot cattle. J Anim Sci. (2015) 93:3164–8. doi: 10.2527/jas.2014-8802
74. Oliveira BR, Ribas MN, Machado FS, Lima JAM, Cavalcanti LFL, Chizzotti ML, et al. Validation of a system for monitoring individual feeding and drinking behaviour and intake in young cattle. Animal. (2018) 12:634–9. doi: 10.1017/S1751731117002002
75. Trénel P, Jensen MB, Decker EL, Skjøth F. Technical note: quantifying and characterizing behavior in dairy calves using the icetag automatic recording device. J Dairy Sci. (2009) 92:3397–401. doi: 10.3168/jds.2009-2040
76. Bonk S, Burfeind O, Suthar VS, Heuwieser W. Technical note: evaluation of data loggers for measuring lying behavior in dairy calves. J Dairy Sci. (2013) 96:3265–71. doi: 10.3168/jds.2012-6003
77. Finney G, Gordon A, Scoley G, Morrison SJ. Validating the icerobotics iceqube tri-axial accelerometer for measuring daily lying duration in dairy calves. Livest Sci. (2018) 214:83–7. doi: 10.1016/j.livsci.2018.05.014
78. Roland L, Schweinzer V, Kanz P, Sattlecker G, Kickinger F, Lidauer L, et al. Technical note: evaluation of a triaxial accelerometer for monitoring selected behaviors in dairy calves. J Dairy Sci. (2018) 101:10421–7. doi: 10.3168/jds.2018-14720
79. de Passillé AM, Jensen MB, Chapinal N, Rushen J. Technical note: use of accelerometers to describe gait patterns in dairy calves. J Dairy Sci. (2010) 93:3287–93. doi: 10.3168/jds.2009-2758
80. Rodriguez-baena DS, Gomez-vela FA, García-torres M, Divina F, Barranco CD, Daz-diaz N, et al. Identifying livestock behavior patterns based on accelerometer dataset. J Comput Sci. (2020) 41:10176. doi: 10.1016/j.jocs.2020.101076
81. Pillen JL, Pinedo PJ, Ives SE, Covey TL, Naikare HK, Richeson JT. Alteration of activity variables relative to clinical diagnosis of bovine respiratory disease in newly received feedlot cattle. Bovine Practitioner. (2016) 50:1–8. doi: 10.21423/bovine
82. Lowe G, Mccane B, Sutherland M, Waas J, Schaefer A, Cox N, et al. Automated collection and analysis of infrared thermograms for measuring eye and cheek temperatures in calves. Animals. (2020) 10:292. doi: 10.3390/ani10020292
83. Ferrari S, Piccinini R, Silva M, Exadaktylos V, Berckmans D, Guarino M. Cough sound description in relation to respiratory diseases in dairy calves. Prev Vet Med. (2010) 96:276–80. doi: 10.1016/j.prevetmed.2010.06.013
84. Burfeind O, Schirmann K, von Keyserlingk MAG, Veira DM, Weary DM, Heuwieser W. Technical note: evaluation of a system for monitoring rumination in heifers and calves. J Dairy Sci. (2011) 94:426–30. doi: 10.3168/jds.2010-3239
85. Rodriguez V, Minuti A, Piccioli-Cappelli F, Vailati-Riboni M, Britti D, Trevisi E, et al. Daily rumination pattern recorded by an automatic rumination-monitoring system in pre–weaned calves fed whole bulk milk and ad libitum calf starter. Livest Sci. (2018) 212:127–30. doi: 10.1016/j.livsci.2018.04.010
86. Rodrigues JPP, Pereira LGR, Neto H. do CD, Lombardi MC, Lage CF de A, Coelho SG, et al. Technical note: evaluation of an automatic system for monitoring rumination time in weaning calves. Livest Sci. (2019) 219:86–90. doi: 10.1016/j.livsci.2018.11.017
87. Toaff-rosenstein RL, Velez M, Tucker CB. Technical note: use of an automated grooming brush by heifers and potential for radiofrequency identification–based measurements of this behavior. J Dairy Sci. (2017) 100:8430–7. doi: 10.3168/jds.2017-12984
88. Lowe G, Sutherland M, Waas J, Schaefer A, Cox N, Stewart M. Infrared thermography – a non–invasive method of measuring respiration rate in calves. Animals. (2019) 9:535. doi: 10.3390/ani9080535
89. Carslake C, Jorge A V, Kaler J. Machine learning algorithms to classify and quantify multiple behaviours in dairy calves using a sensor: moving beyond classification in precision livestock. Sensors. (2021) 21:88. doi: 10.3390/s21010088
90. Guo Y, He D, Chai L. A machine vision–based method for monitoring. Animals. (2020) 10:190. doi: 10.3390/ani10020190
91. Johnson RW. The concept of sickness behavior: a brief chronological account of four key discoveries. Vet Immunol Immunopathol. (2002) 87:443–50. doi: 10.1016/S0165-2427(02)00069-7
92. Svensson C, Jensen MB. Short communication: identification of diseased calves by use of data from automatic milk feeders. J Dairy Sci. (2007) 90:994–7. doi: 10.3168/jds.S0022-0302(07)71584-9
93. Rushen J, Passillé AM De. Automated measurement of acceleration can detect effects of age, dehorning and weaning on locomotor play of calves. Appl Anim Behav Sci. (2012) 139:169–74. doi: 10.1016/j.applanim.2012.04.011
94. Luu J, Føske J, Marie A, Passillé D, Rushen J. Which measures of acceleration best estimate the duration of locomotor play by dairy calves? Appl Anim Behav Sci. (2013) 148:21–7. doi: 10.1016/j.applanim.2013.07.004
95. Größbacher V. Discriminating spontaneous locomotor play of dairy calves using accelerometers. J Dairy Sci. (2020) 103:1866–73. doi: 10.3168/jds.2019-17005
96. Weary DM, Huzzey JM, von Keyserlingk MA. Board-invited review: using behavior to predict and identify ill health in animals. J Anim Sci. (2009) 87:770–7. doi: 10.2527/jas.2008-1297
97. Cramer MC, Ollivett TL, Stanton AL. Associations of behavior-based measurements and clinical disease in preweaned, group-housed dairy calves. J Dairy Sci. (2016) 99:7434–43. doi: 10.3168/jds.2015-10207
98. Knight CH. Review: sensor techniques in ruminants: more than fitness trackers. Animal. (2020) 14:187–95. doi: 10.1017/S1751731119003276
99. Mottram T. Animal board invited review: precision livestock farming for dairy cows with a focus on oestrus detection. Animal. (2016) 10:1575–84. doi: 10.1017/S1751731115002517
100. Colditz IG, Hine BC. Resilience in farm animals: biology, management, breeding and implications for animal welfare. Anim Prod Sci. (2016) 56:1961–83. doi: 10.1071/AN15297
101. Scheffer M, Bascompte J, Brock WA, Brovkin V, Carpenter SR, Dakos V, et al. Early-warning signals for critical transitions. Nature. (2009) 461:53–9. doi: 10.1038/nature08227
102. Scheffer M. Anticipating critical transitions. Science. (2012) 338:344–8. doi: 10.1126/science.1225244
103. van Dixhoorn IDE, de Mol RM, van der Werf JTN, van Mourik S, van Reenen CG. Indicators of resilience during the transition period in dairy cows: a case study. J Dairy Sci. (2018) 101:10271–82. doi: 10.3168/jds.2018-14779
Keywords: calf, early disease detection, precision livestock farming, sensor, health monitoring
Citation: Sun D, Webb L, van der Tol PPJ and van Reenen K (2021) A Systematic Review of Automatic Health Monitoring in Calves: Glimpsing the Future From Current Practice. Front. Vet. Sci. 8:761468. doi: 10.3389/fvets.2021.761468
Received: 19 August 2021; Accepted: 04 November 2021;
Published: 26 November 2021.
Edited by:
Marcia Endres, University of Minnesota Twin Cities, United StatesReviewed by:
Joao H. C. Costa, University of Kentucky, United StatesGillian Scoley, Queen's University Belfast, United Kingdom
Robert Elliott James, Virginia Tech, United States
Copyright © 2021 Sun, Webb, van der Tol and van Reenen. This is an open-access article distributed under the terms of the Creative Commons Attribution License (CC BY). The use, distribution or reproduction in other forums is permitted, provided the original author(s) and the copyright owner(s) are credited and that the original publication in this journal is cited, in accordance with accepted academic practice. No use, distribution or reproduction is permitted which does not comply with these terms.
*Correspondence: Dengsheng Sun, ZGVuZ3NoZW5nLnN1bkB3dXIubmw=