- 1Laboratory of Gastrointestinal Microbiology, Jiangsu Key Laboratory of Gastrointestinal Nutrition and Animal Health, College of Animal Science and Technology, Nanjing Agricultural University, Nanjing, China
- 2National Center for International Research on Animal Gut Nutrition, Nanjing Agricultural University, Nanjing, China
Time-restricted feeding (TRF) mode is a potential strategy in improving the health and production of farm animals. However, the effect of TRF on microbiota and their metabolism in the large intestine of the host remains unclear. Therefore, the present study aimed to investigate the responses of microbiome and metabolome induced by TRF based on a growing-pig model. Twelve crossbred growing barrows were randomly allotted into two groups with six replicates (1 pig/pen), namely, the free-access feeding group (FA) and TRF group. Pigs in the FA group were fed free access while the TRF group were fed free access within a regular time three times per day at 07:00–08:00, 12:00–13:00, and 18:00–19:00, respectively. Results showed that the concentrations of NH4-N, putrescine, cadaverine, spermidine, spermine, total biogenic amines, isobutyrate, butyrate, isovalerate, total SCFA, and lactate were increased while the pH value in the colonic digesta and the concentration of acetate was decreased in the TRF group. The Shannon index was significantly increased in the TRF group; however, no significant effects were found in the Fisher index, Simpson index, ACE index, Chao1 index, and observed species between the two groups. In the TRF group, the relative abundances of Prevotella 1 and Eubacterium ruminantium group were significantly increased while the relative abundances of Clostridium sensu sticto 1, Lactobacillus, and Eubacterium coprostanoligenes group were decreased compared with the FA group. PLS-DA analysis revealed an obvious and regular variation between the FA and TRF groups, further pathway enrichment analysis showed that these differential features were mainly enriched in pyrimidine metabolism, nicotinate and nicotinamide metabolism, glycerolipid metabolism, and fructose and mannose metabolism. In addition, Pearson's correlation analysis indicated that the changes in the microbial genera were correlated with the colonic metabolites. In conclusion, these results together indicated that although the overall microbial composition in the colon was not changed, TRF induced the gradient changes of the nutrients and metabolites which were correlated with certain microbial genera including Lactobacillus, Eubacterium_ruminantium group, Eubacterium coprostanoligenes group, Prevotella 1, and Clostridium sensu sticto 1. However, more studies are needed to understand the impacts of TRF on the health and metabolism of growing pigs.
Introduction
Recently, a considerable amount of reference highlighted the contributions of the type, quantity, and composition of nutrients intake to host health and metabolism both on animals and human beings (1–4). By contrast, there are fewer studies concerning the effects of feeding patterns on health and metabolism. However, to date, limited literature has already indicated that changes in feeding patterns may also affect the metabolism, health, and production of animals (5, 6). Specifically, Rothschild et al. (7) reviewed that TRF trends to reduce the body weight, total cholesterol, and concentrations of triglycerides, glucose, insulin, interleukin, and tumor necrosis factor-α with improving insulin sensitivity (7). Consistently, TRF was reported to ameliorate the serum lipid and liver profiles of the individuals and increased the richness of the gut microbiota on human beings (8). Zarrinpar et al. (9) reported that compared with the FA group, TRF has changed the dynamics both in the relative abundance and compositions of gut microflora on mice as well as liver metabolism. These studies concerning mice and human beings together indicated that TRF has profound effects on the host metabolism and may be a potential remedy for the prevention of metabolic diseases and promoting a profitable and safe swine production. However, the mechanisms underpinning the beneficial effects of TRF on metabolic health remain largely unknown.
In recent years, more and more evidence has indicated that the microbiota plays a crucial role in host metabolism and health (10, 11). Vice versa, factors including diet composition (12), nutritional concentrations (13), and diet types (14) were found to shape the microbial communities. Therefore, we hypothesized that TRF changed the concentration gradient of nutrients, thus, preferentially stimulating the proliferation of certain microorganisms in the intestine and further exerting its beneficial effects on host health and metabolism. As obesity has become a global concern and has a close relationship with food taking, related studies mostly focus on indicators such as glucose and lipid metabolism, which are mostly performed on mice. More studies concerning the effects of TRF on gut microbiota and relevant metabolome are needed. Hence, the present study aimed to explore the metabolome-microbiome responses of growing pigs induced by a time-restricted feeding and to study the relationships between the gut microbiota, gut environment, and metabolites. Specifically, the feeding pattern used in the present study was similar to the three-meal pattern in modern society. Considering the high similarity in anatomy, physiology, polyphagy, habits, metabolism, and gut microbiota between pigs and human beings (15, 16), findings from this study will provide information on the applications of TRF on human beings as well.
Materials and Methods
Ethics Statement
This study was approved by the Nanjing Agricultural University Animal Care and Use Committee (Nanjing, Jiangsu Province, China) (SYXK2019-0066). All animal care procedures in the experiment were operated according to the standard of Experimental Animal Care and Use Guidelines of China (EACUGC2018-01).
Animals, Housing, and Sample
Twelve 105-day growing crossbred barrows (Duroc × Landrace × Large White, average bodyweight = 56.29 ± 1.38 kg) were randomly allotted into two groups with six replicates (1 pig/pen) per group, namely, the free-access feeding group (FA) and TRF group. Pigs in the FA group were fed free-access to feed while the TRF group were fed free-access within a regular time three times per day at 07:00–08:00, 12:00–13:00, and 18:00–19:00, respectively. All pigs were fed with the same commercial pellet feed for growing pigs and ad libitum access to water throughout the experiment period. After a 14-day ad libitum feeding during the pre-experiment period, the trial started and lasted for 21 days. All pigs were slaughtered and colonic digesta samples were collected on the 21st day. After measuring the pH using a portable pH meter (Hanna Instruments, Villafranca, Italy), all samples were stored under −80°C for microbiota, microbial metabolites, and metabolome analysis.
DNA Extraction, 16S rRNA Gene Amplification, and Sequencing
Total DNA from colonic digesta was extracted using the cetrimonium bromide (CTAB) method according to a previous method (17). The sequencing was finished by Shanghai Biozeron Biotechnology Co., Ltd (Shanghai, China). Shortly, the V3–V4 regions of the bacterial 16S rRNA gene were amplified using a universal primer with the barcode [forward primer (5′-ACTC CTRCGGGAGGCAGCAG-3′) and a reverse primer (5′-GGACTACCVGGGTATCTAAT-3′)] (18). Then, sequencing libraries were generated using the NEB Next®Ultra™DNA Library Prep Kit for Illumina (NEB, USA) following the manufacturer's recommendations, and index codes were added. At last, the library was sequenced on an Illumina MiSeq platform and 250/300 bp paired-end reads were generated.
QIIME (version 1.17) was used to demultiplex and quality-filter the raw sequence. Reads that were shorter than 50 bp and those that could not be assembled were discarded. Reads with an exact barcode matching and two nucleotide mismatches in primer matching, or containing ambiguous characters, were removed. According to the overlap sequence, only sequences that overlap longer than 10 bp were assembled according to their overlap sequence. Reads which could not be assembled were discarded. Operational taxonomic units (OTUs) were clustered with a 97% similarity cutoff standard using UPARSE (version 7.1, http://drive5.com/uparse/), and chimeric sequences were identified and removed using UCHIME. The phylogenetic affiliation of each 16S rRNA gene sequence was analyzed by the RDP Classifier (http://rdp.cme.msu.edu/) against the silva (SSU115). Rarefaction curve, Shannon and Simpson diversity indices, and Ace and Chao richness estimators were assessed using an online website (MicrobiomeAnalyst, https://www.microbiomeanalyst.ca/). The principal coordinate analysis (PCoA) was conducted based on the Bray–Curtis distance. EdgeR algorithms were used for performing a differential abundance analysis.
Metabolome Analysis and Data Processing
Metabolome analyses were finished by a commercial company named BioCluster (Shanghai, China). Briefly, 50 mg of the colonic sample was extracted with 800 μL of 80% methanol. A total of 200 μL of supernatant was added with 5 μL of internal standard (140 μg/mL, DL-o-Chlorophenylalanine), then transferred to a vial for LC-MS analysis. LC-MS Analysis was finished using the analysis of the LC-MS platform (Thermo, Ultimate 3000LC, Q Exactive) under the following setups: Column: Hyper gold C18 (100 × 2.1 mm 1.9 μm); Chromatographic separation conditions: Column temperature: 40°C; Flow rate: 0.3 mL/min; Mobile phase A: water +5% acetonitrile +0.1% formic acid; Mobile phase B: acetonitrile +0.1% formic acid; Injection volume: 4 μL; Automatic injector temperature: 4°C; ESI+: Heater Temp 300°C; Sheath Gas Flow rate, 45 arb; Aux Gas Flow Rate, 15 arb; Sweep Gas Flow Rate, 1 arb; spray voltage, 3.0 KV; Capillary Temp, 350°C; S-Lens RF Level, 30%. ESI-: Heater Temp 300°C, Sheath Gas Flow rate, 45 arb; Aux Gas Flow Rate, 15 arb; Sweep Gas Flow Rate, 1 arb; spray voltage, 3.2 KV; Capillary Temp, 350°C; S-Lens RF Level, 60%.
All statistical analyses of the metabolites were done based on the different function modules of a powerful tool, which is available online (version 5.0,) (19). Features with >30% missing values were removed, and the missing values of the remaining features will be replaced by a very small value (half of the minimum positive value found in the data set) by default. No missing values were detected with the criterion. The data then underwent logarithmic transformation and normalization of auto-scaling. Fold change analysis and T-test were conducted to determine the fold change and statistical significance of each metabolite from the colonic samples of growing pigs from the TRF group compared with the FA group. PLS-DA was employed to picture the overall difference between the TRF and FA groups, and to explore the differential metabolites. The metabolites with variable importance projection (VIP) values above 1.0, P < 0.05 were selected as differential metabolites. The differential metabolites were used to execute an enrichment analysis to explore the main pathway changed by the TRF.
Short-Chain Fatty Acids (SCFAs)
The concentrations of SCFAs (acetate, propionate, isobutyrate, butyrate, isovalerate, valerate) were measured by the gas chronograph method according to Wang et al. (20). Shortly, 0.25 g of colonic digesta samples was suspended in 1.5 mL distilled water. After vortex-mixing, the suspension was centrifuged at 12,000 × rmp for 10 min at 4°C. A total of 1 mL of the supernatant was taken and mixed with 0.2 mL of 25% (w/v) metaphosphoric acid. The mixture was frozen at 20°C overnight. After thawing, the mixture was centrifuged at 12,000 × rmp for 20 min at 4°C, then 0.5 mL of the supernatant was taken and mixed with an isovolumic ether. Shaking of the mixture was performed to extract the SCFA in the aqueous phase. The organic phase was taken for further analysis using an Agilent 7890A gas chromatograph (Agilent Technologies, Wilmington, DE) equipped with the flame ionization detector (FID), splitless injection port. Standard curves are established using the gradient mixed standards to calculate the concentration of the measured SCFA. The total SCFAs were calculated by adding the six SCFAs measured.
Lactate
The concentration of lactate was measured by the colorimetric method using the reagent kit (PN: A019-2-1, Nanjing Jiancheng Institute of Biological Engineering, China) following the instructions in the specification.
Ammonia-N
The concentration of Ammonia-N in the colonic digesta was analyzed by the colorimetric method according to Shen et al. (21). Specifically, 0.1 g of colonic digesta were weighed and suspended in 1.5 mL of 0.2 M HCl. Following vortex-mixing, the suspension was centrifuged at 14,000 × g for 20 min at 4°C. A total of 0.5 mL of the supernatant was taken and mixed with 0.5 mL of 0.08% (w/v) sodium nitroprusside-sodium salicylate and 0.5 mL of sodium hypochlorite-sodium hydroxide solution. The mixture was vortex mixed and left to stand for 10 min. Absorbance at 700 nm was recorded. Gradient ammonium chloride solutions were used to establish a robust standard curve to calculate the concentration of Ammonia-N.
Biogenic Amines
Biogenic amines including methylamine, tryptamine, putrescine, cadaverine, tyramine, spermidine, and spermine in the colonic digesta were detected by a high-performance liquid chromatography method according to Yang et al. (22). Gradient mixed standards were also analyzed as the samples to establish a robust curve to calculate the concentrations of the biogenic amines.
Statistical Analysis
The significance of pH, NH4-N, biogenic amines, SCFAs, the differential microbiota, and metabolites between the two groups were analyzed using the unpaired two-tail t-test (IBM SPSS Statistics for Windows, Version 21.0). Pearson correlation was analyzed to evaluate the correlation between the differential microorganisms, microbial metabolites, and the features identified from the metabolome. P < 0.05 was considered to represent a significant difference. Data visualization was completed using the GraphPad Prism (version 8.0.1, GraphPad Software, San Diego, CA) and R software (version 4.0.4).
Results
Bacterial Community Composition in Colonic Digesta of Growing Pigs Under Different Feeding Modes
A total of 517,362 clean sequences were obtained after data filtering under a normal quality control system. Using the standard of 97% similarity level, these clean sequences were clustered into 784 OTUs. The rarefaction curves were gradually flattened out with the increasing sampling quantity (Supplementary Figure 1). The α-diversity indexes of the colonic bacterial community were shown in Figure 1. To a large extent, TRF had no significant effects on the Fisher index, Simpson index, ACE index, Chao1 index, and Observed species (P > 0.05). However, the Shannon index was significantly higher in the TRF group (P = 0.040). Also, the Principal coordinate analysis indicated that TRF could not distinguish the panorama of the colonic bacterial community (Supplementary Figure 2). Whereas, at the family level (Figure 2), the relative abundances of Prevotellaceae (P = 0.023), Campylobacteraceae (P = 0.010), Tannerellaceae (P = 0.012), and Anaeroplasmataceae (P = 0.021) were significantly higher in the TRF group compared to those in the FA group, while the relative abundances of Lactobacillaceae (P < 0.0014) in the FA group were significantly higher than the TRF group. Meanwhile, at the genus level (Figure 3), the relative abundances of Prevotella 1 (P = 0.0029) and Eubacterium ruminantium group (P = 0.017) were significantly higher in the TRF group, and the relative abundances of Clostridium sensu sticto 1 (P = 0.022), Lactobacillus (P = 0.002), and Eubacterium coprostanoligenes group (P = 0.0036) were lower compared with the FA group.
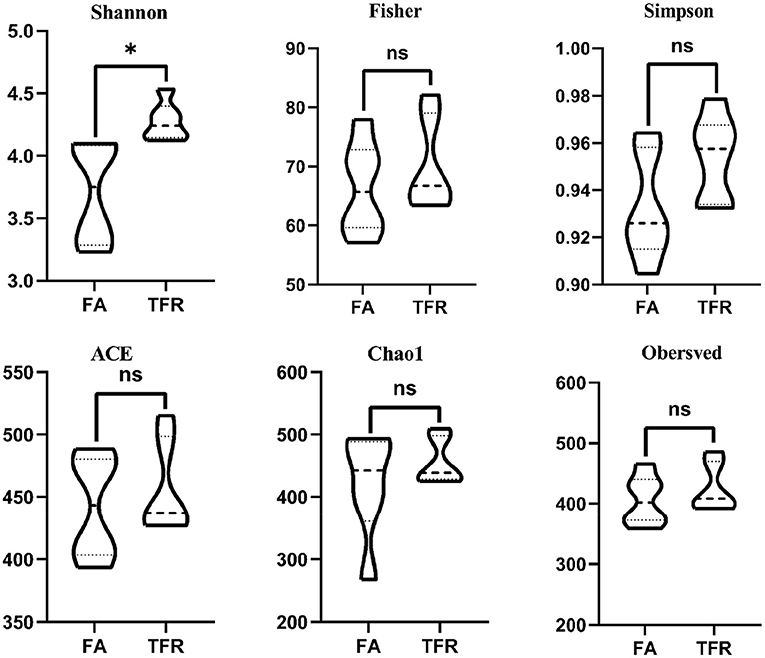
Figure 1. Differences in the colonic bacterial α-diversity index of growing pigs between the FA and TRF groups. FA, free access group; TRF, time-restricted feeding group. *Indicates a significant difference between the two groups; ns represents no significant difference.
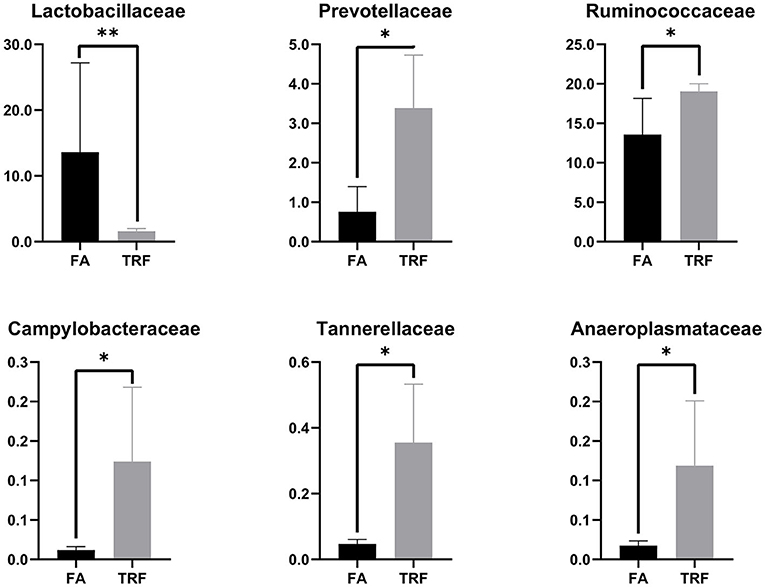
Figure 2. The relative abundance of bacteria in colonic digesta of growing pigs at the family level. FA, free access group; TRF, time-restricted feeding group. **Indicates a significant difference between the FA and TRF groups with P < 0.01; while *indicates a significant difference with P < 0.05.
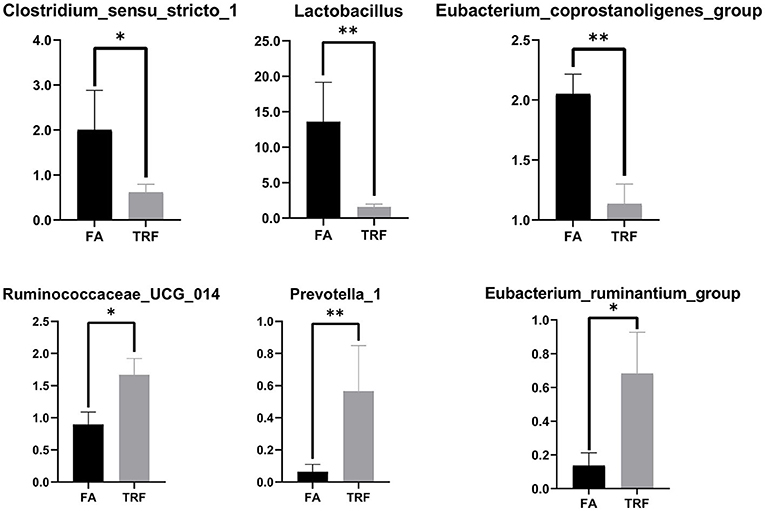
Figure 3. The relative abundances of bacteria in colonic digesta of growing pigs at the genus level. FA, free access group; TRF, time-restricted feeding group. **Indicates a significant difference between the FA and TRF groups with P < 0.01; while *indicates a significant difference with P < 0.05.
The pH, Bacterial Metabolites, and the Metabolome in Colonic Digesta of Growing Pigs
As shown in Table 1, TRF significantly increased the concentrations of NH4-N (P < 0.001), putrescine (P = 0.003), cadaverine (P = 0.010), spermidine (P < 0.001), spermine (P = 0.014), total biogenic amines (P < 0.001), isobutyrate (P < 0.001), butyrate (P = 0.005), isovalerate (P = 0.001), total SCFA (P = 0.008), and lactate (P = 0.024), while it decreased the pH value and the concentration of acetate (P = 0.002) in colonic digesta. TRF had a trend to increase the concentration of methylamine (P = 0.083) and tended to decrease the concentration of tyramine (P = 0.063). Using the LC-MS metabolomic technique, a total of 258 metabolites in the ESI+ mode and 123 metabolites in the ESI- mode were identified. According to the characteristics of the detected metabolites in each ion mode, one of the repeated metabolites detected in both ESI+ and ESI- modes were eliminated, the remaining 332 metabolites were combined for further analysis. These metabolites mainly included amino acids and their derivatives, organic acids, amines, nucleoside, flavonoid, fatty acids, peptides, etc. As shown in Figure 4, the PLS-DA analysis revealed an obvious and regular variation between the FA and TRF groups. Based on the standards of VIP > 1.0 and P < 0.05, a total of 22 significant differential features were identified (Table 2). Results of pathway enrichment analysis showed that these differential features were mainly enriched in pyrimidine metabolism, nicotinate and nicotinamide metabolism, glycerolipid metabolism, and fructose and mannose metabolism (Figure 5).
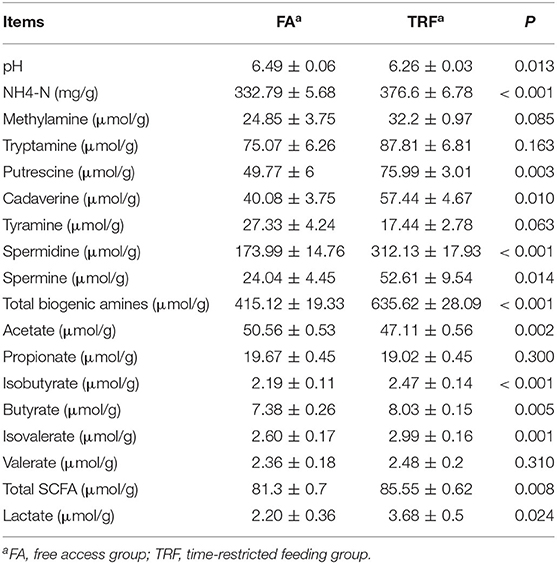
Table 1. The pH value and concentrations of lactic acid, NH4-N, biogenic amines, and SCFAs in colonic digesta of growing pigs.
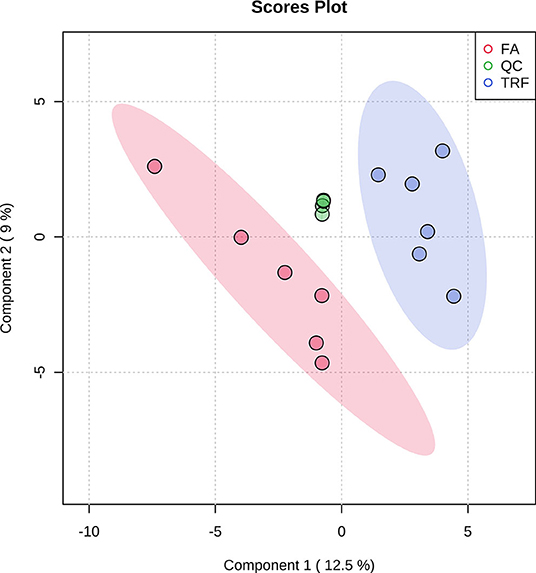
Figure 4. Partial least squares discriminant analysis (PLS-DA) score plot of colonic metabolites (both ESI+ and ESI-) of growing pigs in the FA and TRF groups. FA, free access group; TRF, time-restricted feeding group; QC, quality control. The ellipse represents the 95% confidence interval of each group.
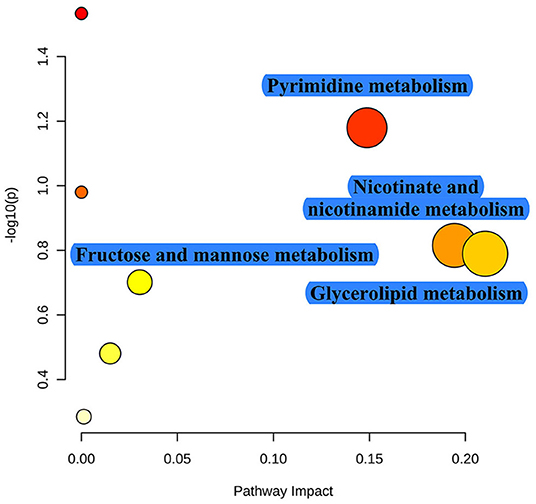
Figure 5. Pathway enrichment of these significant differential metabolites identified between the FA and TRF groups. The x-axis marks the pathway impact and the y-axis represents the pathway enrichment. Each node marks a pathway, with larger sizes and darker colors representing higher pathway enrichment and pathway impact values.
Correlations Between the Bacterial Genera, Bacterial Metabolites, and Differential Features in Colonic Digesta of Growing Pigs
Based on the Pearson correlation analysis, the differential microbiota at the genus level showed a significant correlation with bacterial metabolites in colonic digesta (Figure 6). Specifically, Lachnospiraceae XPB1014 group was negatively correlated with methylamine (r = −0.86, P < 0.001) and total SCFA (r = −0.58, P = 0.047), while it was positively correlated with pH (r = 0.70, P = 0.01). Lachnospiraceae NK3A20 group was negatively correlated with propionate (r = −0.66, P = 0.020), while it was positively correlated with lactate (r = 0.63, P = 0.029), isobutyrate (r = 0.64, P = 0.025), and butyrate (r = 0.58, P = 0.046). Mitsuokella was positively correlated with NH4-N (r = 0.59, P = 0.045), lactate (r = 0.72, P = 0.0082), and isovalerate (r = 0.58, P = 0.049). Coprococcus 2 was negatively correlated with pH (r = −0.69, P = 0.010), while it was positively correlated with spermidine (r = 0.58, P = 0.046), spermine (r = 0.69, P = 0.012), total biogenic amines (r = 0.66, P = 0.020), acetate (r = 0.64, P = 0.024), and total SCFA (r = 0.66, P = 0.020). Clostridium sensu stricto 1 was negatively correlated with methylamine (r = −0.64, P = 0.026) and butyrate (r = −0.58, P = 0.049). Eubacterium ruminantium group was negatively correlated with pH (r = −0.74, P = 0.0055), while it was positively correlated with spermine (r = 0.59, P = 0.045), total biogenic amines (r = 0.64, P = 0.026), acetate (r = 0.66, P = 0.018), butyrate (r = 0.60, P = 0.040), and total SCFA (r = 0.62, P = 0.030). Turicibacter was negatively correlated with butyrate (r = −0.63, P = 0.028). Lactobacillus was negatively correlated with putrescine (r = −0.67, P = 0.017) and cadaverine (r = −0.69, P = 0.013), while it was positively correlated with tyramine (r = 0.69, P = 0.013).
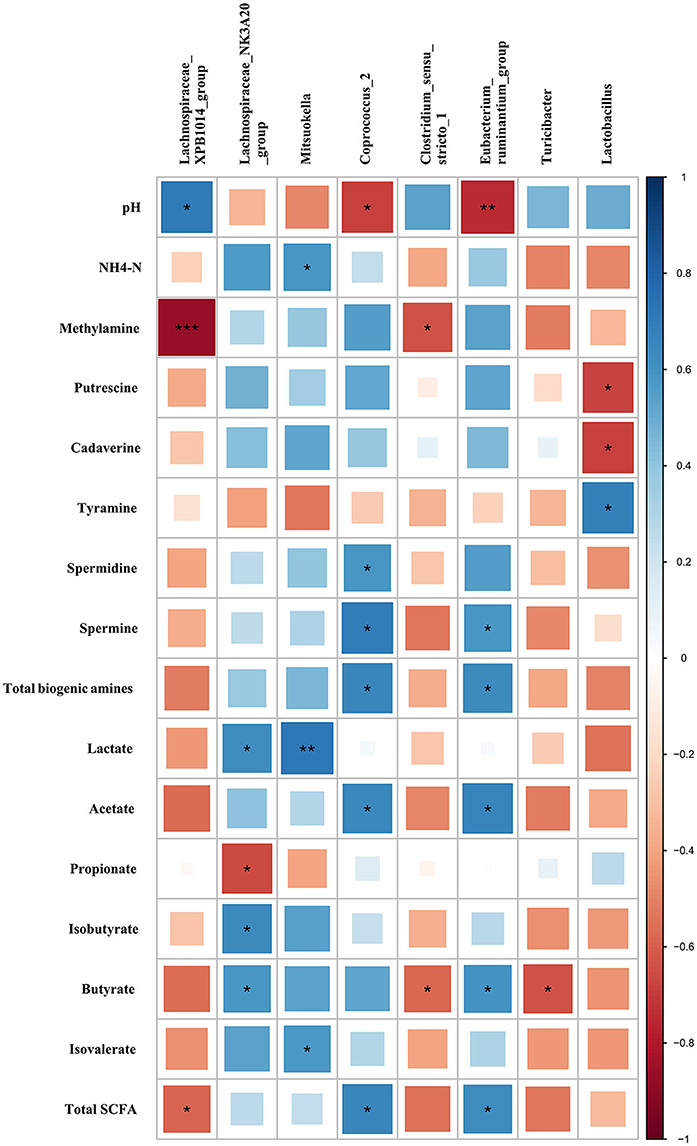
Figure 6. Correlations between the differential microbiota at the genus level with bacterial metabolites in colonic digesta of growing pigs. Each row in the graph represents a pH value and bacterial metabolite (including SCFAs, biogenic amines, lactate, and NH4-N), each column represents a genus, each square represents a Pearson correlation coefficient between a genus and a bacterial metabolite (or pH), while the area of each square represents the size of each correlation coefficient. Red color represents a negative correlation, while blue color represents a positive correlation. ***Indicates a significant difference between the free access group and time-restricted feeding group with P < 0.001; while **, *indicates significant difference with P < 0.01, and P < 0.05 respectively.
Based on the Pearson correlation analysis, the differential microbiota at the genus level showed a significant correlation with the significant differential features in colonic digesta (Figure 7). Specifically, Lachnospiraceae XPB1014 group was negatively correlated with cytidine (r = −0.70, P = 0.011), dihydroartemisinin (r = −0.61, P = 0.033), asparaginylglutamic acid (r = −0.80, P = 0.017), and monoglyceride (r = −0.69, P = 0.014), while it was positively correlated with serinyl-leucine (r = 0.80, P = 0.0019), glycylleucine (r = 0.68, P = 0.014), and methyl-β-galactopyranoside (r = 0.64, P = 0.025). Lachnospiraceae NK3A20 group was positively correlated with DL-pipecolic acid (r = 0.78, P = 0.0029). Campylobacter (r = −0.74, P = 0.0056), Prevotellaceae NK3B31 group (r = −0.74, P = 0.0060), Parabacteroides (r = −0.61, P = 0.0034), Prevotella 1 (r = −0.64, P = 0.0024), and Prevotellaceae UCG 001 (r = −0.73, P = 0.0070) were negatively correlated with D-2-aminobutyric acid. Mitsuokella was positively correlated with 1-methyladenosine (r = 0.79, P = 0.0020), N6,N6,N6-trimethyl-L-lysine (r = 0.66, P = 0.020), DL-pipecolic acid (r = 0.58, P = 0.047), dTMP (r = 0.67, P = 0.018), fructose-1-phosphate (r = 0.67, P = 0.018), and Lysophosphatidylethanolamine (r = 0.61, P = 0.034), while it was negatively correlated with serinyl-leucine (r = −0.60, P = 0.038) and glycylleucine (r = −0.67, P = 0.018). Turicibacter was positively correlated with urobilin (r = 0.62, P = 0.030). Clostridium sensu stricto 1 was negatively correlated with monoglyceride (r = −0.60, P = 0.040). Eubacterium ruminantium group was positively correlated with monoglyceride (r = 0.64, P = 0.024), while it was negatively correlated with hexadecanedioic acid (r = −0.58, P = 0.046). Lactobacillus was negatively correlated with 1-methyladenosine (r = −0.70, P = 0.011), adenosine (r = −0.61, P = 0.036), thymidine (r = −0.71, P = 0.010), and dTMP (r = −0.74, P = 0.0056), while it was positively correlated with niacinamide (r = 0.76, P = 0.004) and D-2-aminobutyric acid (r = 0.65, P = 0.02).
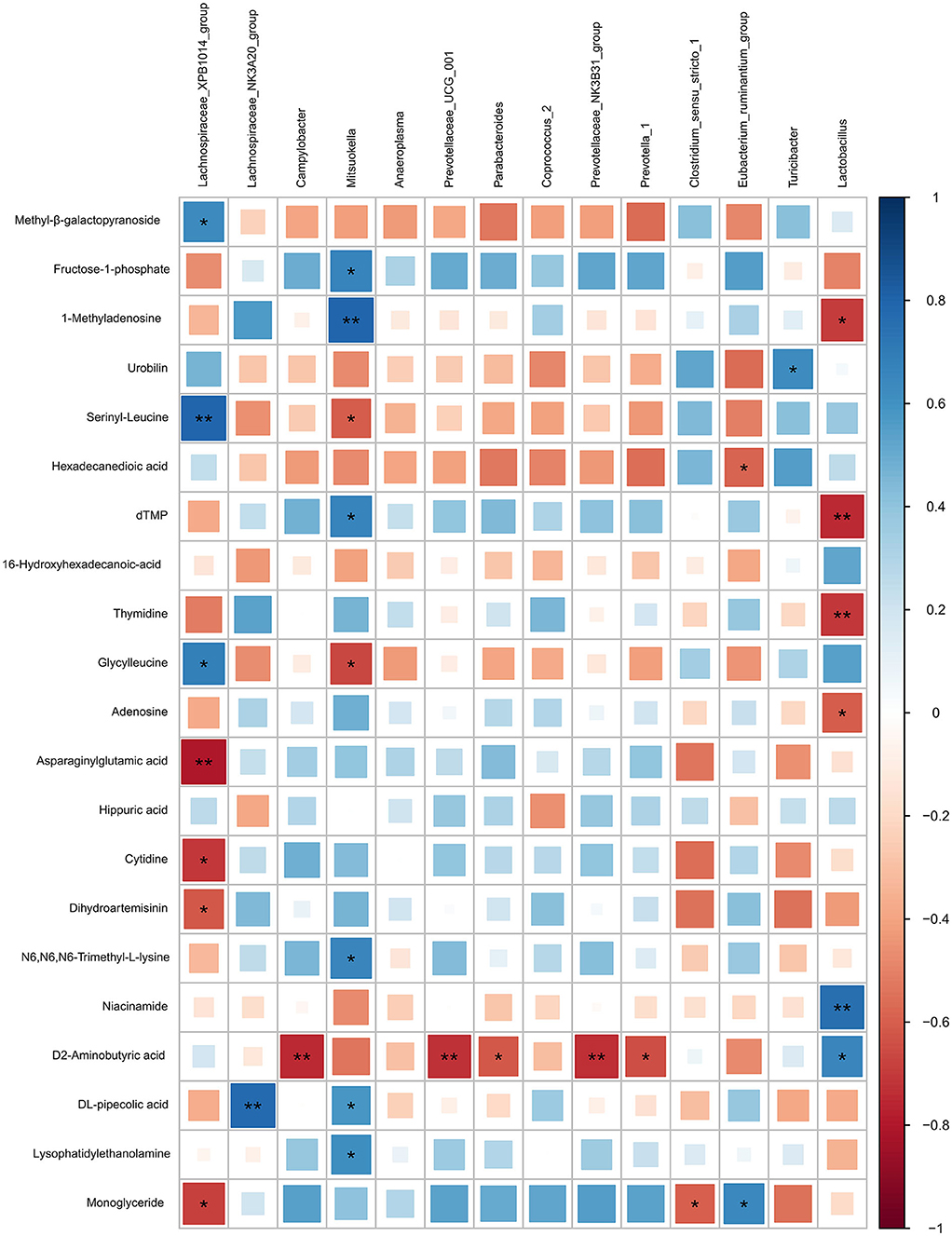
Figure 7. Correlation analysis between the differential microbiota at the genus level with the differential features identified from the LC-MS metabolome in colonic digesta of growing pigs. Each row in the graph represents the differential features, each column represents a genus, each square represents a Pearson correlation coefficient between a genus and a differential feature, while the area of each square represents the size of each correlation coefficient. Red color represents a negative correlation, while blue color represents a positive correlation. **Indicates a significant difference between the free access group and the time-restricted feeding group with P < 0.01; while *indicates a significant difference with P < 0.05.
Discussion
Growing evidence suggests that the nutrients (including fatty acids, amino acids, and saccharides) and the luminal environment (e.g., pH) shapes the microbiota community (23–25). In the present study, the overall composition of the colonic microbiota communities was not changed between the two groups, the most likely reason is that all pigs in both groups were fed with the same commercial feed. However, the Shannon index was significantly increased in the present study indicating that the TRF group improved the evenness of the microbial community. As reported, metabolite cross-feeding promotes a suboptimal community growth and shapes the species diversity in the gut microbiota (26). Therefore, the results may be contributed to the nutritional variations in the colonic lumen along with the different feeding rhythms. Under the free-access mode, a growing pig eats about seven times per day with an average feed intake of 260 g (27). However, our research found that the phased feed intake of growing pigs in a day underwent diurnal rhythmicity and peaked at 12:00–16:00 (unpublished data), which means that a fluctuation in nutrients may exist in the colonic lumen. By contrast, pigs in the TRF group were fed access to feed at identical intervals which may lead to a relative evenness of the nutrients, thus, affecting the microbiota community meanwhile.
A plethora of studies has reported that the bidirectional interactions between the host and the intestinal microbiota are tightly regulated to maintain health and homeostasis (28, 29). Gut microbiota plays pivotal roles in maintaining metabolic hemostasis including the metabolism of amino acids, carbohydrates, lipid, nucleotide, and vitamins (29). Vice versa, nutrients in the intestine taking by the host could domesticate the gut microbes meanwhile (28). Although the overall microbial composition was not considerably different, the TRF group significantly altered the relative abundance of certain taxa like Lactobacillus at the genus level. Surprisingly, we found that the TRF group has decreased the relative abundance of Lactobacillus, however, the decrease did not reduce the content of lactate. These results were inconsistent with those reported previously (30, 31). Zhang et al. (32) have reported that the oral administration of the Lactobacillus strain has increased the production of lactate. The following reasons may explain these results. Firstly, Bifidobacterium and Enterococcus, as well as Lactobacillus, could produce lactate (33). Thus, in line with Zhang et al. (32), we found that the relative abundance of Lactobacillus was not directly correlated with the concentration of lactate. Secondly, as a secondary metabolite, the lactate produced was possibly consumed simultaneously by yeasts or other aerobic bacteria in the FA group (34). Besides, the production efficiency of lactate during fermentation by different strains of Lactobacillus was not identical, especially with different substrates (35). Researchers have found that Mitsuokella had a positive effect on the serum free amino acids in weaned piglets (32), while in the present study, we found that Mitsuokella had a negative correlation with serinyl-leucine and glycylleucine. Eubacterium coprostanoligenes group has been reported to have a function of bio-transforming cholesterol to coprostanol which could further influence the fat metabolism of the host (36). Cholesterol has been shown to exert crucial physiological effects on animals (37). While in the present study, TRF has significantly reduced the relative abundance of Eubacterium coprostanoligenes group which possibly indicates that more cholesterol was utilized in the TRF group. Consistently, differential metabolites were enriched in fat-related metabolism pathways. Prevotella has been reported to associate with the increase of host feed intake (38). However, the feed intake was not affected by TRF in the present study (unpublished data). The possible reason was that although the relative abundance of Prevotellaceae at the family level was increased by TRF, it did not change the relative abundance of Prevotella at the genus level, but increased the relative abundance of Prevotella 1. Despite relating to several diseases (39), several members of Prevotellaceae were reported to produce succinate and could improve the glucose homeostasis status by activating intestinal gluconeogenesis (40). Thus, through promoting host health or energy metabolism, Prevotellaceae was reported to increase the feed efficacy in pigs (41). Herein, we found that TRF has significantly increased the relative abundance of Prevotellaceae at the family level which suggests that TRF would probably improve the production efficacy of swine production. As an evidence, the FCR was indeed improved by TRF in the present study (unpublished data).
Microbial metabolic products, mainly including SCFAs and biogenic amines, are thought to mediate the beneficial health effects of the intestinal microbial community (42). SCFAs were the main end-products of microorganisms fermenting carbohydrates, which are generally believed to have a benefit to host health (43). Specifically, butyrate, as one of the most important SCFAs, has extensive effects on improving immunity and intestinal health and promoting host metabolism (3, 44). In the present study, we found that TRF has increased the concentration of butyrate which indirectly reflected that TRF could improve host metabolism. Accordingly, the Lachnospiraceae NK3A20 group, which was positively correlated with butyrate, was found to be increased by TRF treatment (45). Besides, we also found that D2-aminobutyric acid, an unnatural chiral α-amino acid, in colonic digesta of growing pigs had excessive correlations with the differential bacterial genera. Unfortunately, there are currently few studies concerning the physiological function of D2-aminobutyric acid, thus, further research is still needed. Biogenic amines are produced by intestinal microbiota through the decarboxylation of aromatic or cationic amino acids (46). In the present study, we found that the TRF mode had a trend to decrease the concentration of tyramine. Furthermore, we found that Lactobacillus had a tight correlation with tyramine. Research has found that Lactobacillus can deaminate proteins (47). Moreover, researchers have found that 28% of the Lactobacillus produced tyramine (48). In the present study, TRF has increased the concentration of putrescine, spermidine, and spermine. Putrescine was reported to stimulate the synthesis of epithelial DNA and RNA (49). In line with this notion, pathway analysis indicates that TRF has increased the progress of pyrimidine metabolism. Furthermore, putrescine could mitigate intestinal atrophy by suppressing inflammatory responses in weanling piglets (50). Spermidine and spermine were reported to promote adipogenesis (51, 52), while cadaverine exerts protective effects on epithelial cells (53). Consistently, researchers have found that Lactobacillus was negatively correlated with cadaverine (25). The decreased production of biogenic amines may be contributed to the inhibitory effects of Lactobacillus on the growth of amine-positive bacteria (54). However, some genera of amine positive lactic acid bacteria strains, including Lactobacillus plantarum (FI8595) and Lactococcus lactis subsp. cremoris (MG 1363), had a crucial role on the increase of cadaverine and accumulation of putrescine (55). However, the biogenic amines function probably depends on the dose and physiological state of the host (56, 57).
Conclusions
In conclusion, the overall composition of gut microbiota community between the two groups was not influenced. However, TRF treatment significantly altered the relative abundance of certain families and genera including Lactobacillus, Eubacterium ruminantium group, Eubacterium coprostanoligenes group, Prevotella 1, and Clostridium sensu sticto 1. Furthermore, TRF treatment induced the gradient changes of metabolites in colonic digesta. Interestingly, the correlation results suggested that gradients of metabolites induced by TRF were correlated with the differential microbial genera indicating that the metabolites might mediate the effect of TFR on the gut microbiota. However, more research is needed to understand the benefits and risks of TRF on the health and metabolism of growing pigs.
Data Availability Statement
The data presented in the study are deposited in the repository of Sequence Read Archive, accession number: PRJNA715648.
Ethics Statement
The animal study was reviewed and approved by Nanjing Agricultural University Animal Care and Use Committee (Nanjing, Jiangsu Province, China) (SYXK2019-0066).
Author Contributions
YS and WZ conceived and designed the experiments. HW, PX, ZL, and YS performed the experiments and analyzed the data. HW and YS wrote the paper. All authors contributed to the article and approved the submitted version.
Funding
This work was supported by grants from the National Natural Science Foundation of China (31872362 and 32072688).
Conflict of Interest
The authors declare that the research was conducted in the absence of any commercial or financial relationships that could be construed as a potential conflict of interest.
Supplementary Material
The Supplementary Material for this article can be found online at: https://www.frontiersin.org/articles/10.3389/fvets.2021.681202/full#supplementary-material
Supplementary Figure 1. Rarefaction curve comparing the number of OTUs found in the 16S rDNA gene libraries from microbiota in the colon.
Supplementary Figure 2. Principal coordinate analysis (PcoA) of the colonic bacterial community of the growing pigs (n = 6). FA, free access group; TRF, time-restricted feeding group.
References
1. Batson KL, Calderón HI, Tokach MD, Woodworth JC, Goodband RD, Dritz SS, et al. Effect of coarse wheat bran and crude protein level in nursery pig diets without pharmacological levels of zinc oxide. Kansas Agr Exp Station. (2020) 6:4. doi: 10.4148/2378-5977.7985
2. Petry AL, Huntley NF, Bedford MR, Patience JF. Xylanase increased the energetic contribution of fiber and improved the oxidative status, gut barrier integrity, and growth performance of growing pigs fed insoluble corn-based fiber. J Anim Sci. (2020) 98:233. doi: 10.1093/jas/skaa233
3. Wang H, Ren E, Xiang X, Su Y, Zhu W. Dynamic changes in serum metabolomic profiles of growing pigs induced by intravenous infusion of sodium butyrate. Metabolites. (2020) 10:20. doi: 10.3390/metabo10010020
4. Zhuo Y, Cao M, Gong Y, Tang L, Jiang X, Li Y, et al. Gut microbial metabolism of dietary fibre protects against high energy feeding induced ovarian follicular atresia in a pig model. Br J Nutr. (2021) 125:38–49. doi: 10.1017/S0007114520002378
5. Liu J, Liu Z, Chen L, Zhang H. iTRAQ-based proteomic analysis reveals alterations in the liver induced by restricted meal frequency in a pig model. Nutrition. (2016) 32:871–6. doi: 10.1016/j.nut.2016.01.020
6. Xie C, Duan X, Long C, Wu X. Hepatic lipid metabolism is affected by a daily 3-meal pattern with varying dietary crude protein with a pig model. Anim Nutr. (2020) 6:16–23. doi: 10.1016/j.aninu.2019.11.001
7. Rothschild J, Hoddy KK, Jambazian P, Varady KA. Time-restricted feeding and risk of metabolic disease: a review of human and animal studies. Nutr Rev. (2014) 72:308–18. doi: 10.1111/nure.12104
8. Zeb F, Wu X, Chen L, Fatima S, Chen A, Xu C, et al. Time-restricted feeding is associated with changes in human gut microbiota related to nutrient intake. Nutrition. (2020) 78:110797. doi: 10.1016/j.nut.2020.110797
9. Zarrinpar A, Chaix A, Yooseph S, Panda S. Diet and feeding pattern affect the diurnal dynamics of the gut microbiome. Cell Metab. (2014) 20:1006–17. doi: 10.1016/j.cmet.2014.11.008
10. Gardiner GE, Metzler-Zebeli BU, Lawlor PG. Impact of intestinal microbiota on growth and feed efficiency in pigs: a review. Microorganisms. (2020) 8:1886. doi: 10.3390/microorganisms8121886
11. Tremaroli V, Bäckhed F. Functional interactions between the gut microbiota and host metabolism. Nature. (2012) 489:242–9. doi: 10.1038/nature11552
12. Zhou L, Fang L, Sun Y, Su Y, Zhu W. Effects of the dietary protein level on the microbial composition and metabolomic profile in the hindgut of the pig. Anaerobe. (2016) 38:61–9. doi: 10.1016/j.anaerobe.2015.12.009
13. Lin S, Yang X, Yuan P, Yang J, Wang P, Zhong H, et al. Undernutrition shapes the gut microbiota and bile acid profile in association with altered gut-liver FXR signaling in weaning pigs. J Agr Food Chem. (2019) 67:3691–701. doi: 10.1021/acs.jafc.9b01332
14. Huang L, Ren P, Ouyang Z, Wei T, Kong X, Li T, et al. Effect of fermented feed on growth performance, holistic metabolism and fecal microbiota in weanling piglets. Anim Feed Sci Technol. (2020) 266:114505. doi: 10.1016/j.anifeedsci.2020.114505
15. Walters EM, Prather RS. Advancing swine models for human health and diseases. Mo Med. (2013) 110:212–5.
16. Xiao L, Estelle J, Kiilerich P, Ramayo-Caldas Y, Xia Z, Feng Q, et al. A reference gene catalogue of the pig gut microbiome. Nat Microbiol. (2016) 1:1–6. doi: 10.1038/nmicrobiol.2016.161
17. Dai ZL, Zhang J, Wu G, Zhu WY. Utilization of amino acids by bacteria from the pig small intestine. Amino Acids. (2010) 39:1201–15. doi: 10.1007/s00726-010-0556-9
18. Zhang Y, Yu K, Chen H, Su Y, Zhu W. Caecal infusion of the short-chain fatty acid propionate affects the microbiota and expression of inflammatory cytokines in the colon in a fistula pig model. Microb Biotechnol. (2018) 11:859–68. doi: 10.1111/1751-7915.13282
19. Chong J, Liu P, Zhou G, Xia J. Using microbiome analyst for comprehensive statistical, functional, and meta-analysis of microbiome data. Nat Protoc. (2020) 15:799–821. doi: 10.1038/s41596-019-0264-1
20. Wang XF, Mao SY, Liu JH, Zhang LL, Cheng YF, Jin W. Effect of the gynosaponin on methane production and microbe numbers in a fungus-methanogen co-culture. J Anim Feed Sci. (2011) 20:272–84. doi: 10.22358/jafs/66184/2011
21. Shen J, Liu Z, Yu Z, Zhu W. Monensin and nisin affect rumen fermentation and microbiota differently in vitro. Front Microbiol. (2017) 8:1111. doi: 10.3389/fmicb.2017.01111
22. Yang YX, Mu CL, Zhang JF, Zhu WY. Determination of biogenic amines in digesta by high performance liquid chromatography with precolumn dansylation. Anal Lett. (2014) 47:1290–8. doi: 10.1080/00032719.2013.871550
23. Agans R, Gordon A, Kramer DL, Perez-Burillo S, Rufián-Henares JA, Paliy O. Dietary fatty acids sustain the growth of the human gut microbiota. Appl Environ Microbiol. (2018) 84:e0152518. doi: 10.1128/AEM.01525-18
24. Ma N, Guo P, Zhang J, He T, Kim SW, Zhang G, et al. Nutrients mediate intestinal bacteria–mucosal immune crosstalk. Front Immunol. (2018) 9:5. doi: 10.3389/fimmu.2018.00005
25. Yu M, Li Z, Chen W, Rong T, Wang G, Ma X. Microbiome-metabolomics analysis investigating the impacts of dietary starch types on the composition and metabolism of colonic microbiota in finishing pigs. Front Microbiol. (2019) 10:1143. doi: 10.3389/fmicb.2019.01143
26. Henson MA, Phalak P. Suboptimal community growth mediated through metabolite crossfeeding promotes species diversity in the gut microbiota. PLoS Comput Biol. (2018) 14:e1006558. doi: 10.1371/journal.pcbi.1006558
27. Hyun Y, Ellis M, McKeith FK, Wilson ER. Feed intake pattern of group-housed growing-finishing pigs monitored using a computerized feed intake recording system. J Anim Sci. (1997) 75:1443–51. doi: 10.2527/1997.7561443x
28. Shin D, Chang SY, Bogere P, Won K, Choi JY, Choi YJ, et al. Beneficial roles of probiotics on the modulation of gut microbiota and immune response in pigs. PLoS ONE. (2019) 14:e0220843. doi: 10.1371/journal.pone.0220843
29. Meng Q, Luo Z, Cao C, Sun S, Ma Q, Li Z, et al. Weaning alters intestinal gene expression involved in nutrient metabolism by shaping gut microbiota in pigs. Front Microbiol. (2020) 11:694. doi: 10.3389/fmicb.2020.00694
30. Jin X, Chen W, Chen H, Chen W, Zhong Q. Combination of lactobacillus plantarum and Saccharomyces cerevisiae DV10 as starter culture to produce mango slurry: microbiological, chemical parameters and antioxidant activity. Molecules. (2019) 24:4349. doi: 10.3390/molecules24234349
31. Tian S, Wang J, Yu H, Wang J, Zhu W. Changes in ileal microbial composition and microbial metabolism by an early-life galacto-oligosaccharides intervention in a neonatal porcine model. Nutrients. (2019) 11:1753. doi: 10.3390/nu11081753
32. Zhang D, Liu H, Wang S, Zhang W, Wang J, Tian H, et al. Fecal microbiota and its correlation with fatty acids and free amino acids metabolism in piglets after a Lactobacillus strain oral administration. Front microbiol. (2019) 10:785. doi: 10.3389/fmicb.2019.00785
33. Black F, Einarsson K, Lidbeck A, Orrhage K, Nord CE. Effect of lactic acid producing bacteria on the human intestinal microflora during ampicillin treatment. J Infect Dis. (1991) 23:247–54. doi: 10.3109/00365549109023408
34. Hao W, Wang HL, Ning TT, Yang FY, Xu CC. Aerobic stability and effects of yeasts during deterioration of non-fermented and fermented total mixed ration with different moisture levels. Asian-australas J Anim Sci. (2015) 28:816–26. doi: 10.5713/ajas.14.0837
35. Othman M, Ariff AB, Rios-Solis L, Halim M. Extractive fermentation of lactic acid in lactic acid bacteria cultivation: a review. Front Microbiol. (2017) 8:2285. doi: 10.3389/fmicb.2017.02285
36. Freier TA, Beitz DC, Li L, Hartman PA. Characterization of eubacterium coprostanoligenes sp. nov., a cholesterol-reducing anaerobe. Int J Syst Evol Microbiol. (1994) 44:137–42. doi: 10.1099/00207713-44-1-137
37. Sáiz-Vazquez O, Puente-Martínez A, Ubillos-Landa S, Pacheco-Bonrostro J, Santabárbara J. Cholesterol and Alzheimer's disease risk: a meta-meta-analysis. Brain Sci. (2020) 10:386. doi: 10.3390/brainsci10060386
38. Yang H, Yang M, Fang S, Huang X, He M, Ke S, et al. Evaluating the profound effect of gut microbiome on host appetite in pigs. BMC Microbiol. (2018) 18:1–10. doi: 10.1186/s12866-018-1364-8
39. Brinkman BM, Hildebrand F, Kubica M, Goosens D, Del Favero J, Declercq W, et al. Caspase deficiency alters the murine gut microbiome. Cell Death Dis. (2011) 2:e220. doi: 10.1038/cddis.2011.101
40. De Vadder F, Kovatcheva-Datchary P, Zitoun C, Duchampt A, Backhed F, Mithieux G. Microbiota-produced succinate improves glucose homeostasis via intestinal gluconeogenesis. Cell Metab. (2016) 24:151–7. doi: 10.1016/j.cmet.2016.06.013
41. Quan J, Cai G, Yang M, Zeng Z, Ding R, Wang X, et al. Exploring the fecal microbial composition and metagenomic functional capacities associated with feed efficiency in commercial DLY pigs. Front microbiol. (2019) 10:52. doi: 10.3389/fmicb.2019.00052
42. Den Besten G, Van Eunen K, Groen AK, Venema K, Reijngoud DJ, Bakker BM. The role of short-chain fatty acids in the interplay between diet, gut microbiota, and host energy metabolism. J Lipid Res. (2013) 54:2325–40. doi: 10.1194/jlr.R036012
43. Tan Z, Dong W, Ding Y, Ding X, Zhang Q, Jiang L. Changes in cecal microbiota community of suckling piglets infected with porcine epidemic diarrhea virus. PLoS ONE. (2019) 14:e0219868. doi: 10.1371/journal.pone.0219868
44. Bedford A, Gong J. Implications of butyrate and its derivatives for gut health and animal production. Anim Nutr. (2018) 4:151–9. doi: 10.1016/j.aninu.2017.08.010
45. Xia G, Sun J, Fan Y, Zhao F, Ahmed G, Jin Y, et al. β-sitosterol attenuates high grain diet-induced inflammatory stress and modifies rumen fermentation and microbiota in sheep. Animals. (2020) 10:171. doi: 10.3390/ani10010171
46. Kabouridis PS, Lasrado R, McCallum S, Chng SH, Snippert HJ, Clevers H, et al. The gut microbiota keeps enteric glial cells on the move; prospective roles of the gut epithelium and immune system. Gut Microbes. (2015) 6:398–403. doi: 10.1080/19490976.2015.1109767
47. Cayré ME, Vignolo G, Garro O. Modeling lactic acid bacteria growth in vacuum-packaged cooked meat emulsions stored at three temperatures. Food Microbiol. (2003) 20:561–6. doi: 10.1016/S0740-0020(02)00154-5
48. Curiel JA, Ruiz-Capillas C, de Las Rivas B, Carrascosa AV, Jiménez-Colmenero F, Muñoz R. Production of biogenic amines by lactic acid bacteria and enterobacteria isolated from fresh pork sausages packaged in different atmospheres and kept under refrigeration. Meat Sci. (2011) 88:368–73. doi: 10.1016/j.meatsci.2011.01.011
49. Girdhar SR, Barta JR, Santoyo FA, Smith TK. Dietary putrescine (1, 4-diaminobutane) influences recovery of turkey poults challenged with a mixed coccidial infection. J Nutr. (2006) 136:2319–24. doi: 10.1093/jn/136.9.2319
50. Liu B, Jiang X, Cai L, Zhao X, Dai Z, Wu G, et al. Putrescine mitigates intestinal atrophy through suppressing inflammatory response in weanling piglets. J Anim Sci Biotechno. (2019) 10:1–14. doi: 10.1186/s40104-019-0379-9
51. Hyvönen MT, Koponen T, Weisell J, Pietilä M, Khomutov AR, Vepsäläinen J, et al. Spermidine promotes adipogenesis of 3T3-L1 cells by preventing interaction of ANP32 with HuR and PP2A. Biochem. (2013) 453:467–74. doi: 10.1042/BJ20130263
52. He L, Han M, Qiao S, He P, Li D, Li N, et al. Soybean antigen proteins and their intestinal sensitization activities. Curr Protein Pept Sci. (2015) 16:613–21. doi: 10.2174/1389203716666150630134602
53. McCormick BA, Fernandez MI, Siber AM, Maurelli AT. Inhibition of Shigella flexneri-induced transepithelial migration of polymorphonuclear leucocytes by cadaverine. Cell Microbiol. (1999) 1:143–56. doi: 10.1046/j.1462-5822.1999.00014.x
54. Xie C, Wang HH, Deng SL, Xu XL. The inhibition of cell-free supernatant of Lactobacillus plantarum on production of putrescine and cadaverine by four amine-positive bacteria in vitro. LWT-Food Sci Techno. (2016) 67:106–11. doi: 10.1016/j.lwt.2015.11.028
55. Kuley E, Balikci E, Özogul I. Gökdogan S, Özogul F. Stimulation of cadaverine production by foodborne pathogens in the presence of Lactobacillus, Lactococcus, Streptococcus spp. J Food Sci. (2012) 77:M650–8. doi: 10.1111/j.1750-3841.2012.02825.x
56. Amendola R, Cervelli M, Fratini E, Polticelli F, Sallustio DE, Mariottini P. Spermine metabolism and anticancer therapy. Curr Cancer Drug Targets. (2009) 9:118–30. doi: 10.2174/156800909787580935
Keywords: growing pigs, metabolome, microbiome, time-restricted feeding, microbial metabolites
Citation: Wang H, Xia P, Lu Z, Su Y and Zhu W (2021) Metabolome-Microbiome Responses of Growing Pigs Induced by Time-Restricted Feeding. Front. Vet. Sci. 8:681202. doi: 10.3389/fvets.2021.681202
Received: 27 March 2021; Accepted: 20 May 2021;
Published: 22 June 2021.
Edited by:
Claudio Forte, University of Turin, ItalyReviewed by:
Padraig Strappe, Central Queensland University, AustraliaCongying Chen, Jiangxi Agricultural University, China
Copyright © 2021 Wang, Xia, Lu, Su and Zhu. This is an open-access article distributed under the terms of the Creative Commons Attribution License (CC BY). The use, distribution or reproduction in other forums is permitted, provided the original author(s) and the copyright owner(s) are credited and that the original publication in this journal is cited, in accordance with accepted academic practice. No use, distribution or reproduction is permitted which does not comply with these terms.
*Correspondence: Yong Su, eW9uZy5zdSYjeDAwMDQwO25qYXUuZWR1LmNu