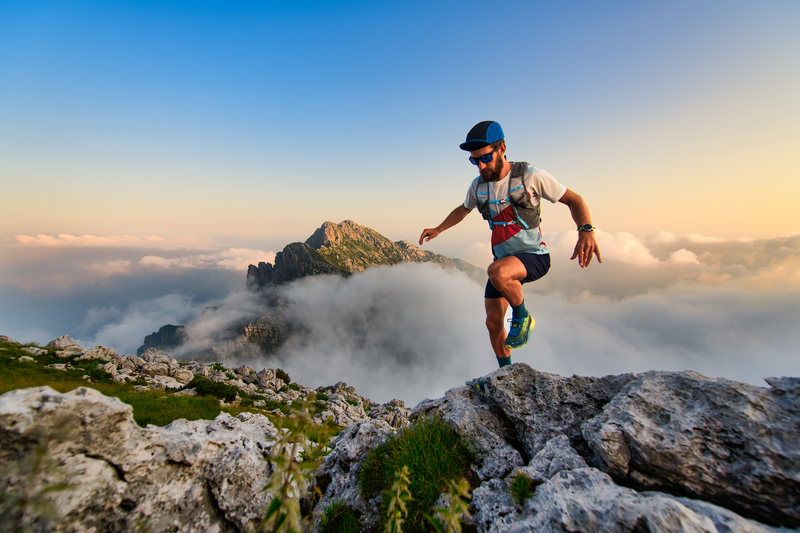
94% of researchers rate our articles as excellent or good
Learn more about the work of our research integrity team to safeguard the quality of each article we publish.
Find out more
ORIGINAL RESEARCH article
Front. Vet. Sci. , 10 June 2021
Sec. Animal Nutrition and Metabolism
Volume 8 - 2021 | https://doi.org/10.3389/fvets.2021.650248
This article is part of the Research Topic Nutritional Requirements in Production Animals View all 8 articles
Microbial crude protein (MCP) produced in rumen could be estimated by a variety of protocols of experimental sampling and analysis. However, a model to estimate this value is necessary when protein requirements are calculated for small ruminants. This model could be useful to calculate rumen degradable protein (RDP) requirements from metabolizable protein (MP). Then, our objective was to investigate if there is a difference in MCP efficiency between sheep and goats, and to fit equations to predict ruminal MCP production from dietary energy intake. The database consisted of 19 studies with goats (n = 176) and sheep (n = 316), and the variables MCP synthesis (g/day), total digestible nutrients (TDN), and organic matter (OM) intakes (g/day), and OM digestibility (g/kg DM) were registered for both species. The database was used for two different purposes, where 70% of the values were sorted to fit equations, and 30% for validation. A meta-analytical procedure was carried out using the MIXED procedure of SAS, specie was considered as the fixed dummy effect, and the intercept and slope nested in the study were considered random effects. No effect of specie was observed for the estimation of MCP from TDN, digestible Organic Matter (dOM), or metabolizable energy (ME) intakes (P > 0.05), considering an equation with or without an intercept. Therefore, single models including both species at the same fitting were validated. The following equations MCP (g/day) = 12.7311 + 59.2956 × TDN intake (AIC = 3,004.6); MCP (g/day) = 15.7764 + 62.2612 × dOM intake (AIC = 2,755.1); and MCP (g/day) = 12.7311 + 15.3000 × ME intake (AIC = 3,007.3) presented lower values for the mean square error of prediction (MSEP) and its decomposition, and similar values for the concordance correlation coefficient (CCC) and for the residual mean square error (RMSE) when compared with equations fitted without an intercept. The intercept and slope pooled test was significant for equations without an intercept (P < 0.05), indicating that observed and predicted data differed. In contrast, predicted and observed data for complete equations were similar (P > 0.05).
Microbial crude protein (MCP) synthesized in the rumen is dependent on the energy supply (1–4). It is known that MCP synthesis is maximized when fermentable carbohydrates and N compounds are supplied synchronously (5, 6). Therefore, main feeding systems preconize models to estimate MCP based on energy compounds as digestible OM (dOM), metabolizable energy (ME), or TDN (7–13), once these models could be used to calculate rumen degradable protein (RDP) requirements from metabolizable protein (MP) requirements. Moreover, the general importance of the estimation of microbial protein for ruminants must be considered, as it accounts for between 50 and 90% of the protein entering the duodenum and provides most of the amino acids needed for growth and milk protein synthesis (14, 15).
According to Dijkstra et al. (16), empirical equations in most protein evaluation systems usually consider MCP synthesis calculated from the amount of available energy, applying a fixed or variable yield of microbial protein formed per unit energy degraded. As reported by Galyean and Tedeschi (4), the validity of predicting MCP synthesis from TDN intake using the factor of 0.13, mostly used in American systems for cattle, has been neither extensively assessed experimentally nor evaluated. Hence, theses authors proposed equations with an intercept included. On the other hand, this information for goats and sheep is scarce, and little information could be found in the literature. NRC (9) proposed to predict crude protein (CP) requirements without an equation to estimate MCP and RDP requirements, which was based on percentage true protein in MCP, in average CP digestibility, and the percentage of undegradable dietary protein (UDP) in CP. This kind of simplification could reduce the accuracy of nutrient requirement predictions in sheep and goats, mainly when using tropical forages. Diets based on low-quality forages usually require an improved synchronization between energy and protein to achieve the maximum degradation profile.
When sheep and goats are compared in the fermentation process without diet interference, little differences are observed in pH, volatile fatty acids, and ammonia nitrogen concentrations in rumen fluid. However, interspecies differences in ruminal microbial composition are found, favoring goats in the concentration of rumen microbial protein (17, 18). Carro et al. (18) compared methods to estimate MCP in sheep and goats and reported that there were no interspecies differences in total purine derivative excretion in urine. Besides, no differences were detected when ruminal bacteria production (liquid or solid associated) was compared between species, and no dietary interactions were found in this research. Then, our objective was to investigate if there is a difference in MCP efficiency between sheep and goats, and to fit equations to predict ruminal MCP production from dietary energy intake.
A complete database composed of individual experimental units from sheep and goat trials was obtained to conduct a meta-analysis. The spreadsheet was composed of general information (e.g., title, author name, breed, sex, treatment, type of diet) and all necessary quantitative data. The original database consisted of 19 studies (Appendix) with goats (6 studies), sheep (12 studies), and with both species (1 study), conducted at the Federal University of Bahia, Bahia Southwest State University, Federal University of Ceará, Federal University of Paraiba, and Santa Cruz State University facilities between 2010 and 2019. The detailed descriptive statistics of the database filtered for each nutrient evaluation were described in Table 1. Data were collected when MCP synthesis (g/day), TDN intake (kg/day) (Figure 1), OM intake (kg/day) (Figure 2), CP intake (kg/day), and OM digestibility (g/kg DM) were measured for sheep or goat. The TDN data were converted in ME (Figure 3) by the constants 4.404 (8), which converts TDN to digestible energy (DE), and 0.88 (19), which converts DE in ME, resulting in the following conversion factor: ME intake (Mcal/day) = 3.87552 × TDN intake (kg/day).
Table 1. Descriptive statistics of the variables used to fit and validate models to estimate microbial crude protein efficiency in the rumen of goat and sheep species from energy intake measurements.
Figure 1. Total databank used to establish the relationship between total digestible nutrient (TDN) intake (kg/day) and microbial crude protein (MCP—g/day) produced in the rumen of sheep and goats, made from 19 experiments (n = 492).
Figure 2. Total databank used to establish the relationship between digestible organic matter (dOM) intake (kg/day) and microbial crude protein (MCP—g/day) produced in the rumen of sheep and goats, made from 18 experiments (n = 435).
Figure 3. Total databank used to establish the relationship between metabolizable energy (ME) intake (Mcal/day) and microbial crude protein (MCP—g/day) produced in the rumen of sheep and goats, made from 19 experiments (n = 492).
The animals included in the database were some of the most common genotypes of sheep and goat species used in tropical systems: pure breed Santa Inês sheep, crossbred sheep (Santa Inês × Dorper; Santa Inês × local non-defined breed), Morada Nova sheep, Bergamacia sheep, Boer goats, crossbred Boer goats × dairy breeds, and Saanen and Anglo-Nubian goats. In all experiments evaluated, animals were managed under tropical conditions, in feedlot management, and fed ad libitum.
The forage sources included Tifton-85 hay, Buffel grass hay, Cassava hay, Elephant grass silage, sorghum silage, corn silage, and pearl millet silage. The concentrate components included soybean hulls, peanut cake, cottonseed cake, palm kernel cake, detoxified castor cake, spineless cactus, cottonseed hulls, ground corn, soybean meal, and crude glycerin. All animals were supplemented with a mineral mixture in the concentrate components. The relationship between forage and concentrate ranged from 200 to 700 g of forage/kg of fresh feed. Nutrient intake was recorded daily in all the experiments, and values per animal for each experiment were recorded into the database. At least organic matter (OM) digestibility or total digestible nutrients (TDN) were required to incorporate the study in the database. Digestibility trials that generate these data were carried out using total feces collection or its prediction using different internal markers. Microbial crude protein production was measured by the purine derivative method or rumen bacterial RNA isolation.
The database was divided into two subsets, in which 70% of the data were used to fit the proposed models, and 30% were used to validate the equations. Data were randomly divided using the Excel software, where both subsets had data from all 30 experiments.
Two different approaches were used to predict MCP production, where independent variables were the TDN intake and the dOM intake. The TDN intake was used to calculate the ME intake, generating a third approach. These constructions were made based on 70% of the dataset separated for this finality. All models were evaluated with and without an intercept. The effect of animal specie (sheep or goat) was investigated by adding a dummy variable (20). A complete model was defined to consider also the inter-experiment variation, as follows:
where Yijk = amount of MCP in the rumen (g/day) in the kth observation into experiment jth and specie ith; Xijk = Intake of TDN, dOM, or ME (kg/day) in the kth observation into experiment jth and specie ith; Di = dummy variable referent to the ith animal species with D = 0 for sheep and D = 1 for goat; ß1 and ß2 = estimated slopes for sheep and goats, respectively; α1 and α2 = estimated intercepts for sheep and goats, respectively; E(i)j = effect of the jth experiment nested with the ith animal specie; and εijk = random error.
In case the parameters associated with the dummy variables would not be statistically significant for some independent variables tested, a reduced model was fitted without considering the D effect, as follows:
where Yijk = amount of MCP in the rumen (g/day) in the kth observation into experiment jth and specie ith; Xijk = intake of TDN, dOM, or ME (kg/day) in the kth observation into experiment jth and specie ith; β1 = estimated slope for small ruminants; α1 = estimated intercept for small ruminants; E(i)j = effect of the jth experiment nested with the ith animal specie; and εijk is the random error.
Same inferences were produced to evaluate the above models without the intercept. Theoretically, the absence of an intercept could make biological sense because, in the absence of energy intake (slope = 0), represented by TDN, dOM, and ME, no amount of MCP could be produced, and then two other approaches were tested, as follows:
Meta-analysis techniques (21) were applied. The MIXED procedure of SAS 9.2 (22) was used to estimate the coefficients, considering the influence of the species on the intercept and the slope (D = 0 for sheep and D = 1 for goat). The random effects of the experiments were considered both on the intercept and slopes (SUBJECT = study) according to Huhtanen et al. (23). It should be emphasized that linear regression models were analyzed considering an unstructured (co)variance matrix (TYPE = UN). All variance components in the linear models were estimated using the restricted maximum likelihood method. For all statistical procedures, the critical level of the probability for type-I error was fixed at 0.05.
Evaluation of the equations was carried out from the 30% remaining subset. A total of 153 experimental units were used in this process, and the completely descriptive characterization of these data was presented in Table 1. Mathematical comparisons were performed using the MES software (Model Evaluation System, version 3.1, Texas A&M University, College Station, TX, USA) according to Tedeschi (24), after calculating Mayer's intercept and slope test (25), the root mean square of error (RMSE), the concordance correlation coefficient (CCC), the mean bias, slope bias, and random errors decomposed from the mean square error of prediction (MSEP), the predicted mean, and the mean deviation. All statistical procedures were performed using 0.05 as the critical level of probability for type I error occurrence.
A complete model with an intercept was adjusted to estimate MCP production (g/day), considering TDN intake (kg/day) as the independent variable (Table 2). The inclusion of both a slope (P = 0.39) and an intercept (P = 0.88) associated with the dummy variable did not improve the equation (AIC = 2,989.7). The specie factor was not statistically necessary to construct an adequate model. Therefore, the reduced model presentation (AIC = 3,004.6) had both a slope and an intercept fitted (P < 0.01), producing the following equation:
The same scenario was observed when the TDN intake model was fitted without an intercept (Table 2). The inclusion of the slope (P = 0.23) associated with the dummy variable did not improve the equation (AIC = 3,012.9). The specie factor was also not statistically necessary to construct an adequate model. Therefore, the reduced model presentation (AIC = 3,021.5) had the slope fitted (P < 0.01) and can also be considered as an alternative equation:
The two above equations (Equations 5 and 6) were evaluated by a validation process (Table 3). Predicted and observed data from the equation fitted with an intercept (Equation 5) for TDN intake were similar (P = 0.43). Therefore, it is possible to infer that this equation is adequate to predict MCP in sheep and goats. The same inference could not be made for the equation (Equation 6) without an intercept (Table 3), which had a difference between predicted and observed data (P = 0.04). Comparison relative to MSEP and its decomposition was also positive for the equation with an intercept (Equation 5), once MSEP was lower for that, and only 0.06% slope bias was counted. Besides, random errors prevailed in the equation with an intercept for TDN intake (Equation 5), which is suitable. However, both equations (Equations 5 and 6) with or without an intercept had no difference in the predicted mean (P > 0.05) and similar values for CCC and RMSE.
Table 2. Estimated parameters and statistic evaluation of the relationships between intake of total digestible nutrients (TDN), of digestible organic matter (dOM), and of metabolizable energy (ME) intakes with ruminal microbial crude protein in complete (different parameters for each specie) and reduced (same parameters for both species) modes, where the letter “D” represents a dummy variable (D = 0 for goat and D = 1 for sheep), with models with and without intercept (Noint).
Table 3. General evaluation of the proposed models, with or without intercept (Noint), to estimate microbial crude protein efficiency in sheep and goat species from energy intake measurements.
A complete model with an intercept was adjusted to estimate MCP production (g/day), considering dOM intake (kg/day) as the independent variable (Table 2). The inclusion of both a slope (P = 0.36) and an intercept (P = 0.93) associated to the dummy variable did not improve the equation (AIC = 2,739.7). Therefore, the reduced model presentation (AIC = 2,755.1) had both a slope and an intercept fitted (P < 0.05), producing the following equation:
The same scenario was observed when the dMO intake model was fitted without an intercept (Table 2). The inclusion of a slope (P = 0.16) associated to the dummy variable did not improve the equation (AIC = 2,761.4). Therefore, the reduced model presentation (AIC = 2,769.9) had the slope fitted (P < 0.01) and can also be considered as an alternative equation:
The two above equations (Equations 5 and 6) were compared by a validation process (Table 3). Predicted and observed data from the equation fitted with an intercept (Equation 7) for dMO intake were similar (P = 0.57), which could lead to infer that this equation is adequate to predict MCP in sheep and goats. The same inference could be made for the equation (Equation 8) without an intercept (Table 3), which had no difference between predicted and observed data (P < 0.36). Comparison relative to MSEP and its decomposition was positive for the equation with an intercept (Equation 7), once MSEP was lower for that, and a 0.65% slope bias was counted contrasting with 1.45% for the equation without an intercept. Also, random errors prevailed in the equation with an intercept for dMO intake (Equation 7), which is suitable. However, both equations (Equations 7 and 8) with or without an intercept had no difference in the predicted mean (P > 0.05) and similar values for CCC and RMSE.
A complete model with an intercept was adjusted to estimate MCP production (g/day), considering ME intake (Mcal/day) as the independent variable (Table 2). The inclusion of both a slope (P = 0.39) and an intercept (P = 088) associated with the dummy variable did not improve the equation (AIC = 2,995.2). Therefore, the reduced model presentation (AIC = 3,007.3) had both a slope and an intercept fitted (P < 0.05), producing the equation
The same scenario was observed when the ME intake model was fitted without an intercept (Table 2). The inclusion of a slope (P = 0.23) associated with the dummy variable did not improve the equation (AIC = 3,018.3). Therefore, the reduced model presentation (AIC = 3,024.2) had the slope fitted (P < 0.01) and can also be considered as an alternative equation:
The two above equations (Equations 9 and 10) were compared by a validation process (Table 3). Predicted and observed data from the equation fitted with an intercept (Equation 9) for ME intake were similar (P = 0.43). Therefore, it is possible to infer that this equation is adequate to predict MCP in sheep and goats. The same inference could not be made for the equation (Equation 10) without an intercept (Table 3), which had a difference between predicted and observed data (P = 0.04). Comparison relative to MSEP and its decomposition was also positive for the equation with an intercept (Equation 9), once MSEP was lower for that, and only 0.06% slope bias was counted. Besides, random errors prevailed in the equation with an intercept for MEI (Equation 9), which is suitable. However, both equations (Equations 9 and 10) with or without an intercept had no difference in the predicted mean (P > 0.05) and similar values for CCC and RMSE.
The relationship between MCP synthesis and energy available in the rumen was well-documented. The synchronism between protein and energy degradation in the rumen is one of the factors that impacts MCP efficiency (26–30). According to Schwab and Broderick (31), when there is excessive N relative to energy compounds in the rumen, the ruminal N waste increases. This result means that lower levels of N compounds were incorporated to MCP synthesized in the rumen due to the reduced carbohydrate sources. Then, it is known that MCP synthesis is driven by the ruminant's energy intake.
Energy intake and its availability in rumen could be expressed in different forms. The dOM intake directly expresses the energy that can be used by the microbial population in the rumen, once it represents the sum of all nutrients that can generate energy and that can be accessed by microorganisms. The mathematical relationship between MCP synthesis and intake of dOM was established by Gomes et al. (32) when they supplied increasing levels of soluble starch to sheep and observed the increase in dMO intake and microbial N synthesis linearly.
Sinclair et al. (33) obtained similar results in sheep, but these authors highlighted the importance of synchronization between these nutrients to improve the dMO utilization by rumen microorganisms. Stern and Hoover (34) made a compilation of 64 manuscripts published from 1970 to 1979, and they observed a mean value of 16.9 g (±6.2) of MCP synthesized in rumen per 100 g of dOM for sheep and cattle, being the values ranging from 6.3 to 30.7 g of MCP. The authors attributed this difference to the variety of microbial markers used, content and source of N and carbohydrates, rumen dilution rate, dietary sulfur, and feed frequency. In this study, it can be noted through Equation (8) that the relationship found for sheep and goats was 8.26 g of MCP synthesized in rumen per 100 g of dOM, where diversity of dietary types was included in the dataset.
The TDN intake as a base for MCP estimation started to be further investigated also in the 1970s. However, most studies were started in cattle to predict RDP and UDP requirements better. Burroughs et al. (35) proposed that MCP synthesis averaged 13.05% of TDN for cattle. Burroughs et al. (36) reported that estimation of MCP synthesis could be from 10.4% of TDN based upon three evaluations: the first is that approximately 52% of TDN is digested in the rumen; the second is that 25% of the digested TDN becomes MCP when adequate N is present; the third is that 80% of MCP is alpha-amino protein.
According to Hackmann and Firkins (37), the knowledge about energy metabolism in rumen microorganisms is the key to understand how to improve growth efficiency and how to predict microbial protein production. The MCP synthesis prediction is a necessary step to improve the protein requirement' systems. In years later, TDN was adopted as a pattern to predict MCP synthesis and RDP by NRC (38), allowing this system to convert MP requirements into crude protein requirements for beef cattle. This committee refused the two equations proposed by the “Ruminant Nitrogen Usage” committee in 1985 (39), which were based in estimates generated by different forage to concentrate ratios and a negative intercept for the relationship between MCP and TDN [MCP (g/day) = 6.25 – 31.86 + 26.12 × TDN (kg/day)]. NRC (40) highlighted that a negative intercept had no biological logic and that equations based on the forage-to-concentrate ratio are misleading because it suggests that both intake and concentration of TDN change in a similar direction. For dairy cattle, NRC (8) also adopted the constant 130 g MCP/kg TDN for heifers and cows, and they proposed that the RDP requirement could be calculated by 1.18 × MCP yield.
Russell et al. (41), when proposing the CNCPS system, made some criticism concerning TDN for MCP prediction. The authors stated that ruminal microbial growth is driven by available carbohydrates rather than TDN, considering that TDN is also composed of EE and CP besides total carbohydrates, and they would not be the primary source of energy for microorganisms in the rumen. However, according to Lucas and Smart (42), EE and CP and NFC are nutritional entities with constant digestion rate, represented by a slope between consumed and digested nutrients, and with an intercept that represents the endogenous fraction. In other words, NDF is the key nutrient impacting the most variability in TDN, while the other components would be mostly driven by nutrient intake. Thus, it can be seen that the main criticism that 40 directed to TDN could be questioned once TDN can reflect the oscillations in the carbohydrate fermentation rate.
Therefore, even though TDN was questioned for the MCP estimate, the most recent American System for beef cattle (12) continued using the TDN as the MCP predictor, adopting two equations proposed by Galyean and Tedeschi (4). These authors proposed an equation to estimate MCP based only on TDN intake (MCP = 0.087 × TDNI + 42.73) but with an adjustment in the equation parameters when diets have high EE (MCP = 0.096 × TDNI + 53.33). NASEM (12) recognized that microbial growth is measured with high variability, which impacts directly in the standard MSEPs and indirectly in the MP requirement prediction. However, the authors also recognized that this high variability is caused by the available techniques and depends on many other variables that cannot be accounted for by a single empirical equation.
The Brazilian system for beef cattle (11) was based exclusively on data from tropical forages, as well as this work that was made for sheep and goats. The BR-Corte (11) preconized equations based both in TDN and dMO, differing in their quadratic parameters, and CP as a component of those equations. However, it must be emphasized that the data of this work did not fit in this type of model when tested. This lack of fit probably is due to the difference in the specie type, and the number of observations; once the cited system has used 2,102 individual observations, while this work was proposed with 592 observations to fit equations.
For sheep and goats, NRC (9) adopted only one approach. This system presented a basic simplification proposed by Sahlu et al. (43) and imported from NRC (40). NRC (9) stated that even though MP requirements are preferred compared with CP, for some users, the application of MP requirements might be difficult. In the absence of an equation capable to predict MCP for sheep and goats, this system took into consideration that MCP is 0.64 of CP, which corresponds to 80% of true protein and 80% of digestibility, and that 16% of CP is UDP. These premises led to the following simplification: CP = MP/[64 + (0.16 × %UDP)/100], which allowed NRC (9) to predict CP requirements without proposing an equation to estimate MCP and RDP requirements. This kind of simplification could reduce the accuracy of nutrient requirement predictions in sheep and goats, mainly when using tropical forages, which require an improved synchronization between energy and protein to achieve the maximum degradation profile.
CSIRO (10), working with cattle and sheep, proposed a specific equation based on ME intake, applying an adjustment for the intake level above maintenance. This system proposed some adjustments for the latitude of the region, the day of the year, and the type of forage. All the proposed models were exponential, always considering the intake level as an exponential parameter and the ME intake as a slope parameter. In this work, an equation based on the ME intake was also proposed because this is the preferred energy unit for some nutrient requirement systems. The contribution of the adequate MCP prediction is basically to improve dietary balance for sheep and cattle, minimizing environmental N excretions and maximizing animal performance. These findings can contribute to environmental and economic sustainability in small ruminant production systems.
There are no differences to fit MCP efficiency between sheep and goats. The MCP synthesis in the rumen can be predicted from energy intake using combined equations that encompass both sheep and goats, once the specie was not a factor affecting this prediction. The approaches for this prediction can be based on NDT, dOM, or ME intake by the following equations: MCP (g/day) = 12.7311 + 59.2956 × TDN intake (AIC = 3,004.6); MCP (g/day) = 15.7764 + 62.2612 × dOM intake (AIC = 2,755.1); and MCP (g/day) = 12.7311 + 15.3000 × ME intake (AIC = 3,007.3). The same approaches were tested after deleting intercept parameters from the models, but after the validation process, these equations were not accurate satisfactorily.
The raw data supporting the conclusions of this article will be made available by the authors, without undue reservation.
SS: writing—original draft, data curation, conceptualization, methodology, formal analysis, supervision, and project administration. GC: conceptualization, data curation, and investigation. JA, ES, MP, EP, AP, and LL: data curation and investigation. DZ: validation, methodology, and formal analysis. SV, IT: conceptualization, visualization, and validation. MT and LM: visualization and validation.
This work was supported by the Coordination for the Improvement of Higher Education Personnel (CAPES), Brazilian National Council for Scientific and Technological Development (CNPq), Bahia State Research Support Foundation (FAPESB), and the National Institute of Science and Technology in Animal Science (INCT—CA).
The authors declare that the research was conducted in the absence of any commercial or financial relationships that could be construed as a potential conflict of interest.
The Supplementary Material for this article can be found online at: https://www.frontiersin.org/articles/10.3389/fvets.2021.650248/full#supplementary-material
DM, dry matter; CP, crude protein; RDP, rumen degradable protein; UDP, undegradable dietary protein; MP, metabolizable protein; MCP, microbial crude protein; OM, organic matter; dMO, digestible organic matter; TDN, total digestible nutrients; ME, metabolizable energy; DE, digestible energy; RMSE, root mean square of error; CCC, concordance correlation coefficient; MSEP, mean square error of prediction.
1. Clark JH, Klusmeyer TH, Cameron MR. Microbial protein synthesis flows of nitrogen fractions to the duodenum of dairy cows. J Dairy Sci. (1992) 75:2304–23. doi: 10.3168/jds.S0022-0302(92)77992-2
2. Firkins JL. Maximizing microbial protein synthesis in the rumen. J Nutr. (1996) 126:1347S−54S. doi: 10.1093/jn/126.suppl_4.1347S
3. Gozho GN, Mutsvangwa T. Influence of carbohydrate source on ruminal fermentation characteristics, performance, microbial protein synthesis in dairy cows. J Dairy Sci. (2008) 91:2726–35. doi: 10.3168/jds.2007-0809
4. Galyean ML, Tedeschi LO. Predicting microbial protein synthesis in beef cattle: relationship to intakes of total digestible nutrients crude protein. J Anim Sci. (2014) 92:5099–111. doi: 10.2527/jas.2014-8098
5. Dewhurst RJ, Davies DR, Merry RJ. Microbial protein supply from the rumen. Anim Feed Sci Technol. (2000) 85:1–21. doi: 10.1016/S0377-8401(00)00139-5
6. Richardson JM, Wilkinson RG, Sinclair LA. Synchrony of nutrient supply to the rumen dietary energy source their effects on the growth metabolism of lambs. J Anim Sci. (2003) 81:1332–47. doi: 10.2527/2003.8151332x
7. AFRC. Energy and Protein Requirements of Ruminants. An Advisory Manual Prepared by the AFRC Technical Committee on Responses to Nutrients. London: CAB International (1993).
8. National Research Council (NRC). Nutrient Requirements of Dairy Cattle. National Academies Press (2001).
9. National Research Council (NRC). Nutrient Requirements of Small Ruminants: Sheep, Goats, Cervids and New World Camelids. National Academies Press (2006).
11. BR-Corte. Nutrient Requirements of Zebu and Crossbreed Cattle BR-Corte. 3rd ed. Viçosa: Federal University of Viçosa (2016).
12. National Academies of Sciences Engineering Medicine (NASEM). Nutrient Requirements of Beef Cattle. National Academies Press (2016).
13. Noziere P, Sauvant D, Delaby L. INRA Feeding System for Ruminants. Wageningen: Academic Publishers (2018).
14. Gidlund H, Vaga M, Ahvenjärvi S, Rinne M, Ramin M, Huhtanen P. Predicting omasal flow of nonammonia N milk protein yield from in vitro-determined utilizable crude protein at the duodenum. J Dairy Sci. (2018) 101:1164–76. doi: 10.3168/jds.2017-13422
15. Broderick GA, Huhtanen P, Ahvenjärvi S, Reynal SM, Shingfield KJ. Quantifying ruminal nitrogen metabolism using the omasal sampling technique in cattle - a meta-analysis. J Dairy Sci. (2010) 93:3216–30. doi: 10.3168/jds.2009-2989
16. Dijkstra J, France J, Davies DR. Different mathematical approaches to estimating microbial protein supply in ruminants. J Dairy Sci. (1998) 81:3370–84. doi: 10.3168/jds.S0022-0302(98)75902-8
17. Hadjipanayiotou M, Antoniou T. A comparison of rumen fermentation patterns in sheep goats given a variety of diets. J. Sci. Food Agric. (1983) 34:1319–22. doi: 10.1002/jsfa.2740341202
18. Carro MD, Cantalapiedra-Hijar G, Ranilla MJ, Molina-Alcaide E. Urinary excretion of purine derivatives, microbial protein synthesis, nitrogen use, ruminal fermentation in sheep goats fed diets of different quality. J Anim Sci. (2012) 90:3963–72. doi: 10.2527/jas2011-4577
19. Teixeira IA, Almeida AK, Fernandes MH, Resende KT. Applying the California net energy system to growing goats. Transl Anim Sci. (2019) 3:999–1010. doi: 10.1093/tas/txz021
21. St-Pierre NR. Invited Review: Integrating quantitative findings from multiple studies using mixed model methodology. J Dairy Sci. (2001) 84:741–55. doi: 10.3168/jds.S0022-0302(01)74530-4
22. Littell RC, Milliken GA, Stroup WW, Wolfinger RD. SAS System for Mixed Models. Cary, NC: SAS Inst. (1996).
23. Huhtanen P, Detmann E, Krizsan SJ. Prediction of rumen fiber pool in cattle from dietary, fecal animal variables. J Dairy Sci. 99:5345–57 (2016). doi: 10.3168/jds.2015-10842
24. Tedeschi LO. Assessment of the adequacy of mathematical models. Agric Syst. 89:225–47 (2006). doi: 10.1016/j.agsy.2005.11.004
25. Mayer D, Stuart M, Swain A. Regression of real-world data on model output: an appropriate overall test of validity. Agric Syst. (1994) 45:93–104. doi: 10.1016/S0308-521X(94)90282-8
26. Nocek JE, Russell JB. Protein energy as an integrated system relationship of ruminal protein carbohydrate availability to microbial synthesis milk production. J Dairy Sci. (1988) 71:2070–107. doi: 10.3168/jds.S0022-0302(88)79782-9
27. Hoover WH, Stokes SR. Balancing carbohydrates proteins for optimum rumen microbial yield. J Dairy Sci. (1991) 74:3630–44. doi: 10.3168/jds.S0022-0302(91)78553-6
28. Chanjula P, Wanapat M, Wachirapakorn C, Rowlinson P. Effect of synchronizing starch sources protein (NPN) in the rumen on feed intake, rumen microbial fermentation, nutrient utilization performance of lactating dairy cows. Asian Australas J Anim Sci. (2004) 17:1400–10. doi: 10.5713/ajas.2004.1400
29. Hall MB, Huntington GB. Nutrient synchrony: sound in theory, elusive in practice. J Anim Sci. (2008) 86:E287–92. doi: 10.2527/jas.2007-0516
30. Zhang J, Zheng N, Shen W, Zhao S, Wang J. Synchrony degree of dietary energy and nitrogen release influences microbial community, fermentation, and protein synthesis in a rumen simulation system. Microorganisms. (2020) 8:231. doi: 10.3390/microorganisms8020231
31. Schwab CG, Broderick GA. A 100-year review: protein amino acid nutrition in dairy cows. J Dairy Sci. (2017) 100:10094–112. doi: 10.3168/jds.2017-13320
32. Gomes MJ, Hovell FD, Chen XB, Nengomasha EM, Fikremariam D. The effect of starch supplementation of straw on microbial protein supply in sheep. Anim Feed Sci Technol. (1994) 49:277–86. doi: 10.1016/0377-8401(94)90052-3
33. Sinclair LA, Garnsworthy PC, Newbold JR, Buttery PJ. Effects of synchronizing the rate of dietary energy nitrogen release in diets with a similar carbohydrate composition on rumen fermentation microbial protein synthesis in sheep. J Agric Sci. (1995) 124:463–72. doi: 10.1017/S0021859600073421
34. Stern MD, Hoover WH. Methods for determining factors affecting rumen microbial protein synthesis: a review. J Anim Sci. (1979) 49:1590–603. doi: 10.2527/jas1979.4961590x
35. Burroughs W, Nelson DK, Mertens DR. Protein physiology its application in the lactating cow: the metabolizable protein feeding standard. J Anim Sci. (1975) 41:933–44. doi: 10.2527/jas1975.413933x
36. Burroughs W, Nelson DK, Mertens DR. Evaluation of protein nutrition by metabolizable protein urea fermentation potential. J Dairy Sci. (1975) 58:611–9. doi: 10.3168/jds.S0022-0302(75)84617-0
37. Hackmann TJ, Firkins JL. Maximizing efficiency of rumen microbial protein production. Front Microbiol. (2015) 6:465. doi: 10.3389/fmicb.2015.00465
38. National Research Council (NRC). Nutrient Requirements of Beef Cattle. National Academies Press (1996).
41. Russell JB, O'connor JD, Fox DG, Van Soest PJ, Sniffen CJ. A net carbohydrate and protein system for evaluating cattle diets: I. Ruminal fermentation. J Anim Sci. (1992) 70:3551–61. doi: 10.2527/1992.70113551x
42. Lucas HL, Smart WWG. Chemical composition and the digestibility of forages. In: Proceedings of 16th Pasture and Crop Improvement Conference. Mississippi (1959).
Keywords: bacteria, goat, microorganisms, sheep, rumen, yield
Citation: Santos SA, de Carvalho GGP, Azevêdo JAG, Zanetti D, Santos EM, Pereira MLA, Pereira ES, Pires AJV, Valadares Filho SdC, Teixeira IAMdA, Tosto MSL, Leite LC and Mariz LDS (2021) Metabolizable Protein: 1. Predicting Equations to Estimate Microbial Crude Protein Synthesis in Small Ruminants. Front. Vet. Sci. 8:650248. doi: 10.3389/fvets.2021.650248
Received: 06 January 2021; Accepted: 26 April 2021;
Published: 10 June 2021.
Edited by:
Polyana Pizzi Rotta, Universidade Federal de Viçosa, BrazilReviewed by:
Yosra Ahmed Soltan, Alexandria University, EgyptCopyright © 2021 Santos, de Carvalho, Azevêdo, Zanetti, Santos, Pereira, Pereira, Pires, Valadares Filho, Teixeira, Tosto, Leite and Mariz. This is an open-access article distributed under the terms of the Creative Commons Attribution License (CC BY). The use, distribution or reproduction in other forums is permitted, provided the original author(s) and the copyright owner(s) are credited and that the original publication in this journal is cited, in accordance with accepted academic practice. No use, distribution or reproduction is permitted which does not comply with these terms.
*Correspondence: Stefanie Alvarenga Santos, c3RlZmFuaWUuYWx2YXJlbmdhQHVmYmEuYnI=
Disclaimer: All claims expressed in this article are solely those of the authors and do not necessarily represent those of their affiliated organizations, or those of the publisher, the editors and the reviewers. Any product that may be evaluated in this article or claim that may be made by its manufacturer is not guaranteed or endorsed by the publisher.
Research integrity at Frontiers
Learn more about the work of our research integrity team to safeguard the quality of each article we publish.