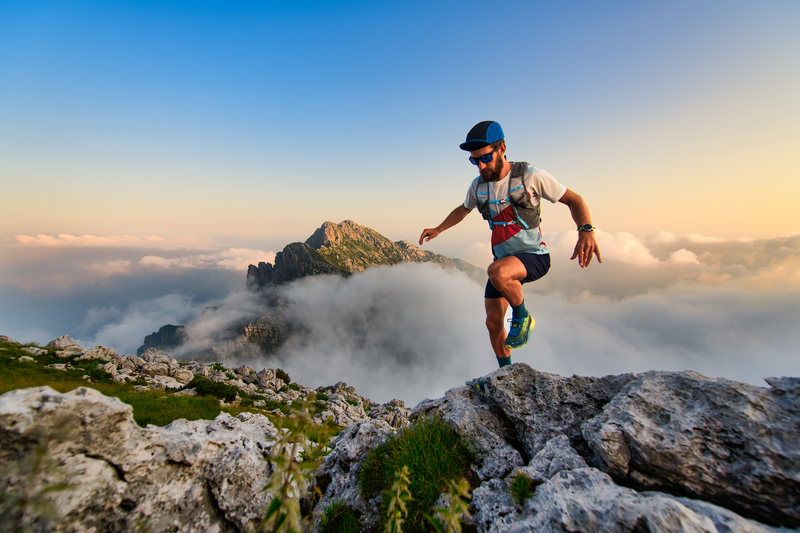
94% of researchers rate our articles as excellent or good
Learn more about the work of our research integrity team to safeguard the quality of each article we publish.
Find out more
ORIGINAL RESEARCH article
Front. Vet. Sci. , 05 July 2021
Sec. Veterinary clinical, anatomical, and comparative pathology
Volume 8 - 2021 | https://doi.org/10.3389/fvets.2021.666486
The giant panda is one of the rarest animals in the world. Skin diseases seriously endanger the health of giant panda and are considered the second major cause of its morbidity. Skin microbiota is a complex ecosystem, and the community structure and the pathogenic potential of bacteria on giant panda skin remain largely unclear. In order to understand the skin bacterial flora of captive giant pandas, the microbiota in giant panda skin samples collected during different seasons was profiled via 16S rRNA gene sequencing. In total, 522 genera from 53 bacterial phyla were detected, with Proteobacteria (40.5%), Actinobacteria (23.1%), Firmicutes (21.1%), Bacteroidetes (9.5%), Cyanobacteria (2.1%), and Thermi (1.2%) as the predominant phyla and Streptococcus (13.9%), Acinetobacter (9.2%), Staphylococcus (2.9%), Pseudomonas (5.9%), Dermacoccus (4.8%), Brachybacterium (2.9%), Escherichia (2.7%), Chryseobacterium (2.1%), Arthrobacter (1.6%), Kocuria (1.5%), Psychrobacter (1.2%), Deinococcus (1.1%), and Flavobacterium (1.1%) as the predominant genera. The results indicated that the diversity was lower in winter than in other seasons and higher in autumn than in other seasons, and the abundance in spring was significantly higher than that in other seasons. Several skin disease-associated bacteria were detected as opportunists in the skin microbiota of healthy giant pandas. In this study, the results indicated that the high diversity and abundance of the skin bacteria may have enhanced the occurrence of skin disease in autumn and spring and that skin disease-associated bacteria are the normal components of the skin microbiota.
The giant panda is one of the rarest animals in the world (1). The skin of the giant panda hosts millions of bacteria, fungi, and viruses that make up its skin microbiome. Skin microbes play a vital role in preventing invading pathogens (2). The outermost layer of the skin consists of a stratum corneum, which is rich in lipids and proteins, with hair follicles and glands interspersed among them. These hair follicles and glands secrete lipids, antibacterial peptides, enzymes, salts, and many other compounds (3). Protecting the host from invasion of pathogenic microorganisms is one of the most important functions of the skin (4). Although most microorganisms living on the skin are harmless, or even beneficial, some resident microorganisms are potentially pathogenic under certain conditions (5). However, sufficient knowledge regarding the community structure and pathogenic potential of bacteria on giant panda skin is currently lacking. Skin disease is a refractory disease that mainly damages the skin and hair of the giant panda, thereby affecting its growth and appearance. It reduces the immunity of the giant panda and increases its morbidity and mortality due to other diseases (6).
The gut microbial diversity of giant pandas has been previously described (7). However, the microbial community structures of giant panda skin and fur are yet to be fully researched. Studies that have been conducted on the skin microbial community of giant pandas so far have focused mainly on traditional culture-based methods. Several bacteria and fungi have been found to be conditionally associated with skin disease of giant pandas, including Cladosporium cladosporioides (8), Pestalotiopsis hainanensis (9), Microsporum gypseum (6), Streptococcus, and Staphylococcus (10). Traditionally, skin microbial communities have been explored using culture methods. However, because culturing selects microbes that thrive under artificial growth conditions, it underestimates the overall diversity of the community (11). Therefore, in order to avoid bias due to culturing and to capture the complete diversity of microbiomes, researchers use more advanced next-generation sequencing and bioinformatics, which are culture-independent methods (ribosomal DNA sequencing) that are widely used to characterize the microbiota of both humans and animals (12, 13). These original sequencing methods use sequence variations in conservative taxonomic markers as molecular fingerprints to identify members of microbial communities. Generally, 16S ribosomal RNA (rRNA) genes are used for bacteria (14).
In order to investigate the bacterial flora inhabiting captive giant panda skin, the bacterial flora of giant panda skin samples collected during different seasons were analyzed via 16S rRNA gene sequencing. This study is the first to reveal the diversity of skin bacterial communities in captive giant pandas. It described the bacterial flora on giant pandas during different seasons and discussed the possible effects of these flora on the pandas, which provided insights into controlling giant panda skin disease.
Samples were collected from clinically healthy giant pandas (24 females and 23 males) at the China Conservation and Research Center for the Giant Panda (Ya'an, China). The pandas were housed in dozens of independent enclosures on a mountain where an environment similar to that of wild pandas with heavy broad-leaved forests, green bamboos, and thorns was maintained. Each enclosure, which included an open outdoor area and a closed indoor area, housed one or two giant pandas. The pandas were free to move around indoors and outdoors in their own enclosures, but rarely encountered pandas from other enclosures. All pandas were fed a diet of ~10% steamed cornbread and fruits and 90% bamboo shoots and allowed access to drinking water ad libitum. Samples were collected from the upper thoracic limb, head, or dorsum via manually scraping appropriate amounts of hair and dander from the skin surface of an ~5.0 × 5.0 cm area, following the removal of most hair. Sampling spots were located on the front part of the body and the surface layer of the skin was avoided, in order to minimize the artificial impact caused by the environment on the skin microbiota. The personnel who performed the sampling wore sterile protective clothing, hats, masks, and latex gloves. Samples were quickly placed in sterile plastic sample bags and shipped to the laboratory on ice within 2 h and stored in a −80°C freezer. Sampling was conducted in March, June, September, and December, representing the four seasons of spring, summer, fall, and winter, respectively. In total, 9 samples were collected for spring, 10 samples were collected for summer, 18 samples were collected for autumn, and 10 samples were collected for winter (Supplementary Table 1).
Total genomic DNA was extracted from samples using the CTAB/SDS method (15). The V4 regions of 16S rRNA genes from all 47 samples were amplified with specific primers (16S V4: 515F: 5′-GTGCCAGCMGCCGCGGTAA-3′, 806R: 5′-GGACTACHVGGGTWTCTAAT-3′), using a Phusion® High-Fidelity PCR Master Mix kit (New England Biolabs, Ipswich, MA, USA). Sequencing libraries were generated and barcoded using a TruSeq® DNA PCR-Free Sample Preparation Kit (Illumina, San Diego, CA, USA) following the instructions of the manufacturer. Quality of the library was assessed via a Qubit@ 2.0 Fluorometer (Thermo Scientific, Waltham, MA, USA) and an Agilent Bioanalyzer 2100 system. The amplicons were sequenced on an Illumina HiSeq 2500 platform with 250-bp paired-end reads.
Adapters and low-quality reads were removed by preprocessing raw reads according to the quality control process of QIIME tags, using the following procedures (16): filtered read pairs were merged using FLASH (V1.2.7, http://ccb.jhu.edu/software/FLASH/) (17); OTUs were assigned at 97% sequence similarity using Uparse software (Uparse v7.0.1001, http://drive5.com/uparse/) (18); and they were annotated against the Silva Database (http://www.arb-silva.de/) (19) using the RDP classifier algorithm (Version 2.2, http://sourceforge.net/projects/rdp-classifier/) (20). Subsequent analysis of alpha diversity and beta diversity was performed using QIIME (Version 1.9.1) and displayed via R software (Version 2.15.3). Linear discriminant analysis coupled with effect size (LEfSe) was performed to identify bacterial taxa differentially represented between seasons at different levels (21).
The sample collection and all the experiments were performed in a style to minimize risk to the giant pandas. All experimental protocols of this study were approved by the Sichuan Agricultural University Animal Ethics Committee and China Conservation and Research Center for the Giant Panda Animal Ethics Committee.
The amplicons of the 16S rRNA V4 region of 47 samples were sequenced using the Illumina platform. After quality filtering, 2,531,535 reads of 16S rDNA were obtained, and the reads for each sample were between 40,763 and 66,561. Filtered reads were then subjected to taxonomic classification. Following the removal of singleton OTUs (operational taxonomic units), a total of 8,818 OTUs were obtained from all samples and 1,125–2,794 OTUs were identified for each sample. The samples were then rarefied to 40,763 reads for the subsequent analysis of alpha diversity and beta diversity. The rarefaction curves started to flatten at 6,803 sequences (Figure 1A). Good's coverage index (the proportion of non-singleton OTUs in the dataset as a measure of overall OTU sampling completion was used to assess the adequacy of sampling) estimated that 97.7–99.2% of the total species were represented at the rarefied depth in each sample (Figure 1B). These results indicated that the sequencing depth was sufficient to represent the diversity in each sample.
Figure 1. The rarefaction curves started to flatten at a sequence number of 40,000 (A). Good's coverage index estimated that 97.7–99.2% of all species were represented in each sample (B).
Samples were grouped via a beta diversity matrix. PCoA analysis, based on unweighted UniFrac distance metrics of beta diversity, revealed that samples clustered together according to seasons (Figure 2). ANOSIM analysis of the samples from different seasons showed that a skin microbiome corresponding to one season was significantly different from those corresponding to other seasons (Supplementary Table 2), indicating that the skin microbiomes of giant pandas are associated with the seasons. Multiple algorithms such as ACE (abundance-based coverage estimator, a non-parametric asymptotic species richness estimator, to estimate the number of missing species in a sample; the higher values of the ACE estimator indicate higher community richness) and Shannon (entropy estimator, taking into account the species richness and evenness of the community, which varied from zero for communities with a single taxon; the higher value of the Shannon index indicates higher community diversity) were used to estimate the alpha diversity (Figures 3A,B). The ACE estimator was the highest in samples from spring, followed by autumn, winter, and summer (Figures 3A,B). However, although differences between the samples from autumn, winter, and summer were not significant, samples from the other three seasons were all significantly different from the samples from spring (Figures 3A,B). The Shannon index was the highest in the samples from autumn, followed by spring, summer, and winter, in that order (Figures 3A,B). Again, although differences between the samples from autumn, winter, and summer were not significant, samples from the three seasons were all significantly different from the samples from spring (Figures 3A,B). These results indicated that species diversity in winter was lower than that in other seasons, while that in autumn was higher than that in other seasons. Species richness in spring was significantly higher than that in other seasons.
Figure 2. Beta diversity of giant panda (Ailuropoda melanoleuca) bacterial skin microbiota from different seasons. The principal coordinate analysis based on unweighted UniFrac metrics indicates that giant panda (A. melanoleuca) skin bacterial microbiota is associated with seasons. The close clustering of the samples from each season demonstrates the high phylogenetic similarities of the respective microbiota.
Figure 3. Species richness and diversity of giant panda (A. melanoleuca) skin bacterial microbiota measured by 16S rDNA sequencing. Comparison of alpha diversity between seasons are shown in (A) (ACE) and (B) (Shannon), respectively (Wilcoxon rank-sum test; *p < 0.05, **p < 0.01, ***p < 0.001).
The 8,818 OTUs obtained from all 47 samples were classified into 53 phyla, 139 classes, 243 orders, 312 families, and 522 genera (Supplementary Table 3). At the phylum level, Proteobacteria (40.5%), Firmicutes (21.1%), Actinobacteria (23.1%), Bacteroidetes (9.5%), Cyanobacteria (2.1%), and Thermi (1.2%) were the predominant taxa (>1%) in all samples, on average (Figure 4A). At the genus level, Streptococcus (13.9%), Staphylococcus (2.9%), Acinetobacter (9.2%), Pseudomonas (5.9%), Dermacoccus (4.8%), Brachybacterium (2.9%), Escherichia (2.7%), Chryseobacterium (2.1%), Arthrobacter (1.6%), Kocuria (1.5%), Psychrobacter (1.2%), Deinococcus (1.1%), and Flavobacterium (1.1%) were the predominant genera (>1%) in all samples, on average (Figure 4B). The relative abundance of the taxa varied across different seasons. With respect to the predominant phyla, Proteobacteria (38%) was the main phylum, followed by Actinobacteria (27.1%), Firmicutes (18.8%), Bacteroidetes (9.5%), Cyanobacteria (2.4%), and Acidobacteria (1.4%) in the spring; Proteobacteria (36.3%) was the main phylum, followed by Actinobacteria (29%), Firmicutes (22%), Bacteroidetes (6.8%), Thermi (2.5%), and Cyanobacteria (1.3%) in the summer; Proteobacteria accounted for 45.8%, followed by Actinobacteria (21.5%), Firmicutes (14.8%), Bacteroidetes (12.7%), Cyanobacteria (1.5%), and Thermi (1.3%) in the autumn; and Proteobacteria (37.5%) was the main phylum, followed by Firmicutes (33.5%), Actinobacteria (16.7%), Bacteroidetes (6.6%), and Cyanobacteria (3.6%) in the winter. With respect to the predominant genera, the genera showing a relative abundance of more than 1% were Streptococcus (13.1%), Pseudomonas (6.9%), Dermacoccus (6.3%), Acinetobacter (4.3%), Brachybacterium (4.1%), Arthrobacter (3.5%), Staphylococcus (3.1%), Chryseobacterium (3.0%), Escherichia (1.7%), Flavobacterium (1.7%), Psychrobacter (1.3%), and Kocuria (1.1%) in the spring; Streptococcus (17.1%), Acinetobacter (10.2%), Dermacoccus (7%), Escherichia (4.4%), Pseudomonas (3.9%), Kocuria (3.5%), Brachybacterium (2.5%), Deinococcus (2.4%), Staphylococcus (1.5%), Paracoccus (1.4%), and Chryseobacterium (1.2%) in the summer; Acinetobacter (11.5%), Pseudomonas (6.7%), Streptococcus (5.3%), Staphylococcus (4.2%), Dermacoccus (4.1%), Brachybacterium (2.6%), Escherichia (2.5%), Chryseobacterium (2.2%), Deinococcus (1.3%), Flavobacterium (1.3%), Sphingobacterium (1.2%), Arthrobacter (1.1%), Kocuria (1.1%), Psychrobacter (1.1%), Pediococcus (1%), and Aeromonas (1.0%) in the autumn; and Streptococcus (27.1%), Acinetobacter (8.6%), Pseudomonas (5.3%), Brachybacterium (2.8%), Dermacoccus (2.7%), Escherichia (2.1%), Psychrobacter (2.0%), Chryseobacterium (2.0%), Staphylococcus (1.6%), Arthrobacter (1.4%), Corynebacterium (1.3%), Brevibacterium (1.1%), Flavobacterium (1.1%), and Clostridium (1.0%) in the winter.
Figure 4. Stacked bar plots showing average percentages of giant panda (A. melanoleuca) skin bacterial populations from different seasons. (A) Skin bacterial microbiota composition at the phyla level (top 20). (B) Skin bacterial microbiota composition at the genera level (top 20).
Season-related genera were identified via linear discriminant analysis (LDA) and effect size analysis. Five genera were associated with winter (n = 1), summer (n = 2), spring (n = 1), and autumn (n = 1), respectively (Figure 5A). Arthrobacter was associated with spring, Kocuria and Deinococcus were associated with summer, Acinetobacter was associated with autumn, and Streptococcus was associated with winter (Figures 5A,B). Currently, the reports pertaining to bacterial skin diseases in giant pandas are scant, including Streptococcus and Staphylococcus (10). However, we detected the presence of related bacteria that may cause skin diseases in humans and animals, including Streptococcus, Staphylococcus, Acinetobacter, Pseudomonas, Arthrobacter, and Propionibacterium (Figure 5B). Streptococcus was more abundant than Staphylococcus, Acinetobacter, Pseudomonas, Arthrobacter, and Propionibacterium. The average relative abundance of Streptococcus was 13.9%, while those of Staphylococcus, Acinetobacter, Pseudomonas, Arthrobacter, and Propionibacterium were 2.9, 9.2, 5.9, 1.6, and 0.02%, respectively. Streptococcus, Acinetobacter, and Arthrobacter were season-related, where Arthrobacter was more abundant in the spring, Acinetobacter was more abundant in the autumn, and Streptococcus was more abundant in the winter. However, there were no significant variations between Pseudomonas and Propionibacterium during different seasons (Figure 5B).
Figure 5. Season-related genera and abundance variation of skin disease-associated bacteria. (A) Skin bacteria associated with seasons identified via linear discriminant analysis coupled with effect size (LEfSe) using default parameters. (B) Heatmap showing the relative abundance of season-related genera (denoted by “*”) and skin disease-associated bacteria (only taxa with a defined genus are shown).
The skin microbiome hosts a wide variety of microorganisms, including bacteria, viruses, and fungi. Skin microbiota protects against harmful microbes, maintains skin homeostasis, and informs our immune system (22). On the other hand, microbial dysbiosis may cause or exacerbate skin diseases. In this study, we profiled skin bacterial flora of captive giant pandas for the first time using 16S rRNA-based NGS sequencing. We detected 522 genera in 53 bacterial phyla. Among these, Proteobacteria, Actinobacteria, and Firmicutes were the predominant phyla, and Streptococcus, Acinetobacter, and Staphylococcus were the predominant genera. Our results showed that the skin bacterial community of the giant panda differs from that of humans and other animals (canine and feline). Previous studies showed that canine skin was dominated by Fusobacterium and Pseudomonas, feline skin was dominated by Porphyromonas and Staphylococcus, and human skin was dominated by Propionibacterium and Staphylococcus (13, 23, 24). Skin microbiota in different hosts may be affected by genetic differences and pelage characteristics, as well as different hygiene practices and environmental exposures between host species.
The skin bacterial flora of captive giant pandas change with the seasons. Moreover, the bacterial abundance in spring was significantly higher than that in other seasons and the diversity in winter was lower than that in other seasons, and skin bacterial diversity in autumn was higher than that in other seasons. Spring is warm and humid in Sichuan, China. High temperatures compounded by the humid environment of spring are optimal for the growth and reproduction of bacteria, and this may have contributed to the differences seen in abundance. Cold and dry winter conditions may have contributed to the difference in diversity. Interestingly, we found that the diversity and abundance of bacterial communities were not solely related to the temperature and humidity of the environment. For example, the ambient temperature is higher in summer, but the Shannon diversity index indicated that microbial diversity in samples collected during summer was not higher than that in samples collected during spring and autumn. The ACE index showed that microbial abundance in samples collected in summer was lower than that in samples collected in other seasons. However, other studies have reported different results, and studies of the human skin microbiome show that despite exposure to the external environment, the bacterial, fungal, and viral communities in the skin were largely stable over time (12). A study of the skin microbiota in healthy dogs showed that temporality (season of birth and time spent in the kennel) affected all skin sites (13). The stability of the skin microbiome seems to vary between species over time. Changes in the skin microbiome are not just related to changes in ambient temperature and humidity, but also to the state of immune activation, host genetic predisposition, barrier status, microbe localization, and microbe–microbe interactions (3). This may be because animals interact with their living environment and are constantly exposed to soil, water, plants, and sewage. Therefore, the skin microbiome of animals is prone to change with seasonal changes. Humans generally live in a relatively stable environment, due to which changes in the skin microbiome are not obvious. At the genus level, five genera were significantly more abundant during the specific seasons. Arthrobacter was associated with spring, Kocuria and Deinococcus were associated with summer, Acinetobacter was associated with autumn, and Streptococcus was associated with winter. These bacterial taxa have been isolated from the environment as well as animals and physiologically characterized (25–32). Streptococcus is a common resident of human skin. Streptococcus pyogenes is a very important human pathogen that is commonly associated with skin or throat infections, but may also cause life-threatening conditions including sepsis, streptococcal toxic shock syndrome, and necrotizing fasciitis (33). An increase in the abundance of Streptococcus in winter may increase the possibility of panda skin being infected by Streptococcus. Acinetobacter is a common bacterium found in human and animal skin (29, 34). These bacteria, which have already been recognized as important nosocomial pathogens in humans, are becoming increasingly recognized in opportunistic pathogens of animals. Acinetobacter baumannii is a gram-negative skin pathogen. A. baumannii causes a variety of infections, most of which involve the respiratory tract, although bacteremia and skin wound infections have also been reported (35, 36). There are no case reports of skin diseases caused by A. baumannii in giant pandas. Arthrobacter, Kocuria, and Deinococcus are common commensal bacteria found on human skin, but occasionally, infections have been reported (37–40).
Microbiota of giant pandas also carries other taxa, including Staphylococcus, Pseudomonas, and Propionibacterium, that are known to cause bacterial skin diseases in humans and other animals. However, the abundance of these taxa does not change significantly with the seasons. Staphylococcus, although generally identified as a commensal, commonly causes human bacterial infections of the skin and other soft tissues, bones, bloodstream, and the respiratory tract (41). Staphylococcus is responsible for most bacterial skin infections and can initiate or exacerbate skin disorders in the broader context of barrier defects or altered immunity. It has been reported in an infection case of an aged giant panda, causing pressure ulcers on the body surface (10). Pseudomonas is a gram-negative bacterium, and Pseudomonas aeruginosa, which is most often associated with opportunistic infections, can also appear in healthy individuals. P. aeruginosa infections range from local skin infections to life-threatening diseases (42). The gram-positive anaerobic bacterium Propionibacterium acnes is part of the normal microbiome of human skin and mucosal surfaces. Although P. acnes is commonly associated with skin health, it is also an opportunistic pathogen associated with a range of human infections and clinical conditions (43). In the present study, it was found that potentially pathogenic bacteria were ubiquitous across all samples. Bacterial skin diseases in giant pandas are mainly reported as staphylococcal and streptococcal infections. Clinically, aged pandas and pandas with low immunity are prone to skin diseases (10). Commensal bacteria of the skin, mucosa, or gastrointestinal tract, including staphylococci and P. acnes, are often opportunistic pathogens. It is important to study potential skin pathogens. The development of bacterial skin disease is multifactorial and may not only be associated with the bacterial community structure but also host skin integrity and body immunity as well as associated with the fungal and viral communities of skin surfaces. However, the high diversity and abundance of the skin bacteria observed in this study during the spring and autumn may increase the risk of the bacterial pathogens infecting the host. In addition, the fact that captive pandas monitored in this study were all healthy may have limited our investigation of pathogenic bacteria inhabiting the skin of captive pandas. Thus, applying metagenomics technology to study captive panda skin microbiomes in the future may enable a more complete understanding of the captive panda skin microbiota.
In this study, the skin microbiota of healthy captive giant pandas was profiled using 16S rRNA-based NGS sequencing. The results indicated that the skin microbiota of giant pandas changes with the seasons and that the diversity and abundance of skin bacteria was the highest in autumn, followed by spring. This indicates that the risk of bacterial skin diseases in giant pandas may increase in autumn and spring. Several skin disease-associated bacteria were detected in the skin microbiota of healthy giant pandas, indicating that skin disease-associated bacteria are normal components of the skin microbiota of giant pandas and may cause bacterial skin diseases, conditionally. It is essential for us to have a better understanding of the microbial population that lives on the skin of giant pandas, to be able to describe the skin microbiota of healthy pandas, identify changes in the skin microbiota that occur in a disease state, and potentially identify better measures to treat skin conditions. The present study, to our knowledge, revealed the skin microbiota of the captive giant pandas for the first time and provided insights into the development of bacterial skin diseases in these endangered animals.
The datasets generated for this study can be found in the National Center for Biotechnology Information (NCBI). (SRA: https://www.ncbi.nlm.nih.gov/sra/PRJNA688828).
The animal study was reviewed and approved by Sichuan Agricultural University Animal Ethics Committee and China Conservation and Research Center for the Giant Panda Animal Ethics Committee.
XM, GL, CY, MH, CW, YG, and SL carried out the sample collections, conceived the study, and drafted the manuscript. GL, SC, QY, XH, YW, QZ, and RW participated in the data analysis. JD, ZZu, SY, YH, ZZh, XM, and GP participated in the study design and coordination and helped draft the manuscript. All authors have read and approved the final manuscript.
This study was supported by the National Key Laboratory of Giant Panda National Park open funds (KLSFGAGP2020.023) and Applied Basic Research Project in Sichuan Province (2018JY0183), as well as Giant Pandas International Cooperation funds (2017)115.
The authors declare that the research was conducted in the absence of any commercial or financial relationships that could be construed as a potential conflict of interest.
We thank China Conservation and Research Center for the Giant Panda for providing panda hair and dander samples and Editage (www.editage.cn) for English language editing.
The Supplementary Material for this article can be found online at: https://www.frontiersin.org/articles/10.3389/fvets.2021.666486/full#supplementary-material
1. Ma X, Li C, Hou J, Gu Y. Isolation and identification of culturable fungi from the genitals and semen of healthy giant pandas (Ailuropoda melanoleuca). BMC Vet Res. (2017) 13:344. doi: 10.1186/s12917-017-1231-0
2. Scharschmidt TC, Fischbach MA. What lives on our skin: ecology, genomics and therapeutic opportunities of the skin microbiome. Drug Discov Today Dis Mech. (2013) 10: e83–9. doi: 10.1016/j.ddmec.2012.12.003
3. Chen YE, Fischbach MA, Belkaid Y. Skin microbiota-host interactions. Nature. (2018) 553:427–36. doi: 10.1038/nature25177
4. Proksch E, Brandner JM, Jensen JM. The skin: an indispensable barrier. Exp Dermatol. (2008) 17:1063–72. doi: 10.1111/j.1600-0625.2008.00786.x
5. Yamazaki Y, Nakamura Y, Núñez G. Role of the microbiota in skin immunity and atopic dermatitis. Allergol Int. (2017) 66:539–44. doi: 10.1016/j.alit.2017.08.004
6. Zhang Y, Ma X, Gu Y, Yuan S, Li D, Huang X, et al. Identification and pathogenicity of Microsporum gypseum isolated from Ailuropoda melanoleuca. Chin Vet Sci. (2015) 45:551–9. doi: 10.1007/s11430-014-4953-x
7. Yang S, Gao X, Meng J, Zhang A, Zhou Y, Long M, et al. Metagenomic analysis of bacteria, fungi, bacteriophages, and helminths in the gut of giant pandas. Front Microbiol. (2018) 9:1717. doi: 10.3389/fmicb.2018.01717
8. Ma X, Gu Y, Liu X, Li D, Ling S, Hou J, et al. Phaeohyphomycotic dermatitis in a giant panda (Ailuropoda melanoleuca) caused by Cladosporium cladosporioides. Med Mycol Case Rep. (2013) 2:119–21. doi: 10.1016/j.mmcr.2013.05.003
9. Yu G, Jia-Xue R, Xiao-Ping M, De-Sheng L, Xiao-Min L, Shan-Shan L, et al. A Pestalotiopsis hainanensis strain from the body surface of Ailuropoda melanoleuca. Mycosystema. (2014) 33:731–7. doi: 10.13346/j.mycosystema.130131
10. Wang C, Ma X, Li D, Gu Y. Laboratory examination and experience of an aged giant panda after death. Anim Husband Vet Med. (2015) 47:75–6.
11. Kong HH, Segre JA. Skin microbiome: looking back to move forward. J Invest Dermatol. (2012) 132:933–9. doi: 10.1038/jid.2011.417
12. Oh J, Byrd AL, Park M, Kong HH, Segre JA. Temporal stability of the human skin microbiome. Cell. (2016) 165:854–66. doi: 10.1016/j.cell.2016.04.008
13. Cuscó A, Belanger JM, Gershony L, Islas-Trejo A, Levy K, Medrano JF, et al. Individual signatures and environmental factors shape skin microbiota in healthy dogs. Microbiome. (2017) 5:139. doi: 10.1186/s40168-017-0355-6
14. Woese CR, Fox GE. Phylogenetic structure of the prokaryotic domain: the primary kingdoms. Proc Natl Acad Sci USA. (1977) 74:5088–90. doi: 10.1073/pnas.74.11.5088
15. Bansal S, Thakur S, Mangal M, Mangal AK, Gupta RK. DNA barcoding for specific and sensitive detection of Cuminum cyminum adulteration in Bunium persicum. Phytomedicine. (2018) 50:178–83. doi: 10.1016/j.phymed.2018.04.023
16. Caporaso JG, Kuczynski J, Stombaugh J, Bittinger K, Bushman FD, Costello EK, et al. QIIME allows analysis of high-throughput community sequencing data. Nat Methods. (2010) 7:335–6. doi: 10.1038/nmeth.f.303
17. Magoč T, Salzberg SL. FLASH: fast length adjustment of short reads to improve genome assemblies. Bioinformatics. (2011) 27:2957–63. doi: 10.1093/bioinformatics/btr507
18. Edgar RC. UPARSE: highly accurate OTU sequences from microbial amplicon reads. Nat Methods. (2013) 10:996–8. doi: 10.1038/nmeth.2604
19. Quast C, Pruesse E, Yilmaz P, Gerken J, Schweer T, Yarza P, et al. The SILVA ribosomal RNA gene database project: improved data processing and web-based tools. Nucleic Acids Res. (2013) 41:D590–6. doi: 10.1093/nar/gks1219
20. Wang Q, Garrity GM, Tiedje JM, Cole JR. Naive Bayesian classifier for rapid assignment of rRNA sequences into the new bacterial taxonomy. Appl Environ Microbiol. (2007) 73:5261–7. doi: 10.1128/AEM.00062-07
21. Segata N, Izard J, Waldron L, Gevers D, Miropolsky L, Garrett WS, et al. Metagenomic biomarker discovery and explanation. Genome Biol. (2011) 12:R60. doi: 10.1186/gb-2011-12-6-r60
22. Stehlikova Z, Kostovcik M, Kostovcikova K, Kverka M, Juzlova K, Rob F, et al. Dysbiosis of skin microbiota in psoriatic patients: Co-occurrence of fungal and bacterial communities. Front Microbiol. (2019) 10:438. doi: 10.3389/fmicb.2019.00438
23. Grice EA, Segre JA. The skin microbiome. Nat Rev Microbiol. (2011) 9:244–53. doi: 10.1038/nrmicro2537
24. Older CE, Diesel AB, Lawhon SD, Queiroz CRR, Henker LC, Rodrigues Hoffmann A. The feline cutaneous and oral microbiota are influenced by breed and environment. PLoS ONE. (2019) 14:e0220463. doi: 10.1371/journal.pone.0220463
25. Zbinden A, Bostanci N, Belibasakis GN. The novel species Streptococcus tigurinus and its association with oral infection. Virulence. (2015) 6:177–82. doi: 10.4161/21505594.2014.970472
26. Carvalheira A, Ferreira V, Silva J, Teixeira P. Enrichment of Acinetobacter spp. from food samples. Food Microbiol. (2016) 55:123–7. doi: 10.1016/j.fm.2015.11.002
27. Lin H, Wang Y, Huang J, Lai Q, Xu Y. Deinococcus planocerae sp. nov., isolated from a marine flatworm. Antonie Leeuwenhoek. (2017) 110:811–817. doi: 10.1007/s10482-017-0854-2
28. Srinivasan S, Lim S, Lim JH, Jung HY, Kim MK. Deinococcus rubrus sp. nov., a bacterium isolated from antarctic coastal sea water. J Microbiol Biotechnol. (2017) 27:535–41. doi: 10.4014/jmb.1609.09002
29. Mitchell KE, Turton JF, Lloyd DH. Isolation and identification of Acinetobacter spp. from healthy canine skin. Vet Dermatol. (2018) 29:240–e87. doi: 10.1111/vde.12528
30. Ruan A, Gao Y, Fang C, Xu Y. Isolation and characterization of a novel nicotinophilic bacterium, Arthrobacter sp. aRF-1 and its metabolic pathway. Biotechnol Appl Biochem. (2018) 65:848–56. doi: 10.1002/bab.1682
31. Napolitani M, Troiano G, Bedogni C, Messina G, Nante N. Kocuria kristinae: an emerging pathogen in medical practice. J Med Microbiol. (2019) 68:1596–603. doi: 10.1099/jmm.0.001023
32. Pompilio A, Di Bonaventura G, Gherardi G. An overview on complex isolates: identification to the species/subspecies level and antibiotic resistance. Int J Mol Sci. (2019) 20:480. doi: 10.3390/ijms20030480
33. Ibrahim J, Eisen JA, Jospin G, Coil DA, Khazen G, Tokajian S. Genome analysis of Streptococcus pyogenes associated with pharyngitis and skin infections. PLoS ONE. (2016) 11:e0168177. doi: 10.1371/journal.pone.0168177
34. Fyhrquist N, Ruokolainen L, Suomalainen A, Lehtimäki S, Veckman V, Vendelin J, et al. Acinetobacter species in the skin microbiota protect against allergic sensitization and inflammation. J Allergy Clin Immunol. (2014) 134:13019.e1. doi: 10.1016/j.jaci.2014.07.059
35. Sebeny PJ, Riddle MS, Petersen K. Acinetobacter baumannii skin and soft-tissue infection associated with war trauma. Clin Infect Dis. (2008) 47:444–9. doi: 10.1086/590568
36. Mihu MR, Martinez LR. Novel therapies for treatment of multi-drug resistant Acinetobacter baumannii skin infections. Virulence. (2011) 2:97–102. doi: 10.4161/viru.2.2.15061
37. Guerrero DM, Perez F, Conger NG, Solomkin JS, Adams MD, Rather PN, et al. Acinetobacter baumannii-associated skin and soft tissue infections: recognizing a broadening spectrum of disease. Surg Infect. (2010) 11:49–57. doi: 10.1089/sur.2009.022
38. Castelino M, Eyre S, Moat J, Fox G, Martin P, Ho P, et al. Optimisation of methods for bacterial skin microbiome investigation: primer selection and comparison of the 454 versus MiSeq platform. BMC Microbiol. (2017) 17:23. doi: 10.1186/s12866-017-0927-4
39. Kolikonda MK, Jayakumar P, Sriramula S, Lippmann S. Kocuria kristinae infection during adalimumab treatment. Postgrad Med. (2017) 129:296–8. doi: 10.1080/00325481.2017.1250606
40. Zeng B, Zhao J, Guo W, Zhang S, Hua Y, Tang J, et al. High-Altitude living shapes the skin microbiome in humans and pigs. Front Microbiol. (2017) 8:1929. doi: 10.3389/fmicb.2017.01929
41. Parlet CP, Brown MM, Horswill AR. Commensal staphylococci influence Staphylococcus aureus skin colonization and disease. Trends Microbiol. (2019) 27:497–507. doi: 10.1016/j.tim.2019.01.008
42. Wu DC, Chan WW, Metelitsa AI, Fiorillo L, Lin AN. Pseudomonas skin infection: clinical features, epidemiology, and management. Am J Clin Dermatol. (2011) 12:157–69. doi: 10.2165/11539770-000000000-00000
Keywords: giant panda (Ailuropoda melanoleuca), skin microbiome, 16S rRNA gene, high-throughput sequencing, seasonality
Citation: Ma X, Li G, Yang C, He M, Wang C, Gu Y, Ling S, Cao S, Yan Q, Han X, Wen Y, Zhao Q, Wu R, Deng J, Zuo Z, Yu S, Hu Y, Zhong Z and Peng G (2021) Skin Microbiota of the Captive Giant Panda (Ailuropoda Melanoleuca) and the Distribution of Opportunistic Skin Disease-Associated Bacteria in Different Seasons. Front. Vet. Sci. 8:666486. doi: 10.3389/fvets.2021.666486
Received: 13 February 2021; Accepted: 03 June 2021;
Published: 05 July 2021.
Edited by:
Cleverson D. Souza, Washington State University, United StatesReviewed by:
Ajar Nath Yadav, Eternal University, IndiaCopyright © 2021 Ma, Li, Yang, He, Wang, Gu, Ling, Cao, Yan, Han, Wen, Zhao, Wu, Deng, Zuo, Yu, Hu, Zhong and Peng. This is an open-access article distributed under the terms of the Creative Commons Attribution License (CC BY). The use, distribution or reproduction in other forums is permitted, provided the original author(s) and the copyright owner(s) are credited and that the original publication in this journal is cited, in accordance with accepted academic practice. No use, distribution or reproduction is permitted which does not comply with these terms.
*Correspondence: Ming He, MzI3NjM2NzgwQHFxLmNvbQ==; Chengdong Wang, Mjg1OTM0MDEyQHFxLmNvbQ==; Yu Gu, Z3V5dTYzMkBzaWNhdS5lZHUuY24=
†These authors have contributed equally to this work and share first authorship
Disclaimer: All claims expressed in this article are solely those of the authors and do not necessarily represent those of their affiliated organizations, or those of the publisher, the editors and the reviewers. Any product that may be evaluated in this article or claim that may be made by its manufacturer is not guaranteed or endorsed by the publisher.
Research integrity at Frontiers
Learn more about the work of our research integrity team to safeguard the quality of each article we publish.