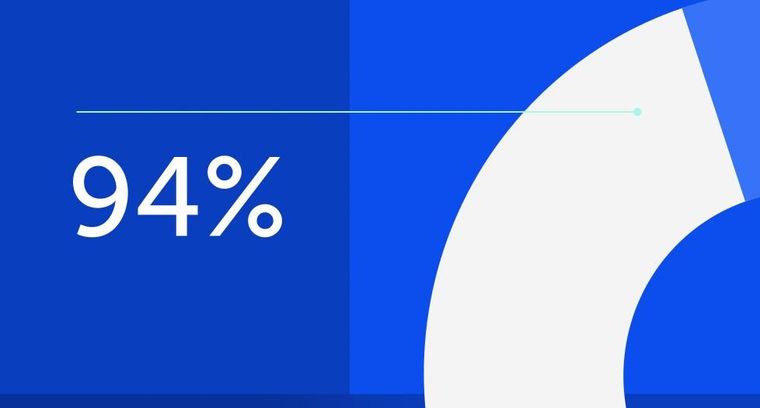
94% of researchers rate our articles as excellent or good
Learn more about the work of our research integrity team to safeguard the quality of each article we publish.
Find out more
ORIGINAL RESEARCH article
Front. Vet. Sci., 21 July 2021
Sec. Animal Behavior and Welfare
Volume 8 - 2021 | https://doi.org/10.3389/fvets.2021.693290
Genome-wide association study (GWAS) using dog breed standard values as phenotypic measurements is an efficient way to identify genes associated with morphological and behavioral traits. As a result of strong human purposeful selections, several specialized behavioral traits such as herding and hunting have been formed in different modern dog breeds. However, genetic analyses on this topic are rather limited due to the accurate phenotyping difficulty for these complex behavioral traits. Here, 268 dog whole-genome sequences from 130 modern breeds were used to investigate candidate genes underlying dog herding, predation, temperament, and trainability by GWAS. Behavioral phenotypes were obtained from the American Kennel Club based on dog breed standard descriptions or groups (conventional categorization of dog historical roles). The GWAS results of herding behavior (without body size as a covariate) revealed 44 significantly associated sites within five chromosomes. Significantly associated sites on CFA7, 9, 10, and 20 were located either in or near neuropathological or neuronal genes including THOC1, ASIC2, MSRB3, LLPH, RFX8, and CHL1. MSRB3 and CHL1 genes were reported to be associated with dog fear. Since herding is a restricted hunting behavior by removing killing instinct, 36 hounds and 55 herding dogs were used to analyze predation behavior. Three neuronal-related genes (JAK2, MEIS1, and LRRTM4) were revealed as candidates for predation behavior. The significantly associated variant of temperament GWAS was located within ACSS3 gene. The highest associated variant in trainability GWAS is located on CFA22, with no variants detected above the Bonferroni threshold. Since dog behaviors are correlated with body size, we next incorporate body mass as covariates into GWAS; and significant signals around THOC1, MSRB3, LLPH, RFX8, CHL1, LRRTM4, and ACSS3 genes were still detected for dog herding, predation, and temperament behaviors. In humans, these candidate genes are either involved in nervous system development or associated with mental disorders. In conclusion, our results imply that these neuronal or psychiatric genes might be involved in biological processes underlying dog herding, predation, and temperament behavioral traits.
Dogs are man's best friend and the first domesticated animal, originating from a now-extinct wolf population. Dogs have shared living space and food sources with humans and have maintained this close relationship for more than 11,000 years (1). For only 200–300 years, humans have selectively bred dogs for excellence in herding, hunting, and obedience and have created diverse breeds with a wealth of behaviors. At the same time, humans have also bred dogs for different morphological traits such as body types, sizes, skull shapes, coat colors, and textures according to human preferences and needs. Two major bottlenecks in dog history, i.e., early domestication and the creation of modern breeds, have characterized long-range linkage disequilibrium (LD) within dog breeds, providing an excellent natural model for studying morphology, complex diseases, and behaviors (2). Over the past two decades, scientists have attempted to explain the genetic basis of phenotypic variation among dog breeds. Many cross-breed researches were performed including morphologic traits (3–5), diseases (6), behavior or cognition (6–8), and athletic ability (9).
Dog behavior traits have been reported to be highly heritable, with a mean among-breed heritability (h2) of 0.51 ± 0.12 (standard deviation) for 14 behavioral traits. Specifically, high h2 values were observed for attachment and attention-seeking (0.56), chasing (0.62), stranger-directed aggression (0.68), and trainability (0.73) (7). However, the genetic mapping of behavior among dog breeds remains challenging. One reason is that behavior and cognition are complex traits, which are difficult to define and measure accurately (10). Therefore, different methods have been developed to classify and describe behavioral phenotypes. Behavioral studies across and within dog breeds have been explored and discussed. With the use of large single-nucleotide polymorphism (SNP) datasets and C-BARQ data of diverse breeds, dog fearlessness and aggression traits have been mapped to be associated with GNAT3-CD36 (CFA18) and IGSF1 (CFAX) loci (8). In the same study, variants within body size genes (IGF1 and HMGA2) showed significant associations with dog behaviors such as dog rivalry, separation anxiety, touch sensitivity, and owner-directed aggression (8). In one recent study, using breed-averaged C-BARQ data as phenotypes, 131 SNPs were demonstrated to be significantly associated with dog behavioral differences among 101 breeds, and the identified neurological candidate genes were highly expressed in the brain (7). In addition, the among-breed heritability of 14 behavioral traits was significantly higher than the heritability assessed in large within-breed samples (7). This study only did genome-wide association study (GWAS) considering body size covariates, and loci that affect both body size and behavior might have been missed. Another GWAS of dog cognition (with and without body mass factors) using breed-averaged phenotypic values identified five SNPs significantly associated with breed differences in dog communication, memory, inhibitory control, and physical reasoning and identified 188 genes related to breed cognitive differences (6).
Behavioral traits often exhibit complexity, polygenic control, and susceptibility to environmental influences. And they are inherited in linkage with other traits; for example, behavioral traits in dog are related to body size (11). For some behavioral studies, within-breed studies have shown good results and have been able to obtain more specific behavioral or cognitive loci. Recently, using the C-BARQ data as phenotypes, 11 SNPs within eight genomic regions were detected to be significantly related with six canine personality traits in Labrador retrievers (12). Two chromosome regions of CFA7:75–79 Mb and CFA20:8–11 Mb were investigated to be significantly associated with fearfulness in German Shepherd (13). Meanwhile, a locus of CFA11:12.8 Mb was found to be significantly associated with fearfulness when investigated in Great Dane (14). These regions and the contained genes all correspond to the neuropsychiatric or neuronal gene regions in humans. In addition, human obsessive-compulsive disorder (OCD) has phenotypes similar to those in canine compulsive disorder (CCD), such as repetitive and time-consuming behaviors (15). Four CCD candidate genes, i.e., CDH2, CTNNA2, ATXN1, and PGCP, were mapped by case–control GWAS in Doberman pinschers and validated in high-risk breeds (16). Structural variants on CFA6 containing GTF2I and GTF2IRD1 genes could contribute to behavioral differences (extreme sociability) between dogs and wolves, and these two genes are associated with human Williams–Beuren syndrome, which is characterized by a happy and friendly disposition (17). Notably, HS6ST2 gene was first reported to be associated with dog sociability behavior (8) and recently was detected to be significantly related to human neuroticism in GWAS of 405,274 UK Biobank samples (18). This indicates that dogs could be good natural models for studying the molecular etiology of human neural disorders.
Herding dogs were bred to help people manage livestock, and they excel at controlling livestock movement. Herding derives from predatory behavior by amplifying some predatory instincts such as eye staring, stalking, and chasing while suppressing other instincts as crush, bite, or kill the prey (19). Herding dogs are energetic, enthusiastic, and eager to work. If they are not properly trained or assigned tasks, they even use the inclination to herd other creatures including human beings (20). They also exhibit characteristics such as agility, bravery, steadiness, and relatively low aggressiveness (21). The current study used breed-specific behaviors and groupings from the American Kennel Club (AKC), the most authoritative organization for the registration and classification of purebred dogs in the United States. The AKC recognizes and classifies 197 modern purebred dog breeds into seven loosely defined groups based on their breed features (heritage, physical attributes, and behavior) and historical roles: sporting, hound, working, terrier, toy, non-sporting, and herding groups (22). The AKC group method has been successfully applied in identifications of genetic factors contributing to athleticism in sporting and hound dogs (9) as well as relationship investigations between artificial selection and human-directed play behavior (23). Genetic mapping of dog herding behavior has been first studied as qualitative variable in 148 dog breeds (24); and three other dog behaviors including pointing, boldness, and trainability were studied using cross-breed mapping.
Different dog breed specific traits are selected based on different human purposes; thus, each dog breed has its unique temperament and trainability characteristic. Temperament is of great importance for dog breeding, especially in choosing good guide dogs (25). Pet owners are also interested in matching dog with suitable temperament (26). Among the genetic studies of temperament traits, dog activity-impulsivity endophenotype was first studied through the association analysis of candidate gene DRD4 (27). Trainability levels were detected to have significant differences between seven breed groups (conventional breed categories), which implies that dog behavior traits such as trainability and boldness are partly caused by original function of breed. In the same study, scores of trainability, boldness, calmness, and dog sociability were all detected with significant differences among dog breeds (28). These breed-level behavioral differences can be used as phenotypes to study underlying genetic mechanisms, which will help us understand how these behaviors developed in dogs.
Significant brain neuroanatomical variations among breeds with different behavioral specialties, such as herding, hunting, guarding, and companionship, are likely due to human selection for the behavior (29). It is reasonable to hypothesize that using a cross-breed research strategy could help us find loci that control significant behavioral variations between breeds. Therefore, this study used behavioral groupings provided by the AKC to perform cross-breed GWAS to find genetic markers associated with behavioral differences among breeds. Incorporating body size factors into dog behavior GWAS can bring both merits and drawbacks, as body size-related variants could also play roles in behaviors through their effects on brain architectures (30), while controlling body size factors could reveal genetic variants that are not explained by the brain or body size (7). Inspired by Gnanadesikan et al. (6), significant signals identified in GWASs either with or without body mass corrections were regarded as candidates in our analysis. This study provides clues to the molecular genetic mechanisms underlying canine behaviors such as herding, predation, temperament, and trainability. Understanding the formation of breed-specific behaviors in dogs will also pave the way for further elucidations of mechanisms underlying human neuropsychiatric disorders.
All 268 whole-genome sequences of dogs that were used in this study have been extracted from vcf file data of 722 canine individuals (https://www.ncbi.nlm.nih.gov/bioproject/PRJNA448733), which is deposited by Dr. Elaine A. Ostrander group of the National Institutes of Health (NIH) (5). Many sources (n = 128) such as NIH Intramural Sequencing Center are involved in the data generation with funds such as Intramural Program of the National Human Genome Research Institute. The 268 dog genomes consist of 130 established dog breeds (Supplementary Table 1), and the selection criterion is same as described in (5). For herding behavior, dogs were divided into cases and controls according to whether they belong to the AKC herding group (conventional categorization) (https://www.akc.org/dog-breeds/herding/) or not. Forty-three herding group dogs were obtained, containing 15 modern dog breeds (Supplementary Table 1). In addition, an extra six modern dog breeds with a herding phenotype (Rottweiler, Bernese Mountain Dog, Fonni's Dog, Lapponian Herder, Samoyed, and Swedish Lapphund) were selected according to the article (24), although these breeds were classified to working group in the AKC. Twelve cases were obtained in this step, and finally 55 herding dogs were available as a case group. Herding dogs and hunting dogs are selected to meet different job requirements, and therefore, they have different degrees of prey-driven instincts. Thus, hounds and herding dogs can serve as good cases and controls for studying hunting behavior, such as aggressive behavior. In order to decipher this complex behavior, 36 hound group dogs were set as cases and 55 herding group dogs were regarded as controls for GWAS. Temperament and trainability traits were referred to the average scores of the AKC breed standard. When the ideal physical characteristics and temperament of a dog breed are specified in a written document, the breed becomes the standard breed. Therefore, different dog breeds have different levels of temperament (outgoing, friendly, alert/responsive, reserved with strangers, and aloof/wary) (Figure 1A) and trainability (eager to please, easy training, agreeable, independent, and may be stubborn) (Figure 1B). Since kennel club group classifications are not the most accurate way to apply those phenotypes, we only set the top two levels as cases and last two levels as controls, and the middle levels (agreeable and alert/responsive) were not included in GWAS analysis and considered as missing (NA). In total, 105 cases and 81 controls for the temperament analysis and 98 cases and 85 controls for trainability analysis were finally obtained (Table 1). Phenotype information of dog breed temperament and trainability traits were collected on 20 December 2020.
Figure 1. Classifications of trainability and temperament traits among modern dog breeds. Trait level information is obtained from AKC website (https://www.akc.org/dog-breeds/) (accessed on 20 December 2020), and each breed has a specific score for one of these five phenotype levels. (A) Aloof/wary, reserved with strangers, alert/responsive, friendly and outgoing were used to describe temperament character of each dog breed stereotype. (B) May be stubborn, independent, agreeable, easy training and eager to please were applied to describe trainability character of each dog breed stereotype.
Table 1. Summary of dog behavioral phenotypes used in genome-wide association study (GWAS) analysis.
To obtain high-quality and only biallelic variants [single-nucleotide variants (SNVs) and small indels] for GWAS, vcf file of 722 dog genomes was first filtered by PLINK 1.90 with the following functions: –max-alleles 2, –min-alleles 2, –minQ 20, –max-missing 0.9 (31). Then individual dogs for each GWAS were extracted from the above-filtered vcf file, and variants with missing value >1% (–maf 0.01) were removed using PLINK 1.90 (31). After filtering, 14,489,548, 14,654,804, 14,984,476, and 14,853,066 biallelic variants were used for GWASs of herding, predation, temperament, and trainability traits, respectively.
GWAS was conducted by applying a univariate linear mixed model with sex and kinship (relatedness matrix) as covariates. The model is available in GEMMA 0.98, and two steps of calculation were applied (32). A centered relatedness matrix was calculated in the first step, which was used as a covariate to adjust for sample structure after eigen-decomposition in the second step (32). The Wald test was applied for the association significance assessment. Bonferroni thresholds [Pbon = –log(0.05/number of analyzed variants)] were used to identify significant association sites for herding (Pbon = 8.46) and predation (Pbon = 8.47) behavior. As no associated variants were above Bonferroni thresholds for temperament and trainability, suggestive thresholds [Psug = –log(1/number of analyzed variants)] of temperament (Psug = 7.18) and trainability (Psug = 7.17) were applied. The suggestive threshold was first introduced by Lander and Kruglyak (33), which represents one false positive that is expected per genome scan under the null hypothesis. Manhattan and quantile–quantile (QQ) plots were generated by qqman package (34). To account for body size factors in dog behavior, dog standard breed weight (SBW) and height (SBH) were further included in GWASs as covariates. The average values of body size were collected from (5). Only dog breeds that have SBW and SBH values were chosen for further analysis. The variant filtering conditions are the same as above. After filtering, 255 individuals with 14,416,697 variants, 88 dogs with 14,542,561 variants, 178 dogs with 14,829,902 variants, and 177 dogs with 14,726,409 variants were analyzed in herding (Pbon = 8.46, Psug = 7.16), predation (Pbon = 8.46, Psug = 7.16), temperament (Pbon = 8.47, Psug = 7.17), and trainability (Pbon = 8.47, Psug = 7.17) GWASs, respectively. Bonferroni and suggestive thresholds were shown in the figures of GWAS results.
The genomic inflation factor lambda (λ) was calculated with the following formula: λ = median (qchisq(1 – p, 1))/qchisq(0.5, 1) where p is a vector of p-values in GWAS results. The lambda inflation factor indicates the rate of excess false positive and the extent of the bulk inflation. When values of λ <1.1 are obtained, significant population stratification will not be considered, which was also observed in the GWAS of canine complex traits (35). The QQ plot shows the observed vs. expected –log p-values. The straight line in the QQ plot indicates the distribution of variant markers under the null hypothesis, and the skew at the right edge indicates those markers that are more strongly associated with the trait than would be expected by chance.
The detected associated signals were annotated by National Center for Biotechnology Information (NCBI) Canis lupus familiaris 3.1 Annotation Release 105. The positions were viewed by Genome Data Viewer with CanFam3.1 reference genome (https://www.ncbi.nlm.nih.gov/genome/gdv/?org=canis-lupus-familiaris).
The allele frequencies of significantly associated sites were investigated in cases and controls for each GWAS trait setting separately using VCFtools 0.1.16 (36). The results of altered allele frequencies within these traits are shown in Table 2.
Table 2. Genome-wide association study (GWAS) significant associated variants of dog herding, predation, temperament, and trainability behavior traits.
LD of each significantly associated site was analyzed by PLINK 1.90 (31) using the following functions: –ld-window-kb 5,000, –ld-window 99,999, –ld-window-r2 0.8. Sites with r2-value more than 0.8 are listed in Supplementary Table 2. Genes near or located around these LD sites were annotated by Genome Data Viewer.
We next analyzed variants that were only present in 55 dogs with herding behavior. First, a total of 268 samples were quality controlled for all types of variants using VCFtools 0.1.16 (36). Only variants with minor allele frequency (MAF) >0.05, genotype quality (GQ) score > 20, and mean depth values >10x were selected. After separate filtering, 10,415,191 variants of 213 control dogs and 9,864,535 variants of 55 herding behavior dogs remained for further analysis. Private variants were analyzed by comparing the above-filtered vcf files of 55 herding dogs and 213 controls using “–diff-site” function in VCFtools 0.1.16 (36). The private variants were further annotated by SnpEff 5.0 with Ensembl genome 101 release (37). We have acquired 987,046 sites that were absent or rare (MAF <0.05) in non-herding controls, and these variants were present in at least one herding dog. Variants within protein-coding genes were selected for further analysis. Variants with possible functions (high and moderate impact) in protein-coding genes were chosen, and 611 high impact variants within 270 genes and 6,740 moderate-impact variants within 2,133 genes were left. After merging genes of high and moderate impacts, 2,287 private genes remained. Gene Ontology (GO) analysis was performed using these 2,287 genes with the online software WebGestalt [http://www.webgestalt.org/; (38)]. The top 10 significant biological processes and cellular components were chosen for further analysis; WebGestalt applied the false discovery rate (FDR) method to account for multiple testing.
The variant filtered quality conditions such as MAF and mean depth values could influence the variant content of filtered vcf files of cases and controls. For example, one variant has a MAF of 0.049 in 213 controls, while its MAF is 0.051 in 55 cases; then it will be one private variant because it is absent in quality-filtered vcf file of 213 controls due to MAF <0.05. To prioritize the private candidate variants, in these possibly functional private variants, their altered allele frequencies were further checked in raw vcf files of 55 herding and 213 control dogs separately using “–freq” function in VCFtools 0.1.16 (36). Variants present in more than one herding dog but not in controls, or variants with altered allele frequency differences >0.1 between cases and controls, are listed (Supplementary Table 3).
Gene expressions of 10 candidate genes (THOC1, ASIC2, MSRB3, LLPH, RFX8, CHL1, JAK2, MEIS1, LRRTM4, and ACSS3) were further examined by online database SCDevDB (https://scdevdb.deepomics.org) for single-cell atlas in the human neural developmental pathway (39). The cell types were oocyte, zygote, 2-cell, 4-cell, 8-cell, 16-cell, blastocyst, human embryonic stem cells (hESC), H1_24_wells, H1_96_wells, neural_D12 (neural cells generate from H1 cell line, 12 days after differentiation), neural_D26 (neural cells generate from H1 cell line, 26 days after differentiation), neural_D54 (neural cells generate from H1 cell line, 54 days after differentiation), and neural_D80 (neural cells generate from H1 cell line, 80 days after differentiation). Cell details are available at https://scdevdb.deepomics.org/data-summary/; data information of neural cell lines was referenced in (40).
These genes were further investigated in Allen Developing Mouse Brain Atlas [http://developingmouse.brain-map.org; (41)]. Days of embryonic (E) specimen age and postnatal (P) specimen age, which is relative to birth (P0), are used to define the mouse brain development stages.
We investigated four dog behavioral trait phenotypes (herding, predation, temperament, and trainability) using a univariate linear mixed model incorporating in GEMMA 0.98 (32). Sex and relatedness matrices (correcting for population stratification) were used as covariates to perform association tests on one single trait phenotype. For GWAS of dog herding behavior, 55 dogs with herding behavior and 213 control dogs were used. Forty-four significantly associated variants within regions of five chromosomes (CFA6, CFA7, CFA9, CFA10, and CFA20) were above the Bonferroni threshold (Figure 2). The most significantly associated region is located on CFA20 (16594598–16610335) including 16 associated sites, all of which were near or in one long non-coding RNA (lncRNA): LOC111091431. Five of them were within LOC111091431, and one variant at position 16,607,008 was located in the exonic region of the lncRNA. Moreover, this variant (CFA20:16607008 A>T) was only present in dogs with herding behavior (Table 2). Another variant (CFA20:16603809 A>C) was located only 164 bp upstream of LOC111091431. More importantly, LOC111091431 is located 159,124 bp upstream of the neural cell adhesion molecule L1-like protein (CHL1), a neural-associated gene. On CFA7, one variant was 594 bp upstream of THO Complex 1 (THOC1) gene. One, two, and eight significantly associated intron variants were detected in acid sensing ion channel subunit 2 (ASIC2), methionine sulfoxide reductase B3 (MSRB3), and regulatory factor X8 (RFX8) genes, respectively, on chromosomes 9 and 10 (Table 2). Genes such as MSRB3 (42), THOC1 (43), ASIC2 (44), and RFX8 (45) are reported to have either neuropathological or neuronal functions. These genes near significantly associated variants are indicated in Figure 2A. Other loci were located in genes that are not functionally annotated or were located in intergenic regions and away from genes. For instance, two associated regions on CFA10 were located around 8.1 and 8.6 Mb, and the closest genes in these regions were long-term synaptic facilitation protein (LLPH) and LOC111097584.
Figure 2. Manhattan plots of herding behavior genome-wide association study (GWAS). The plots show the –log10 p-values for all variants of GWAS. Red horizontal line represents the Bonferroni genome-wide significance threshold, and blue horizontal line indicates suggestive significance threshold. (A) Without including body size as a covariate. Candidate genes around significantly associated sites (above the Bonferroni threshold) were marked with red color in the Manhattan plot; they are THOC1 of CFA7; ASIC2 of CFA9; MSRB3, LLPH, and RFX8 of CFA10; and CHL1 of CFA20. (B) Including body size as a covariate. Candidate genes (THOC1, MSRB3, LLPH, RFX8, and CHL1) were marked in the plot.
Prey drive is the innate behavioral pattern of carnivores to pursue and capture prey, and it is a fundamental characteristic of herding dogs. Through selective breeding, humans have been able to reduce prey-driven behavior of herding dogs while maintaining their hunting skills (46). Therefore, we investigated the genetic difference between herding and hunting dogs. Thirty-six hound group dogs and 55 herding group dogs were selected to study the predation differences between these two groups. This may provide further understanding of formation of herding behavior. Three chromosome regions on CFA1, 10, and 17 showed significant signals (Figure 3A). Three genes nearest to these regions were janus kinase 2 (JAK2) (about 1 kb), meis homeobox 1 (MEIS1) (around 26 kb), and leucine-rich repeat transmembrane neuronal 4 (LRRTM4) (~313 kb) (Table 2 and Figure 3A).
Figure 3. Genome-wide association study (GWAS) of dog predation analysis between hound and herding group dogs. Manhattan plots demonstrate the p-value distribution across all chromosomes. The Bonferroni and suggestive GWAS significance thresholds are indicated with the red and blue horizontal lines, respectively. (A) Without including body size as a covariate. JAK2, MEIS1, and LRRTM4 were the genes nearest to the significantly associated regions of CFA1, CFA10, and CFA17. (B) Including body size as a covariate. Significantly associated region of CFA17 remained after incorporating body size covariates into GWAS.
To clarify potential genes that are associated with dog temperament and trainability traits, phenotypes based on breed-averaged measures were grouped as described on the AKC website (https://www.akc.org). The phenotypes were classified into five levels (Figure 1). The GWAS for dog temperament trait was based on 105 dogs of extraversion type and 81 dogs of aloof type. There was only one variant above the suggestive threshold located in the intron region of Acyl-CoA synthetase short chain family member 3 (ACSS3) gene on CFA15 (Table 2 and Figure 4A). For trainability GWAS, 98 high and 85 low trainability-level dogs were selected for analysis, and only one variant was detected near LOC111091672 with a suggestive significant association for trainability (Table 2).
Figure 4. Temperament genome-wide association study (GWAS) reveals an intron variant (CFA15:23,340,008 A>T) of ACSS3 gene. Manhattan plots showing the association of whole genome variants with temperament levels in dogs. Bonferroni and suggestive thresholds are indicated with red and blue lines. (A) Without including body size as a covariate. The ACSS3 intron variant is above the suggestive threshold. (B) Including body size as a covariate. The ACSS3 intron variant is above the Bonferroni threshold.
As body size has been reported to be related with dog behaviors, we then performed GWAS adding body size values into covariates. As shown in Figures 2–5, similar results were observed after incorporating SBW and SBH into analysis for herding, predation, temperament, and trainability. In the new herding GWAS, the significantly associated chromosome regions were similar to the results without body size covariates, except for the associated site on CFA9. Although the p-values (p = 4.03E−09) for the CFA9 variants (CFA9:40067785 and CFA9:40068138) increased, they were still close to the Bonferroni threshold (Figure 2B). Bonferroni significantly associated signals of dog herding behavior around candidate genes like THOC1, MSRB3, LLPH, RFX8, and CHL1 (Figure 2B). For the predation GWAS analysis, only the region of CFA17 remained significantly associated after incorporating body mass covariates into analysis, while variants near MEIS1 gene on CFA10 were above the suggestive threshold (Figure 3B). In the new temperament GWAS analysis, the same variant of ACSS3 showed a smaller p-value (1.92E−09) above the Bonferroni threshold (Figure 4B). No significant association was found for trainability after adding body size factors. GWAS QQ plots can be referred to Supplementary Figure 1.
Figure 5. One variant is above the suggestive threshold of dog trainability genome-wide association study (GWAS). Manhattan plots showing the association of whole genome variants with trainability levels in dogs. Bonferroni and suggestive thresholds are indicated with red and blue lines. (A) Without including body size as a covariate. One variant on CFA15 slightly passed the suggestive threshold. (B) Including body size as a covariate. No variants were detected above the suggestive threshold.
Causative variants are usually not directly detected by GWAS, and phenotypically based causal variants may be in LD with GWAS-related markers. LD of each GWAS significant association site for herding behavior was calculated by Plink 1.90 (31). The results are shown in Supplementary Table 2. Only sites with r2 > 0.8 were chosen for further analysis. Except variants that already exceeded the significant threshold, six other variants within genes are shown in Table 3. A variant on CFA6 (CFA6:39,977,184 G>A) was located in the intron region of PIGQ gene, which has been reported to be the causative gene for human early-onset epilepsy (47). One missense variant (NC_006592.3:g.8037693G>A, XP_013972688.1:p.Gly179Ser) was detected in MSRB3 gene, and the other four variants were located in exon regions of one lncRNA (LOC111097584) near MSRB3 (38 kb downstream). These five potentially functional variants may promote the development of dog herding behavior through directly or indirectly affecting the functions of MSRB3 and LOC111097584. Ten species were chosen to analyze the MSRB3 missense variant conservation. Six mammals have amino acid D; and three species including dogs, chickens, and chimpanzees have G in this position (Supplementary Figure 2). This indicated that the missense variant is not conserved.
Table 3. Interesting linkage disequilibrium (LD) sites of herding genome-wide association study (GWAS) significant variants with r2 > 0.8.
Private functional variants that were only present in herding dogs could contribute to the herding behavior trait formation. Therefore, we analyzed the private functional genes of herding dogs in an attempt to find candidate genes. To obtain variants that were only present in 55 dogs with herding behavior, high-quality variants of 55 herding and 213 control dogs were separately filtered. After different sites were compared between cases and controls, the variants that only existed in 55 herding dogs were annotated by SnpEff 5.0 software (37). The remaining 7,351 private (611 high impact and 6,740 moderate) variants were chosen for further analysis, and these private variants were in 2,287 protein-coding genes (Supplementary Table 3). Considering that functional variants can impact gene function, these 2,287 genes were used in the GO analysis. Among the top 10 significantly enriched biological processes, 112 genes were enriched in nervous system development process (GO:0007399), and 54 genes were in neuron projection development process (GO:0031175) (Table 4). Moreover, 76 genes were enriched in neuron part (GO:0097458) within cellular component analysis. Details of the gene names and private functional variants are listed in Supplementary Table 3.
As these genes are related to nervous system or human mental disorders, 10 candidate genes, i.e., THOC1, ASIC2, LLPH, RFX8, MSRB3, CHL1, JAK2, MEIS1, LRRTM4, and ACSS3, were used for further analysis in a single-cell expression database of human neural developmental. Except ASIC2, nine candidate genes were detected to be highly expressed in different early development stages of neural cells, which were generated after 12, 26, 54, and 80 days' differentiation (Supplementary Figure 3). It is noted that RFX8 gene showed unique high expression in neural cells of 12 days.
After these 10 genes were checked in Allen Developing Mouse Brain Atlas, three genes including ASIC2, CHL1, and MEIS1 showed high expressions in mouse brain development stages (E11.5, E13.5, E15.5, E18.5, P4, P14, and P28). This suggests that ASIC2 is also a neurodevelopmental gene.
Research on the genetic mechanism of dog behaviors can help us understand dog domestication process and guide us on how to get along with dogs, which is important for dog welfare. Moreover, it could also provide clues to research of human behavior and health disorders. Dog genomes have undergone strong artificial selection with increased haplotype homozygosity and LD (2). Therefore, compared with human studies, GWAS with smaller dog samples can even produce good results (2, 15). For example, GWAS with whole-genome sequences across diverse breeds has proved to be a powerful method to study canine morphological traits (5). Here, we used genomic data from 268 modern dogs to perform GWAS for four behaviors and tried to find the genetic clues behind these phenotypes. In this study, phenotypes were based on dog breed standard values or group information from the AKC, which is valid for revealing genomic regions and variants for several specific phenotypes such as dog fear, aggression, boldness, cognition, and athleticism (4, 6, 7, 9, 24). Some dog behaviors have been reported to be highly heritable and higher than those assessed within breeds, and it is hypothesized that specific loci associated with behavioral differences between breeds can be found using across-breed genome-wide approach (7). Previous behavior or cognition GWASs were all performed with SNP chip data (≤173 K), whereas we used nearly 15 M variants of 130 dog breeds in this study, which were obtained by whole genome resequencing. It has higher coverage of non-coding regions of the dog genome, which have important roles in dog behavioral traits such as differentiating dog from wolf (48). In this study, several promising candidate genes with neuronal or psychiatric were detected to be associated with breed differences of herding, predation, temperament, and trainability traits.
Herding is a complex behavioral trait that requires dogs to be fearless and bold when facing large numbers of sheep or cattle. The genome-wide significant loci of fearless were mapped on CFA7:75–79 Mb and CFA20:8–11 Mb (13), and those of boldness were discovered on CFA10:6.8–8.8 Mb (4). In our herding GWAS results, nearby genomic regions of 67.1 Mb on CFA7 and 16.6 Mb on CFA20 were detected to be significantly associated (Table 2). Furthermore, two regions of 8–8.1 and 8.6 Mb on CFA10 were also significantly related. These regions were either near or in the regions that were reported with dog behaviors before. The area of CFA10:8–8.6 Mb has been found to be associated with at least two morphological (ear type and body size) (3, 5, 24) and two behavioral (boldness and fear) traits (4, 7), including genes such as MSRB3 and HMGA2. MSRB3 has been reported to be associated with human deafness (42, 49), brain morphology, and late-onset Alzheimer's disease (50). It is also involved in stress resistance in Drosophila (51). Furthermore, according to GWAS Catalog database (https://www.ebi.ac.uk/gwas/genes/MSRB3), MSRB3 was detected to be significantly associated with brain area volumes with the largest number of associations among the 31 reported traits. These reports suggest that MSRB3 gene plays multiple roles in the nervous system. Except MSRB3 region, we also identified a fragment downstream of HMGA2, which is closer to the LLPH gene. It has been reported that LLPH is involved in regulating neuronal development and synaptic transmission (52). Ear shape and body mass are two common targets of selection in domestic breeding, and selective breeding for specific traits in dogs may result in this region being selected. Also, body size was investigated to be correlated with dog behaviors (11), which were also observed in several genome-wide mapping of dog behaviors (4, 8, 24). One plausible explanation for these associations could be pleiotropy of these regions, which implies that genetic variants could affect both behavior and morphology traits in dogs. Alternatively, morphological and behavioral traits may have been co-selected due to genetic linkage (53).
We also localized another region on CFA10 (41.5 Mb) that was associated with herding behavior, which covered exons 9 and 10 of the RFX8 gene (Table 2). This region is ~1.99 Mb apart from the top significantly associated site (CFA10:43493767) of dog rivalry behavior (7). It was suggested that RFX8 could play roles in Schwann cell proliferation, as it was detected to be most prominently expressed in the schwannoma cell line (45). Schwann cells are important for the nervous system, as they direct the regeneration of peripheral axons (54). Meanwhile, RFX8 has been identified as a candidate gene underlying human neurodevelopmental disorders (55). A significantly associated region on CFA20 covered the uncharacterized lncRNA-LOC111091431, the closest to which is a neural-associated gene, CHL1. LncRNAs are thought to be commonly but not absolutely involved in transcriptional regulation of nearby genes and often function as cis in enhancer activity (56). Thus, it is assumed that LOC111091431 may influence the formation of behavior through unknown interactions with CHL1, but its exact function remains to be verified. It was reported that CHL1 could promote neurite outgrowth (57) and regulate cell migration during nerve regeneration (58). It is suspected that CHL1 is also associated with intelligence (59); this could be an explanation of the higher learning ability of herding dogs. Meanwhile, CHL1 was detected to be significantly associated with dog fear (7) and human 3p syndrome mental impairment (60). Mice with CHL1 deficiency demonstrated exploratory behavior changes in novel environments (61) and affected several behavioral parameters such as emotional reactivity (stress) and motor coordination (62). It was also supposed that CHL1 could participate in nervous system development and signal transduction by regulating synaptic vesicle recycling (63).
In addition to requirements of courage, herding dogs have hunting instincts such as chasing. They are CCD-like behavioral traits that are manifested by dogs using pacing and circling to maintain and control the herd. Some CCD behaviors derive from predatory behavior, like tail chasing and fly snapping (15). The same study reported a strongly associated region of CCDs between 61.83 and 63.87 Mb on CFA7, including CDH2 gene (15, 16). It is noted in our findings that the region significantly associated with herding was localized between 67.13 and 67.16 Mb on CFA7, ~3.26 Mb from the above-mentioned CCD interval. In addition, a significantly related variant CFA7:67137186 T>G was only 594 bp upstream of THOC1 gene. However, the abovementioned variant is located within 27 Ts in a row, which suggests that it is unlikely to be regulatory. It is noted that THOC1 gene is involved in presynaptic development and plays roles in dopamine neuron survival (43). It is also one causative gene for human late-onset hearing loss (64). Herding dogs have been selectively bred to detect and react to slight differences in whistle commands from a long distance of nearly 1 km, and excellent hearing ability is necessary for herding tasks (65). Therefore, genes that are essential for auditory functions such as MSRB3 and THOC1 were detected in our herding GWAS analysis.
Significantly associated regions of herding GWAS were also mapped on CFA9 containing ASIC2 gene. ASIC2 was reported to play roles in hippocampal neurons (44) and innate fear-like behaviors in mice (66). GO analysis revealed that ASIC2 was detected in multiple neural cell components (Table 4). ASIC2 was also detected among private genes of herding dog (Supplementary Table 3). ASIC2 was detected in high expressions in mouse brain development processes. However, significant signals were absent in the GWAS analysis including body mass factors (Figure 2B). Though gene functions of LOC611691 and OR28H03 detected on CFA6 were not related with neural function, one high LD site with the associated variant was located within PIGQ gene (Table 3). It has been reported that PIGQ is associated with the neurologic disorder of severe early-onset epilepsy (47). Overall, genes MSRB3, LLPH, RFX8, CHL1, THOC1, and ASIC2 are our top candidates based on herding GWAS and likely functional variation in behavioral genes.
Hunting dogs exhibit higher prey-driven behavior in orientation, chasing, grab-bite, and kill-bite (67). They usually show more excitement and aggression when hunting. However, herding dogs have higher abilities of eye-stalk and chase but strongly inhibit the grasping, biting, and killing instincts to prevent them from hurting livestock (19, 21). In a study of the behavioral interactions between dogs and livestock during herding, dog lip-licking and barking occurred less frequently, while stalking, crouching, and chasing were more frequent. Moreover, not a single case of biting was observed (21). Different neurotransmitters have been detected among three dog breeds with distinct predatory behaviors: Border Collies, Siberian Huskies, and Sharplaninatz (68). The GWAS between hound and herding dogs revealed three genes for prey-driven behavior (Figure 3A). The JAK2 gene is located 1,193 bp downstream of CFA1 association region (Table 2), which has been previously detected to be associated with dog snout ratio and curly tail (3, 4). One study found that dog chasing behavior has been significantly associated with skull shape. Specifically, hound or herding dog breeds tend to have long skulls as their historical roles in pursuit of potential prey animals or livestock, while companion dogs such as toy group canines tend to have short skulls. It implies that skull shape is an indicator of hunting-related behavior (11). Artificial selection based on morphological traits (like short skulls) could have affected dog behavior traits (like tendency to hunt). Meanwhile, JAK2 is widely expressed and found to be potentially associated with dozens of traits by GWAS Catalog (https://www.ebi.ac.uk/gwas/genes/JAK2). Among these diverse roles, JAK2 gene is involved in synaptic plasticity and has an essential role in the induction of NMDA-receptor dependent long-term depression (69). Inactivation of JAK2 can cause memory loss in Alzheimer's disease (70). We found that MEIS1 gene was detected as the nearest gene to the significantly associated region on CFA10 (Table 2 and Figure 3A), and MEIS1 was reported to be associated with restless legs syndrome (71). Patients with this neurological disorder have an irresistible urge to move their legs, which can affect sleep quality and even cause mood problems, like depression. Hyperactivity was also observed in heterozygous MEIS1-deficient mice, suggesting its role in the specification of neuronal progenitors (72). Therefore, we propose that MEIS1 may be associated with greater search and chase impulses in hounds when confronted with prey. The nearest gene to the significantly associated region on CFA17 for predation was LRRTM4. It has been reported that LRRTM4 facilitates formation of excitatory synapse development on hippocampal dentate gyrus granule cells (73). More importantly, this gene was close to the strongest associated signal in GWAS analysis of children with aggressive behavior (74). Combined with the gene function and the report in humans, we suggest that LRRTM4 may play a role in the differences in aggressive behavior between hounds and herding dogs. Moreover, only LRRTM4 gene was left to be significantly associated with predation after correcting for breed standard body sizes.
Well-behaved dogs are appealing and conducive to establishing good interaction with humans. Temperament and trainability are the foundation of a dog's daily socialization or sports training, which are interesting traits for both dog owners and breeders. Dog fetching behavior has been detected to have suggestive association with CFA22:32270336, which is 2.6 Mb away from our significantly associated signal CFA:34873149 (12). Fetching behavior has been proven to be the most efficient training method for building human–dog relationships, and it is a good indicator of trainability. The significantly associated gene of temperament GWAS has been detected to be ACSS3 gene (Figure 4). Recently, ACSS3 has been reported to be significantly associated with human depressive symptoms (75) and antidepressant response (76). Our results suggested that the ACSS3 gene may contribute to the development of temperament in dogs. Different breeds of dogs have been strongly artificially selected to perform different tasks, accompanied by the production of multiple personalities. Increasing numbers of researches are focusing on the possibilities of dogs as models for studying neurological diseases (29, 77). Although the variant significantly associated with dog trainability is nearest to LOC111091672, the nearest protein-coding genes upstream and downstream are SPRY2 (distance of 1.50 Mb) and SLITRK1 (distance of 1.45 Mb). SPRY2 was detected to be highly expressed in the human brain, with the highest expression in the cerebellum [http://biogps.org/#goto=genereport&id=10253; (78)]. Variants in SLITRK1 gene are associated with human psychiatric disorders such as Tourette's syndrome (79) and OCD (80). MacLean et al. found that trainability had a very high heritability (h2 = 0.73) (7), indicating that the percentage of variance explained in the GWASs should be high. However, our top vs. bottom GWAS designed based on the AKC breed standard descriptions was underpowered. The AKC written descriptions of dog breed temperament and trainability are not accurate enough for detecting variants controlling the behavioral differences among breeds. This may be one reason for the less signals obtained in GWAS of temperament and trainability.
The selection of genomic regulatory regions could contribute large effects on the formation of canine breed standards (81). Notably, epigenetic variations also play important roles in the behavioral formation (82–84). This might due to the fact that gene coding regions are more conserved than non-coding regions, and protein-coding regions typically evolve at a slower rate. Behavioral selection for dog domestication might be caused by the regulation of gene expressions in the hypothalamus (85). Several variants within lncRNAs or potential gene regulatory regions were detected in our studies, which implies that they could play crucial roles in herding behavior formation through regulating gene expressions of the candidate neural genes.
Enrichment analysis was performed with candidate genes obtained from private variant analysis. Several processes or cellular components related to neurology function were obtained (Table 4). This indicates that changes in the regulation of neuron and nervous system development could contribute to herding behavior formation. These seven candidate genes could be involved in the early neural system development (Supplementary Figure 3), which raises their possibilities of being regarded as candidate genes underlying dog behaviors. To increase the credibility of mapping, only variants above the Bonferroni genome-wide significance threshold were considered as candidates for herding and predation GWAS. Overall, seven promising candidate genes were identified for dog herding (THOC1, MSRB3, LLPH, RFX8, and CHL1), predation (LRRTM4), and temperament (ACSS3) between dog breeds after correcting with body mass in this study. Though associations of ASIC2, JAK2, and MEIS1 gene regions were not above significant threshold after controlling body size, they could still have potential roles on dog behaviors through effects on dog brain architectures, which are related with body mass.
There are several limitations in this study. Specifically, herding group dogs are from different breeds that share herding behavior, but we were not able to determine if all herding dog breeds share a common ancestor. Phenotypic classification based on breed standard described by the AKC is not robust enough to detect all the genetic variants between dog breeds, especially for trainability. Further studies using breed-average C-BARQ values could improve the accuracy. Even though GWASs using small numbers of dog individuals of very many breeds have proved to be powerful methods to identifying variants influencing morphology (5), it is still prudent to simply apply GWASs to behavioral traits.
To fine-map the casual variants or genes for these behavioral traits accurately, professional behavioral scientists are required to perform accurate phenotypic dissections for those traits, which will be performed in Dog10K project (86). With more accurate phenotypic definitions of dog behavioral traits and more dog whole-genome sequences released by Dog10k project, the understanding of genetic mechanisms underlying these behavioral traits will be significantly enhanced. In the following studies, accurate behavioral measurement methods such as Herding Trait Characterization (HTC) could be applied to evaluate a large number of dogs from diverse breeds (87). Similar to this study, GWASs using breed-average scores of HTC questionnaire as phenotypes can be applied to identify genetic differences among dog breeds. To improve genetic mapping accuracy and reveal additional genes for these four dog behaviors, GWASs can be performed using genotype and phenotype data from the same canine individuals.
Cross-breed mapping approaches can effectively identify loci that may affect genetic differences between breeds that cannot be studied by segregation within breeds. The classic example is that the specific negative correlation between longevity and size is a strictly between-breed phenomenon and is difficult to conduct genetic analysis by within-breed studies (24). The herding behavior is also a clear between-breed behavior. Therefore, the method of classifying behaviors according to the historical roles of dogs and analyzing herding behaviors among dog breeds is reasonable. This was also reflected in the genetic mapping of herding, pointing, boldness, and athleticism in dogs, and convincing genes appropriate to behaviors were obtained (4, 9, 24). Zapata et al. (77) performed a genome-wide scan of several dog behaviors of diverse breeds and also identified genes that overlap with human neurodevelopmental and psychopathological genes, implying that dogs and humans share some degree of common molecular mechanisms during neurological development. Hence, this study may provide genetic clues to further elucidate the formation of behavioral traits in dogs and provide potential models for studying complex neuropsychiatric disorders in humans.
The original contributions presented in the study are included in the article/Supplementary Material, further inquiries can be directed to the corresponding author/s.
SS: data analysis, writing-original draft, review, and editing. FX: conceptualization, data analysis, writing-original draft, review, and editing. BB: supervision, writing-review, and editing. All authors contributed to the article and approved the submitted version.
The authors declare that the research was conducted in the absence of any commercial or financial relationships that could be construed as a potential conflict of interest.
We are grateful to Dr. Ostrander and her co-authors for the availability of 722 canine genomes. SS and FX are fellows of the China Scholarship Council (CSC). Open Access Publications are supported by the University of Göttingen.
The Supplementary Material for this article can be found online at: https://www.frontiersin.org/articles/10.3389/fvets.2021.693290/full#supplementary-material
1. Bergström A, Frantz L, Schmidt R, Ersmark E, Lebrasseur O, Girdland-Flink L, et al. Origins and genetic legacy of prehistoric dogs. Science. (2020) 370:557–64. doi: 10.1126/science.aba9572
2. Lindblad-Toh K, Wade CM, Mikkelsen TS, Karlsson EK, Jaffe DB, Kamal M, et al. Genome sequence, comparative analysis and haplotype structure of the domestic dog. Nature. (2005) 438:803–19. doi: 10.1038/nature04338
3. Boyko AR, Quignon P, Li L, Schoenebeck JJ, Degenhardt JD, Lohmueller KE, et al. A simple genetic architecture underlies morphological variation in dogs. PLoS Biol. (2010) 8:e1000451. doi: 10.1371/journal.pbio.1000451
4. Vaysse A, Ratnakumar A, Derrien T, Axelsson E, Pielberg GR, Sigurdsson S, et al. Identification of genomic regions associated with phenotypic variation between dog breeds using selection mapping. PLoS Genet. (2011) 7:e1002316. doi: 10.1371/journal.pgen.1002316
5. Plassais J, Kim J, Davis BW, Karyadi DM, Hogan AN, Harris AC, et al. Whole genome sequencing of canids reveals genomic regions under selection and variants influencing morphology. Nat Commun. (2019) 10:1489 doi: 10.1038/s41467-019-09373-w
6. Gnanadesikan GE, Hare B, Snyder-Mackler N, Call J, Kaminski J, Miklósi Á, et al. Breed differences in dog cognition associated with brain-expressed genes and neurological functions. Integr Comp Biol. (2020) 60:976–90. doi: 10.1093/icb/icaa112
7. MacLean EL, Snyder-Mackler N, VonHoldt BM, Serpell JA. Highly heritable and functionally relevant breed differences in dog behaviour. Proc Biol Sci. (2019) 286:20190716. doi: 10.1098/rspb.2019.0716
8. Zapata I, Serpell JA, Alvarez CE. Genetic mapping of canine fear and aggression. BMC Genomics. (2016) 17:572. doi: 10.1186/s12864-016-2936-3
9. Kim J, Williams FJ, Dreger DL, Plassais J, Davis BW, Parker HG, et al. Genetic selection of athletic success in sport-hunting dogs. Proc Natl Acad Sci USA. (2018) 115:E7212–21. doi: 10.1073/pnas.1800455115
10. Chittka L, Rossiter SJ, Skorupski P, Fernando C. What is comparable in comparative cognition? Philos Trans R Soc Lond B Biol Sci. (2012) 367:2677–85. doi: 10.1098/rstb.2012.0215
11. McGreevy PD, Georgevsky D, Carrasco J, Valenzuela M, Duffy DL, Serpell JA. Dog behavior co-varies with height, bodyweight and skull shape. PLoS ONE. (2013) 8:e80529. doi: 10.1371/journal.pone.0080529
12. Ilska J, Haskell MJ, Blott SC, Sánchez-Molano E, Polgar Z, Lofgren SE, et al. Genetic characterization of dog personality traits. Genetics. (2017) 206:1101–11. doi: 10.1534/genetics.116.192674
13. Sarviaho R, Hakosalo O, Tiira K, Sulkama S, Salmela E, Hytonen MK, et al. Two novel genomic regions associated with fearfulness in dogs overlap human neuropsychiatric loci. Transl Psychiatry. (2019) 9:18. doi: 10.1038/s41398-018-0361-x
14. Sarviaho R, Hakosalo O, Tiira K, Sulkama S, Niskanen J, Hytönen M, et al. A novel genomic region on chromosome 11 associated with fearfulness in dogs. Transl Psychiatry. (2020) 10:169. doi: 10.1038/s41398-020-0849-z
15. Dodman NH, Karlsson EK, Moon-Fanelli A, Galdzicka M, Perloski M, Shuster L, et al. A canine chromosome 7 locus confers compulsive disorder susceptibility. Mol Psychiatry. (2010) 15:8–10. doi: 10.1038/mp.2009.111
16. Tang R, Noh HJ, Wang D, Sigurdsson S, Swofford R, Perloski M, et al. Candidate genes and functional noncoding variants identified in a canine model of obsessive-compulsive disorder. Genome Biol. (2014) 15:R25. doi: 10.1186/gb-2014-15-3-r25
17. Shuldiner E, Koch IJ, Kartzinel RY, Hogan A, Brubaker L, Wanser S, et al. Structural variants in genes associated with human Williams-Beuren syndrome underlie stereotypical hypersociability in domestic dogs. Sci Adv. (2017) 3:e1700398. doi: 10.1126/sciadv.1700398
18. Luciano M, Davies G, Summers KM, Hill WD, Hayward C, Liewald DC, et al. The influence of X chromosome variants on trait neuroticism. Mol Psychiatry. (2021) 26:483–91. doi: 10.1038/s41380-019-0388-2
19. Coppinger R, Coppinger L. Chapter 6. Behavioral Conformation. In: Dogs: A Startling New Understanding of Canine Origin, Behavior & Evolution. New York, NY: Scribner (2001). p. 189–224.
20. Renna CH. Herding Dogs: Selection and Training the Working Farm Dog. Allenhurst, NJ: Kennel Club Books (KCB) (2008).
21. Early J, Aalders J, Arnott E, Wade C, McGreevy P. Sequential analysis of livestock herding dog and sheep interactions. Animals. (2020) 10:352. doi: 10.3390/ani10020352
23. Kolm N, Temrin H, Miklósi Á, Kubinyi E, Garamszegi LZ. The link between selection for function and human-directed play behaviour in dogs. Biol Lett. (2020) 16:20200366. doi: 10.1098/rsbl.2020.0366
24. Jones P, Chase K, Martin A, Davern P, Ostrander EA, Lark KG. Single-nucleotide-polymorphism-based association mapping of dog stereotypes. Genetics. (2008) 179:1033–44. doi: 10.1534/genetics.108.087866
25. Bray EE, Sammel MD, Cheney DL, Serpell JA, Seyfarth RM. Effects of maternal investment, temperament, and cognition on guide dog success. Proc Natl Acad Sci USA. (2017) 114:9128–33. doi: 10.1073/pnas.1704303114
26. Jones AC, Gosling SD. Temperament and personality in dogs (Canis familiaris): a review and evaluation of past research. Appl Anim Behav Sci. (2005) 95:1–53. doi: 10.1016/j.applanim.2005.04.008
27. Hejjas K, Vas J, Topál J, Szántai E, Rónai Z, Székely A, et al. Association of polymorphisms in the dopamine D4 receptor gene and the activity-impulsivity endophenotype in dogs. Anim Genet. (2007) 38:629–33. doi: 10.1111/j.1365-2052.2007.01657.x
28. Turcsán B, Kubinyi E, Miklósi Á. Trainability and boldness traits differ between dog breed clusters based on conventional breed categories and genetic relatedness. Appl Anim Behav Sci. (2011) 132:61–70. doi: 10.1016/j.applanim.2011.03.006
29. Hecht EE, Smaers JB, Dunn WD, Kent M, Preuss TM, Gutman DA. Significant neuroanatomical variation among domestic dog breeds. J Neurosci. (2019) 39:7748–58. doi: 10.1523/JNEUROSCI.0303-19.2019
30. Horschler DJ, Hare B, Call J, Kaminski J, Miklósi Á, MacLean EL. Absolute brain size predicts dog breed differences in executive function. Anim Cogn. (2019) 22:187–98. doi: 10.1007/s10071-018-01234-1
31. Purcell S, Neale B, Todd-Brown K, Thomas L, Ferreira MA, Bender D, et al. PLINK: a tool set for whole-genome association and population-based linkage analyses. Am J Hum Genet. (2007) 81:559–75. doi: 10.1086/519795
32. Zhou X, Stephens M. Genome-wide efficient mixed-model analysis for association studies. Nat Genet. (2012) 44:821–4. doi: 10.1038/ng.2310
33. Lander E, Kruglyak L. Genetic dissection of complex traits: guidelines for interpreting and reporting linkage results. Nat Genet. (1995) 11:241–7. doi: 10.1038/ng1195-241
34. Turner SD. qqman: an R package for visualizing GWAS results using QQ and manhattan plots. bioRxiv [Preprint]. (2014) 005165. doi: 10.1101/005165
35. Hayward JJ, Castelhano MG, Oliveira KC, Corey E, Balkman C, Baxter TL, et al. Complex disease and phenotype mapping in the domestic dog. Nat Commun. (2016) 7:10460. doi: 10.1038/ncomms10460
36. Danecek P, Auton A, Abecasis G, Albers CA, Banks E, DePristo MA, et al. The variant call format and VCFtools. Bioinformatics. (2011) 27:2156–8. doi: 10.1093/bioinformatics/btr330
37. Cingolani P, Platts A, Wang LL, Coon M, Nguyen T, Wang L, et al. A program for annotating and predicting the effects of single nucleotide polymorphisms, SnpEff: SNPs in the genome of Drosophila melanogaster strain w1118; iso-2; iso-3. Fly. (2012) 6:80–92. doi: 10.4161/fly.19695
38. Liao Y, Wang J, Jaehnig EJ, Shi Z, Zhang B. WebGestalt 2019: gene set analysis toolkit with revamped UIs and APIs. Nucleic Acids Res. (2019) 47:W199–205. doi: 10.1093/nar/gkz401
39. Wang Z, Feng X, Li SC. SCDevDB: a database for insights into single-cell gene expression profiles during human developmental processes. Front Genet. (2019) 10:903. doi: 10.3389/fgene.2019.00903
40. Yao Z, Mich JK, Ku S, Menon V, Krostag A-R, Martinez RA, et al. A single-cell roadmap of lineage bifurcation in human ESC models of embryonic brain development. Cell Stem Cell. (2017) 20:120–34. doi: 10.1016/j.stem.2016.09.011
41. Sunkin SM, Ng L, Lau C, Dolbeare T, Gilbert TL, Thompson CL, et al. Allen Brain Atlas: an integrated spatio-temporal portal for exploring the central nervous system. Nucleic Acids Res. (2013) 41:D996–1008. doi: 10.1093/nar/gks1042
42. Ahmed ZM, Yousaf R, Lee BC, Khan SN, Lee S, Lee K, et al. Functional null mutations of MSRB3 encoding methionine sulfoxide reductase are associated with human deafness DFNB74. Am J Hum Genet. (2011) 88:19–29. doi: 10.1016/j.ajhg.2010.11.010
43. Maeder CI, Kim J-I, Liang X, Kaganovsky K, Shen A, Li Q, et al. The THO complex coordinates transcripts for synapse development and dopamine neuron survival. Cell. (2018) 174:1436–49. e20. doi: 10.1016/j.cell.2018.07.046
44. Askwith CC, Wemmie JA, Price MP, Rokhlina T, Welsh MJ. Acid-sensing ion channel 2 (ASIC2) modulates ASIC1 H+-activated currents in hippocampal neurons. J Biol Chem. (2004) 279:18296–305. doi: 10.1074/jbc.M312145200
45. Sugiaman-Trapman D, Vitezic M, Jouhilahti E-M, Mathelier A, Lauter G, Misra S, et al. Characterization of the human RFX transcription factor family by regulatory and target gene analysis. BMC Genomics. (2018) 19:181. doi: 10.1186/s12864-018-4564-6
46. Siniscalchi M, Bertino D, d'Ingeo S, Quaranta A. Relationship between motor laterality and aggressive behavior in sheepdogs. Symmetry. (2019) 11:233. doi: 10.3390/sym11020233
47. Martin HC, Kim GE, Pagnamenta AT, Murakami Y, Carvill GL, Meyer E, et al. Clinical whole-genome sequencing in severe early-onset epilepsy reveals new genes and improves molecular diagnosis. Hum Mol Genet. (2014) 23:3200–11. doi: 10.1093/hmg/ddu030
48. Sahlén P, Yanhu L, Xu J, Kubinyi E, Wang G-D, Savolainen P. Variants that differentiate wolf and dog populations are enriched in regulatory elements. Genome Biol Evol. (2021) 13:evab076. doi: 10.1093/gbe/evab076
49. Kwon T-J, Cho H-J, Kim U-K, Lee E, Oh S-K, Bok J, et al. Methionine sulfoxide reductase B3 deficiency causes hearing loss due to stereocilia degeneration and apoptotic cell death in cochlear hair cells. Hum Mol Genet. (2014) 23:1591–601. doi: 10.1093/hmg/ddt549
50. Hibar DP, Adams HH, Jahanshad N, Chauhan G, Stein JL, Hofer E, et al. Novel genetic loci associated with hippocampal volume. Nat Commun. (2017) 8:13624. doi: 10.1038/ncomms13624
51. Lim D-H, Han JY, Kim J-R, Lee YS, Kim H-Y. Methionine sulfoxide reductase B in the endoplasmic reticulum is critical for stress resistance and aging in Drosophila. Biochem Biophys Res Commun. (2012) 419:20–6. doi: 10.1016/j.bbrc.2012.01.099
52. Yu N-K, Kim HF, Shim J, Kim S, Kim DW, Kwak C, et al. A transducible nuclear/nucleolar protein, mLLP, regulates neuronal morphogenesis and synaptic transmission. Sci Rep. (2016) 6:22892. doi: 10.1038/srep22892
53. Webster MT, Kamgari N, Perloski M, Hoeppner MP, Axelsson E, Hedhammar Å, et al. Linked genetic variants on chromosome 10 control ear morphology and body mass among dog breeds. BMC Genomics. (2015) 16:474. doi: 10.1186/s12864-015-1702-2
54. Son Y-J, Thompson WJ. Schwann cell processes guide regeneration of peripheral axons. Neuron. (1995) 14:125–32. doi: 10.1016/0896-6273(95)90246-5
55. Coe BP, Stessman HA, Sulovari A, Geisheker MR, Bakken TE, Lake AM, et al. Neurodevelopmental disease genes implicated by de novo mutation and copy number variation morbidity. Nat Genet. (2019) 51:106–16. doi: 10.1038/s41588-018-0288-4
56. Kopp F, Mendell JT. Functional classification and experimental dissection of long noncoding RNAs. Cell. (2018) 172:393–407. doi: 10.1016/j.cell.2018.01.011
57. Hillenbrand R, Molthagen M, Montag D, Schachner M. The close homologue of the neural adhesion molecule L1 (CHL1): patterns of expression and promotion of neurite outgrowth by heterophilic interactions. Eur J Neurosci. (1999) 11:813–26. doi: 10.1046/j.1460-9568.1999.00496.x
58. Buhusi M, Midkiff BR, Gates AM, Richter M, Schachner M, Maness PF. Close homolog of L1 is an enhancer of integrin-mediated cell migration. J Biol Chem. (2003) 278:25024–31. doi: 10.1074/jbc.M303084200
59. Angeloni D, Wei M, Lerman M. Two single nucleotide polymorphisms (SNPs) in the CALL gene for association studies with IQ. Psychiatr Genet. (1999) 9:165–7. doi: 10.1097/00041444-199909000-00011
60. Angeloni D, Lindor NM, Pack S, Latif F, Wei MH, Lerman MI. CALL gene is haploinsufficient in a 3p– syndrome patient. Am J Med Genet. (1999) 86:482–5. doi: 10.1002/(sici)1096-8628(19991029)86:5<482::aid-ajmg15>3.0.co;2-l
61. Montag-Sallaz M, Schachner M, Montag D. Misguided axonal projections, neural cell adhesion molecule 180 mRNA upregulation, and altered behavior in mice deficient for the close homolog of L1. Mol Cell Biol. (2002) 22:7967–81. doi: 10.1128/mcb.22.22.7967-7981.2002
62. Pratte M, Rougon G, Schachner M, Jamon M. Mice deficient for the close homologue of the neural adhesion cell L1 (CHL1) display alterations in emotional reactivity and motor coordination. Behav Brain Res. (2003) 147:31–9. doi: 10.1016/s0166-4328(03)00114-1
63. Leshchyns'ka I, Sytnyk V, Richter M, Andreyeva A, Puchkov D, Schachner M. The adhesion molecule CHL1 regulates uncoating of clathrin-coated synaptic vesicles. Neuron. (2006) 52:1011–25. doi: 10.1016/j.neuron.2006.10.020
64. Zhang L, Gao Y, Zhang R, Sun F, Cheng C, Qian F, et al. THOC1 deficiency leads to late-onset nonsyndromic hearing loss through p53-mediated hair cell apoptosis. PLoS Genet. (2020) 16:e1008953. doi: 10.1371/journal.pgen.1008953
65. Yokoyama JS, Lam ET, Ruhe AL, Erdman CA, Robertson KR, Webb AA, et al. Variation in genes related to cochlear biology is strongly associated with adult-onset deafness in border collies. PLoS Genet. (2012) 8:e1002898. doi: 10.1371/journal.pgen.1002898
66. Price MP, Gong H, Parsons MG, Kundert JR, Reznikov LR, Bernardinelli L, et al. Localization and behaviors in null mice suggest that ASIC1 and ASIC2 modulate responses to aversive stimuli. Genes Brain Behav. (2014) 13:179–94. doi: 10.1111/gbb.12108
67. Mehrkam LR, Wynne CD. Behavioral differences among breeds of domestic dogs (Canis lupus familiaris): current status of the science. Appl Anim Behav Sci. (2014) 155:12–27. doi: 10.1016/j.applanim.2014.03.005
68. Arons CD, Shoemaker WJ. The distribution of catecholamines and β-endorphin in the brains of three behaviorally distinct breeds of dogs and their F1 hybrids. Brain Res. (1992) 594:31–9. doi: 10.1016/0006-8993(92)91026-b
69. Nicolas CS, Peineau S, Amici M, Csaba Z, Fafouri A, Javalet C, et al. The Jak/STAT pathway is involved in synaptic plasticity. Neuron. (2012) 73:374–90. doi: 10.1016/j.neuron.2011.11.024
70. Chiba T, Yamada M, Sasabe J, Terashita K, Shimoda M, Matsuoka M, et al. Amyloid-β causes memory impairment by disturbing the JAK2/STAT3 axis in hippocampal neurons. Mol Psychiatry. (2009) 14:206–22. doi: 10.1038/mp.2008.105
71. Winkelmann J, Schormair B, Lichtner P, Ripke S, Xiong L, Jalilzadeh S, et al. Genome-wide association study of restless legs syndrome identifies common variants in three genomic regions. Nat Genet. (2007) 39:1000–6. doi: 10.1038/ng2099
72. Spieler D, Kaffe M, Knauf F, Bessa J, Tena JJ, Giesert F, et al. Restless Legs Syndrome-associated intronic common variant in Meis1 alters enhancer function in the developing telencephalon. Genome Res. (2014) 24:592–603. doi: 10.1101/gr.166751.113
73. Siddiqui TJ, Tari PK, Connor SA, Zhang P, Dobie FA, She K, et al. An LRRTM4-HSPG complex mediates excitatory synapse development on dentate gyrus granule cells. Neuron. (2013) 79:680–95. doi: 10.1016/j.neuron.2013.06.029
74. Pappa I, St Pourcain B, Benke K, Cavadino A, Hakulinen C, Nivard MG, et al. A genome-wide approach to children's aggressive behavior: the EAGLE consortium. Am J Med Genet B Neuropsychiatr Genet. (2016) 171:562–72. doi: 10.1002/ajmg.b.32333
75. Arnau-Soler A, Macdonald-Dunlop E, Adams MJ, Clarke T-K, MacIntyre DJ, Milburn K, et al. Genome-wide by environment interaction studies of depressive symptoms and psychosocial stress in UK Biobank and Generation Scotland. Transl Psychiatry. (2019) 9:1–13. doi: 10.1038/s41398-018-0360-y
76. Hunter AM, Leuchter AF, Power RA, Muthén B, McGrath PJ, Lewis CM, et al. A genome-wide association study of a sustained pattern of antidepressant response. J Psychiatr Res. (2013) 47:1157–65. doi: 10.1016/j.jpsychires.2013.05.002
77. Zapata I, Hecht EE, Serpell JA, Alvarez CE. Genome scans of dog behavior implicate a gene network underlying psychopathology in mammals, including humans. bioRxiv [Preprint]. (2021). doi: 10.1101/2020.07.19.211078
78. Wu CL, Jin XF, Tsueng G, Afrasiabi C, Su AI. BioGPS: building your own mash-up of gene annotations and expression profiles. Nucleic Acids Res. (2016) 44:D313–D6. doi: 10.1093/nar/gkv1104
79. Abelson JF, Kwan KY, O'Roak BJ, Baek DY, Stillman AA, Morgan TM, et al. Sequence variants in SLITRK1 are associated with Tourette's syndrome. Science. (2005) 310:317–20. doi: 10.1126/science.1116502
80. Wendland J, Kruse M, Murphy D. Functional SLITRK1 var321, varCDfs and SLC6A4 G56A variants and susceptibility to obsessive-compulsive disorder. Mol Psychiatry. (2006) 11:802–4. doi: 10.1038/sj.mp.4001848
81. Consortium EP. An integrated encyclopedia of DNA elements in the human genome. Nature. (2012) 489:57–74. doi: 10.1038/nature11247
82. Hammock EA, Young LJ. Microsatellite instability generates diversity in brain and sociobehavioral traits. Science. (2005) 308:1630–4. doi: 10.1126/science.1111427
83. Spady TC, Ostrander EA. Canine behavioral genetics: pointing out the phenotypes and herding up the genes. Am J Hum Genet. (2008) 82:10–8. doi: 10.1016/j.ajhg.2007.12.001
84. Janowitz Koch I, Clark MM, Thompson MJ, Deere-Machemer KA, Wang J, Duarte L, et al. The concerted impact of domestication and transposon insertions on methylation patterns between dogs and grey wolves. Mol Ecol. (2016) 25:1838–55. doi: 10.1111/mec.13480
85. Saetre P, Lindberg J, Leonard JA, Olsson K, Pettersson U, Ellegren H, et al. From wild wolf to domestic dog: gene expression changes in the brain. Brain Res Mol Brain Res. (2004) 126:198–206. doi: 10.1016/j.molbrainres.2004.05.003
86. Ostrander EA, Wang G-D, Larson G, Vonholdt BM, Davis BW, Jagannathan V, et al. Dog10K: an international sequencing effort to advance studies of canine domestication, phenotypes and health. Natl Sci Rev. (2019) 6:810–24. doi: 10.1093/nsr/nwz049
Keywords: dog behavior, GWAS, herding, predation, temperament, trainability, neurological genes
Citation: Shan S, Xu F and Brenig B (2021) Genome-Wide Association Studies Reveal Neurological Genes for Dog Herding, Predation, Temperament, and Trainability Traits. Front. Vet. Sci. 8:693290. doi: 10.3389/fvets.2021.693290
Received: 10 April 2021; Accepted: 15 June 2021;
Published: 21 July 2021.
Edited by:
Jean-Loup Rault, University of Veterinary Medicine Vienna, AustriaReviewed by:
Carlos Alvarez, Nationwide Children's Hospital, United StatesCopyright © 2021 Shan, Xu and Brenig. This is an open-access article distributed under the terms of the Creative Commons Attribution License (CC BY). The use, distribution or reproduction in other forums is permitted, provided the original author(s) and the copyright owner(s) are credited and that the original publication in this journal is cited, in accordance with accepted academic practice. No use, distribution or reproduction is permitted which does not comply with these terms.
*Correspondence: Fangzheng Xu, ZmFuZ3poZW5nLnh1QHN0dWQudW5pLWdvZXR0aW5nZW4uZGU=
Disclaimer: All claims expressed in this article are solely those of the authors and do not necessarily represent those of their affiliated organizations, or those of the publisher, the editors and the reviewers. Any product that may be evaluated in this article or claim that may be made by its manufacturer is not guaranteed or endorsed by the publisher.
Research integrity at Frontiers
Learn more about the work of our research integrity team to safeguard the quality of each article we publish.