- 1Centre for Agricultural Bioinformatics, Indian Council of Agricultural Research (ICAR)-Indian Agricultural Statistics Research Institute, New Delhi, India
- 2Indian Council of Agricultural Research (ICAR)-Central Institute for Research on Buffaloes, Hisar, India
- 3Animal Biotechnology Centre, Indian Council of Agricultural Research (ICAR)-National Dairy research Institute, Karnal, India
Water buffalo (Bubalus bubalis) are an important animal resource that contributes milk, meat, leather, dairy products, and power for plowing and transport. However, mastitis, a bacterial disease affecting milk production and reproduction efficiency, is most prevalent in populations having intensive selection for higher milk yield, especially where the inbreeding level is also high. Climate change and poor hygiene management practices further complicate the issue. The management of this disease faces major challenges, like antibiotic resistance, maximum residue level, horizontal gene transfer, and limited success in resistance breeding. Bovine mastitis genome wide association studies have had limited success due to breed differences, sample sizes, and minor allele frequency, lowering the power to detect the diseases associated with SNPs. In this work, we focused on the application of targeted gene panels (TGPs) in screening for candidate gene association analysis, and how this approach overcomes the limitation of genome wide association studies. This work will facilitate the targeted sequencing of buffalo genomic regions with high depth coverage required to mine the extremely rare variants potentially associated with buffalo mastitis. Although the whole genome assembly of water buffalo is available, neither mastitis genes are predicted nor TGP in the form of web-genomic resources are available for future variant mining and association studies. Out of the 129 mastitis associated genes of cattle, 101 were completely mapped on the buffalo genome to make TGP. This further helped in identifying rare variants in water buffalo. Eighty-five genes were validated in the buffalo gene expression atlas, with the RNA-Seq data of 50 tissues. The functions of 97 genes were predicted, revealing 225 pathways. The mastitis proteins were used for protein-protein interaction network analysis to obtain additional cross-talking proteins. A total of 1,306 SNPs and 152 indels were identified from 101 genes. Water Buffalo-MSTdb was developed with 3-tier architecture to retrieve mastitis associated genes having genomic coordinates with chromosomal details for TGP sequencing for mining of minor alleles for further association studies. Lastly, a web-genomic resource was made available to mine variants of targeted gene panels in buffalo for mastitis resistance breeding in an endeavor to ensure improved productivity and the reproductive efficiency of water buffalo.
Introduction
Water buffalo (Bubalus bubalis) have been domesticated for more than 5,000 years, are also known as “Black Gold” due to their economic value. Its contribution to the Indian GDP in terms of milk, meat, horns, hides, leather, dairy products like cream, butter, yogurt, and cheese, power, plowing, and transporting people and crops have made buffalo an important animal resource in rural areas. India ranks first in world milk production due to the largest bovine population, where buffalo contributes 55%. The estimated world population of water buffalo is 208 million which is spread across over 77 countries in five continents with a major population (96%) in Asia (1). Water buffalo is an efficient converter of poor-quality forages into high quality milk and meat, making it a very valuable genetic resource for countries having “Low External-Input System” (2). Its efficiency of rumen fermentation (3) and nitrogen utilization (4) ability make it the animal of preference. However, intense selection for dairy productivity often results in increased levels of inbreeding, due to the limited number of bulls in the gene pool. Furthermore, climate change with a rising temperature humidity index (THI) has induced mastitis and many other diseases in major dairy animals including buffalo like retained placenta, metritis, ovarian cysts, claw diseases, milk fever, ketosis, and displaced abomasum (5–7).
Mastitis infections cause inflammation of the mammary gland and udder tissue, which is one of the most complex, costlier endemic diseases of dairy animals. It occurs as an immune response to invasion by a group of bacterial species in the teat canal. This may also occur due to chemical, mechanical, or thermal injury to the udder. This disease causes huge economic loss due to lesser milk production, premature culling, veterinary costs, and the risk of drug residues (8). Thus, there is a need to reduce mastitis to increase profitability and health (9).
Reduced milk production in buffalo mastitis costs US$8.80 per lactation (10). The direct loss happens due to a reduction in milk yield (70%), milk discard after treatment (9%), cost of veterinary services (7%), and premature culling (14%). There is also an indirect loss by adverse reproductive efficiency because of an imbalance in luteinizing hormone (LH) and estradiol that leads to failure of ovulation (11). In India, a total annual loss due to mastitis (clinical and subclinical) in buffalo has been estimated at US$526 million (10). Both clinical and subclinical mastitis is associated with mammary inflammation, a difference seen in clinical detectable and undetectable changes, respectively. With clinical mastitis, clinical visible signs are accompanied in the milk or mammary parenchyma. In subclinical mastitis conditions, a specific diagnostic test is performed (12).
Antibiotic treatment is effective in only 60% of cases of mastitis, which is due to an increase in b-lactamase producing organisms (10). The indiscriminate use of antibiotics has also led to a rise in resistant bacterial strains in Indian buffalo (13). All these are of global concern which is highly alarming due to the rise in AMR (antimicrobial resistance) and HGT (Horizontal Gene Transfer) of ARG (Antibiotic Resistance Genes) (14). The traditional breeding approach for the reduction of mastitis has not been effective due to its low heritability and its unfavorable genetic correlation with milk yield (15).
Recent studies on GWAS of mastitis disease in bovines have revealed that breed difference, sample size, and minor allele frequency, may lower the power to detect the disease-associated SNPs (16). Though universal NGS array based GWAS and genomic selection have been successful in animal breeding for better genetic gain of productivity traits, mastitis resistance breeding has still had limited success. GWAS has a major limitation in that it implicates the entire genome in disease predisposition where most association signals reflect variants/genes with no direct biological relevance to the disease (17). A control group of animals may contain unexposed individuals with susceptible genotypes. In such situations, a candidate gene approach tends to have greater statistical power than GWAS (18). GWAS can miss data on intron retention, which works in disease resistance, as reported in water buffalo (19). The success of the candidate gene approach depends on the correct choice of targeted gene panel (TGP) for SNP discovery, focusing on disease associated pathways in the investigation (20).
In genome assisted selection, the candidate gene approach using TGP and GWAS complement each other, rather than being contradictory. TGP can be used pragmatically in dissecting the genetics of complex disease by the mining of extremely low frequency alleles required in case-control association analysis. This ensures that minor novel alleles are not missed, which are not present in the prefabricated SNP array/chip (21, 22). TGP approach offers multifold advantages like sequencing at higher depth (500–1000X coverage) than the whole genome/exome sequencing, allowing the discovery of extremely rare variants which are potentially the most valuable alleles for association studies (23, 24). It is rapid and cost effective, especially in the case of association studies where the sample size is limited and mining of causative mutations in a single assay is imperative (25). Variant mining of TGP can be done either by direct amplicon sequencing (if TGP <50) or by target enrichment by magnetic beads followed by NGS data generation (26).
Post-GWAS, prioritization of candidate genes for mastitis resistance breeding in cattle has been reported (27). A computational approach has been reported to identify the candidate genes for disease association studies (28). A genomic resource database for cattle candidate genes and genetic markers for milk production and mastitis has also been developed (29). However, there is no targeted gene panel genomic resource of water buffalo for mastitis association studies. Recently available WGA with chromosome wise genomic data can be used for the development of genomic resources cataloging TGP. Since taurine and babuline genomes have extensively conserved milk and mastitis associated genes, comparative genomics approaches can quickly enrich buffalo genomic resource development.
The present work aims to develop a targeted gene panel of mastitis genes in water buffalo. It anticipates the prediction of mastitis associated candidate genes in the water buffalo genome using publically available bovine mastitis genes. It also aims to validate the RNA-Seq library in water buffalo, along with predicting biochemical pathways mediating this disease through the protein-protein network analysis. We present a web-genomic resource that is required for variant mining for future association studies of mastitis disease.
Materials and Methods
Mining and Mapping of Bovine Mastitis Associated Genes
An intensive literature search was carried out covering >40 literature across water buffalo (Bubalus bubalis) and cattle (Bos taurus) genes. Distinct terms associated with mastitis, namely, mastitis resistance, tolerance, and traits association were used as keywords for this search. These records were compiled and filtered to remove duplicates to finally fetch the genes associated with mastitis for further analyses in the present study.
Earlier studies on buffalo mastitis genes were reported even before the availability of the de novo genome assembly but then chromosomal number and position in the physical map were not known. By applying the NGS-based variant mining from TGP in a given buffalo population, the targeted genes or region of interest can be mapped. To accomplish this, the entire set of genes reported in Bubalus bubalis was downloaded from NCBI (30). Prior to further analyses, these were subjected to manual curation based on the organism specific term “Bubalus bubalis.” The finally filtered genes were used to map mastitis associated genes to obtain their genomic coordinates in the chromosome-wise genome assembly. In-house Perl scripts were used to map, based on gene symbol and its alias. Based on these parameters, mapping was accomplished for genes indicating features like gene symbol, gene ID, description, chromosome number, chromosome start and end location, strand orientation, and exon count.
Validation of Predicted TGP Genes in the Water Buffalo Gene Expression Atlas
Since genes in TGP panels are computationally predicted using cattle genome, their expressional reliability was validated in the RNA-Seq data of water buffalo available in the gene expression atlas of 50 different tissues (31). This validation was done using an in-house generated Perl script.
Functional Prediction of Mastitis Associated Candidate Genes
The function and pathway enrichment analysis of genes were performed using PANTHER (32) and DAVID v6.8 (Database for Annotation, Visualization, and Integrated Discovery) available at https://david.ncifcrf.gov/ (33, 34). It utilizes the Kyoto Encyclopedia of Genes and Genomes (KEGG) for pathway analysis and Gene Ontology (GO) analyses for functional prediction.
Annotation tool DAVID had Bubalus bubalis specific 91 genes. Since, in bovines, mastitis-associated genes are highly conserved, further analysis was done by taking advantage of this using cattle (Bos taurus) in PANTHER (http://pantherdb.org/data/). KEGG Mapper (Version 4.1), a mapping tool was used to reconstruct pathways (35). KEGG Orthology (KO) annotation was retrieved for key candidate genes and pathway reconstruction was performed specific to the Bubalus bubalis species.
Prediction of Cross-Talking Proteins by Protein-Protein Network Analysis
The list of genome-based candidate genes was further enriched by computational prediction of mastitis disease associated proteins (DAP) using protein-protein interaction (PPI) analysis. The amino acid sequences of mastitis associated genes were retrieved from NCBI, using advanced search options with the keywords “mastitis” and organism “Bubalus bubalis” (36). The cross talk of candidate gene proteins with additional predicted putative candidate gene proteins were predicted computationally by PPI analysis using the STRING database (Version 11.0; https://string-db.org/) (37). This database scores and integrates the various sources of PPI after collecting these from the public domain and complements the information with computational predictions. STRING database aims at a comprehensive and objective global network, considering both, physical as well as functional interactions. Proteins are represented as nodes and the interactions among them are pictured as edges in the network. Furthermore, Cytoscape (Version 3.7.1) (38) was used to visualize the PPI network. To get the most inter-connected nodes from the constructed PPI network complex, additional Cytoscape plugins (MCODE and CentiScape) were used. To predict the extended interaction among proteins, the retrieved number of proteins was increased up to 100. All these interactions were predicted specific to Bubalus bubalis species.
Evaluation of Mastitis TGP Based Variant Mining (SNP and Indel)
RNA-Seq data of mammary gland tissues (ERR315636) and Refseq of water buffalo (GCA_003121395.1) were downloaded from NCBI. The data was processed by FastQC (39) to check the quality. After the quality was checked low-quality reads and adapters were removed using the Trimmomatic tool V. 0.36 (40) by selecting the parameters of Phred quality score ≥20, Minimum reads length = 25 and Leading:4, Trailing:4 and Sliding-window:4:20. Filtered pair-end reads were aligned to the reference (genome assembly of water buffalo) using Burrows-Wheeler Aligner (bwa-0.7.17) tool (41, 42). The Sequence Alignment Map (SAM) file was converted to Binary Alignment Map (BAM) using SAMtools-1.9 (43) followed by sorting of BAM file. Variant calling analysis for analyzing genotype likelihoods was done with mpileup function with BCFtools 1.9 (44). SNPs were filtered at p < 0.05, read depth (d) ≥10, quality depth (Q) ≥30, MQ (minimum root mean square mapping quality) ≥40, and flanking sequence length 50. Genomic coordinates of SNPs and indels from the VCF file were mapped with coordinates of 101 genes using in-house generated shell scripting.
Development of Web Resource: Water Buffalo-MSTdb (WBMSTDb)
Water Buffalo Mastitis Database (WBMSTDb) is an online relational database that catalogs information of bovine mastitis associated genes, their information along with the functions and annotations, predicted genes and their functions by close and other interaction PPI networks and in-silico SNPs and indels. The web resource also houses various pathways where these genes are known to be involved. It has been developed using “three tier architecture,” namely, a client tier, middle tier, and database tier (Figure 1). Client-end assists to display the information related to services available and build the front-end layer of the application for end-users of their customized search related to mastitis in buffalo. The database layer has been developed using PHP (Hypertext Preprocessor) which is an open-source server-side scripting language. The application layer, also known as the middle tier or logic tier takes the information from the presentation tier and controls the application's core functionality. Database tier has the information related to mastitis associated genes, gene pathways, proteins, and PPI, functions, and variants, etc. cataloged in MySQL database using SQL query language. WBMSTDb has been developed using LAMP (Linux-Apache-MySQL-PHP) technology and launched at Apache server.
Results
Mining and Mapping of Bovine Mastitis Associated Genes
A total of 159 mastitis associated genes were sourced from literature, taking water buffalo (Bubalus bubalis) and cattle (Bos taurus) as reference. After removing the duplicates and alternative gene alias, 129 genes associated with mastitis were used for further study. Out of 129 unique genes associated with mastitis, 120 genes were retrieved from highly diverse cattle breeds like Canadian Holsteins, Holstein, Holstein Friesian, and Brown Swiss, Chinese Holstein, Sanhe cattle, Chinese Simmental, Luxi Yellow, and Bohai Black. The remaining 9 genes were reported both in cattle breeds mentioned and in buffalo breeds like Murrah and Egyptian buffalo.
A total number of 34,139 genes were retrieved from Bubalus bubalis from NCBI (30) for mapping. After manual curation, 34,067 genes (98.41%) were obtained, which were further used for mapping 129 mastitis associated genes. Of these, 101 genes were successfully mapped. Supplementary Table 1 shows details including the mapping location (chromosome coordinates), chromosome number, strand orientation, the total number of exons, gene type, organism based on the literature survey of these genes, whether the information was retrieved from cattle or buffalo studies along with references. Chromosome-wise mapping of all these 101 genes is depicted in Figure 2.
Validation of Predicted TGP Genes in Water Buffalo Gene Expression Atlas
The mastitis associated genes under study are revalidated by using recently published work of the gene expression atlas (30) of the domestic water buffalo (Bubalus bubalis) containing 23,492 genes expressed across 50 different tissues. After removing the duplicates, the remaining 23,489 genes were used for the revalidation of 101 key candidate genes. It was observed that 85 out of 101 key candidate genes were common in 23,489 genes (Figure 3), except for genes that include: selectin P (SELP), Amyloid beta precursor protein (APP), BTG anti-proliferation factor 1 (BTG1), lipopolysaccharide binding protein (LBP), CD46 molecule (CD46), lactotransferrin (LF), carboxypeptidase (CP), matrix metallopeptidase 9 (MMP9), BRCA1 DNA repair associated (BRCA1), RNA binding motif single stranded interacting protein 1 (RBMS1), ETS proto-oncogene 2, transcription factor (ETS2), transforming acidic coiled-coil containing protein 3 (TACC3), hepatocyte growth factor (HGF), serum amyloid A 3 (SAA3), orosomucoid 1 (ORM1), and cytochrome c oxidase subunit II (COX2). The common gene list is included in Supplementary Table 2.
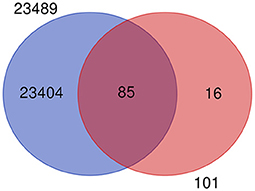
Figure 3. Venn diagram showing the common and unique genes when compared to gene expression atlas of water buffalo and genes from milk traits.
Functional Prediction of Mastitis Associated Candidate Genes
The roles of the mastitis associated genes in ontological terms were explored by their functional annotation. An organism specific classification system for the functionally annotated genes using PANTHER (32) was obtained for Bos taurus only as it does not have Bubalus bubalis. A genetically close bovine species, Bos taurus was used for this analysis to predict the biological role of the genes. Out of 101 genes, 98 were annotated successfully on molecular, biological, and cellular functions based on their role and classification to a PANTHER family. The predicted protein of mastitis associated genes and their pathways were also obtained. For example, gene enoyl-CoA hydratase and 3-hydroxyacyl CoA dehydrogenase (EHHADH) are classified to PANTHER family, peroxisomal bifunctional enzyme (Family ID: PTHR23309). There are a total of 121 genes in this particular family. The molecular function of oxidoreductase activity and biological process includes fatty acid catabolic process and oxidation-reduction process. The protein class annotated for gene EHHADH is dehydrogenase. Supplementary Table 3 includes details of all the 98 annotated genes in Bos taurus.
Gene annotation with species Bubalus bubalis using DAVID revealed annotation of 26 genes (Table 1). With respect to biological processes, genes such as toll like receptor 2 and 4 (TLR2 and TLR4), C-C motif chemokine ligand 3 (CCL3), complement C3 (C3), C-X-C motif chemokine ligand 8 (CXCL8), and CD14 molecule (CD14) were found to be involved in inflammatory response (44–47). These genes along with S100 calcium binding protein A8 (S100A8) and lipopolysaccharide binding protein (LBP) were involved in innate immune response (48, 49). Interleukin1 beta (IL1B) was involved in processes like fever generation, immune response, and positive regulation of cell division which provides evidence for the cause of symptoms in mastitis disease condition (50).
The molecular functions in which most of these genes are involved are related to binding and kinase activity. For instance, AKT serine/threonine kinase 3 (AKT3) and CCL3 are involved in kinase and ATP binding function (51, 52). TLR2 and TLR4 molecules are involved in transmembrane signaling receptor activity (53). Many genes are involved in calcium, metal, zinc, ion binding functions like lactoperoxidase (LPO), calmodulin 2 (CALM2), S100 calcium binding protein A8 (S100A8), in calcium-ion binding, lactotransferrin (LTF) in iron ion binding, and tumor protein p53 (TP53) in the metal-ion binding process (54–56).
Cellular processes include signal components, secreted molecules, glycoproteins, disulfide bond molecules, cytokines, and extracellular space. Maximum genes are involved in signaling processes, a list of genes is given in Table 1. Interleukin 6 and 12B (IL6 and IL12B) are involved in all the cellular processes as stated (57, 58). Protein family annotation for these 26 genes and information from protein databases such as Simple Modular Architecture Research Tool (SMART), Protein Information Resource (PIR), and InterPro is included with protein family ID and functions associated in WBMSTDb (59–61). The details are available at http://webtom.cabgrid.res.in/wbmstdb/index.php under the tab: “Search—Mastitis Gene Panel—Gene Annotation.”
The pathway analyses of these genes as identified through KEGG Mapper revealed that out of the 101 mastitis associated genes under study, there were six genes for which KEGG ontology was not assigned. These are, mannose-binding protein A (MBLA), actin beta (ACTB), FEZ family zinc finger 2 (FEZF2), osteoclast stimulating factor 1 (OSTF1), RNA binding motif single stranded interacting protein 1 (RBMS1), and RELA proto-oncogene, NF-kB subunit (RELA).
A total of 225 pathways in various categories were classified, namely, metabolism, genetic and environmental information processing, cellular process, organismal systems such as immune system, and human diseases. The pathways revealed the involvement of these genes in diverse roles. The majority of these genes were involved in infection or disease susceptibility by microbial organisms like Salmonella, Shigellosis, Pertussis, Legionellosis, Staphylococcus aureus, Yersinia, etc. (Table 2).
Nine genes were found to be involved in apoptosis activity like BCL2 associated X, apoptosis regulator (BAX), BCL2 related protein A1 (BCL2A1), MCL1 apoptosis regulator, BCL2 family member (MCL1), Fos proto-oncogene, AP-1 transcription factor subunit (FOS), growth arrest and DNA damage inducible beta (GADD45B), tumor protein p53 (TP53), AKT serine/threonine kinase 3 (AKT3), baculoviral IAP repeat containing 3 (BIRC3), and mitogen-activated protein kinase kinase 7 (MAP2K7). Table 2 describes the mastitis associated genes involved in various infectious pathways.
Prediction of Cross-Talking Proteins by Protein-Protein Network Analysis
The panels of 111 proteins were used for PPI network analysis. Among these, 10 proteins were buffalo specific, which were obtained directly from NCBI. The remaining 101 proteins were retrieved from the STRING database using their genetic codes. The construction of the PPI network revealed 83 nodes and 227 edges. These predicted proteins were further searched for other cross talking proteins in Bubalus bubalis. This resulted in a final PPI network of 193 nodes and 1,480 edges (Table 3). PPI predicted 1,707 proteins associated with mastitis disease in water buffalo. Based on the degree, between-ness, and closeness of the network, they were classified into two groups, viz., (1) directly interacting 83 proteins with cross-talk with 227 proteins as first-degree interaction (2) other predicted proteins (193) which are having cross-talks with 1,480 predicted proteins as second-degree interaction in the network.
Visualization of a network with highly connected nodes using Cytoscape detected the hub proteins (Figure 4). Hub molecule analysis revealed six protein nodes (>40 edges) that are highly connected, namely, TP53 (number of edges: 56), Signal transducer and activator of transcription 3 (STAT3) (number of edges: 49), IL1B (number of edges: 46), Jun oncogene (JUN) (number of edges: 45), AKT3 (number of edges: 41), and acetyl-CoA acyltransferase 1 (ACAA1) (number of edges: 40). Out of these, TP53, STAT3, IL1B, and AKT3 were found to be the first interacting partners, i.e., key candidate genes involved in mastitis, while JUN and ACAA1 were the other interacting partners. Furthermore, using Cytoscape plugins, CentiScape, and MCODE, the highest PPI scores by three centrality methods were computed. These scores along with other parameters of these genes are shown in Table 4. A total of nine clusters identified from the PPI network were highly connected subnetworks. A cluster of highly connected proteins' sub-network revealed 22 nodes and 211 edges with a MCODE score value of 20 (Figure 5).
Evaluation of Mastitis TGP Based Variant Mining (SNPs and Indels)
The overall read mapping rate and concordant pair alignment rate of RNA-Seq data on the reference assembly was 94.4 and 88.9%, respectively. On mapping the genomic coordinates of SNPs and indels from the VCF file of mammary tissues transcriptome over coordinates of 101 genes using in-house Perl scripts, a total of 1,458 SNPs and indels were obtained. Out of these, 1,306 were SNPs and 152 were indels. The highest number of SNPs and indels (261) was observed for the gene, BCL2 apoptosis regulator located on chromosome number 22 which is involved in apoptosis regulating activity. Gene CD46 was mapped for 116 SNPs and indels. For the genes MAF bZIP transcription factor F (MAFF), APP, signal sequence receptor subunit 1 (SSR1), and CP: 42, 37, 36, and 36 SNPs and indels were mapped. Similarly, for 11 genes, namely, uncoupling protein 3 (UCP3), DNA nucleotidylexotransferase (DNTT), C-C motif chemokine ligand 20 (CCL20), FEZ family zinc finger 2 (FEZF2), colony stimulating factor 2 (CSF2), CCL3, interleukin 6 (IL6), Acyl CoA thioesterase 1 (ACOT1), SAA3, MBLA, and Orosomucoid 1 (AGP), there was no SNP and indel mapping. Supplementary Table 4 has the details of the SNP and indels mapped for the predicted mastitis genes.
Development of Web Resource: Water Buffalo-MSTdb (WBMSTDb)
Water Buffalo Mastitis Database (WBMSTDb) is an open source and user-friendly web resource of targeted gene panels, which can be used for academic purposes in future mastitis association studies (website: http://webtom.cabgrid.res.in/wbmstdb/). WBMSTDb has five different tabs, namely, Home, Search, Pathways, Tutorial, and Team. Home tab deals with the brief introduction of this web resource along with various datasets related to mastitis cataloged in it. The objectives, developmental procedures, and rationale of its development are well-documented here. The Search tab is categorized into three sub-tabs, i.e., Mastitis Gene Panel, Mastitis Disease Associated Proteins, and Mastitis Disease Associated Predicted Proteins. Furthermore, the sub-tab Mastitis Gene Panel is categorized into two sub-sub-tabs, namely, “Gene Information” and “Gene Annotation.” The “Gene Information” sub-sub-tab has the information related to Gene symbol, Gene ID, Gene Description, Chromosome Number, Gene Start Position, Gene End position, Orientation, and Exon Counts. Provision has been made for the browser for simpler visualization. As users may have targeted regions within the gene for SNP mining, like UTR region or exonic regions only, so to cater to such needs, provision has been made for the convenient visualization of regions of each ORF by integrating the TGP with the genome browser. The “Gene Annotation Information” sub-sub tab has information related to Gene ID, Gene name, Species, GO term BP (Biological processes), GO term CC (Cellular components), GO term MF (Molecular Functions), Interpro database, the PIR superfamily database, SMART database, and Up keywords. The “Mastitis Disease Associated Proteins” sub-tab provides information on Protein names, Length, and accession Numbers. The “Mastitis Disease Associated Predicted Proteins” sub-tab provides two types of interaction images, i.e., “Major Interaction” and “Distant Interaction.” The information of PPI provides Node1, Node2, Neighborhood chromosome, Gene fusion, Phylogenetic co-occurrence, Homology, Co-expression, Experimentally determined interaction, Database annotation, and Auto-text mining. The “Function Annotation” provides ProteinID, Description, Gene-observed, Gene-background, False-discovery rate, Matched proteinID, Database. The “Pathways” tab deals with the information like Pathways name, Pathways Id, Numbers of Enzyme, Enzyme, Sequence of Enzyme count, Sequence Id, and Pathway Figures. Users can download the targeted gene panel for very high coverage (600–1000X) sequencing to mine for extremely low frequency alleles potentially associated with mastitis disease. The Tutorials tab contains the guidelines for users and terminology used in the database contents. Finally, the Team tab has the contact list of researchers involved in the analyses and development of the web resource, WBMSTDb. The various interfaces for users are shown systematically in Figure 6.
Discussion
Mining and Mapping of Bovine Mastitis Associated Genes
In a panel of successfully identified 129 mastitis associated genes, 110 and 19 genes were from cattle and buffalo populations, respectively. This availability of a higher number of mastitis genes of cattle in GenBank is expected as cattle are a much more widely distributed and well-studied bovine species than water buffalo. The successful mapping of mastitis associated genes (101) of heterologous species cattle over the water buffalo genome reveals their extensive conservation during the divergence of these bovine species. This high conservation agrees with earlier mapping studies of water buffalo depicting syntenic block coverage of >80% (62). Many mastitis associated genes like complement C5 (C5) and chemokine (C-X-C motif) receptor 1 (CXCR1), are reported to be conserved as high as up to 99% sequence similarity (63, 64).
This extensive conservation of cattle genes in water buffalo is due to their common ancestor, homologous proteins, common gene family, common CNV, common gene statistics, across species selection signature of milk pathways are also revealed in buffalo genome Benchmarking Universal Single-Copy Orthologs (BUSCO) mapping studies (62). The remaining 28 cattle genes, which could not be mapped over the buffalo genome, might be due to their acquired species specificity in cattle during species divergence (Supplementary Table 5). This structural genomic difference of river buffalo and cattle has also been reported in comparative genomic and transcriptomic studies (65).
The key candidate genes are mapped to the chromosome location and position using the entire set of buffalo genes from NCBI. Maximum key genes of mastitis were mapped to chromosome 3 as also reported in water buffalo previous studies (66, 67). Interestingly, many alleles of mastitis genes of chromosome 3 are also associated with fertility traits. Mastitis also affects fertility, as this disease may lead to early embryonic death, infertility, or sterility in water buffaloes (68).
Validation of Predicted TGP Genes in Water Buffalo Gene Expression Atlas
The successful mapping of 85% TGP genes over water buffalo species specific gene expression atlas demonstrates the reliability of predicted genes as they get transcribed in buffalo tissues. The remaining non-validated (15%) TGP genes might be due to spatiotemporal gene expression data in the atlas. In an atlas, all genes are not expected to express all the time. This will vary with the tissue as well as in the timeline for the sampling of transcriptome data generation. This spatiotemporal gene expression in the milk tissue of bovines has been reported (69).
Functional Prediction of Mastitis Associated Candidate Genes
Mastitis genes regulating the proteins may have a significant functional role in the modulation and regulation of mastitis disease. Proteins like complement C4 (C4), BRCA1, TLR4, MBLA, and calcium voltage-gated channel auxiliary subunit alpha2delta 1 (CACNA2D1) are reported potential biomarkers in mastitis (70) which were also found in our study. The functional prediction of the key genes associated with mastitis disease was found to be involved in the immune and inflammatory response. For instance, genes like CXCL8, CD14, S100A8, CSF2, C3, Interferon regulatory factor 1 (IRF1), IL1B, IL6, LPO, LBP, TLR2, and TLR4 are well-known immune and inflammatory responsive genes (71). Mastitis is a complex disease causing inflammation of the mammary gland where origin, severity, and outcome of the disease depend on the environment, pathogen virulence, and host immunity (9). Mastitis is characterized by physical, chemical, and biological changes in milk quality like the presence of blood, water, pus, clots, flakes, and shreds consisting of fibrin as well as cellular debris, udder enlargement, somatic cell count, and asymmetry of the gland (72). In our predictive functional annotation analysis, the key candidate genes were found to mediate such responses including mastitis fever generation (73).
Prediction of Cross-Talking Proteins by Protein-Protein Network Analysis
A protein-protein interaction study has revealed the various cross talking proteins of mastitis genes in water buffalo that can be used to understand the disease and associated proteins (74). Mastitis associated predicted proteins can be classified using the degree, between-ness, and closeness of the network (75). The top hub molecules of the interaction network predicted were TP53, STAT3, IL1B, and AKT3. The functional characterization of these proteins provides evidence of their role in signaling and the symptoms caused by mastitis. For example, AKT3 expression increases during lactation in the mammary gland and immune cells, is also involved in the TLR pathways regulating as proinflammatory cytokines (51). The role of IL1B as an inflammatory cytokine in mastitis disease resistance has been reported in water buffalo (76). TP53 gene has been reported as a key candidate gene that drives mastitis resistance genes (77). Furthermore, IL1B is reported as a hub molecule in cattle mastitis (78). The Serotransferrin (TF) gene is reported as a major regulator of a set of genes that are responsible for the resistance/susceptibility of mastitis (79). Enhanced semaphorin 5A (SEMA5A) gene expression has been reported to induce at least nine genes related to the host's immune response including tumor necrosis factor alpha (TNF-a) and interleukin 8 (IL-8) (80). Since the heritability of adaptive immune traits ranges from 0.25 to 0.35 in dairy cattle, thus the genes associated with it are valuable in mastitis resistance breeding (81). The high immune responder approach has been utilized to assess the dairy cattle's ability to trigger antibody and cell-mediated immune response against pathogens. The cows with superior adaptive immune response have been reported to show a lower occurrence of mastitis and metritis (82). How these predicted proteins have cross talk with mastitis associated proteins needs to be studied further to understand the genetic mechanism of this disease by a bottom up approach, predicting additional genes from these putative proteins. The genes of enlisted DAP can be used for future SNP discovery as well as for designing drugs (83).
Evaluation of Mastitis TGP Based Variant Mining (SNPs and Indels)
Variant mining for SNP (1,306) and indel (152) were found to be limited in number with respect to the total genes targeted (101). Since the genic region confines to <3% of the total genome, transcriptome data is expected to have less SNPs for WGS based SNP mining. In the case of water buffalo, the average exon length is 181.74 bp with respect to the average intron length of 3,435 bp, which also indicates a lower abundance of SNPs (62) in the genic region. This lower number of SNPs in genic region was also reported in water buffalo targeted sequencing in the Jafarabadi breed (84). In another study of the Murrah water buffalo breed, genic region SNPs (79.40%) were found in nearly one-fifth of non-genic region SNPs (19.26%) in terms of their relative abundance (85). Though exonic variations are very limited in number, they do play an important role in population differentiation at the genetic level (86). Non-synonymous SNP polymorphism in the genic region of mastitis genes is very valuable for mastitis disease resistance breeding in bovines (87).
Such genic region SNPs with known physical map locations have been used in dissecting mastitis resistance QTL in dairy cattle (15). The use of indel as a marker has the advantage of making genotyping and multiplexing easier (88). Such indel polymorphism in milk genes and its trait association studies are reported in goats (89). A higher number of variants, i.e., 261 and 116, were found in gene BCL2 (Gene ID 281020) and CD46 (Gene 280851), respectively. A longer gene is expected to have a higher number of variants which is also evident by the higher number of exons (BCL2 with 7 and CD46 with 17 exons). BCL2 is also known to have copy number variants (90) and isforms (91), which might have contributed to the increased number of SNPs. The SNP of the BCL2 gene has been found to be associated with heat stress in water buffalo (92). Similarly, the SNP of gene CD46 was also found to be associated with mastitis in bovines (93). SNP studies of TGP genes can pave the knowledge discovery of pathway genes mediating mastitis disease by integrative analysis with transcriptomic data (24, 94).
Development of Web Resource: Water Buffalo-MSTdb (WBMSTDb)
The Water Buffalo-MSTdb (WBMSTDb) is the first targeted gene panel genomic resource of water buffalo for mastitis association studies. It is freely accessible to the global community. This web genomic resource can be used for the marker discovery required in mastitis resistance breeding of water buffalo. Users can download the complete ORF of the genes to be targeted for mining the variants. A genome browser can assist in the depiction of various parts of an ORF of the gene. Since TGP contains the entire open reading frame having 5′UTR, exons, introns, and 3′UTR with genomic coordinates, it can be used for SNP, simple-sequence repeat (SSR), and indel marker discovery, which are required in association studies for mastitis resistance breeding. Such marker discovery assay can be designed using NGS technology with the sequencing of very high depth coverage (500–1000X), by both target enrichment method (>50 gene target genes) using magnetic beads or direct amplicon sequencing (if the number of genes are <50) in a single rapid cost-effective assay (25).
Many mastitis candidate gene association studies have been successfully reported for cattle. For example, in case of mannan binding lectin serine peptidase 2 (MASP2) (95), TLR4 (96), ATPase Na+/K+ transporting subunit alpha 1 (ATP1A1) (97), IL-8 (98), mitogen-activated protein kinase kinase kinase kinase 4 (MAP4K4) (99), lipocalin-2 (LNC2) (100), TLR2 (101), CD14 (102), TLR4 (103), Exon3 TL-4 (104), CD46 (93), phosphodiesterase 9A (PDE9A) (105), and insulin-like growth factor 1 (IGF-1) (106) have been reported. A similar approach is required in water buffalo for mastitis resistance breeding. Target gene panel approach with very limited sample size and limited gene number with case-control association study model has been successful in the diagnosis of susceptible individuals. A target gene panel covering both coding and non-coding region having SSR has been successfully used for improved diagnostic of disease (107).
Conclusion
The mapping of cattle mastitis associated genes in water buffalo genome assembly has revealed their extensive conservation. Mapping can be used advantageously in making targeted gene panels (TGP), which are required for low frequency variant mining in the water buffalo population. Such low frequency alleles are very valuable in disease association analysis and often get missed in GWAS. The limitations of GWAS studies in mastitis disease resistance breeding could be overcome by supplementing candidate gene association analysis approaches using our developed TGP in WBMSTDb. Protein-protein interaction has predicted disease associated protein and revealed the biochemical pathway mediating that takes place as a result of mastitis disease. These proteins can be used for future drug design. The predicted TGP genes were successfully validated in a transcriptome of various buffalo tissues. Successful variant mining was also done using the transcriptome data of the buffalo mammary gland. This TGP can be used in buffalo breeding programs for selection and culling and better milk and reproductive efficiency.
Data Availability Statement
All datasets generated for this study are included in the web resource: http://webtom.cabgrid.res.in/wbmstdb/.
Author Contributions
DK conceived the theme of the study. SJ, JJ, MI, JK, AG, and UA performed the computational analysis and developed genomic resources. SJ, JJ, MI, and DK drafted the manuscript. VN, SK, RK, TD, AR, and DK edited the manuscript. All co-authors read and approved the final manuscript.
Conflict of Interest
The authors declare that the research was conducted in the absence of any commercial or financial relationships that could be construed as a potential conflict of interest.
Acknowledgments
Authors are thankful to the Indian Council of Agricultural Research, Ministry of Agriculture and Farmers' Welfare, Government of India for providing financial assistance in the form of a CABin grant as well as the use of the Advanced Super Computing Hub for Omics Knowledge in Agriculture (ASHOKA) facility at ICAR-IASRI, New Delhi, India created under National Agricultural Innovation Project, and funded by the World Bank. All the co-authors wish to dedicate this manuscript and work to the COVID-19 warriors of India and the globe. We are extremely thankful to them because they make it possible to work safely and remotely. We also thank Dr. S. C. Gupta for critical suggestions and help, and Dr. Neelam Gupta, Animal Science Division, ICAR Head Quarter, New Delhi.
Supplementary Material
The Supplementary Material for this article can be found online at: https://www.frontiersin.org/articles/10.3389/fvets.2021.593871/full#supplementary-material
References
1. Minervino AH, Zava M, Vecchio D, Borghese A. Bubalus bubalis: a short story. Front Vet Sci. (2020) 7:971. doi: 10.3389/fvets.2020.570413
2. FAO. Successes and failures with animal nutrition practices and technologies in developing countries. In: Proceedings of the FAO Electronic Conference. Rome (2011). p. 1–30.
3. Wanapat M, Ngarmsang A, Korkhuntot S, Nontaso N, Wachirapakorn C, Beakes G, et al. A comparative study on the rumen microbial population of cattle and swamp buffalo raised under traditional village conditions in the northeast of Thailand. Asian Austral J Anim Sci. (2000) 13:918–21. doi: 10.5713/ajas.2000.918
4. Devendra C. Perspectives on animal production systems in Asia. Livest Sci. (2007) 106:1–8. doi: 10.1016/j.livsci.2006.05.005
5. Fleischer P, Metzner M, Beyerbach M, Hoedemaker M, Klee W. The relationship between milk yield and the incidence of some diseases in dairy cows. J Dairy Sci. (2001) 84:2025–35. doi: 10.3168/jds.S0022-0302(01)74646-2
6. Funk DA. Major advances in globalization and consolidation of the artificial insemination industry. J Dairy Sci. (2006) 89:1362–8. doi: 10.3168/jds.S0022-0302(06)72203-2
7. Rojas-Downing MM, Nejadhashemi AP, Harrigan T, Woznicki SA. Climate change and livestock: impacts, adaptation, and mitigation. Clim Risk Manag. (2017) 16:145–63. doi: 10.1016/j.crm.2017.02.001
8. Bhosale RR, Osmani RA, Ghodake PP, Shaikh SM, Chavan SR. Mastitis: an intensive crisis in veterinary science. Int J Pharma Res Health Sci. (2014) 2:96–103. doi: 10.30750/ijpbr.2.1.19
9. De Vliegher S, Fox LK, Piepers S, McDougall S, Barkema HW. Invited review: mastitis in dairy heifers: nature of the disease, potential impact, prevention, and control. J Dairy Sci. (2012) 95:1025–40. doi: 10.3168/jds.2010-4074
10. Varshney JP, Naresh R. Evaluation of a homeopathic complex in the clinical management of udder diseases of riverine buffaloes. Homeopathy. (2004) 93:17–20. doi: 10.1016/j.homp.2003.11.007
11. Kadarmideen HN, Thompson R, Simm G. Linear and threshold model genetic parameters for disease, fertility and milk production in dairy cattle. Anim Sci. (2000) 71:411–9. doi: 10.1017/S1357729800055338
12. Fragkou IA, Boscos CM, Fthenakis GC. Diagnosis of clinical or subclinical mastitis in ewes. Small Rumin Res. (2014) 118:86–92. doi: 10.1016/j.smallrumres.2013.12.015
13. Kaur M, Verma R, Bansal BK, Mukhopadhyay CS, Arora JS. Status of sub-clinical mastitis and associated risk factors in Indian water buffalo in Doaba region of Punjab, India. Indian J Dairy Sci. (2015) 8:483–7. Available online at: https://www.researchgate.net/profile/C-S-Mukhopadhyay/publication/283119182_Status_of_sub-clinical_mastitis_and_associated_risk_factors_in_Indian_water_buffalo_in_Doaba_region_of_Punjab_India/links/562b51f008ae22b17033153e/Status-of-sub-clinical-mastitis-andassociated-risk-factors-in-Indian-water-buffalo-in-Doaba-region-of-Punjab-India.pdf
14. Lerminiaux NA, Cameron AD. Horizontal transfer of antibiotic resistance genes in clinical environments. Can J Microbiol. (2019) 65:34–44. doi: 10.1139/cjm-2018-0275
15. Kadri NK, Guldbrandtsen B, Lund MS, Sahana G. Genetic dissection of milk yield traits and mastitis resistance quantitative trait loci on chromosome 20 in dairy cattle. J Dairy Sci. (2015) 98:9015–25. doi: 10.3168/jds.2015-9599
16. Fang L, Sahana G, Su G, Yu Y, Zhang S, Lund MS, et al. Integrating sequence-based GWAS and RNA-Seq provides novel insights into the genetic basis of mastitis and milk production in dairy cattle. Sci Rep. (2017) 7:1–6. doi: 10.1038/srep45560
17. Tam V, Patel N, Turcotte M, Bossé Y, Paré G, Meyre D. Benefits and limitations of genome-wide association studies. Nat Rev Genet. (2019) 20:467–84. doi: 10.1038/s41576-019-0127-1
18. Amos W, Driscoll E, Hoffman JI. Candidate genes versus genome-wide associations: which are better for detecting genetic susceptibility to infectious disease? Proc R Soc Lond B Biol Sci. (2011) 278:1183–8. doi: 10.1098/rspb.2010.1920
19. Abou Mossallam AA, El Nahas SM, Balabel EA, El Hassan TS, Osman NM. Intron retention in Cathelicidin-4 in river buffalo. Bull Natl Res Cent. (2019) 43:1–8. doi: 10.1186/s42269-019-0158-y
20. Wilkening S, Chen B, Bermejo JL, Canzian F. Is there still a need for candidate gene approaches in the era of genome-wide association studies? Genom. (2009) 93:415–9. doi: 10.1016/j.ygeno.2008.12.011
21. Border R, Keller MC. Commentary: fundamental problems with candidate gene-by-environment interaction studies–reflections on Moore and Thoemmes (2016). J Child Psychol Psychiatry. (2017) 58:328–30. doi: 10.1111/jcpp.12669
22. Moore SR. Commentary: what is the case for candidate gene approaches in the era of high-throughput genomics? A response to Border and Keller (2017). J Child Psychol Psychiatry. (2017) 58:331–4. doi: 10.1111/jcpp.12697
23. Ciani M, Benussi L, Bonvicini C, Ghidoni R. Genome wide association study and next generation sequencing: a glimmer of light toward new possible horizons in frontotemporal dementia research. Front Neurosci. (2019) 13:506. doi: 10.3389/fnins.2019.00506
24. Miles AM, Huson HJ. Graduate student literature review: understanding the genetic mechanisms underlying mastitis. J Dairy Sci. (2020) 104:1183–91. doi: 10.3168/jds.2020-18297
25. Reid ES, Papandreou A, Drury S, Boustred C, Yue WW, Wedatilake Y, et al. Advantages and pitfalls of an extended gene panel for investigating complex neurometabolic phenotypes. Brain. (2016) 139:2844–54. doi: 10.1093/brain/aww221
26. Illumina. Targeted Gene Sequencing. (2021). Available online at: https://sapac.illumina.com/techniques/sequencing/dna-sequencing/targeted-resequencing/targeted-panels.html
27. Cai Z, Guldbrandtsen B, Lund MS, Sahana G. Prioritizing candidate genes post-GWAS using multiple sources of data for mastitis resistance in dairy cattle. BMC Genom. (2018) 19:1. doi: 10.1186/s12864-018-5050-x
28. Patnala R, Clements J, Batra J. Candidate gene association studies: a comprehensive guide to useful in silico tools. BMC Genet. (2013) 14:1. doi: 10.1186/1471-2156-14-39
29. Ogorevc J, Kunej T, Razpet A, Dovc P. Database of cattle candidate genes and genetic markers for milk production and mastitis. Anim Genet. (2009) 40:832–51. doi: 10.1111/j.1365-2052.2009.01921.x
30. National Center for Biotechnology Information. Gene and Bubalus bubalis. (2020). Available online at: https://www.ncbi.nlm.nih.gov/gene/?term=bubalus+bubalis
31. Young R, Lefevre L, Bush SJ, Joshi A, Singh SH, Jadhav SK, et al. A gene expression atlas of the domestic water buffalo (Bubalus bubalis). Front Genet. (2019) 10:668. doi: 10.3389/fgene.2019.00668
32. Mi H, Muruganujan A, Ebert D, Huang X, Thomas PD. PANTHER version 14: more genomes, a new PANTHER GO-slim and improvements in enrichment analysis tools. Nucl Acids Res. (2019) 47:D419–26. doi: 10.1093/nar/gky1038
33. Jiao X, Sherman BT, Huang DW, Stephens R, Baseler MW, Lane HC, et al. DAVID-WS: a stateful web service to facilitate gene/protein list analysis. Bioinformatics. (2012) 28:1805–6. doi: 10.1093/bioinformatics/bts251
34. Huang J, Wang H, Wang C, Li J, Li Q, Hou M, et al. Single nucleotide polymorphisms, haplotypes and combined genotypes of lactoferrin gene and their associations with mastitis in Chinese Holstein cattle. Mol Bio Rep. (2010) 37:477–83. doi: 10.1007/s11033-009-9669-1
35. Kanehisa M, Sato Y. KEGG Mapper for inferring cellular functions from protein sequences. Protein Sci. (2020) 29:28–35. doi: 10.1002/pro.3711
36. National Center for Biotechnology Information. Mastitis and Bubalus bubalis. (2020). Available online at: https://www.ncbi.nlm.nih.gov/protein/?term=(mastitis%5BText+Word%5D)+AND+bubalus+bubalis%5BOrganism%5D
37. Szklarczyk D, Gable AL, Lyon D, Junge A, Wyder S, Huerta-Cepas J, et al. STRING v11: protein–protein association networks with increased coverage, supporting functional discovery in genome-wide experimental datasets. Nucl Acids Res. (2019) 47:D607–13. doi: 10.1093/nar/gky1131
38. Smoot ME, Ono K, Ruscheinski J, Wang PL, Ideker T. Cytoscape 2.8: new features for data integration and network visualization. Bioinformatics. (2011) 27:431–2. doi: 10.1093/bioinformatics/btq675
39. Babraham Bioinformatics. FastQC. Available online at: https://www.bioinformatics.babraham.ac.uk/projects/fastqc/
40. Bolger AM, Lohse M, Usadel B. Trimmomatic: a flexible trimmer for Illumina sequence data. Bioinformatics. (2014) 30:2114–20. doi: 10.1093/bioinformatics/btu170
41. Li H, Durbin R. Fast and accurate short read alignment with Burrows–Wheeler transform. Bioinformatics. (2009) 25:1754–60. doi: 10.1093/bioinformatics/btp324
42. Abuín JM, Pichel JC, Pena TF, Amigo J. BigBWA: approaching the Burrows–Wheeler aligner to Big Data technologies. Bioinformatics. (2015) 31:4003–5. doi: 10.1093/bioinformatics/btv506
43. Li H, Handsaker B, Wysoker A, Fennell T, Ruan J, Homer N, et al. The sequence alignment/map format and SAMtools. Bioinformatics. (2009) 25:2078–9. doi: 10.1093/bioinformatics/btp352
44. Li H. A statistical framework for SNP calling, mutation discovery, association mapping and population genetical parameter estimation from sequencing data. Bioinformatics. (2011) 27:2987–93. doi: 10.1093/bioinformatics/btr509
45. Boehmer JL, Bannerman DD, Shefcheck K, Ward JL. Proteomic analysis of differentially expressed proteins in bovine milk during experimentally induced Escherichia coli mastitis. J Dairy Sci. (2008) 91:4206–18. doi: 10.3168/jds.2008-1297
46. Goldammer T, Zerbe H, Molenaar A, Schuberth HJ, Brunner RM, Kata SR, et al. Mastitis increases mammary mRNA abundance of β-defensin 5, toll-like-receptor 2 (TLR2), and TLR4 but not TLR9 in cattle. Clin Diagn Lab Immunol. (2004) 11:174–85. doi: 10.1128/CDLI.11.1.174-185.2004
47. Islam M, Takagi M, Fukuyama K, Komatsu R, Albarracin L, Nochi T, et al. Transcriptome analysis of the inflammatory responses of bovine mammary epithelial cells: exploring immunomodulatory target genes for bovine mastitis. Pathogens. (2020) 9:200. doi: 10.3390/pathogens9030200
48. Zhao XW, Yang YX, Huang DW, Cheng GL, Zhao HL. Comparative proteomic analysis of proteins expression changes in the mammary tissue of cows infected with Escherichia coli mastitis. J Vet Sci. (2015) 16:253. doi: 10.4142/jvs.2015.16.3.253
49. Zeng R, Bequette BJ, Vinyard BT, Bannerman DD. Determination of milk and blood concentrations of lipopolysaccharide-binding protein in cows with naturally acquired subclinical and clinical mastitis. J Dairy Sci. (2009) 92:980–9. doi: 10.3168/jds.2008-1636
50. Sbuster DE, Kehrli ME, Stevens MG. Cytokine production during endotoxin-induced mastitis in lactating dairy cows. Am J Vet Res. (1993) 54:80.
51. Ullah F, Bhattarai D, Cheng Z, Liang X, Deng T, Rehman ZU, et al. Comparative analysis of V-AKT murine thymoma viral oncogene homolog 3 (AKT3) gene between cow and buffalo reveals substantial differences for mastitis. Biomed Res Int. (2018). doi: 10.1155/2018/1463732
52. Viale E, Tiezzi F, Maretto F, De Marchi M, Penasa M, Cassandro M. Association of candidate gene polymorphisms with milk technological traits, yield, composition, and somatic cell score in Italian Holstein-Friesian sires. J Dairy Sci. (2017) 100:7271–81. doi: 10.3168/jds.2017-12666
53. De Schepper S, De Ketelaere A, Bannerman DD, Paape MJ, Peelman L, Burvenich C. The toll-like receptor-4 (TLR-4) pathway and its possible role in the pathogenesis of Escherichia coli mastitis in dairy cattle. Vet Res. (2008) 39:1–23. doi: 10.1051/vetres:2007044
54. Cameron CM, Rillema JA. Extracellular calcium ion concentration required for prolactin to express its actions on casein, ribonucleic acid, and lipid biosynthesis in mouse mammary gland explants. Endocrinology. (1983) 113:1596–600. doi: 10.1210/endo-113-5-1596
55. Kawai K, Hagiwara S, Anri A, Nagahata H. Lactoferrin concentration in milk of bovine clinical mastitis. Vet Res Commun. (1999) 23:391–8. doi: 10.1023/A:1006347423426
56. Kussendrager KD, Van Hooijdonk AC. Lactoperoxidase: physico-chemical properties, occurrence, mechanism of action and applications. Br J Nutr. (2000) 84:19–25. doi: 10.1017/S0007114500002208
57. Ibrahim HM, El-Seedy YY, Gomaa NA. Cytokine response and oxidative stress status in dairy cows with acute clinical mastitis. J Dairy Vet Anim Res. (2016) 3:1–6. doi: 10.15406/jdvar.2016.03.00064
58. Song X, Zhang W, Wang T, Jiang H, Zhang Z, Fu Y, et al. Geniposide plays an anti-inflammatory role via regulating TLR4 and downstream signaling pathways in lipopolysaccharide-induced mastitis in mice. Inflammation. (2014) 37:1588–98. doi: 10.1007/s10753-014-9885-2
59. Blum M, Chang HY, Chuguransky S, Grego T, Kandasaamy S, Mitchell A, et al. The InterPro protein families and domains database: 20 years on. Nucl Acids Res. (2021) 49:D344–54. doi: 10.1093/nar/gkaa977
60. Letunic I, Khedkar S, Bork P. SMART: recent updates, new developments and status in 2020. Nucl Acids Res. (2021) 49:D458–60. doi: 10.1093/nar/gkaa937
61. Natale DA, Arighi CN, Blake JA, Bona J, Chen C, Chen SC, et al. Protein Ontology (PRO): enhancing and scaling up the representation of protein entities. Nucl Acids Res. (2017) 45:D339–46. doi: 10.1093/nar/gkw1075
62. Mintoo AA, Zhang H, Chen C, Moniruzzaman M, Deng T, Anam M, et al. Draft genome of the river water buffalo. Ecol Evol. (2019) 9:3378–88. doi: 10.1002/ece3.4965
63. El-Halawany N, Abd-El-Monsif AS, Al-Tohamy AF, Abdel-Latif MA, Abdel-Shafy H, Ghazi YA, et al. Effect of complement component 5 polymorphisms on mastitis resistance in Egyptian buffalo and cattle. Res Vet Sci. (2018) 119:45–51. doi: 10.1016/j.rvsc.2018.05.015
64. El Nahas SM, Abou Mossallem AA, Abdelhamid MI, Warda M. A study on IL8RB gene polymorphism as a potential immuno-compromised adherent in exaggeration of parenteral and mammo-crine oxidative stress during mastitis in buffalo. J Adv Res. (2017) 8:617–25. doi: 10.1016/j.jare.2017.07.002
65. Li W, Bickhart DM, Ramunno L, Iamartino D, Williams JL, Liu GE. Genomic structural differences between cattle and River Buffalo identified through comparative genomic and transcriptomic analysis. Data Brief. (2018) 19:236–9. doi: 10.1016/j.dib.2018.05.015
66. da Rosa FT, Moreira CG, Barbero MM, Hurtado Lugo NA, de Camargo GM, Aspicueta Borquis RR, et al. Associations between MUC1 gene polymorphism and resistance to mastitis, milk production and fertility traits in Murrah water buffaloes. J Appl Anim Res. (2020) 48:151–5. doi: 10.1080/09712119.2020.1749641
67. Höglund JK, Sahana G, Brøndum RF, Guldbrandtsen B, Buitenhuis B, Lund MS. Fine mapping QTL for female fertility on BTA04 and BTA13 in dairy cattle using HD SNP and sequence data. BMC Genom. (2014) 15:1–0. doi: 10.1186/1471-2164-15-790
68. Perumal P, Chamuah JK, Srivastava N, Vupru K, Srivastava SK. Infectious causes of infertility in buffalo bull (Bubalus bubalis). Int J Biores Stress Manage. (2013) 4:84–90. Available online at: https://ibic.lib.ku.ac.th/e-Bulletin/IBBU201302001.pdf
69. Li C, Cai W, Zhou C, Yin H, Zhang Z, Loor JJ, et al. RNA-Seq reveals 10 novel promising candidate genes affecting milk protein concentration in the Chinese Holstein population. Sci Rep. (2016) 6:1. doi: 10.1038/srep26813
70. Prajapati BM, Gupta JP, Pandey DP, Parmar GA, Chaudhari JD. Molecular markers for resistance against infectious diseases of economic importance. Vet World. (2017) 10:112. doi: 10.14202/vetworld.2017.112-120
71. Buitenhuis B, Røntved CM, Edwards SM, Ingvartsen KL, Sørensen P. In depth analysis of genes and pathways of the mammary gland involved in the pathogenesis of bovine Escherichia coli-mastitis. BMC Genom. (2011) 12:1–0. doi: 10.1186/1471-2164-12-130
72. Fagiolo A, Lai O. Mastitis in buffalo. Ital J Anim Sci. (2007) 6:200–6. doi: 10.4081/ijas.2007.s2.200
73. Heringstad B, Chang YM, Gianola D, Klemetsdal G. Genetic analysis of clinical mastitis, milk fever, ketosis, and retained placenta in three lactations of Norwegian red cows. J Dairy Sci. (2005) 88:3273–81. doi: 10.3168/jds.S0022-0302(05)73010-1
74. Suratanee A, Plaimas K. Network-based association analysis to infer new disease-gene relationships using large-scale protein interactions. PLoS ONE. (2018) 13:e0199435. doi: 10.1371/journal.pone.0199435
75. Rezaei-Tavirani M, Rezaei-Tavirani S, Mansouri V, Rostami-Nejad M, Rezaei-Tavirani M. Protein-protein interaction network analysis for a biomarker panel related to human esophageal adenocarcinoma. Asian Pac J Cancer Prev. (2017) 18:3357. doi: 10.5812/ijcm.65701
76. Mingala CN, Odbileg R, Konnai S, Ohashi K, Onuma M. Molecular cloning, sequencing and phylogenetic analysis of inflammatory cytokines of swamp type buffalo contrasting with other bubaline breeds. Comp Immunol Microbiol Infect Dis. (2007) 30:119–31. doi: 10.1016/j.cimid.2006.11.005
77. Kerr DE, Wellnitz O. Mammary expression of new genes to combat mastitis. J Anim Sci. (2003) 81:38–47. doi: 10.2527/2003.81suppl_338x
78. Li L, Chen X, Chen Z. Identification of key candidate genes in dairy cow in response to Escherichia coli mastitis by bioinformatical analysis. Front Genet. (2019) 10:1251. doi: 10.3389/fgene.2019.01251
79. Hogarth CJ, Fitzpatrick JL, Nolan AM, Young FJ, Pitt A, Eckersall PD. Differential protein composition of bovine whey: a comparison of whey from healthy animals and from those with clinical mastitis. Proteomics. (2004) 4:2094–100. doi: 10.1002/pmic.200300723
80. Sugimoto M, Fujikawa A, Womack JE, Sugimoto Y. Evidence that bovine forebrain embryonic zinc finger-like gene influences immune response associated with mastitis resistance. Proc Natl Acad Sci USA. (2006) 103:6454–9. doi: 10.1073/pnas.0601015103
81. Thompson-Crispi KA, Sewalem A, Miglior F, Mallard BA. Genetic parameters of adaptive immune response traits in Canadian Holsteins. J Dairy Sci. (2012) 95:401–9. doi: 10.3168/jds.2011-4452
82. Thompson-Crispi KA, Hine B, Quinton M, Miglior F, Mallard BA. Association of disease incidence and adaptive immune response in Holstein dairy cows. J Dairy Sci. (2012) 95:3888–93. doi: 10.3168/jds.2011-5201
83. Shastry BS. SNPs in disease gene mapping, medicinal drug development and evolution. J Hum Genet. (2007) 52:871–80. doi: 10.1007/s10038-007-0200-z
84. Upadhyay MR, Patel AB, Subramanian RB, Shah TM, Jakhesara SJ, Bhatt VD, et al. Single nucleotide variant detection in Jaffrabadi buffalo (Bubalus bubalis) using high-throughput targeted sequencing. Front Life Sci. (2015) 8:192–9. doi: 10.1080/21553769.2015.1031915
85. Mishra DC, Sikka P, Yadav S, Bhati J, Paul SS, Jerome A, et al. Identification and characterization of trait-specific SNPs using ddRAD sequencing in water buffalo. Genom. (2020) 112:3571–8. doi: 10.1016/j.ygeno.2020.04.012
86. Zhan X, Dixon A, Batbayar N, Bragin E, Ayas Z, Deutschova L, et al. Exonic versus intronic SNPs: contrasting roles in revealing the population genetic differentiation of a widespread bird species. Heredity. (2015) 114:1–9. doi: 10.1038/hdy.2014.59
87. Jacob KK, Radhika G, Aravindakshan TV. An in silico evaluation of non-synonymous single nucleotide polymorphisms of mastitis resistance genes in cattle. Anim. Biotechnol. (2020) 31:25–31. doi: 10.1080/10495398.2018.1524770
88. Väli Ü, Brandström M, Johansson M, Ellegren H. Insertion-deletion polymorphisms (indels) as genetic markers in natural populations. BMC Genet. (2008) 9:1–8. doi: 10.1186/1471-2156-9-8
89. Zhang Y, Wang K, Liu J, Zhu H, Qu L, Chen H, et al. An 11-bp indel polymorphism within the CSN1S1 gene is associated with milk performance and body measurement traits in chinese goats. Animals. (2019) 9:1114. doi: 10.3390/ani9121114
90. Mei C, Junjvlieke Z, Raza SH, Wang H, Cheng G, Zhao C, et al. Copy number variation detection in Chinese indigenous cattle by whole genome sequencing. Genom. (2020) 112:831–6. doi: 10.1016/j.ygeno.2019.05.023
91. Warren CF, Wong-Brown MW, Bowden NA. BCL-2 family isoforms in apoptosis and cancer. Cell Death Dis. (2019) 10:1–2. doi: 10.1038/s41419-019-1407-6
92. Kapila N, Sharma A, Kishore A, Sodhi M, Tripathi PK, Mohanty AK, et al. Impact of heat stress on cellular and transcriptional adaptation of mammary epithelial cells in riverine buffalo (Bubalus bubalis). PLoS ONE. (2016) 11:e0157237. doi: 10.1371/journal.pone.0157237
93. Wang X, Zhong J, Gao Y, Ju Z, Huang J. A SNP in intron 8 of CD46 causes a novel transcript associated with mastitis in Holsteins. BMC Genom. (2014) 15:1. doi: 10.1186/1471-2164-15-630
94. Deng T, Liang A, Liang S, Ma X, Lu X, Duan A, et al. Integrative analysis of transcriptome and GWAS data to identify the hub genes associated with milk yield trait in buffalo. Front Genet. (2019) 10:36. doi: 10.3389/fgene.2019.00036
95. Zhang H, Wei Y, Zhang F, Liu Y, Li Y, Li G, et al. Polymorphisms of MASP2 gene and its relationship with mastitis and milk production in Chinese Holstein cattle. Biotechnol Biotechnol Equip. (2019) 33:589–96. doi: 10.1080/13102818.2019.1596755
96. Mesquita AQ, Mesquita AJ, Jardim EA, Kipnis AP. Association of TLR4 polymorphisms with subclinical mastitis in Brazilian holsteins. Braz J Microbiol. (2012) 43:692–7. doi: 10.1590/S1517-83822012000200034
97. Liu YX, Xu CH, Gao TY, Sun Y. Polymorphisms of the ATP1A1 gene associated with mastitis in dairy cattle. Genet Mol Res. (2012) 11:651–60. doi: 10.4238/2012.March.16.3
98. Muhaghegh DM. Single nucleotide polymorphism in the promoter region of bovine Interleukin 8 gene and its association with milk production traits and somatic cell score of Holstein cattle in Iran. Iran. J Biotechnol. (2014) 12:36–41. doi: 10.15171/ijb.1016
99. Bhattarai D, Chen X, ur Rehman Z, Hao X, Ullah F, Dad R, et al. Association of MAP4K4 gene single nucleotide polymorphism with mastitis and milk traits in Chinese Holstein cattle. J Dairy Res. (2017) 84:76. doi: 10.1017/S0022029916000832
100. Pokorska J, Piestrzyńska-Kajtoch A, Kułaj D, Ochrem A, Radko A. Polymorphism of bovine lipocalin-2 gene and its impact on milk production traits and mastitis in Holstein Friesian cattle. Electron J Biotechnol. (2019) 40:17–21. doi: 10.1016/j.ejbt.2019.04.004
101. Prebavathy T, Thanislass J, Dhanammal L, Ganesan R, Mukhopadhyay HK. Association between SNPs in TLR2 gene segment corresponding to LRR functional domain of TLR2 receptor and bovine mastitis. Asian J Anim Sci. (2015) 9:45–56. doi: 10.3923/ajas.2015.45.56
102. Li J, Wang Q, Chen F, Wang H, Chen J, Wang Z, et al. SNPs of CD14 change the mastitis morbidity of Chinese Holstein. Mol Med Rep. (2017) 16:9102–10. doi: 10.3892/mmr.2017.7727
103. Gupta PH, Patel NA, Rank DN, Joshi CG. Genetic polymorphism of toll-like receptors 4 gene by polymerase chain reaction-restriction fragment length polymorphisms, polymerase chain reaction-single-strand conformational polymorphism to correlate with mastitic cows. Vet World. (2015) 8:615. doi: 10.14202/vetworld.2015.615-620
104. Noori R, Mahdavi AH, Edriss MA, Rahmani HR, Talebi M, Soltani-Ghombavani M. Association of polymorphism in Exon 3 of toll-like receptor 4 gene with somatic cell score and milk production traits in Holstein dairy cows of Iran. S Afr J Anim Sci. (2013) 43:493–8. doi: 10.4314/sajas.v43i4.6
105. Yang SH, Bi XJ, Xie Y, Li C, Zhang SL, Zhang Q, et al. Validation of PDE9A gene identified in GWAS showing strong association with milk production traits in Chinese Holstein. Int J Mol Sci. (2015) 16:26530–42. doi: 10.3390/ijms161125976
106. Sugimoto M, Sugimoto Y. Variant in the 5′ untranslated region of insulin-like growth factor 1 receptor is associated with susceptibility to mastitis in cattle. G3 Genes Genomes Genet. (2012) 2:1077–84. doi: 10.1534/g3.112.003095
Keywords: GWAS, mammary gland, markers, mastitis, TGP, water buffalo
Citation: Jaiswal S, Jagannadham J, Kumari J, Iquebal MA, Gurjar AKS, Nayan V, Angadi UB, Kumar S, Kumar R, Datta TK, Rai A and Kumar D (2021) Genome Wide Prediction, Mapping and Development of Genomic Resources of Mastitis Associated Genes in Water Buffalo. Front. Vet. Sci. 8:593871. doi: 10.3389/fvets.2021.593871
Received: 11 August 2020; Accepted: 30 April 2021;
Published: 18 June 2021.
Edited by:
Antonio Minervino, Federal University of Western Pará, BrazilReviewed by:
Madhu Tantia, National Bureau of Animal Genetic Resources (NBAGR), IndiaAngela Cánovas, University of Guelph, Canada
Copyright © 2021 Jaiswal, Jagannadham, Kumari, Iquebal, Gurjar, Nayan, Angadi, Kumar, Kumar, Datta, Rai and Kumar. This is an open-access article distributed under the terms of the Creative Commons Attribution License (CC BY). The use, distribution or reproduction in other forums is permitted, provided the original author(s) and the copyright owner(s) are credited and that the original publication in this journal is cited, in accordance with accepted academic practice. No use, distribution or reproduction is permitted which does not comply with these terms.
*Correspondence: Dinesh Kumar, dinesh.kumar@icar.gov.in