- Department of Population Health and Pathobiology, College of Veterinary Medicine, North Carolina State University, Raleigh, NC, United States
Diversity, ecology, and evolution of viruses are commonly determined through phylogenetics, an accurate tool for the identification and study of lineages with different pathological characteristics within the same species. In the case of PRRSV, evolutionary research has divided into two main branches based on the use of a specific gene (i.e., ORF5) or whole genome sequences as the input used to produce the phylogeny. In this study, we performed a review on PRRSV phylogenetic literature and characterized the spatiotemporal trends in research of single gene vs. whole genome evolutionary approaches. Finally, using publicly available data, we produced a Bayesian phylodynamic analysis following each research branch and compared the results to determine the pros and cons of each particular approach. This study provides an exploration of the two main phylogenetic research lines applied for PRRSV evolution, as well as an example of the differences found when both methods are applied to the same database. We expect that our results will serve as a guidance for future PRRSV phylogenetic research.
Introduction
Viral diseases affecting livestock are a major problem because of their rapid spread, negative impact in animal health, potential spillover to humans, and detrimental effect on economic systems (1, 2). In 2019, international commerce of livestock and swine products surpassed $20 billion worldwide, from which the U.S. alone reached over $7 billion as reported by the United States Department of Agriculture (USDA) (3). For those reasons, controlling infectious diseases affecting swine is an ever-growing challenge, shaped by the constant race between the potential of pathogens to evolve and spread, and the ability of researchers to elucidate mechanisms and to develop effective prophylactic and therapeutic measures to reduce their impact on the swine population.
Viruses in general are one of the infectious agents with the highest mutation rates, which hinders the ability of researchers to predict their evolution and spread due to their ever-changing genome (4, 5). This is particularly common in the case of RNA viruses such as Porcine Reproductive and Respiratory Syndrome Virus (PRRSV), currently one of the most deleterious diseases for the swine industry worldwide, reaching up to 60% prevalence in growing-finishing herds (1, 2, 6, 7). PRRSV is an enveloped positive sense single-stranded RNA virus in the family Arteriviridae (8) with a genome of ~15 kb that encodes at least nine open reading frames (ORFs) (9). ORF5 in particular is widely used for phylogenetic analysis, since its structure encompasses both hypervariable and conserved segments, allowing the classification of strains in a reasonably accurate way (10–12). Based on its genetic diversity and antigenic properties, PRRSV is classified into two distinct genotypes with different species (13): Type 1 and Type 2, that are mostly circulating in Europe and North America respectively (11, 14). Due to its genetic nature, its recombination ability is one of the main shapers of PRRSV evolution and diversity (15, 16), along with its mutation rate, that was recently estimated at 0.00672 (16).
Over the last decade, the concept and application of interdisciplinary sciences have improved infectious disease control measures by the combination of the genetic, geographic, and historical data of pathogens (17–20), providing a much deeper understanding of their evolutionary trajectory and therefore allowing the application of targeted control strategies and treatments based on this new information (16). However, due to the multiple possibilities that interdisciplinary approaches offer, there is often an open discussion to determine the most accurate method to apply in a given scientific scenario.
Early molecular studies of PRRSV applied RT-PCR to detect virus, leading to the first PRRSV phylogenetic reconstruction based on ORF-5 and ORF-7 sequences that was able to differentiate the European and American clades (21). This paper was followed by numerous phylogenetic studies using ORF-5 given the compromise of sites evolving at different rates, which generated well-resolved trees during a period where whole genome sequencing was particularly challenging. However, despite the increasing availability of whole genome sequences, scientists are still divided by the support of the use of whole genomes or single genes (22), particularly for evolutionary analyses of organisms like PRRSV, that is an exceptionally diverse virus (23).
Multiple factors have a key role in this decision, especially for research analyzing field isolates and samples that need to be sequenced, since the economic effort needed, along with the requirement of specialized laboratories, equipment and skilled personnel is remarkably higher to perform whole genome sequencing than single genes (24). In the case of whole genome defenders, they advocate the consideration of all nucleotides to identify all changes between genomes, rather than the changes in specific and usually conserved genes (caused for example by horizontal gene transfer) (25, 26). On the other hand, opinions of scientists supporting the use of single genes or multi-gene approaches (but not whole genome application) (27, 28) argue that by considering whole genomes, there is the possibility to detect changes in non-coding genes that could misclassify sequences.
The goal of this study is to evaluate and compare the different patterns and trends on PRRSV research in relation to the application of whole genomes or single genes, and assess the potential variations observed on the same analyses when one of the two approaches is applied using the same genetic database.
Materials and Methods
Bibliometric Analyses
The bibliometric search for the available publications was performed in Scopus, using the search criteria “TITLE-ABS-KEY [(PRRSV AND [whole AND genome OR ORF5]) OR (porcine AND reproductive AND respiratory AND syndrome AND [whole AND genome OR ORF5]) OR (PRRSV AND [phylogeny OR phylogenetics OR evolution])]” and downloaded the obtained results in bib format. Using the R package Bibliometrix (29), the journal, year of publication, title, abstract, author names, and author affiliations of all resulting publications were extracted. From this initial database, a manual screening was performed: original or literature reviews, the study area (global vs. country level), and the use of whole genome or ORF5 gene were extracted.
Genetic Databases
All PRRSV whole genome sequences available were downloaded from Genbank as a.gb file and ran the python package “gbmunge” (https://github.com/sdwfrost/gbmunge) to retrieve the available metadata for each sequence. From that database, 765 sequences, for which geographic and temporal information was available, were selected for subsequent analyses. Sequences were aligned using MEGA X (30). Using this updated database, the sequences were aligned along with three PRRSV ORF5 sequences, EU556220, DQ405282, and DQ405279, to identify the region of the whole genome in which ORF5 was located. Then, ORF5 region of the sequences was manually identified and saved in a second database used for analyses.
Recombination Analyses
The recombination detection program (RDP) v5.3 was used to search for recombination within our data set (31). The alignment was screened using five methods (BootScan, Chimaera, MaxChi, RDP, and SiScan).
Phylogeny
To find the best substitution model for each database, the ModelFinder tool (32) built into IQ-Tree version 1.6.1 (33) was used. The marginal likelihood value supported the use of the general time-reversible model (GTR) with gamma-distributed rate heterogeneity plus a proportion of invariable sites (GTR+G+I) (34) for both databases (Supplementary Table 1). To determine the best fitting node-age and branch-rate model, each combination of molecular clock and branch rate was run to compare the marginal likelihood estimated by the stepping-stone and path-sampling methods, supporting the use of uncorrelated relaxed molecular clock model and coalescent logistic growth as the tree prior for both ORF-5 and whole genome databases. Both phylogenies were then estimated by Bayesian inference through Markov chain Monte Carlo (MCMC), implemented in BEAST v2.6.0 (35). The model was run for 100 million generations, sampling every 10,000th generation and removing 10% of the chain as burn-in in both cases. The probabilities of ancestral states were inferred from the Bayesian discrete-trait analysis and visualized as pie charts on each node. Visualization of the trees was performed using FigTree v1.4.4 (Rambaut1).
Phylodynamics
The spatiotemporal spread patterns observed for both databases were performed via Bayesian continuous phylogeographic analysis, following the model selection described in the phylogeny section. An uncorrelated relaxed molecular clock model with lognormal distribution (36) and the Bayesian SkyGrid with covariates as the coalescent tree prior (37) were also applied. To ensure an effective sample size (ESS) value over 200, analyses were run for 200 million generations, sampling every 10,000th generation and removing 10% of the chain as burn-in. To determine the relative genetic diversity over time of each database we used Bayesian SkyGrid, as this approach relies on a non-parametric coalescent model to estimate the effective population size over time (38).
Results
Bibliometric Analyses and Genetic Databases
The bibliometric search recognized 374 articles under our search criteria, from which only 49 were global studies. From that total, 23 literature reviews and 351 original articles, from which 190 of them applied whole genome analyses, and 155 used single genes, were identified. Detailed information about the 6 remaining articles was not available at the time of the screening (October 2020). One hundred and twenty five of the publications using single genes chose ORF5, leaving only 30 articles with a different genome section [i.e., ORF7 (10), Nsp2 (39)].
For both whole genome sequences (WGS) and ORF5 sequences, the countries with the highest scientific productivity were China (WGS = 295, ORF5 = 141), the United States (WGS = 89, ORF5 = 88), and South Korea (WGS = 55, ORF5 = 50) (Figure 1A). Overall, PRRSV research (whole and partial genome studies) evidenced higher scientific productivity per year up to 2018, with a rapid decrease maintained until our search was performed (October 2020) (Figure 1B, Supplementary Figure 1). In addition, 12 countries only evidenced articles using WGS, while 8 countries only produced articles related to ORF5 sequences (Supplementary Table 2).
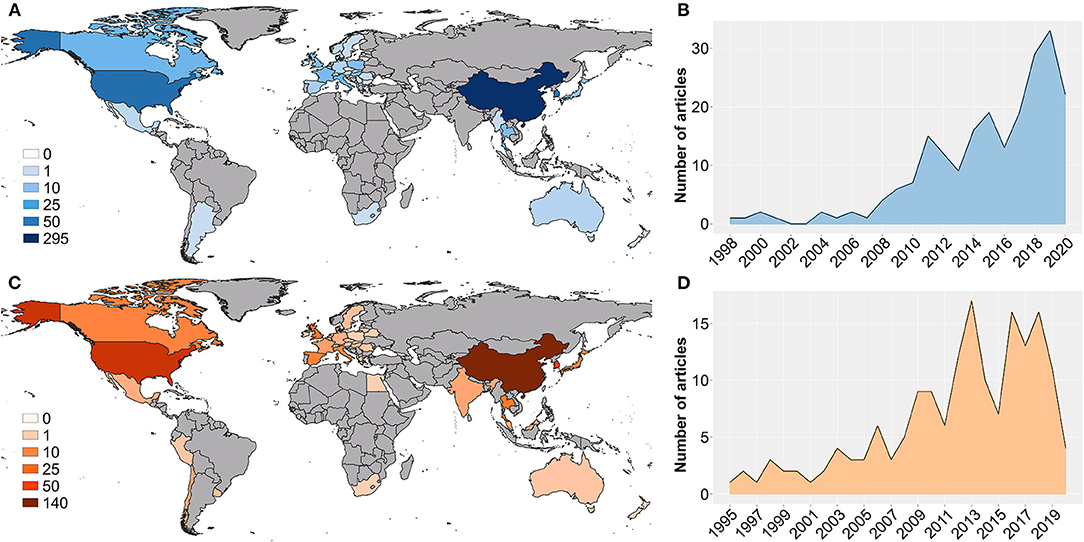
Figure 1. Global annual scientific production involving whole genome sequences (blue) and single genes (orange) where A represents the number of publications per country of whole genome-related research, B represents the global annual scientific production of whole genome-related research, C represents the number of publications per country of single genes-related research, and D represents the global annual scientific production of single genes-related research.
In relation to the produced genetic databases, the starting point after running the gbmunge package was of 765 whole genome PRRSV sequences with most of the necessary metadata available (Supplementary Table 1). To avoid sampling bias, a similar number of sequences was chosen from different locations to reach a total of 100. The ORF5 database consisted of the exact same sequences from which the ORF5 section of the genome was manually identified and isolated.
Recombination Analyses
From the total number of sequences with metadata we obtained (765), the Recombination Detection Program (RDP) detected 491 recombinant sequences from the whole genome database, and 393 from the ORF5 database (Figure 1), making a difference of 98 between the two (data available upon request).
Phylogeny and Ancestral Reconstruction
Phylogenetic results for the whole genome database showed that the most likely center of origin for the PRRSV sequences analyzed was Belarus with a root state posterior probability (RSPP) = 10%. This original lineage then diverged into two groups likely driven by geographical distance and independent subsequent evolution, one with a higher probability of being originated in China (RSPP = 26%), and a second one likely originated in Hungary (RSPP = 16%) (Figure 2A). The amount of lineages present over time showed an overall increase in the number of different lineages with two main periods of growth from 1600 to 1750 and from 1950 to the present day (Figure 2B).
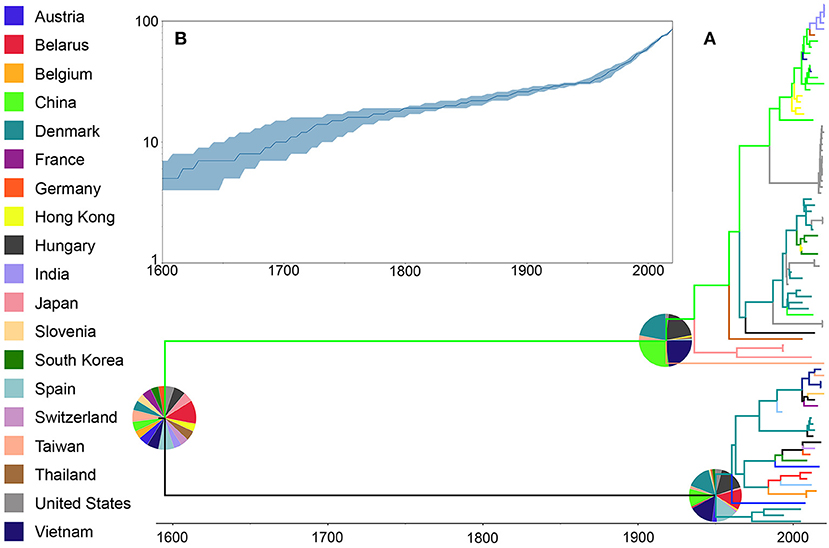
Figure 2. Phylogenetic history of PRRSV inferred using whole genome sequences. (A) Maximum clade credibility (MCC) phylogeny, colored according to the country of origin of each sequence. The probabilities of ancestral states (inferred from the Bayesian discrete-trait analysis) are shown in pie charts on each node. (B) Spatiotemporal patterns represented through lineages through time plot.
When the ORF5 sequence database was analyzed, the ancestral reconstruction also evidenced Belarus as the most likely country of origin (RSPP = 11%) (Figure 3). Similarly to the pattern shown by WGS, the analysis showed that this ancestral lineage diverged into two clearly defined groups, both of them with a higher probability of being originated in Denmark (RSPP = 45, and 26% respectively). In the case of the number of lineages through time, this database showed no new lineages appearing until after 1,800 when it presented two clear isolated diversification events that maintained the number of lineages until ~1,900, which was the starting point of a rapid exponential increase in the number of lineages up to the day of the screening (Figure 3B). Finally, the 95% highest probability density (HPD) values of both trees showed similar uncertainty patterns, with the most recent nodes showing less uncertainty than the ancestral (Supplementary Figure 2). However, based on these HPD values the ORF-5 database showed less accuracy to reconstruct the ancestral nodes, suggesting whole genome as the most robust approach for this type of studies.
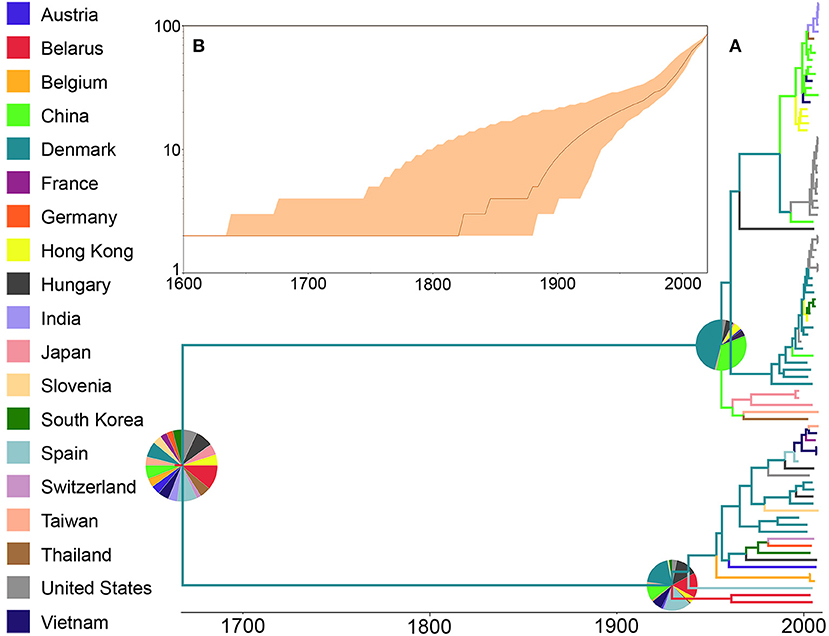
Figure 3. Phylogenetic history of PRRSV inferred using ORF5 sequences. (A) Maximum clade credibility (MCC) phylogeny, colored according to the country of origin of each sequence. The probabilities of ancestral states (inferred from the Bayesian discrete-trait analysis) are shown in pie charts on each node. (B) Spatiotemporal patterns represented through lineages through time plot.
Phylodynamics
When the genetic diversity obtained for the analysis of whole genome vs. ORF5 databases was compared over time, SkyGrid plot revealed an overall higher genetic diversity exhibited by the ORF5 sequences. In addition, there is a noticeable difference in the pattern of diversity increase, where the ORF5 database showed constant growth while the whole genome database started to experience an increase in diversity from 1983, with a sharp decrease in 2009 that was not detected by the ORF5 result (Figure 4).
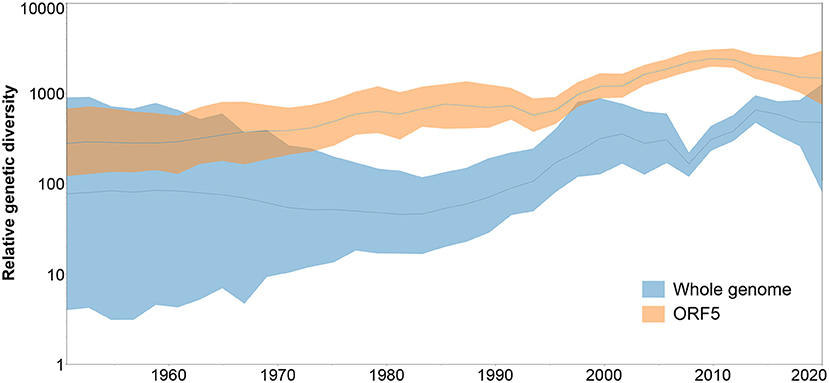
Figure 4. Spatiotemporal patterns in the relative genetic diversity represented through the Bayesian SkyGrid plot, where dark lines represent the mean values, while shaded light regions correspond to the 95% highest posterior density (HPD).
Finally, when we compared the dispersal velocity of PRRSV based on each database, we observed that ORF5 sequences showed higher spread velocity (2948.7 km/year) than whole genome sequences (1956.4 km/year) (Supplementary Table 3).
Discussion
Our bibliometric screening detected an overall higher number of articles considering the use of whole genomes than single genes. However, this happened only after 2010, when the increase in the amount of whole genome related publications started to grow and surpassed the application of ORF5 that had been applied in the previous years. The decrease in productivity observed around 2018 could be the result of the detection of an outbreak of African Swine Fever in China (40), which would likely trigger a deviation on research efforts toward that disease. This growth in whole genome sequence application could have been triggered by the increase in the availability and affordability of RNA-seq technology once it became more accessible for general research, surpassing the levels of the use of ORF5 sequences, that had been posed as the standard gene applied to study PRRSV evolution due to their high variability (11). This hypothesis would also fit with our observation on country productivity, where countries with access to sequencing are leading scientific production for both whole genome studies and single genes. Not surprisingly, these increased publication rates are linked to wealthy economies with high pig production given the elevated cost of sequencing studies, although not every country presented publications in both areas.
The bibliographic search was performed using the Scopus database, as it is a large, multidisciplinary database that includes MEDLINE and has been described to combine the characteristics of both Web of Science and PubMed, allowing an improved service for educational and academic needs, also favoring Natural Science and Biomedical research literature (41). Furthermore, previous research compared the amount of publications retrieved by those three different databases, obtaining a more extensive number of detected publications using Scopus (42).
Another expected result was the number of recombinant sequences detected on each database. Recombinant sequences should be considered when choosing between whole genomes or single genes, particularly in RNA viruses such as PRRSV (12, 43, 44). A common claim between scientists supporting the use of single genes for evolutionary analysis relies on the presence of numerous non-coding regions (introns) that could interfere with those analyses and cause bias on the results. However, we observed that the whole genome database detected a higher number of recombinants. Although there are no studies assessing the proportion of recombinant sequences detected due to non-coding regions, numerous publications have mentioned the implications and importance of considering these non-coding sequences on recombination, evolution, and chromosomal stability assessments (45–47).
The shape of the phylogenetic trees obtained for both databases was similar. This suggests that for analyses focusing on the evolutionary patterns as well as the identification and taxonomy of this virus, both approaches could fulfill the needs of the study. However, in the case of the ancestral reconstruction studies, as well as in the reconstruction of phylodynamic patterns, we observed numerous differences between the two datasets, showing that the choice between whole genome or single genes should be considered carefully depending on the study performed. Particularly in the case of ancestral reconstruction studies, where our whole genome database showed more accuracy on the estimated ancestral nodes (measured as HPD values) than our ORF-5 database. It is important to keep in mind that the main goal of this project was not to perform a phylodynamic study of PRRSV or to determine its ancestral origin, but to identify the similarities and differences observed when identical evolutionary analyses were performed in the same sequences of our database using the whole genome or only its ORF5 segment. Interestingly, with the set of sequences used in our analyses, the evolutionary trends and shape of the trees obtained coincide with previously published studies in PRRSV evolutionary history (11, 16), suggesting that even though higher number of sequences will generally produce more robust analyses, inferences and patterns can be identified with reduced amounts of data as a baseline for subsequent and more elaborated studies.
We faced some limitations during the development of this study. Firstly, the affiliation information extracted by the Bibliometrix R package did not necessarily correspond to the country where the initial research was developed. This is a common limitation in bibliometric studies that has been previously assessed via sensitivity analyses with no significant changes on the obtained results (48). In addition, publications using whole genome sequencing are generally complex and include wide collaboration networks where authors from different locations share resources and data. Therefore, one single article may be detected by bibliometric measurements in more than one country. Likewise, our search only included papers that were already published and available by October 2020. Therefore, the productivity of this year must not be considered final, because papers submitted but not yet published before our search day are likely to get published after our search was done or even in 2021.
Finally, this study represents a comparison of the most commonly applied evolutionary analyses in PRRSV research using whole genome or single gene sequences as an input. Here, we show the similarities and differences on the results driven by the use of the whole genome or the ORF-5 section of the same set of genes, analyze the evolution and patterns of research on each area over time and highlight the need to take these differences into consideration when deciding the most appropriate approach to apply depending on the specific aim of the research performed, particularly in analyses that involve ancestral reconstruction.
Data Availability Statement
The original contributions generated for the study are included in the article/Supplementary Material, further inquiries can be directed to the corresponding author.
Author Contributions
EC and AF-D-D were responsible for the conception of the study and manuscript writing and revisions. AF-D-D was responsible for the acquisition of data and data analysis. AF-D-D and MJ were responsible for data analysis and interpretation, manuscript editing, and revisions. BP was responsible for manual data screening and revision of the manuscript. EC was responsible for project supervision and administration. All authors have read and agreed to the published version of the manuscript.
Funding
This work was partially supported by the USDA National Institute of Food and Agriculture, Animal Health project 1021578.
Conflict of Interest
The authors declare that the research was conducted in the absence of any commercial or financial relationships that could be construed as a potential conflict of interest.
Acknowledgments
Authors would like to thank Dr. Rocio Crespo and Dr. Glen Almond for her insightful comments and suggestions that helped improved our manuscript.
Supplementary Material
The Supplementary Material for this article can be found online at: https://www.frontiersin.org/articles/10.3389/fvets.2021.658512/full#supplementary-material
Footnotes
1. ^Rambaut, A. “Figtree v1.4.4. Available online at: http://tree.bio.ed.ac.uk/software/figtree/”.
References
1. Holtkamp DJ, Kliebenstein JB, Neumann E, Zimmerman JJ, Rotto H, Yoder TK, et al. Assessment of the economic impact of porcine reproductive and respiratory syndrome virus on United States pork producers. J Swine Health Prod. (2013) 21:72. doi: 10.31274/ans_air-180814-28
2. Fablet C, Marois-Crehan C, Grasland B, Simon G, Rose N. Factors associated with herd-level PRRSV infection and age-time to seroconversion in farrow-to-finish herds. Vet Microbiol. (2016) 192:10–20. doi: 10.1016/j.vetmic.2016.06.006
4. Malpica JM, Fraile A, Moreno I, Obies CI, Drake JW, García-Arenal F. The rate and character of spontaneous mutation in an RNA virus. Genetics. (2002) 162:1505–11. doi: 10.1093/genetics/162.4.1505
5. Pal C, Maciá MD, Oliver A, Schachar I, Buckling A. Coevolution with viruses drives the evolution of bacterial mutation rates. Nature. (2007) 450:1079–81. doi: 10.1038/nature06350
6. Neumann EJ, Kliebenstein JB, Johnson CD, Mabry JW, Bush EJ, Seitzinger AH, et al. Assessment of the economic impact of porcine reproductive and respiratory syndrome on swine production in the United States. J Am Vet Med Assoc. (2005) 227:385–92. doi: 10.2460/javma.2005.227.385
7. Pileri E, Mateu E. Review on the transmission porcine reproductive and respiratory syndrome virus between pigs and farms and impact on vaccination. Vet Res. (2016) 47:108. doi: 10.1186/s13567-016-0391-4
8. Cavanagh D, Brian D, Enjuanes L, Holmes K, Lai M, Laude H, et al. Recommendations of the coronavirus study group for the nomenclature of the structural proteins, mRNAs, and genes of coronaviruses. Virology. (1990) 176:306–307. doi: 10.1016/0042-6822(90)90259-T
9. Dokland T. The structural biology of PRRSV. Virus Res. (2010) 154:86–97. doi: 10.1016/j.virusres.2010.07.029
10. Pesente P, Rebonato V, Sandri G, Giovanardi D, Ruffoni LS, Torriani S. Phylogenetic analysis of ORF5 and ORF7 sequences of porcine reproductive and respiratory syndrome virus (PRRSV) from PRRS-positive Italian farms: a showcase for PRRSV epidemiology and its consequences on farm management. Vet Microbiol. (2006) 114:214–24. doi: 10.1016/j.vetmic.2005.11.061
11. Shi M, Lam TT-Y, Hon C-C, Hui K-H, Faaberg KS, Wennblom T, et al. Molecular epidemiology of PRRSV: a phylogenetic perspective. Virus Res. (2010) 154:7–17. doi: 10.1016/j.virusres.2010.08.014
12. Martín-Valls G, Kvisgaard LK, Tello M, Darwich L, Cortey M, Burgara-Estrella A, et al. Analysis of ORF5 and full-length genome sequences of porcine reproductive and respiratory syndrome virus isolates of genotypes 1 and 2 retrieved worldwide provides evidence that recombination is a common phenomenon and may produce mosaic isolates. J Virol. (2014) 88:3170–81. doi: 10.1128/JVI.02858-13
13. Kuhn JH, Lauck M, Bailey AL, Shchetinin AM, Vishnevskaya TV, Bào Y, et al. Reorganization and expansion of the nidoviral family Arteriviridae. Arch Virol. (2016) 161:755–68. doi: 10.1007/s00705-015-2672-z
14. Mardassi H, Mounir S, Dea S. Identification of major differences in the nucleocapsid protein genes of a Quebec strain and European strains of porcine reproductive and respiratory syndrome virus. J Gen Virol. (1994) 75:681–5. doi: 10.1099/0022-1317-75-3-681
15. Linhares D, Cano J, Torremorell M, Morrison RB. Comparison of time to PRRSv-stability and production losses between two exposure programs to control PRRSv in sow herds. Prev Vet Med. (2014) 116:111–9. doi: 10.1016/j.prevetmed.2014.05.010
16. Jara M, Rasmussen DA, Corzo CA, Machado G. Porcine reproductive and respiratory syndrome virus dissemination across pig production systems in the United States. Transboundary Emerg Dis. (2021) 68:667–83. doi: 10.1101/2020.04.09.034181
17. Frias-De-Diego A, Jara M, Escobar LE. Papillomavirus in wildlife. Front Ecol Evol. (2019) 7:406. doi: 10.3389/fevo.2019.00406
18. Jara M, Escobar LE, Rodriges RO, Frias-De-Diego A, Sanhueza J, Machado G. Spatial distribution and spread potential of sixteen Leptospira serovars in a subtropical region of Brazil. Transboundary Emerg Dis. (2019) 66:2482–95. doi: 10.1111/tbed.13306
19. Jara M, Frias-De-Diego A, Machado G. Phylogeography of equine infectious anemia virus. Front Ecol Evol. (2020) 8:127. doi: 10.3389/fevo.2020.00127
20. Krasteva S, Jara M, Frias-De-Diego A, Machado G. Nairobi Sheep Disease Virus: A Historical and Epidemiological Perspective. Fron Vet Sci. (2020) 7:419. doi: 10.3389/fvets.2020.00419
21. Suárez P, Zardoya R, Martín MJ, Prieto C, Dopazo J, Solana A, et al. Phylogenetic relationships of European strains of porcine reproductive and respiratory syndrome virus (PRRSV) inferred from DNA sequences of putative ORF-5 and ORF-7 genes. Virus Res. (1996) 42:159–65. doi: 10.1016/0168-1702(95)01305-9
22. Dudas G, Bedford T. The ability of single genes vs full genomes to resolve time and space in outbreak analysis. BMC Evol Biol. (2019) 19:1–17. doi: 10.1186/s12862-019-1567-0
23. Stadejek T, Oleksiewicz M, Potapchuk D, Podgorska K. Porcine reproductive and respiratory syndrome virus strains of exceptional diversity in eastern Europe support the definition of new genetic subtypes. J Gen Virol. (2006) 87:1835–41. doi: 10.1099/vir.0.81782-0
24. Schwarze K, Buchanan J, Fermont JM, Dreau H, Tilley MW, Taylor JM, et al. The complete costs of genome sequencing: a microcosting study in cancer and rare diseases from a single center in the United Kingdom. Genet Med. (2020) 22:85–94. doi: 10.1038/s41436-019-0618-7
25. Snel B, Bork P, Huynen MA. Genome phylogeny based on gene content. Nat Genet. (1999) 21:108–10. doi: 10.1038/5052
26. Du W, Cao Z, Wang Y, Sun Y, Blanzieri E, Liang Y. Prokaryotic phylogenies inferred from whole-genome sequence and annotation data. BioMed Res Int. (2013) 2013:409062. doi: 10.1155/2013/409062
27. Spatafora JW, Sung G-H, Johnson D, Hesse C, O'rourke B, et al. A five-gene phylogeny of Pezizomycotina. Mycologia. (2006) 98:1018–28. doi: 10.1080/15572536.2006.11832630
28. Vitorino L, Chelo IM, Bacellar F, Ze-Ze L. Rickettsiae phylogeny: a multigenic approach. Microbiology. (2007) 153:160–8. doi: 10.1099/mic.0.2006/001149-0
29. Aria M, Cuccurullo C. Bibliometrix: an R-tool for comprehensive science mapping analysis. J Informetrics. (2017) 11:959–75. doi: 10.1016/j.joi.2017.08.007
30. Kumar S, Stecher G, Li M, Knyaz C, Tamura K. MEGA X: molecular evolutionary genetics analysis across computing platforms. Mol Biol Evol. (2018) 35:1547–9. doi: 10.1093/molbev/msy096
31. Martin DP, Murrell B, Golden M, Khoosal A, Muhire B. RDP4: Detection and analysis of recombination patterns in virus genomes. Virus Evol. (2015) 1:vev003. doi: 10.1093/ve/vev003
32. Kalyaanamoorthy S, Minh BQ, Wong TK, Von Haeseler A, Jermiin LS. ModelFinder: fast model selection for accurate phylogenetic estimates. Nat Methods. (2017) 14:587–9. doi: 10.1038/nmeth.4285
33. Nguyen L-T, Schmidt HA, Von Haeseler A, Minh BQ. IQ-TREE: a fast and effective stochastic algorithm for estimating maximum-likelihood phylogenies. Mol Biol Evol. (2015) 32:268–74. doi: 10.1093/molbev/msu300
34. Tavaré S. Some probabilistic and statistical problems in the analysis of DNA sequences. Lect Math Life Sci. (1986) 17:57–86
35. Bouckaert R, Heled J, Kühnert D, Vaughan T, Wu CH, Xie D, et al. BEAST 2: a software platform for Bayesian evolutionary analysis. PLoS Comput Biol. (2014) 10:e1003537. doi: 10.1371/journal.pcbi.1003537
36. Drummond AJ, Ho SY, Phillips MJ, Rambaut A. Relaxed phylogenetics and dating with confidence. PLoS Biol. (2006) 4:e88. doi: 10.1371/journal.pbio.0040088
37. Gill MS, Lemey P, Bennett SN, Biek R, Suchard MA. Understanding past population dynamics: bayesian coalescent-based modeling with covariates. Syst Biol. (2016) 65:1041–56. doi: 10.1093/sysbio/syw050
38. Hill V, Baele G. Bayesian estimation of past population dynamics in BEAST 1.10 using the Skygrid coalescent model. Mol Biol Evol. (2019) 36:2620–8. doi: 10.1093/molbev/msz172
39. Wang C, Wu B, Amer S, Luo J, Zhang H, Guo Y, et al. Phylogenetic analysis and molecular characteristics of seven variant Chinese field isolates of PRRSV. BMC Microbiol. (2010) 10:1–11. doi: 10.1186/1471-2180-10-146
40. Fekede RJ, Van Gils H, Huang L, Wang X. High probability areas for ASF infection in China along the Russian and Korean borders. Transboundary Emerg Dis. (2019) 66:852–64. doi: 10.1111/tbed.13094
41. Mongeon P, Paul-Hus A. The journal coverage of web of science and scopus: a comparative analysis. Scientometrics. (2016) 106:213–28. doi: 10.1007/s11192-015-1765-5
42. Frias-De-Diego A, Posey R, Pecoraro BM, Fernandes Carnevale R, Beaty A, Crisci E. A century of swine influenza: is it really just about the pigs? Vet Sci. (2020) 7:189. doi: 10.3390/vetsci7040189
43. Alkhamis MA, Perez AM, Murtaugh MP, Wang X, Morrison RB. Applications of bayesian phylodynamic methods in a recent US porcine reproductive and respiratory syndrome virus outbreak. Front Microbiol. (2016) 7:67. doi: 10.3389/fmicb.2016.00067
44. Yu F, Yan Y, Shi M, Liu H-Z, Zhang H-L, Yang Y-B, et al. Phylogenetics, genomic recombination, and NSP2 polymorphic patterns of porcine reproductive and respiratory syndrome virus in China and the United States in 2014–2018. J Virol. (2020) 94:e01813–19. doi: 10.1128/JVI.01813-19
45. Hall DH, Liu Y, Shub DA. Exon shuffling by recombination between self-splicing introns of bacteriophage T4. Nature. (1989) 340:574–6. doi: 10.1038/340574a0
46. Comeron JM, Kreitman M. The correlation between intron length and recombination in Drosophila: dynamic equilibrium between mutational and selective forces. Genetics. (2000) 156:1175–90. doi: 10.1093/genetics/156.3.1175
47. Duret L. Why do genes have introns? Recombination might add a new piece to the puzzle. Trends Genet. (2001) 17:172–5. doi: 10.1016/S0168-9525(01)02236-3
Keywords: bibliometrics, phylodynamics, pig, PRRSV, ORF5, whole genome
Citation: Frias-De-Diego A, Jara M, Pecoraro BM and Crisci E (2021) Whole Genome or Single Genes? A Phylodynamic and Bibliometric Analysis of PRRSV. Front. Vet. Sci. 8:658512. doi: 10.3389/fvets.2021.658512
Received: 25 January 2021; Accepted: 21 May 2021;
Published: 24 June 2021.
Edited by:
Lester J. Perez, University of Illinois at Urbana–Champaign, United StatesReviewed by:
Moh A. Alkhamis, Kuwait University, KuwaitRafael Zardoya, National Museum of Natural Sciences (MNCN), Spain
Copyright © 2021 Frias-De-Diego, Jara, Pecoraro and Crisci. This is an open-access article distributed under the terms of the Creative Commons Attribution License (CC BY). The use, distribution or reproduction in other forums is permitted, provided the original author(s) and the copyright owner(s) are credited and that the original publication in this journal is cited, in accordance with accepted academic practice. No use, distribution or reproduction is permitted which does not comply with these terms.
*Correspondence: Elisa Crisci, ZWNyaXNjaUBuY3N1LmVkdQ==