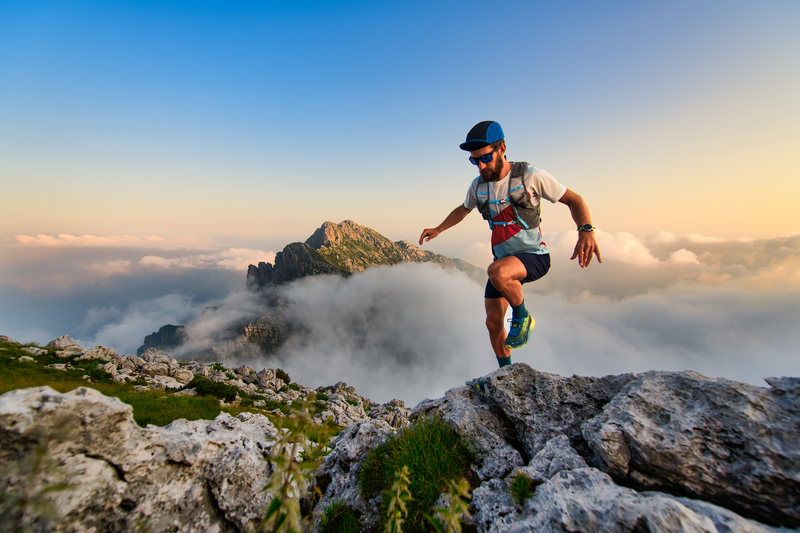
94% of researchers rate our articles as excellent or good
Learn more about the work of our research integrity team to safeguard the quality of each article we publish.
Find out more
EDITORIAL article
Front. Toxicol. , 12 February 2025
Sec. In Vitro Toxicology
Volume 7 - 2025 | https://doi.org/10.3389/ftox.2025.1568453
This article is part of the Research Topic Leveraging Artificial Intelligence and Open Science for Toxicological Risk Assessment View all 6 articles
Editorial on the Research Topic
Leveraging artificial intelligence and open science for toxicological risk assessment
The paradigm shift brought about by artificial intelligence (AI) across scientific disciplines has been nothing short of revolutionary. From unraveling the mysteries of protein folding to enabling autonomous systems, AI has demonstrated its potential to tackle previously intractable problems (Jumper et al., 2021; Abramoff et al., 2023). In toxicology, this transformation arrives at a crucial moment, as we face mounting challenges in chemical safety assessment and an urgent need to reduce reliance on animal testing (Hartung, 2023a; Hartung, 2023b; Kleinstreuer and Hartung, 2024).
This Research Topic emerged from a recognition that while computational toxicology has made significant strides using classical approaches such as physiologically-based pharmacokinetic (PBPK) modeling and quantitative structure-activity relationships (QSAR), the full potential of modern AI techniques remains largely untapped in toxicological risk assessment. The recent advances in machine learning, particularly deep learning, natural language processing, and semantic interoperability, offer unprecedented opportunities to integrate diverse data sources and create more predictive models for human health outcomes.
The five contributions in this Research Topic showcase innovative approaches that bridge traditional toxicological methods with cutting-edge AI applications. Collectively, these works demonstrate the potential for AI to enhance our understanding of chemical hazards while advancing the development of more efficient, ethical, and human-relevant risk assessment strategies.
Vikram et al. “Predicting Carcinogenic Risk from Chemical-Induced Genomic Instability” This review highlights the potential of AI/ML and OMICS technologies to transform traditional toxicological assessments and predict genotoxicity and mutagenicity with higher accuracy. It discusses how AI/ML can establish biomarkers and signatures for early cancer detection, risk assessment, and monitoring of health impacts from chemical exposure. Additionally, it emphasizes how AI may accelerate the screening of chemicals for toxicological evaluation, optimizing resource use, and reducing reliance on animal testing.
Snyder et al. “sendigR: an R package to leverage the value of CDSIC SEND datasets for cross-study analysis” This paper introduces the “sendigR” R package, developed to facilitate cross-study analysis of nonclinical toxicology study data. It provides functions to build and query a relational SEND database and offers a user-friendly web interface for historical control data analyses. The package supports advanced analyses via custom SQL queries and can serve as a foundation for developing user-friendly web-based applications to assess the safety of investigational therapeutics.
Odje et al. “Unleashing the Potential of Cell Painting Assays for Compound Activity and Hazard Prediction” This review explores the use of Cell Painting (CP) assays in drug discovery and toxicology. It discusses integrating CP-based phenotypic data with compound structures into machine learning models to predict activities for various disease endpoints and identify underlying modes of action, all while reducing animal testing.
Corradi et al. “The application of Natural Language Processing for the extraction of mechanistic information in toxicology” This paper demonstrates the use of Natural Language Processing (NLP) to extract information from scientific texts, speeding up reviews and discoveries. It applies NLP to select chemicals for testing toxicological endpoints and construct adverse outcome pathways. The authors present a proof-of-concept for NLP in toxicology and offer an open-source model for recognizing toxicological entities and their relationships.
Trovato et al. “Cross clinical-experimental-computational qualification of in silico drug trials on human cardiac Purkinje cells for pro-arrhythmia risk prediction” This study quantifies drug-induced electrophysiological effects on in silico human cardiac Purkinje cells, comparing them with in vitro rabbit data to assess their accuracy in predicting clinical pro-arrhythmia risk. It demonstrates the higher accuracy of in silico methods compared to in vitro animal models for proarrhythmic risk prediction and highlights the consistency with in vitro experiments used in safety pharmacology.
A common thread throughout these contributions is the emphasis on open science principles and reproducibility. The authors have shared their code, data, and methodologies through public repositories, enabling others to build upon their work. This commitment to transparency and collaboration exemplifies the transformation that AI is bringing not only to scientific problem-solving but also to the way we conduct and share research.
Looking ahead, several challenges and opportunities emerge from these works. First, while AI shows promise in predicting toxicological endpoints, integrating these predictions into regulatory frameworks remains a significant hurdle (Hartung and Kleinstreuer, 2025). Second, the quality and accessibility of training data continue to be limiting factors in developing robust AI models. Finally, there is a pressing need for standardized approaches to validate AI-driven predictions in toxicology.
Nevertheless, the works presented in this Research Topic demonstrate that we are moving closer to establishing a true probabilistic risk assessment framework (Maertens et al., 2022; Maertens et al., 2024) that incorporates the full potential of AI. Such a framework could not only reduce animal testing but also provide more accurate predictions of human health effects. The integration of multiple data streams - from chemical structures to historical animal data, in vitro assays, and omics measurements - through AI approaches represents a promising path forward.
We hope this Research Topic will serve as both an inspiration and a practical resource for researchers working at the intersection of AI and toxicology. As we continue to advance these methods, we move closer to more efficient, ethical, and accurate approaches to chemical safety assessment.
MT: Writing–review and editing. TL: Writing–review and editing. TH: Writing–original draft.
The author(s) declare that financial support was received for the research, authorship, and/or publication of this article. This work has received funding from the European Union’s Horizon 2020 research and innovation program under grant agreement No. 963845 (ONTOX).
TL is founder and owner of Tox-Track Inc. and Insilica LLC. TH holds stock options in and consults Tox-Track LLC Inc. and Insilica LLC. Both are consultants for computational toxicology for Underwriters Laboratories (UL) and receive shares of their respective sales. TH is a member of Apple’s Green Chemistry Advisory Board.
The remaining author declares that the research was conducted in the absence of any commercial or financial relationships that could be construed as a potential conflict of interest.
The author(s) declared that they were an editorial board member of Frontiers, at the time of submission. This had no impact on the peer review process and the final decision.
The author(s) declare that no Generative AI was used in the creation of this manuscript.
All claims expressed in this article are solely those of the authors and do not necessarily represent those of their affiliated organizations, or those of the publisher, the editors and the reviewers. Any product that may be evaluated in this article, or claim that may be made by its manufacturer, is not guaranteed or endorsed by the publisher.
AI, Artificial Intelligence; CDISC, Clinical Data Interchange Standards Consortium; CP, Cell Painting; ML, Machine Learning; NLP, Natural Language Processing; OMICS, The collective methods for studying the whole of biological molecules on organismal or population level; PBPK, Physiology-Based Pharmaco Kinetic(s); QSAR, Quantitative Structure-Activity Relationships; SEND, Standard for the Exchange of Nonclinical Data; SQL, Structured Query Language.
Abramoff, M. D., Whitestone, N., Patnaik, J. L., Rich, E., Ahmed, M., Husain, L., et al. (2023). Autonomous artificial intelligence increases real-world specialist clinic productivity in a cluster-randomized trial. NPJ Digit. Med. 6 (1), 184. doi:10.1038/s41746-023-00931-7
Hartung, T. (2023a). AI as the new frontier in chemical risk assessment. Frontiers in AI, sec. Med. Public Health 40, 559–570. doi:10.3389/frai.2023.1269932
Hartung, T. (2023b). ToxAIcology - the evolving role of artificial intelligence in advancing toxicology and modernizing regulatory science. ALTEX 40, 559–570. doi:10.14573/altex.2309191
Hartung, T., and Kleinstreuer, N. (2025). Challenges and opportunities for validation of AI-based new approach methods. ALTEX 42, 3–21. doi:10.14573/altex.2412291
Jumper, J., Evans, R., Pritzel, A., Green, T., Figurnov, M., Ronneberger, O., et al. (2021). Highly accurate protein structure prediction with AlphaFold. Nature 596, 583–589. doi:10.1038/s41586-021-03819-2
Kleinstreuer, N., and Hartung, T. (2024). Artificial intelligence (AI)-it's the end of the tox as we know it (and I feel fine). Archives Toxicol. 98, 735–754. doi:10.1007/s00204-023-03666-2
Maertens, A., Antignac, E., Benfenati, E., Bloch, D., Fritsche, E., Hoffmann, S., et al. (2024). The probable future of toxicology - probabilistic risk assessment. ALTEX 41, 273–281. doi:10.14573/altex.2310301
Keywords: artificial intelligence, machine learning, toxicology, risk assessment, open science, computational toxicology, predictive modeling
Citation: Teunis M, Luechtefeld T and Hartung T (2025) Editorial: Leveraging artificial intelligence and open science for toxicological risk assessment. Front. Toxicol. 7:1568453. doi: 10.3389/ftox.2025.1568453
Received: 29 January 2025; Accepted: 03 February 2025;
Published: 12 February 2025.
Edited and reviewed by:
Stina Oredsson, Lund University, SwedenCopyright © 2025 Teunis, Luechtefeld and Hartung. This is an open-access article distributed under the terms of the Creative Commons Attribution License (CC BY). The use, distribution or reproduction in other forums is permitted, provided the original author(s) and the copyright owner(s) are credited and that the original publication in this journal is cited, in accordance with accepted academic practice. No use, distribution or reproduction is permitted which does not comply with these terms.
*Correspondence: Thomas Hartung, dGhhcnR1bmdAamhzcGguZWR1
Disclaimer: All claims expressed in this article are solely those of the authors and do not necessarily represent those of their affiliated organizations, or those of the publisher, the editors and the reviewers. Any product that may be evaluated in this article or claim that may be made by its manufacturer is not guaranteed or endorsed by the publisher.
Research integrity at Frontiers
Learn more about the work of our research integrity team to safeguard the quality of each article we publish.