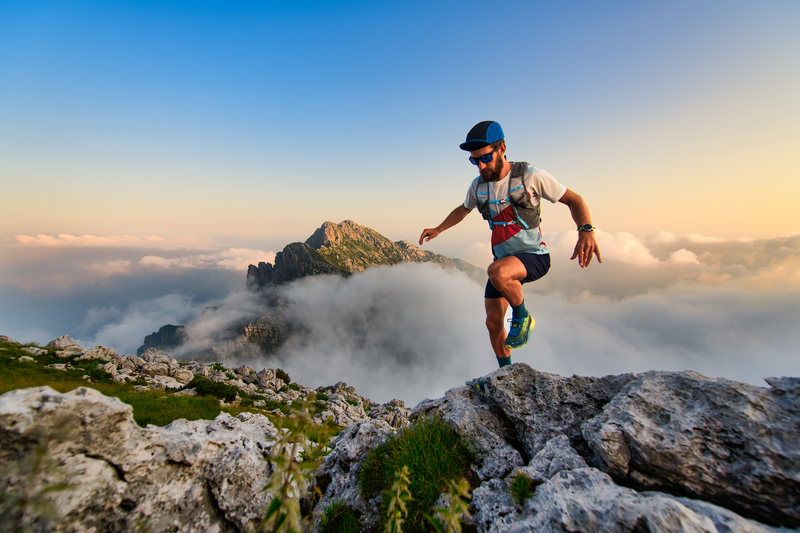
95% of researchers rate our articles as excellent or good
Learn more about the work of our research integrity team to safeguard the quality of each article we publish.
Find out more
ORIGINAL RESEARCH article
Front. Syst. Biol. , 27 March 2024
Sec. Data and Model Integration
Volume 4 - 2024 | https://doi.org/10.3389/fsysb.2024.1367562
Perfluorooctane sulfonate (PFOS) is a ubiquitous pollutant in global aquatic ecosystems with increasing concern for its toxicity to aquatic wildlife through inadvertent exposures. To assess the likely adverse effects of PFOS exposure on aquatic wildlife inhabiting polluted ecosystems, there is a need to identify biomarkers of its exposure and toxicity. We used an integrated systems toxicological framework to identify physiologically relevant biomarkers of PFOS toxicity in fish. An in silico stoichiometric metabolism model of zebrafish (Danio rerio) was used to integrate available (published by other authors) metabolomics and transcriptomics datasets from in vivo toxicological studies with 5 days post fertilized embryo-larval life stage of zebrafish. The experimentally derived omics datasets were used as constraints to parameterize an in silico mathematical model of zebrafish metabolism. In silico simulations using flux balance analysis (FBA) and its extensions showed prominent effects of PFOS exposure on the carnitine shuttle and fatty acid oxidation. Further analysis of metabolites comprising the impacted metabolic reactions indicated carnitine to be the most highly represented cofactor metabolite. Flux simulations also showed a near dose-responsive increase in the pools for fatty acids and acyl-CoAs under PFOS exposure. Taken together, our integrative in silico results showed dyslipidemia effects under PFOS exposure and uniquely identified carnitine as a candidate metabolite biomarker. The verification of this prediction was sought in a subsequent in vivo environmental monitoring study by the authors which showed carnitine to be a modal biomarker of PFOS exposure in wild-caught fish and marine mammals sampled from the northern Gulf of Mexico. Therefore, we highlight the efficacy of FBA to study the properties of large-scale metabolic networks and to identify biomarkers of pollutant exposure in aquatic wildlife.
The health of organisms living in stressed or deteriorating ecosystems is typically assessed using biomarkers that represent normal vs. perturbed physiological functions (Lopez-Barea, 1995; Dahlhoff, 2004; Sarkar et al., 2006; Kerambrun et al., 2011; Kroon et al., 2017). Biomarkers can include the measurement of apical or biochemical endpoints that are representative of organismal fitness, such as survival, growth, and fecundity (Villeneuve and Garcia-Reyero, 2011), or use high-throughput “omics” methods (transcriptomics, proteomics, metabolomics) to assess the entirety of an organism’s biological complexity (Iguchi et al., 2005; Brinke and Buchinger, 2017). The datasets generated from omics analyses allows the generalized analysis of effects on biological pathways using various in silico (i.e., bioinformatics or computational biology) approaches (Ankley et al., 2010; Krewski et al., 2010; Moffat et al., 2015). However, an integrated physiological perspective is difficult to discern using conventional in silico pathways-based approaches as omics changes are mainly assigned to biological functions based upon ontological relationship (Kienhuis et al., 2011; Marmon et al., 2021).
At present, we lack a comprehensive framework for assessing the consequences of perturbations to specific biological pathways on organismal metabolic physiology (and likely fitness) (Ashburner et al., 2000; Hardy et al., 2012; Mi et al., 2013; Wolffe et al., 2020). Exceptions include when dose-response changes in select gene expressions relate to progressively worsening physiological outcomes (Waterman et al., 2010; Haggard et al., 2016). Such as is seen for exposure to hepatic toxicants and the outcome of tumor development (Waterman et al., 2010; Haggard et al., 2016), or exposures to endocrine disrupting chemicals and increasing physiological dysfunction (Sharpe, 2006; Bergman et al., 2012; La Merrill et al., 2020). Therefore, there is a need to understand how the perturbation of select (or ensembles of) biochemical pathways (or metabolic sub-systems), affects large-scale biological networks, in turn impacting physiological functions. This challenge necessitates the use of in silico models and methods that can enable the qualitative or quantitative determination of the metabolites or metabolic fluxes (i.e., catalytic capabilities of enzymes in metabolic pathways) that are required to maintain the overall adaptive potential of an organism’s physiology under changing environmental conditions. Given the multi-level (genome to phenome) and multi-variate (transcriptome, proteome, metabolome) complexity of biological systems, the development and use of physiologically representative in silico models is an active area of research (Thiele et al., 2020; Gopalakrishnan et al., 2022).
In this manuscript we used an integrated systems toxicological framework to identify the physiologically relevant biomarkers of perfluorooctane sulfonate (PFOS) toxicity in fish. We used a novel in silico computational biology approach to integrate metabolomics and transcriptomics datasets from in vivo toxicological studies exposing zebrafish (Danio rerio) to PFOS, with an in silico stoichiometric model of whole-organism metabolism of zebrafish. PFOS was chosen for analysis as it is a near ubiquitous pollutant in global aquatic ecosystems (Muir and Miaz, 2021; Kurwadkar et al., 2022), with concern for its toxicity to aquatic wildlife (Beach et al., 2006; Ankley et al., 2021) and humans through inadvertent exposures (Hansen et al., 2016; Blake and Fenton, 2020; Panieri et al., 2022). Typically, human and wildlife exposure to PFOS (and related per- and polyfluoroalkyl substances, or PFAS) can cause wide-ranging metabolic and endocrine disruptive effects (Ankley et al., 2021; Fenton et al., 2021). Therefore, the elucidation of biomarkers that can represent impacted biological pathways and portend the onset of adverse physiological effects, is a priority area for further research (Lee et al., 2020; Ankley et al., 2021).
The in silico approach taken in this manuscript involved integrating metabolomics and transcriptomics (RNA sequencing) datasets from in vivo toxicological studies, generated by other authors, in which embryo-larval life-stages of zebrafish were exposed to PFOS (Ortiz-Villanueva et al., 2018; Martínez et al., 2019). Zebrafish were chosen as a model organism due to their common use as an in vivo model for aquatic wildlife and human health (Hutchinson et al., 2003; Tanguay, 2018; Breuer and Patten, 2020). Untargeted metabolomics (Ortiz-Villanueva et al., 2018) and transcriptomics datasets (Martínez et al., 2019) from 5 days post fertilized (dpf) embryo-larval zebrafish exposed to PFOS (from 2 – 5 dpf) were used for model parameterization. Specifically, the omics datasets from both studies comprised equivalent exposure concentrations of a solvent control (0.2% v/v dimethyl sulfoxide or DMSO), 0.06, 0.6, or 2 µM PFOS, and were generated by the same research laboratory (Ortiz-Villanueva et al., 2018; Martínez et al., 2019). These datasets were used to constrain or parameterize an in silico stoichiometric model of zebrafish metabolism (Wang et al., 2021).
The zebrafish metabolism model comprised a stoichiometric matrix which balanced the conversions of 8,344 metabolites in 12,909 interlinked metabolic reactions, and with 61% of these reactions controlled by the transcripts of 2,714 genes (Wang et al., 2021). Analysis methods from the constraints-based reconstruction and analysis (COBRA) metabolic modeling framework were used to parameterize the zebrafish model and simulate the impacts of PFOS exposure on the metabolic physiology of zebrafish (Savinell and Palsson, 1992; Schellenberger et al., 2011; Lewis et al., 2012). The COBRA framework has been successfully used to study the optimal metabolic functions of various prokaryotic and eukaryotic organisms (including multi-tissue human metabolic models) (Ibarra et al., 2002; Fong et al., 2005; Duarte et al., 2007; Mo et al., 2009; Bordbar et al., 2011; Yim et al., 2011; Swainston et al., 2013; Aurich et al., 2015; Blais et al., 2017; Opdam et al., 2017; Herrmann et al., 2019; Thiele et al., 2020). Therefore, the in silico approach taken in this study enabled assessment of the adverse effects of PFOS exposure and helped to identify likely biomarkers of its toxicity effects in exposed aquatic wildlife. In particular, a metabolite biomarker discovered using the in silico analyses was later confirmed to be representative of PFOS exposure in aquatic wildlife (fish and dolphins) sampled from the northern Gulf of Mexico, namely, Galveston Bay (TX) and Mobile Bay (AL) (Nolen et al., 2024).
A zebrafish metabolic model constructed by Wang et al. (2021) was parameterized with in vivo experimental data and used for subsequent in silico simulations (overall approach is summarized in Supplementary Material S1). The model mathematically related the biochemical (i.e., enzyme catalyzed) conversions and transport of 8,344 metabolites through an interlinked network of 12,909 reactions, and with 61% of these reactions related to the transcripts of 2,714 genes (SSupplementary Material S2, Zebrafish Metabolic Model). The mathematical model of the metabolic network was as a
To create a base-model parameterized to the metabolic physiology of 5 dpf embryo-larval zebrafish, a combination of existing data (i.e., published by other authors) and new data generated by us for this study was used. For example, the proportional contributions of major biomass components required to generate per Gram dry weight (g DW) of 5 dpf embryo-larval zebrafish was estimated using methods detailed in Feist et al. (2007). Data on 5 dpf zebrafish protein and lipid fractions were obtained from Hachicho et al. (2015), trace ions from Kaushik et al. (2011), and the proportion of DNA was estimated from the genome size (in base pairs) as presented in the zebrafish genome assembly GRCz11 (http://uswest.ensembl.org/Danio_rerio/Info/Annotation) and converted to unit mass composition (Gregory et al., 2007). The organic acids pool was calculated as the remainder proportional fraction (all calculations are shown in Supplementary Material S2, Biomass Composition).
The specific amino acids, organic acids, and fatty acids comprising the protein, organic acid pool, and lipid fractions respectively for 5 dpf zebrafish were quantified in this study using untargeted metabolomics. Briefly, 100 newly fertilized wild-type (AB line) zebrafish embryos were purchased from the Zebrafish International Resource Center (ZIRC) and maintained until 5 dpf in E2 embryo medium (Westerfield, 2000). At 5 dpf, 50 cold-stunned embryo-larval zebrafish were pooled into two separate replicates, freeze-dried overnight (Labconco FreeZone 6 plus), with metabolites extracted into crushed dry ice/acetone using a pestle and mortar. The mixed homogenates were pelleted at 2,000 rcf for 10 min, with the acetone supernatants filtered at 12,000 rcf for 1 h (at 4°C) through Amicon Ultra-0.5 centrifugal filters (Millipore-Sigma). The resulting filtrates were analyzed using mass spectrometry by the Integrated Metabolomics Analysis Core (IMAC) at Texas A&M University.
Untargeted liquid chromatography high resolution accurate mass spectrometry (LC-HRAM) analysis was performed using a Q Exactive Plus orbitrap mass spectrometer (ThermoFisher, Waltham, MA) coupled to a binary pump HPLC (UltiMate 3,000, ThermoFisher). Full MS spectra was obtained at 70,000 resolution (200 m/z) with a scan range of 50–750 m/z. Full MS followed by data dependent (dd) MS2 scans were obtained at 35,000 resolution (MS1) and 17,500 resolution (MS2), with a 1.5 m/z isolation window and a stepped normalized collision energy (NCE of 20, 40, 60). Samples were maintained at 4°C before injection. The injection volume was 10 µL. Chromatographic separation was achieved on a Synergi Fusion 4 μm, 150 mm × 2 mm reverse phase column (Phenomenex, Torrance, CA) maintained at 30°C using a solvent gradient method. Sample acquisition was performed using Xcalibur (ThermoFisher). Data analysis was performed with Compound Discoverer 2.1 (ThermoFisher). The averaged area under curve (AUC) counts for the various biomass components of amino acids (protein fraction), intermediate metabolites (organic acids fraction), and fatty acids (lipid fraction); along with amounts of trace ions and nucleic acids, were converted to µmol gDW-1 zebrafish. The molar conversion to biomass constituents was based upon the protocols of Feist et al. (2007) and are detailed in Supplementary Material S2, Biomass Composition.
Zebrafish growth rate was calculated by considering the change in total body length (mm) over time from ∼2 to 21 dpf, using data from https://zfin.org/zf_info/zfbook/stages/and Kimmel et al. (1995). The relationship between total body length and time was approximately linearized by plotting the cumulative change in total body length (mm) vs. the cumulative length increment per unit time (mm hr-1) (Supplementary Material S2, Growth and Metabolic Rate) (Hopkins, 1992). An oxygen consumption rate (OCR) for 5 dpf zebrafish was also calculated using the allometric relationship between routine (or resting) metabolic rate of zebrafish vs. mass (Rombough and Drader, 2009). And a cost of growth associated ATP maintenance (or COG) was calculated from the correlation between the OCR vs. relative growth rate (RGR) for zebrafish developmental life-stages spanning from 4 to 30 dpf. The RGR and COG were calculated using methods described in Conceição et al. (1998) and are detailed in Supplementary Material S2, Growth and Metabolic Rate. All physiological parameters were used to constrain the in silico stoichiometric model to generate a base-model that was representative of the metabolic physiology of 5 dpf zebrafish.
To convert the 5 dpf zebrafish base-model to condition-specific model’s representative of PFOS exposure, metabolomics and transcriptomics datasets from Martínez et al. (2019) and Ortiz-Villanueva et al. (2018) exposing 5 dpf embryo-larval zebrafish to a solvent control (0.2% v/v DMSO), 0.06, 0.6, or 2 µM PFOS, were obtained and prepared for base-model parameterization. Specifically for the metabolomics datasets, the auto-scaled relative abundances for the up- or downregulated metabolite levels (as reported in Supplementary Material of Ortiz-Villanueva et al. (2018)) were used to scale the AUC’s for the matched metabolites measured in the wild-type zebrafish (as described in Section 2.2) (Supplementary Material S2, PFOS Omics Data). The GSE125072 transcriptomics (RNA-sequencing) datasets of 5 dpf zebrafish exposed to a solvent control (0.2% v/v DMSO), 0.06, 0.6, or 2 µM PFOS were downloaded from the NCBIs GEO website (GSE125072 dataset as FASTQ files) using the NCBIs sequence read archive (SRA) Toolkit as initialized in a Cygwin-64 terminal. The resulting FASTQ files were processed using the OmicsBox bioinformatics toolbox v1.2 using established algorithms (BioBam, Valencia, Spain). For example, sequence alignment was performed using the STAR (v.2.7.9) aligner (Dobin et al., 2013), and using the zebrafish reference genome GRCz11. Gene expression quantification as a transcript count table was performed using the HTSeq package (Anders et al., 2015). Subsequently, all counts were log2 (n + 1) transformed prior to binarization using the BiTrinA toolbox v1.3 (Mundus et al., 2022) in RStudio (v4.2.0), and using the Binarization Across multiple SCales or BASC (A) algorithm (transcriptomics and binarized data is shown in Supplementary Material S2, PFOS Omics Data).
The zebrafish base-model was converted to condition-specific metabolic models representative of the various PFOS exposure concentrations (i.e., 0.2% v/v DMSO (solvent control), 0.06, 0.6, 2 µM PFOS) using the MetaboTools protocols (Aurich et al., 2015; Aurich et al., 2016), and as enabled in the COBRA toolbox (Schellenberger et al., 2011). All protocols were initialized in MATLAB (vR 2021b). The key parameterization steps are summarized as follows: First, the previously developed base-model was transformed to condition-specific model’s representative of PFOS exposure by constraining metabolite availabilities to generate various (or transformed) models reflective of the solvent control (0.2% v/v DMSO), 0.06, 0.6, or 2 µM PFOS exposure concentrations (n = 2 per treatment group) (Supplementary Material S2, PFOS Omics Data). Second, binarized transcriptomics datasets (described in Section 2.4) was used to parameterize the viable min/max bounds for reactions associated with the expressed metabolic enzyme genes only (and with nulled min/max bounds for the remainder of reactions). Third, imposition of the previously stated constraints led to the generation of three condition-specific metabolic models that were representative of PFOS exposures, namely, a Low exposure model (solvent control vs. 0.06 µM PFOS), Medium model (solvent control vs. 0.6 µM PFOS), and High model (solvent control vs. 2 μM PFOS) (Supplementary Material S2, PFOS Omics Data). While replicate datasets were used for model parameterization, single PFOS condition-specific models were generated using the MetaboTools protocols. Once constructed, the functional properties of each condition-specific metabolic model were studied in turn (all models are available in SBML format at https://www.ebi.ac.uk/biomodels/MODEL2403010004).
The structural and functional properties of each condition-specific metabolic model was studied using the COBRA toolbox (v2.0) as initialized in MATLAB (v2021b) (Schellenberger et al., 2011). For each model, the full range of min/max flux constraints required for the optimal production of ATP was determined using the flux variability analysis (FVA) computation (Mahadevan and Schilling, 2003). The production of ATP by each model was selected as a proxy of metabolic performance due to its positive correspondence with in vivo metabolic rate and organismal fitness (Salin et al., 2015) (Supplementary Material S2, Flux Analysis). The extent of intersection or overlap of the computed min/max flux ranges were compared across the three condition-specific metabolic models using a Jaccard index calculation. This analysis allowed identification of impacted reactions and associated metabolic sub-systems under PFOS exposure. Further analysis was performed to determine the extent of metabolite participation in the impacted reactions. Therefore, providing insights into metabolite connectivity in the condition-specific models and helping to identify candidate metabolite biomarkers that were diagnostic of PFOS exposure. Finally, flux balance analysis (FBA) was used to compute the optimal flux through select core metabolic reactions that produced key intermediate organic acids or lipids (Feist and Palsson, 2010; Orth et al., 2010). Optimal fluxes were calculated subject to limiting carbon substrate availability to per mol glucose or palmitolate (or palmitoleic acid), and ensuring unlimited O2, H2O, and CO2 availability to each condition-specific model.
Statistical analysis was performed using the Python programing language (v3.9.5), with associated data handling (pandas) and statistical (scipy, scikit) libraries. The normal distribution of data was tested using the Shapiro-Wilk test, with homogeneity of variance tested using the Levene test. In this manuscript, a non-parametric Spearman’s rank correlation only was tested (with α = 0.05). All graphs were plotted using python’s matplotlib visualization library.
The proportional contributions of major biomass components comprised: organic acid (0.47), protein (0.40), lipid (0.05), trace ion (0.05), and DNA (0.03) fractions (Supplementary Material S2, Biomass Composition). The inverse slope of the relationship between the cumulative change in total body length vs. the cumulative body length increment (Spearman Rank rho = −0.84, p-value = 0.002) yielded a growth rate of 0.011 h-1 (Supplementary Material S2, Growth and Metabolic Rate). An OCR of 52 µmol O2 gDW-1 hr-1 and COG of 209 µmol ATP gDW-1 hr-1 was calculated for 5 dpf embryo-larval zebrafish (Supplementary Material S2, Growth and Metabolic Rate). The OCR was calculated using the allometric relationship between the resting metabolic rate (nmol O2/hr) and mass (mg) of larval zebrafish as calculated by Rombough and Drader (2009). Our computed OCR value (using the allometric relationship) was similar to the experimentally measured OCR of ∼30–40 µmol O2 g wet weight-1 hr-1 for 5 dpf embryo-larval zebrafish reported in Bagatto et al. (2001). All experimentally derived physiological parameters were used as constraints to generate the in silico metabolic base-model for 5 dpf zebrafish. For example, the in vivo growth rate was used to constrain the lower bound of the in silico biomass generating reaction (MAR00021), the OCR was used to constrain the O2 uptake exchange reaction (MAR09048), and the COG was used to constrain the ATP maintenance (or renewal) reaction (MAR06916) (Supplementary Material S2, Zebrafish Metabolic Model).
The analysis of the union of reactions across the three, condition-specific metabolic models yielded a shared pool of 9,846 reactions, which constituted 76% of all reactions in the zebrafish base-model. A Venn diagram of shared or unique reactions amongst the condition-specific models revealed that 96% of metabolic reactions to be shared amongst the three models (Figure 1). Relative to the union of all reactions, the set of unique reactions was relatively small in each of the Low (0.7%), Medium (1.3%), or High (0.3%) PFOS exposed metabolic models. And while approximately twice as many reactions were shared between the Low and Medium models (120) vs. the Medium and High models (66), they only comprised 1.2% and 0.7% of all shared reactions (Figure 1). Therefore, given the relatively minimal distinctions in reactions between the three condition-specific models, comparisons of the simulated metabolic fluxes were sought to identify the likely effects of PFOS exposure on the metabolic physiology of zebrafish.
Figure 1. Venn diagram showing the extent of shared or unique metabolic reactions in the three condition-specific models generated using in vivo omics data from PFOS exposed embryo-larval zebrafish. The condition-specific metabolic models comprised Low PFOS (solvent control vs. 0.06 µM PFOS), Medium PFOS (solvent control vs. 0.6 µM PFOS), and High PFOS (solvent control vs. 2 μM PFOS).
The comparisons of min/max flux values for the reactions comprising the major metabolic sub-systems revealed a prominent effect on the carnitine shuttle (Figure 2). The Low PFOS exposure model showed the carnitine shuttle to be uniquely impacted as its min/max fluxes were non-overlapping with those of all other reactions in the Low condition-specific model. The carnitine shuttle sub-system also appeared to be consistently affected (or represented) across all metabolic models. Given that carnitine availability is essential for the regulation of fatty acid oxidation (Ramsay and Zammit, 2004; Longo et al., 2016), the other major metabolic sub-system to also be concomitantly impacted across all three condition-specific metabolic models included fatty acid oxidation (Figure 2).
Figure 2. Comparisons of min/max flux overlap across various metabolic sub-systems as represented in the zebrafish metabolic model. The heat map shows the extent to which (darker colors) a metabolic sub-system is represented in disjoint or Non-overlapping min/max flux ranges, Overlapping, or Equivalent (with equal min/max flux ranges). (A) Low PFOS = solvent control vs. 0.06 μM PFOS model, (B) Medium PFOS = solvent control vs. 0.6 μM PFOS model, and (C) High PFOS = solvent control vs. 2 μM PFOS model.
The analysis of metabolite connectivity or participation in the impacted metabolic reactions (i.e., non-overlapping and overlapping flux categories) revealed highly represented or conserved biomarkers that were diagnostic of PFOS exposure (Figure 3). An evaluation of highly represented metabolites in the Low PFOS exposed model included: carnitine (or L-carnitine) > cholesterol > malonyl-CoA; for the Medium PFOS model included only cholesterol; and for the High PFOS model included: carnitine > cholesterol > acetyl-CoA > SM-pool (sphingolipid metabolism) > PC-LD pool (glycerophospholipid metabolism) > GSH (glutathione). The prominent representation of carnitine in the Low and High PFOS models implicates impacts of PFOS exposure on long-chain fatty acyl-CoA transmembrane import into the mitochondria and subsequent β-oxidation (Gooding et al., 2004; Longo et al., 2016). The participation of additional intermediate metabolites that are on the nexus of lipid and energy metabolisms (i.e., acetyl-CoA, malonyl-CoA, cholesterol), strongly implicated adverse effects on dyslipidemia and organismal metabolic physiology (Erinc et al., 2021; Fenton et al., 2021).
Figure 3. Rank ordered metabolites listed based upon the extent of their connectivity or participation in the metabolic reactions with disjoint or Non-overlapping and Overlapping min/max flux ranges. This analysis identified carnitine to be highly represented in the Low and High PFOS metabolic models. (Low PFOS = solvent control vs. 0.06 µM PFOS model, Medium PFOS = solvent control vs. 0.6 µM PFOS model, and High PFOS = solvent control vs. 2 μM PFOS model.).
Finally, the metabolic capability of each condition-specific model to produce key biomass precursors was tested using FBA. Specifically, limiting carbon substrate availability to per mol glucose or palmitolate showed the Medium and High PFOS metabolic models to exhibit overall higher fluxes for lipid metabolism vs. for those for organic acids (pyruvate dehydrogenase or PDH; and α-ketoglutarate dehydrogenase or KGDH) (Figure 4). For example, fatty acid oxidation via the mitochondrial β-oxidation reaction of 3-ketoacyl-CoA thiolase (βOXD), and the biomass generating reaction pools for fatty acids and acyl-CoAs was approximately an order of magnitude higher in the Medium and High models vs. the Low model (Figure 4). These flux simulations indicate increased lipid metabolism under elevated PFOS exposure.
Figure 4. Graphs showing the contrasting abilities of the three condition-specific PFOS metabolic models to drive flux through key metabolic reactions or produce key biomass precursors under limited carbon availability of (A) 1 mol glucose or (B) 1 mol palmitolate to produce ATP subject to the availability of various organic carbon substrates. Low PFOS = solvent control vs. 0.06 µM PFOS model, Medium PFOS = solvent control vs. 0.6 µM PFOS model, and High PFOS = solvent control vs. 2 μM PFOS model. PDH = pyruvate dehydrogenase; KGDH = α-ketoglutarate dehydrogenase, βOXD = mitochondrial β-oxidation reaction of 3-ketoacyl-CoA thiolase.
In this manuscript, the analysis of condition-specific zebrafish metabolic models revealed the carnitine shuttle and fatty acid oxidation to be consistently modulated under PFOS exposure (Figure 2; Figure 3). This is not surprising as the two sub-systems are functionally interrelated. The carnitine shuttle involves the use of carnitine as a cofactor to transport acyl moieties of fatty acyl-CoAs across the mitochondrial outer and inner membranes. Carnitine is a hydrophilic quaternary amine endogenously produced from protein degradation (Longo et al., 2016; Li and Zhao, 2021). In the carnitine shuttle, the acyl moiety of acyl-CoAs is transferred from CoA to carnitine (enabled by carnitine palmitoyl transferase I or CPT-I) on the outer mitochondrial membrane. Acyl-carnitine transfer across the inner mitochondrial membrane culminates in its reconversion to acyl-CoA by carnitine palmitoyl transferase II (CPT-II), which is found on the inner (matrix facing) face of the inner mitochondrial membrane, whereas the enzyme carnitine acyl-carnitine translocase replenishes the replaced carnitine by shuttling it back across the mitochondrial membranes to the cytosol, thus renewing the cytosolic carnitine pool and preventing its depletion (Bartlett and Eaton, 2004; Houten and Wanders, 2010).
Modulation of the carnitine shuttle and fatty acid oxidation metabolic sub-systems strongly indicates PPARα induction. PPARα serves as a sensor of free fatty acid levels and is activated by the presence of a variety of saturated and unsaturated fatty acids (Berger and Moller, 2002). Once activated this receptor acts as a transcriptional master-regulator by up-regulating genes required for activating fatty acids to fatty acyl-CoAs (fatty acyl-CoA synthetase), and those involved in fatty acid transport into the cell (fatty acid transport proteins or FATPs), along with mitochondrial and peroxisomal β-oxidation genes that metabolize fatty acyl-CoAs to acetyl-CoA (Berger and Moller, 2002; Rakhshandehroo et al., 2010). As a result, PFOS mediated activation of PPARα is expected to be concomitant with elevated free fatty acid levels and fatty acyl-CoAs. In support of this expectation, carbon-restricted in silico FBA simulations using per mol glucose or palmitolate showed elevated metabolic flux (by almost an order of magnitude) through the fatty acid and fatty acyl-CoA pools in the Mid and High condition-specific PFOS metabolic models, relative to the Low PFOS model (Figure 4).
Taken together, our in silico results indicate dyslipidemia effects under PFOS exposure. The candidate metabolite biomarkers of PFOS exposure include carnitine, free fatty acids, or fatty acyl-CoAs. Whereas PPARα and its downstream target genes, particularly those involved with fatty acyl-CoA mobilization or breakdown in fatty acid oxidation may provide appropriate biomarkers for transcriptomics analyses. These biomarkers agree well with the experimental observations of Ortiz-Villanueva et al. (2018) and Martínez et al. (2019) from whose in vivo exposure studies with embryo-larval zebrafish, the metabolomics and transcriptomics datasets used for model parameterization were derived. While metabolomics analyses indicated effects on lipid metabolism (elevated glycerophospholipid, unsaturated fatty acids) (Ortiz-Villanueva et al., 2018), transcriptomics analyses indicated the induction of lipid transport and metabolism (Martínez et al., 2019). Of specific relevance to the in silico prediction of effects on carnitine (as co-factor) and the carnitine shuttle made here, a human epidemiological study by Hu et al. (2019) showed PFOS body-burdens (in maternal perinatal serum samples) to be uniquely associated with the over-representation of carnitine and activation of the carnitine shuttle (as determined from metabolomics analyses). These findings confirmed PFOS toxicity on lipid regulation and fatty acid metabolism (Hu et al., 2019). Interestingly, the serum concentrations of PFOS shown to illicit effects on the carnitine shuttle (≤200 ng mL-1 or ≤0.4 µm PFOS) (Hu et al., 2019), were within the in vivo toxicological concentrations used for in silico model parameterization in this study (i.e., 0.06, 0.6, 2 µM PFOS) (Ortiz-Villanueva et al., 2018; Martínez et al., 2019). Indicating a concentration-dependent conservation of adverse effects.
The relevance of any biomarker identified from laboratory-based toxicological assessments with model organisms (such as zebrafish), as being predictive of effects in unrelated aquatic wildlife species is likely to depend on the extent of genome or proteome sequence similarity (Lalone et al., 2013; LaLone et al., 2023). Interspecies differences in receptor sensitivity to a pollutant ligand has been clearly shown to relate to differences in the inducibility of metabolic systems and related toxicity (Rusyn et al., 2006; Feige et al., 2010). However, if there is conservation of response for effects on a particular metabolic sub-system, then evaluation of effects on key cofactor metabolites may provide a more generalized approach towards identifying biomarkers. Carnitine presents itself as one such biomarker for studying effects on fatty acid oxidation as it is indispensable for the mitochondrial import of fatty acyl-CoAs (Longo et al., 2016).
In environmental monitoring studies performed by the authors of this manuscript, hepatic carnitine levels were shown to positively correlate with PFOS body-burdens in fish and marine mammals (stranded dolphins) sampled from the northern Gulf of Mexico (namely, from Galveston Bay (TX) and Mobile Bay (AL)) (Nolen et al., 2024). Therefore, the in silico predictions presented in this manuscript and the subsequent in vivo experimental verification reported in Nolen et al. (2024), indicate that the dyslipidemia effects of PFOS exposure likely mediate through disruption of the carnitine shuttle and modulation of carnitine levels as cofactor. Furthermore, the apparent agreement between in silico and in vivo results highlights the efficacy of using FBA to study the physiologically representative properties of large-scale metabolic networks.
An additional cofactor such as Coenzyme A (CoA) may also be a candidate to explore as a biomarker for PFOS effects. CoA is also an essential cofactor responsible for activating carboxylic acid moieties on various intermediate metabolites (including fatty acids) to CoA-thioesters (or acyl-CoAs). In turn, acyl-CoAs participate in key oxidative and biosynthetic reactions (such as fatty acid β-oxidation and the TCA cycle) (Brass, 1994). Therefore, adjustments of free CoA/acyl-CoA ratios contribute to major redirections of carbon flux in healthy vs. stressed metabolic states, although such effects are likely to be inducible under very high levels of PFOS exposure, as appears to be the case under di (2-ethylhexyl) phthalate (DEHP) exposure (Sakurai et al., 1978; Hala et al., 2021).
To frame the in silico predicted effects determined in this manuscript with the broader base of knowledge on the in vivo toxicity effects of PFOS, we find that exposure to PFOS impacts a wide array of biomarkers, spanning from metabolic to endocrine systems. Such effects are explainable by its endocrine disruptive properties (Jensen and Leffers, 2008; Pípal et al., 2022). While endocrine disrupting chemicals (or EDCs) typically affect hormone signaling (Bergman et al., 2012; La Merrill et al., 2020), a broader suite of effects including metabolic disruption can also be considered (Grün and Blumberg, 2009; Heindel et al., 2017). PFOS appears to disrupt hormone production and signaling (Du et al., 2013; Salgado-Freiría et al., 2018), and impacts lipid or carbohydrate metabolisms in exposed organisms (Bjork et al., 2011; Chung et al., 2022). A comprehensive review of PFOS effects on lipid metabolism by Fragki et al. (2021) summarizes PPARα activation to be responsible for dyslipidemia effects. PPARα plays a critical role in regulating fatty acid metabolism via peroxisomal and mitochondrial β-oxidation (Berger and Moller, 2002). However, detailed transcriptomics comparisons between primary human vs. rodent hepatocytes exposed to PFOS indicate strong and concomitant activations of a broader suite of nuclear hormone receptors between the two species. These receptors included the constitutive androstane receptor (CAR) and pregnane X receptor (PXR) (Bjork et al., 2011). CAR/PXR are responsive to xenobiotic (synthetic exogenous chemicals or pollutants) exposures as they induce biotransformation enzyme activities (Wang et al., 2012), and also play a role in energy homeostasis by inducing lipogenesis and inhibiting gluconeogenesis (Moreau et al., 2008). Taken together, the nexus of PPARα (albeit weakly activated in humans; Takacs and Abbott (2007)), CAR, and PXR activations under PFOS exposure implicate dysregulations of energy homeostasis (particularly dyslipidemia) and consequent widespread adverse physiological effects. Therefore, it is not surprising that dyslipidemia is a consistent metabolic effect reported in humans exposed to PFOS (Christensen et al., 2019; Sunderland et al., 2019).
Similarly, the study of PFOS effects on zebrafish supports dyslipidemia effects via elevated fatty acid β-oxidation and nuclear hormone receptor activations (including PXR) reported (Cheng et al., 2016). The analysis of specific effects on fatty acid profiles shows dose-responsive increases in the medium-chain and long-chain saturated fatty acids of lauric (12:0) and myristic (14:0) acid in embryo-larval zebrafish exposed to PFOS (Sant et al., 2021). Overall, while laboratory studies convincingly show effects of PFOS exposure of endocrine and metabolic disruption (Lee et al., 2020), less is known of aquatic wildlife effects in field-based studies (Ankley et al., 2021). This is not surprising given the myriad of confounding variables that can impact biomarker assessments in wild-caught animals (Houde et al., 2011). For example, Guillette et al. (2020) report elevated PFOS body burdens in the serum of Striped Bass (Morone saxatilis) from a PFAS polluted river (Cape Fear River, NC, USA), to positively correlate with biomarkers of innate immune activity and hepatic injury. However, these correlations are potentially confounded by life-stage differences in fish, pathogen exposures, and likely exposures to mixtures of pollutants (as the river basin was also recipient of industrial and municipal discharges) (Heintz and Haws, 2021).
The requirement of a toxicity biomarker to be representative of exposure and/or adverse effects (Sanajou and Şahin, 2021), places a biomarker’s response within close proximity of a toxicants interaction with a molecular or biological target (the so called molecular initiating event) (Ankley et al., 2010). Close correspondence of elevated PFAS exposure with the activation of a responsive target receptor has been shown for the positive correlation between levels of select perfluoroalkyl carboxylates (PFCAs) and PPARα gene expression in the kidney tissue of stranded cetaceans (dolphins and whales) (Kurtz et al., 2019). In addition, Kurtz et al. (2019) also showed a positive correlation of PPARα expression with a target gene, cytochrome P450 4A (cyp4A), which is responsible for fatty acid metabolism (Simpson, 1997), and therefore strongly supporting a biologically relevant biomarker response (Kurtz et al., 2019). The Kurtz et al. (2019) study demonstrates that consideration of a “systems” view, such as the activations of responsive or inducible biomarkers (i.e., PPARα and cyp4a) can provide compelling weight-of-evidence of exposure to a specific pollutant and likely mechanistically associated adverse effects.
In this manuscript, the in silico predictions of effects on the carnitine shuttle and fatty acid metabolism agree with the general consensus of PFOS’ effects on lipid metabolism. Furthermore, our subsequent verification of the positive correlation between in vivo hepatic carnitine levels and PFOS body-burdens in fish and dolphins sampled from the northern Gulf of Mexico (Nolen et al., 2024), lends to the verification and validation of the in silico analyses reported in this manuscript. While the zebrafish metabolism model only accounted for effects on the metabolic reaction network, it precluded direct assessment of effects on nuclear hormone receptors (such as PPARs, CAR, PXR, etc.). We may infer that the metabolic effects simulated in this manuscript are consequent of transcriptional regulatory changes that may include perturbation of various transcription factors. The inclusion of transcriptional regulatory signaling and effects onto the metabolism model constitutes a further avenue for model improvement and research.
The zebrafish metabolic model represented the near entirety of organismal biochemistry (comprising 12,909 reactions) (Wang et al., 2021). As a result, the model did not represent a tissue-specific metabolic phenotype. This is not a limitation of the model as the omics datasets used for its parameterization represented whole-organism transcriptomics and metabolomics changes (Ortiz-Villanueva et al., 2018; Martínez et al., 2019). Furthermore, use of the MetaboTools application enabled the generation of a subset of condition-specific metabolic reactions that were representative of PFOS exposure. While the steady-state assumption of FBA presents a computationally efficient means to solve (or mathematically study the properties of) large scale metabolic models (Schilling et al., 1999; Orth et al., 2010), it precludes consideration of system dynamics (at least as parameterized in its current form). Such absent inclusion of system dynamics is considered a limitation of the FBA approach (Murphy et al., 2018). However, FBA and its extensions present an effective and unified mathematical framework with which to study the properties of large-scale metabolic networks. Specifically, the FBA framework can meaningfully integrate transcriptomics and metabolomics datasets as constraints to determine effects on metabolic phenotype (Lee et al., 2006; Lewis et al., 2012).
The high level of genomic orthology and biochemical conservation between zebrafish and humans ensures relevance to the predictions of adverse effects in humans (Howe et al., 2013; Breuer and Patten, 2020). And conservation of zebrafish endocrinology and biochemistry with other piscine species also makes it an effective model system to study other aquatic wildlife (Hutchinson et al., 2003; Busby et al., 2010). Overall, the results of our in silico analyses agree with the in vivo toxicological effects of PFOS exposure, with the cofactor carnitine uniquely identified as a likely conserved metabolic biomarker of PFOS effects on fatty acid oxidation or dyslipidemia.
Finally, consideration should also be given to the fact that there are over 3,000 structurally related PFAS homologs, which raises concerns for mixtures exposures and toxicity (Wang et al., 2017; Ankley et al., 2021). Despite such variety, only around two dozen or so PFAS are commonly detected in aquatic wildlife (and most of whom are aptly represented in the Environmental Protection Agency’s PFAS priority list (EPA, 2019)). And of these, PFOS is the most prominently detected (and at the highest concentrations) (extensively reviewed in Vendl et al. (2021)). Our own previous research has shown PFOS to be the most prominently detected homolog in aquatic biota (oysters and fish) from Galveston Bay (TX) (Nolen et al., 2022). Given that in humans and fish, PFAS exposures are commonly associated with dyslipidemia effects (Lee et al., 2020; Fenton et al., 2021), we may expect PFOS to be the most causal homolog for this etiology. Furthermore, studies assessing the mixtures toxicity of PFAS indicate additive or synergistic effects of exposure on dyslipidemia (Haug et al., 2023; Sadrabadi et al., 2024) and cell toxicity (Ojo et al., 2020; Addicks et al., 2023; Pierozan et al., 2023). Therefore, the prediction of carnitine as a likely biomarker of PFOS toxicity may also be a relatively modal or representative biomarker of general PFAS exposures.
An integrated in silico systems toxicological framework was used to identify the physiologically relevant biomarkers of PFOS toxicity in fish. FBA simulations revealed the carnitine shuttle and fatty acid oxidation to be consistently affected under PFOS exposure. Specifically, the co-factor carnitine was identified as being a diagnostic metabolite biomarker of PFOS exposure. These simulation predictions agree with in vivo toxicological studies using animal models (including fish) (Lee et al., 2020; Ankley et al., 2021), human epidemiological studies (Christensen et al., 2019; Hu et al., 2019; Sunderland et al., 2019), and our own environmental monitoring data that reports a positive correlation between hepatic carnitine levels and PFOS body-burdens in fish and dolphins sampled from the northern Gulf of Mexico (Nolen et al., 2024). A key finding of our study is that adverse effects on key cofactor metabolites may provide a generalized approach towards identifying causal biomarkers to assess pollutant exposure and toxicity.
The datasets presented in this study can be found in online repositories. The names of the repository/repositories and accession number(s) can be found below: https://www.ncbi.nlm.nih.gov/, GSE125072.
RN: Conceptualization, Data curation, Formal Analysis, Investigation, Project administration, Writing–review and editing. LP: Conceptualization, Funding acquisition, Writing–review and editing. KK: Conceptualization, Funding acquisition, Writing–review and editing. AQ: Conceptualization, Funding acquisition, Project administration, Writing–review and editing. DH: Conceptualization, Data curation, Formal Analysis, Funding acquisition, Investigation, Methodology, Project administration, Resources, Software, Supervision, Validation, Visualization, Writing–original draft, Writing–review and editing.
The author(s) declare that financial support was received for the research, authorship, and/or publication of this article. This work was supported by funds, in-part or whole, from the Galveston Bay Estuary Program Grant No. 582-22-30197 to DH, National Science Foundation Rapid Response Research Grant No. HDBE-1936174 to DH; NIEHS P30ES029067 grant distributed through the Texas A&M Center for Environmental Health Research (TiCER) to DH; and Research and Development program of the Texas General Land Office Oil Spill Prevention and Response Division under Grant No. 20-057-000-B908 to AQ.
We also greatly appreciate the Boost Scholarship to RN by the Research and Graduate Studies Office, Texas A&M University at Galveston.
The authors declare that the research was conducted in the absence of any commercial or financial relationships that could be construed as a potential conflict of interest.
All claims expressed in this article are solely those of the authors and do not necessarily represent those of their affiliated organizations, or those of the publisher, the editors and the reviewers. Any product that may be evaluated in this article, or claim that may be made by its manufacturer, is not guaranteed or endorsed by the publisher.
The Supplementary Material for this article can be found online at: https://www.frontiersin.org/articles/10.3389/fsysb.2024.1367562/full#supplementary-material
Addicks, G. C., Rowan-Carroll, A., Reardon, A. J. F., Leingartner, K., Williams, A., Meier, M. J., et al. (2023). Per- and polyfluoroalkyl substances (PFAS) in mixtures show additive effects on transcriptomic points of departure in human liver spheroids. Toxicol. Sci. 194, 38–52. doi:10.1093/toxsci/kfad044
Anders, S., Pyl, P. T., and Huber, W. (2015). HTSeq--a Python framework to work with high-throughput sequencing data. Bioinformatics 31, 166–169. doi:10.1093/bioinformatics/btu638
Ankley, G. T., Bennett, R. S., Erickson, R. J., Hoff, D. J., Hornung, M. W., Johnson, R. D., et al. (2010). Adverse outcome pathways: a conceptual framework to support ecotoxicology research and risk assessment. Environ. Toxicol. Chem. 29, 730–741. doi:10.1002/etc.34
Ankley, G. T., Cureton, P., Hoke, R. A., Houde, M., Kumar, A., Kurias, J., et al. (2021). Assessing the ecological risks of per- and polyfluoroalkyl substances: current state-of-the science and a proposed path forward. Environ. Toxicol. Chem. 40, 564–605. doi:10.1002/etc.4869
Ashburner, M., Ball, C. A., Blake, J. A., Botstein, D., Butler, H., Cherry, J. M., et al. (2000). Gene ontology: tool for the unification of biology. The Gene Ontology Consortium. Nat. Genet. 25, 25–29. doi:10.1038/75556
Aurich, M. K., Fleming, R. M. T., and Thiele, I. (2016). MetaboTools: a comprehensive toolbox for analysis of genome-scale metabolic models. Front. Physiol. 7, 327. doi:10.3389/fphys.2016.00327
Aurich, M. K., Paglia, G., Rolfsson, Ó., Hrafnsdóttir, S., Magnúsdóttir, M., Stefaniak, M. M., et al. (2015). Prediction of intracellular metabolic states from extracellular metabolomic data. Metabolomics 11, 603–619. doi:10.1007/s11306-014-0721-3
Bagatto, B., Pelster, B., and Burggren, W. W. (2001). Growth and metabolism of larval zebrafish: effects of swim training. J. Exp. Biol. 204, 4335–4343. doi:10.1242/jeb.204.24.4335
Bartlett, K., and Eaton, S. (2004). Mitochondrial beta-oxidation. Eur. J. Biochem. 271, 462–469. doi:10.1046/j.1432-1033.2003.03947.x
Beach, S., Newsted, J., Coady, K., and Giesy, J. P. (2006). Ecotoxicological evaluation of perfluorooctanesulfonate (PFOS). Rev. Environ. Contam. Toxicol. 186, 133–174. doi:10.1007/0-387-32883-1_5
Berger, J., and Moller, D. E. (2002). The mechanisms of action of PPARs. Annu. Rev. Med. 53, 409–435. doi:10.1146/annurev.med.53.082901.104018
Bergman, A., Heindel, J., Jobling, S., Kidd, K. A., and Zoeller, T. R. (2012). Stae of the science of endocrine disrupting chemicals 2012 - summary for decision-makers. Nairobi, Kenya: UNEP and WHO, 1–38.
Bjork, J. A., Butenhoff, J. L., and Wallace, K. B. (2011). Multiplicity of nuclear receptor activation by PFOA and PFOS in primary human and rodent hepatocytes. Toxicology 288, 8–17. doi:10.1016/j.tox.2011.06.012
Blais, E. M., Rawls, K. D., Dougherty, B. V., Li, Z. I., Kolling, G. L., Ye, P., et al. (2017). Reconciled rat and human metabolic networks for comparative toxicogenomics and biomarker predictions. Nat. Commun. 8, 14250. doi:10.1038/ncomms14250
Blake, B. E., and Fenton, S. E. (2020). Early life exposure to per- and polyfluoroalkyl substances (PFAS) and latent health outcomes: a review including the placenta as a target tissue and possible driver of peri- and postnatal effects. Toxicology 443, 152565. doi:10.1016/j.tox.2020.152565
Bordbar, A., Feist, A. M., Usaite-Black, R., Woodcock, J., Palsson, B. O., and Famili, I. (2011). A multi-tissue type genome-scale metabolic network for analysis of whole-body systems physiology. BMC Syst. Biol. 5, 180. doi:10.1186/1752-0509-5-180
Brass, E. P. (1994). Overview of coenzyme A metabolism and its role in cellular toxicity. Chem. Biol. Interact. 90, 203–214. doi:10.1016/0009-2797(94)90010-8
Breuer, M., and Patten, S. A. (2020). A great catch for investigating inborn errors of metabolism-insights obtained from zebrafish. Biomolecules 10, 1352. doi:10.3390/biom10091352
Brinke, A., and Buchinger, S. (2017). Toxicogenomics in environmental science. Adv. Biochem. Eng. Biotechnol. 157, 159–186. doi:10.1007/10_2016_15
Busby, E. R., Roch, G. J., and Sherwood, N. M. (2010). “5 - endocrinology of zebrafish: a small fish with a large gene pool,” in Fish physiology. Editors S. F. Perry, M. Ekker, A. P. Farrell, and C. J. Brauner (United States: Academic Press), 173–247.
Cheng, J., Lv, S., Nie, S., Liu, J., Tong, S., Kang, N., et al. (2016). Chronic perfluorooctane sulfonate (PFOS) exposure induces hepatic steatosis in zebrafish. Aquat. Toxicol. 176, 45–52. doi:10.1016/j.aquatox.2016.04.013
Christensen, K. Y., Raymond, M., and Meiman, J. (2019). Perfluoroalkyl substances and metabolic syndrome. Int. J. Hyg. Environ. Health 222, 147–153. doi:10.1016/j.ijheh.2018.08.014
Chung, S. M., Heo, D.-G., Kim, J.-H., Yoon, J. S., Lee, H. W., Kim, J.-Y., et al. (2022). Perfluorinated compounds in adults and their association with fasting glucose and incident diabetes: a prospective cohort study. Environ. Health 21, 101. doi:10.1186/s12940-022-00915-2
Conceição, L. E. C., Dersjant-Li, Y., and Verreth, J. A. J. (1998). Cost of growth in larval and juvenile African catfish (Clarias gariepinus) in relation to growth rate, food intake and oxygen consumption. Aquaculture 161, 95–106. doi:10.1016/s0044-8486(97)00260-3
Dahlhoff, E. P. (2004). Biochemical indicators of stress and metabolism: applications for marine ecological studies. Annu. Rev. Physiol. 66, 183–207. doi:10.1146/annurev.physiol.66.032102.114509
Dobin, A., Davis, C. A., Schlesinger, F., Drenkow, J., Zaleski, C., Jha, S., et al. (2013). STAR: ultrafast universal RNA-seq aligner. Bioinformatics 29, 15–21. doi:10.1093/bioinformatics/bts635
Du, G., Hu, J., Huang, H., Qin, Y., Han, X., Wu, D., et al. (2013). Perfluorooctane sulfonate (PFOS) affects hormone receptor activity, steroidogenesis, and expression of endocrine-related genes in vitro and in vivo. Environ. Toxicol. Chem. 32, 353–360. doi:10.1002/etc.2034
Duarte, N. C., Becker, S. A., Jamshidi, N., Thiele, I., Mo, M. L., Vo, T. D., et al. (2007). Global reconstruction of the human metabolic network based on genomic and bibliomic data. Proc. Natl. Acad. Sci. U. S. A. 104, 1777–1782. doi:10.1073/pnas.0610772104
EPA (2019). Method 533: determination of per- and polyfluoroalkyl substances in drinking water by isotope dilution anion exchange solid phase extraction and liquid chromatography/tandem mass spectrometry, 1–52.
Erinc, A., Davis, M. B., Padmanabhan, V., Langen, E., and Goodrich, J. M. (2021). Considering environmental exposures to per- and polyfluoroalkyl substances (PFAS) as risk factors for hypertensive disorders of pregnancy. Environ. Res. 197, 111113. doi:10.1016/j.envres.2021.111113
Feige, J. N., Gerber, A., Casals-Casas, C., Yang, Q., Winkler, C., Bedu, E., et al. (2010). The pollutant diethylhexyl phthalate regulates hepatic energy metabolism via species-specific PPARalpha-dependent mechanisms. Environ. Health Perspect. 118, 234–241. doi:10.1289/ehp.0901217
Feist, A. M., Henry, C. S., Reed, J. L., Krummenacker, M., Joyce, A. R., Karp, P. D., et al. (2007). A genome-scale metabolic reconstruction for Escherichia coli K-12 MG1655 that accounts for 1260 ORFs and thermodynamic information. Mol. Syst. Biol. 3, 121. doi:10.1038/msb4100155
Feist, A. M., and Palsson, B. O. (2010). The biomass objective function. Curr. Opin. Microbiol. 13, 344–349. doi:10.1016/j.mib.2010.03.003
Fenton, S. E., Ducatman, A., Boobis, A., DeWitt, J. C., Lau, C., Ng, C., et al. (2021). Per- and polyfluoroalkyl substance toxicity and human health review: current state of knowledge and strategies for informing future research. Environ. Toxicol. Chem. 40, 606–630. doi:10.1002/etc.4890
Fong, S. S., Burgard, A. P., Herring, C. D., Knight, E. M., Blattner, F. R., Maranas, C. D., et al. (2005). In silico design and adaptive evolution of Escherichia coli for production of lactic acid. Biotechnol. Bioeng. 91, 643–648. doi:10.1002/bit.20542
Fragki, S., Dirven, H., Fletcher, T., Grasl-Kraupp, B., Bjerve Gützkow, K., Hoogenboom, R., et al. (2021). Systemic PFOS and PFOA exposure and disturbed lipid homeostasis in humans: what do we know and what not? Crit. Rev. Toxicol. 51, 141–164. doi:10.1080/10408444.2021.1888073
Gooding, J. M., Shayeghi, M., and Saggerson, E. D. (2004). Membrane transport of fatty acylcarnitine and free l-carnitine by rat liver microsomes. Eur. J. Biochem. 271, 954–961. doi:10.1111/j.1432-1033.2004.03997.x
Gopalakrishnan, S., Joshi, C. J., Valderrama-Gómez, M., Icten, E., Rolandi, P., Johnson, W., et al. (2022). Guidelines for extracting biologically relevant context-specific metabolic models using gene expression data. Metab. Eng. 75, 181–191. doi:10.1016/j.ymben.2022.12.003
Gregory, T. R., Nicol, J. A., Tamm, H., Kullman, B., Kullman, K., Leitch, I. J., et al. (2007). Eukaryotic genome size databases. Nucleic Acids Res. 35, D332–D338. doi:10.1093/nar/gkl828
Grün, F., and Blumberg, B. (2009). Endocrine disrupters as obesogens. Mol. Cell Endocrinol. 304, 19–29. doi:10.1016/j.mce.2009.02.018
Guillette, T. C., McCord, J., Guillette, M., Polera, M. E., Rachels, K. T., Morgeson, C., et al. (2020). Elevated levels of per- and polyfluoroalkyl substances in Cape Fear River Striped Bass (Morone saxatilis) are associated with biomarkers of altered immune and liver function. Environ. Int. 136, 105358. doi:10.1016/j.envint.2019.105358
Hachicho, N., Reithel, S., Miltner, A., Heipieper, H. J., Küster, E., and Luckenbach, T. (2015). Body mass parameters, lipid profiles and protein contents of zebrafish embryos and effects of 2,4-dinitrophenol exposure. PLoS One 10, e0134755. doi:10.1371/journal.pone.0134755
Haggard, D. E., Noyes, P. D., Waters, K. M., and Tanguay, R. L. (2016). Phenotypically anchored transcriptome profiling of developmental exposure to the antimicrobial agent, triclosan, reveals hepatotoxicity in embryonic zebrafish. Toxicol. Appl. Pharmacol. 308, 32–45. doi:10.1016/j.taap.2016.08.013
Hala, D., Petersen, L. H., Huggett, D. B., Puchowicz, M. A., Brunengraber, H., and Zhang, G. F. (2021). Overcompensation of CoA trapping by di(2-ethylhexyl) phthalate (DEHP) metabolites in livers of wistar rats. Int. J. Mol. Sci. 22, 13489. doi:10.3390/ijms222413489
Hansen, S., Vestergren, R., Herzke, D., Melhus, M., Evenset, A., Hanssen, L., et al. (2016). Exposure to per- and polyfluoroalkyl substances through the consumption of fish from lakes affected by aqueous film-forming foam emissions - a combined epidemiological and exposure modeling approach. The SAMINOR 2 Clinical Study. Environ. Int. 94, 272–282. doi:10.1016/j.envint.2016.05.030
Hardy, B., Apic, G., Carthew, P., Clark, D., Cook, D., Dix, I., et al. (2012). Toxicology ontology perspectives. Altex 29, 139–156. doi:10.14573/altex.2012.2.139
Haug, M., Dunder, L., Lind, P. M., Lind, L., and Salihovic, S. (2023). Associations of perfluoroalkyl substances (PFAS) with lipid and lipoprotein profiles. J. Expo. Sci. Environ. Epidemiol. 33, 757–765. doi:10.1038/s41370-023-00545-x
Heindel, J. J., Blumberg, B., Cave, M., Machtinger, R., Mantovani, A., Mendez, M. A., et al. (2017). Metabolism disrupting chemicals and metabolic disorders. Reprod. Toxicol. 68, 3–33. doi:10.1016/j.reprotox.2016.10.001
Heintz, M. M., and Haws, L. C. (2021). Correspondence to the Editor Regarding Guillette et al. 2020, Elevated levels of per- and polyfluoroalkyl substances in Cape Fear River Striped Bass (Morone saxatilis) are associated with biomarkers of altered immune and liver function. Environ. Int. 146, 106299. doi:10.1016/j.envint.2020.106299
Herrmann, H. A., Dyson, B. C., Vass, L., Johnson, G. N., and Schwartz, J. M. (2019). Flux sampling is a powerful tool to study metabolism under changing environmental conditions. NPJ Syst. Biol. Appl. 5, 32. doi:10.1038/s41540-019-0109-0
Hopkins, K. D. (1992). Reporting fish growth: a review of the Basics1. J. World Aquac. Soc. 23, 173–179. doi:10.1111/j.1749-7345.1992.tb00766.x
Houde, M., De Silva, A. O., Muir, D. C. G., and Letcher, R. J. (2011). Monitoring of perfluorinated compounds in aquatic biota: an updated review. Environ. Sci. Technol. 45, 7962–7973. doi:10.1021/es104326w
Houten, S. M., and Wanders, R. J. (2010). A general introduction to the biochemistry of mitochondrial fatty acid β-oxidation. J. Inherit. Metab. Dis. 33, 469–477. doi:10.1007/s10545-010-9061-2
Howe, K., Clark, M. D., Torroja, C. F., Torrance, J., Berthelot, C., Muffato, M., et al. (2013). The zebrafish reference genome sequence and its relationship to the human genome. Nature 496, 498–503. doi:10.1038/nature12111
Hu, X., Li, S., Cirillo, P. M., Krigbaum, N. Y., Tran, V., Jones, D. P., et al. (2019). Metabolome wide association study of serum poly and perfluoroalkyl substances (PFASs) in pregnancy and early postpartum. Reprod. Toxicol. 87, 70–78. doi:10.1016/j.reprotox.2019.05.058
Hutchinson, T. H., Yokota, H., Hagino, S., and Ozato, K. (2003). Development of fish tests for endocrine disruptors. Pure Appl. Chem. 75, 2343–2353. doi:10.1351/pac200375112343
Ibarra, R. U., Edwards, J. S., and Palsson, B. O. (2002). Escherichia coli K-12 undergoes adaptive evolution to achieve in silico predicted optimal growth. Nature 420, 186–189. doi:10.1038/nature01149
Iguchi, T., Watanabe, H., and Katsu, Y. (2005). Application of ecotoxicogenomics for studying endocrine disruption in vertebrates and invertebrates. Environ. Health Perspect. 114, 101–105. doi:10.1289/ehp.8061
Jensen, A. A., and Leffers, H. (2008). Emerging endocrine disrupters: perfluoroalkylated substances. Int. J. Androl. 31, 161–169. doi:10.1111/j.1365-2605.2008.00870.x
Kaushik, S., Georga, I., and Koumoundouros, G. (2011). Growth and body composition of zebrafish (Danio rerio) larvae fed a compound feed from first feeding onward: toward implications on nutrient requirements. Zebrafish 8, 87–95. doi:10.1089/zeb.2011.0696
Kerambrun, E., Sanchez, W., Henry, F., and Amara, R. (2011). Are biochemical biomarker responses related to physiological performance of juvenile sea bass (Dicentrarchus labrax) and turbot (Scophthalmus maximus) caged in a polluted harbour? Comp. Biochem. Physiol. C Toxicol. Pharmacol. 154, 187–195. doi:10.1016/j.cbpc.2011.05.006
Kienhuis, A. S., Bessems, J. G., Pennings, J. L., Driessen, M., Luijten, M., van Delft, J. H., et al. (2011). Application of toxicogenomics in hepatic systems toxicology for risk assessment: acetaminophen as a case study. Toxicol. Appl. Pharmacol. 250, 96–107. doi:10.1016/j.taap.2010.10.013
Kimmel, C. B., Ballard, W. W., Kimmel, S. R., Ullmann, B., and Schilling, T. F. (1995). Stages of embryonic development of the zebrafish. Dev. Dyn. 203, 253–310. doi:10.1002/aja.1002030302
Krewski, D., Acosta, D., Andersen, M., Anderson, H., Bailar, J. C., Boekelheide, K., et al. (2010). Toxicity testing in the 21st century: a vision and a strategy. J. Toxicol. Environ. Health B Crit. Rev. 13, 51–138. doi:10.1080/10937404.2010.483176
Kroon, F., Streten, C., and Harries, S. (2017). A protocol for identifying suitable biomarkers to assess fish health: a systematic review. PLoS One 12, e0174762. doi:10.1371/journal.pone.0174762
Kurtz, A. E., Reiner, J. L., West, K. L., and Jensen, B. A. (2019). Perfluorinated alkyl acids in Hawaiian cetaceans and potential biomarkers of effect: peroxisome proliferator-activated receptor alpha and cytochrome P450 4A. Environ. Sci. Technol. 53, 2830–2839. doi:10.1021/acs.est.8b05619
Kurwadkar, S., Dane, J., Kanel, S. R., Nadagouda, M. N., Cawdrey, R. W., Ambade, B., et al. (2022). Per- and polyfluoroalkyl substances in water and wastewater: a critical review of their global occurrence and distribution. Sci. Total Environ. 809, 151003. doi:10.1016/j.scitotenv.2021.151003
LaLone, C. A., Blatz, D. J., Jensen, M. A., Vliet, S. M. F., Mayasich, S., Mattingly, K. Z., et al. (2023). From protein sequence to structure: the next frontier in cross-species extrapolation for chemical safety evaluations. Environ. Toxicol. Chem. 42, 463–474. doi:10.1002/etc.5537
Lalone, C. A., Villeneuve, D. L., Burgoon, L. D., Russom, C. L., Helgen, H. W., Berninger, J. P., et al. (2013). Molecular target sequence similarity as a basis for species extrapolation to assess the ecological risk of chemicals with known modes of action. Aquat. Toxicol. 144-145, 141–154. doi:10.1016/j.aquatox.2013.09.004
La Merrill, M. A., Vandenberg, L. N., Smith, M. T., Goodson, W., Browne, P., Patisaul, H. B., et al. (2020). Consensus on the key characteristics of endocrine-disrupting chemicals as a basis for hazard identification. Nat. Rev. Endocrinol. 16, 45–57. doi:10.1038/s41574-019-0273-8
Lee, J. M., Gianchandani, E. P., and Papin, J. A. (2006). Flux balance analysis in the era of metabolomics. Brief. Bioinform 7, 140–150. doi:10.1093/bib/bbl007
Lee, J. W., Choi, K., Park, K., Seong, C., Yu, S. D., and Kim, P. (2020). Adverse effects of perfluoroalkyl acids on fish and other aquatic organisms: a review. Sci. Total Environ. 707, 135334. doi:10.1016/j.scitotenv.2019.135334
Lewis, N. E., Nagarajan, H., and Palsson, B. O. (2012). Constraining the metabolic genotype-phenotype relationship using a phylogeny of in silico methods. Nat. Rev. Microbiol. 10, 291–305. doi:10.1038/nrmicro2737
Li, N., and Zhao, H. (2021). Role of carnitine in non-alcoholic fatty liver disease and other related diseases: an update. Front. Med. 8, 689042. doi:10.3389/fmed.2021.689042
Longo, N., Frigeni, M., and Pasquali, M. (2016). Carnitine transport and fatty acid oxidation. Biochim. Biophys. Acta 1863, 2422–2435. doi:10.1016/j.bbamcr.2016.01.023
Lopez-Barea, J. (1995). Biomarkers in ecotoxicology: an overview. Arch. Toxicol. Suppl. 17, 57–79. doi:10.1007/978-3-642-79451-3_6
Mahadevan, R., and Schilling, C. H. (2003). The effects of alternate optimal solutions in constraint-based genome-scale metabolic models. Metab. Eng. 5, 264–276. doi:10.1016/j.ymben.2003.09.002
Marmon, P., Owen, S. F., and Margiotta-Casaluci, L. (2021). Pharmacology-informed prediction of the risk posed to fish by mixtures of non-steroidal anti-inflammatory drugs (NSAIDs) in the environment. Environ. Int. 146, 106222. doi:10.1016/j.envint.2020.106222
Martínez, R., Navarro-Martín, L., Luccarelli, C., Codina, A. E., Raldúa, D., Barata, C., et al. (2019). Unravelling the mechanisms of PFOS toxicity by combining morphological and transcriptomic analyses in zebrafish embryos. Sci. Total Environ. 674, 462–471. doi:10.1016/j.scitotenv.2019.04.200
Mi, H., Muruganujan, A., Casagrande, J. T., and Thomas, P. D. (2013). Large-scale gene function analysis with the PANTHER classification system. Nat. Protoc. 8, 1551–1566. doi:10.1038/nprot.2013.092
Mo, M. L., Palsson, B. O., and Herrgård, M. J. (2009). Connecting extracellular metabolomic measurements to intracellular flux states in yeast. BMC Syst. Biol. 3, 37. doi:10.1186/1752-0509-3-37
Moffat, I., Chepelev, N. L., Labib, S., Bourdon-Lacombe, J., Kuo, B., Buick, J. K., et al. (2015). Comparison of toxicogenomics and traditional approaches to inform mode of action and points of departure in human health risk assessment of benzo[a]pyrene in drinking water. Crit. Rev. Toxicol. 45, 1–43. doi:10.3109/10408444.2014.973934
Moreau, A., Vilarem, M. J., Maurel, P., and Pascussi, J. M. (2008). Xenoreceptors CAR and PXR activation and consequences on lipid metabolism, glucose homeostasis, and inflammatory response. Mol. Pharm. 5, 35–41. doi:10.1021/mp700103m
Muir, D., and Miaz, L. T. (2021). Spatial and temporal trends of perfluoroalkyl substances in global ocean and coastal waters. Environ. Sci. Technol. 55, 9527–9537. doi:10.1021/acs.est.0c08035
Mundus, S., Müssel, C., Schmid, F., Lausser, L., Blätte, T., Hopfensitz, M., et al. (2022). Package BiTrinA. Available at: https://cran.r-project.org/web/packages/BiTrinA/BiTrinA.pdf (Accessed January 17, 2023).
Murphy, C. A., Nisbet, R. M., Antczak, P., Garcia-Reyero, N., Gergs, A., Lika, K., et al. (2018). “Linking adverse outcome pathways to dynamic energy budgets: a conceptual model,” in A systems biology approach to advancing adverse outcome pathways for risk assessment. Editors N. Garcia-Reyero, and C. A. Murphy (Cham: Springer International Publishing), 281–302.
Nolen, R. M., Faulkner, P., Ross, A. D., Kaiser, K., Quigg, A., and Hala, D. (2022). PFASs pollution in Galveston Bay surface waters and biota (shellfish and fish) following AFFFs use during the ITC fire at Deer Park (March 17th-20th 2019), Houston, TX. Sci. Total Environ. 805, 150361. doi:10.1016/j.scitotenv.2021.150361
Nolen, R. M., Prouse, A., Russell, M. L., Bloodgood, J., Clark, C. D., Cramichael, R. H., et al. (2024). Evaluation of fatty acids and carnitine as biomarkers of PFOS exposure in biota (fish and dolphins) from Galveston Bay and the northwestern Gulf of Mexico. Comp. Biochem. Physiology Part C Toxicol. Pharmacol. 276, 1–12. doi:10.1016/j.cbpc.2023.109817
Ojo, A. F., Peng, C., and Ng, J. C. (2020). Combined effects and toxicological interactions of perfluoroalkyl and polyfluoroalkyl substances mixtures in human liver cells (HepG2). Environ. Pollut. 263, 114182. doi:10.1016/j.envpol.2020.114182
Opdam, S., Richelle, A., Kellman, B., Li, S., Zielinski, D. C., and Lewis, N. E. (2017). A systematic evaluation of methods for tailoring genome-scale metabolic models. Cell Syst. 4, 318–329. doi:10.1016/j.cels.2017.01.010
Orth, J. D., Thiele, I., and Palsson, B. Ø. (2010). What is flux balance analysis? Nat. Biotechnol. 28, 245–248. doi:10.1038/nbt.1614
Ortiz-Villanueva, E., Jaumot, J., Martínez, R., Navarro-Martín, L., Piña, B., and Tauler, R. (2018). Assessment of endocrine disruptors effects on zebrafish (Danio rerio) embryos by untargeted LC-HRMS metabolomic analysis. Sci. Total Environ. 635, 156–166. doi:10.1016/j.scitotenv.2018.03.369
Panieri, E., Baralic, K., Djukic-Cosic, D., Buha Djordjevic, A., and Saso, L. (2022). PFAS molecules: a major concern for the human health and the environment. Toxics 10, 44. doi:10.3390/toxics10020044
Pierozan, P., Kosnik, M., and Karlsson, O. (2023). High-content analysis shows synergistic effects of low perfluorooctanoic acid (PFOS) and perfluorooctane sulfonic acid (PFOA) mixture concentrations on human breast epithelial cell carcinogenesis. Environ. Int. 172, 107746. doi:10.1016/j.envint.2023.107746
Pípal, M., Wiklund, L., Caccia, S., and Beronius, A. (2022). Assessment of endocrine disruptive properties of PFOS: EFSA/ECHA guidance case study utilising AOP networks and alternative methods. EFSA J. 20, e200418. doi:10.2903/j.efsa.2022.e200418
Rakhshandehroo, M., Knoch, B., Müller, M., and Kersten, S. (2010). Peroxisome proliferator-activated receptor alpha target genes. PPAR Res. 2010, 612089. doi:10.1155/2010/612089
Ramsay, R. R., and Zammit, V. A. (2004). Carnitine acyltransferases and their influence on CoA pools in health and disease. Mol. Asp. Med. 25, 475–493. doi:10.1016/j.mam.2004.06.002
Rombough, P., and Drader, H. (2009). Hemoglobin enhances oxygen uptake in larval zebrafish (Danio rerio) but only under conditions of extreme hypoxia. J. Exp. Biol. 212, 778–784. doi:10.1242/jeb.026575
Rusyn, I., Peters, J. M., and Cunningham, M. L. (2006). Modes of action and species-specific effects of di-(2-ethylhexyl)phthalate in the liver. Crit. Rev. Toxicol. 36, 459–479. doi:10.1080/10408440600779065
Sadrabadi, F., Alarcan, J., Sprenger, H., Braeuning, A., and Buhrke, T. (2024). Impact of perfluoroalkyl substances (PFAS) and PFAS mixtures on lipid metabolism in differentiated HepaRG cells as a model for human hepatocytes. Archives Toxicol. 98, 507–524. doi:10.1007/s00204-023-03649-3
Sakurai, T., Miyazawa, S., and Hashimoto, T. (1978). Effects of di-(2-ethylhexyl)phthalate administration on carbohydrate and fatty acid metabolism in rat liver. J. Biochem. 83, 313–320. doi:10.1093/oxfordjournals.jbchem.a131906
Salgado-Freiría, R., López-Doval, S., and Lafuente, A. (2018). Perfluorooctane sulfonate (PFOS) can alter the hypothalamic–pituitary–adrenal (HPA) axis activity by modifying CRF1 and glucocorticoid receptors. Toxicol. Lett. 295, 1–9. doi:10.1016/j.toxlet.2018.05.025
Salin, K., Auer, S. K., Rey, B., Selman, C., and Metcalfe, N. B. (2015). Variation in the link between oxygen consumption and ATP production, and its relevance for animal performance. Proc. Biol. Sci. 282, 20151028. doi:10.1098/rspb.2015.1028
Sanajou, S., and Şahin, G. (2021). Mechanistic biomarkers in toxicology. Turk J. Pharm. Sci. 18, 376–384. doi:10.4274/tjps.galenos.2020.10270
Sant, K. E., Annunziato, K., Conlin, S., Teicher, G., Chen, P., Venezia, O., et al. (2021). Developmental exposures to perfluorooctanesulfonic acid (PFOS) impact embryonic nutrition, pancreatic morphology, and adiposity in the zebrafish, Danio rerio. Environ. Pollut. 275, 116644. doi:10.1016/j.envpol.2021.116644
Sarkar, A., Ray, D., Shrivastava, A. N., and Sarker, S. (2006). Molecular Biomarkers: their significance and application in marine pollution monitoring. Ecotoxicology 15, 333–340. doi:10.1007/s10646-006-0069-1
Savinell, J. M., and Palsson, B. O. (1992). Network analysis of intermediary metabolism using linear optimization. I. Development of mathematical formalism. J. Theor. Biol. 154, 421–454. doi:10.1016/s0022-5193(05)80161-4
Schellenberger, J., Que, R., Fleming, R. M., Thiele, I., Orth, J. D., Feist, A. M., et al. (2011). Quantitative prediction of cellular metabolism with constraint-based models: the COBRA Toolbox v2.0. Nat. Protoc. 6, 1290–1307. doi:10.1038/nprot.2011.308
Schilling, C. H., Schuster, S., Palsson, B. O., and Heinrich, R. (1999). Metabolic pathway analysis: basic concepts and scientific applications in the post-genomic era. Biotechnol. Prog. 15, 296–303. doi:10.1021/bp990048k
Sharpe, R. M. (2006). Pathways of endocrine disruption during male sexual differentiation and masculinization. Best. Pract. Res. Clin. Endocrinol. Metab. 20, 91–110. doi:10.1016/j.beem.2005.09.005
Simpson, A. E. (1997). The cytochrome P450 4 (CYP4) family. Gen. Pharmacol. 28, 351–359. doi:10.1016/s0306-3623(96)00246-7
Sunderland, E. M., Hu, X. C., Dassuncao, C., Tokranov, A. K., Wagner, C. C., and Allen, J. G. (2019). A review of the pathways of human exposure to poly- and perfluoroalkyl substances (PFASs) and present understanding of health effects. J. Expo. Sci. Environ. Epidemiol. 29, 131–147. doi:10.1038/s41370-018-0094-1
Swainston, N., Mendes, P., and Kell, D. B. (2013). An analysis of a 'community-driven' reconstruction of the human metabolic network. Metabolomics Official J. Metabolomic Soc. 9, 757–764. doi:10.1007/s11306-013-0564-3
Takacs, M. L., and Abbott, B. D. (2007). Activation of mouse and human peroxisome proliferator-activated receptors (alpha, beta/delta, gamma) by perfluorooctanoic acid and perfluorooctane sulfonate. Toxicol. Sci. 95, 108–117. doi:10.1093/toxsci/kfl135
Tanguay, R. L. (2018). The rise of zebrafish as a model for toxicology. Toxicol. Sci. 163, 3–4. doi:10.1093/toxsci/kfx295
Thiele, I., Sahoo, S., Heinken, A., Hertel, J., Heirendt, L., Aurich, M. K., et al. (2020). Personalized whole-body models integrate metabolism, physiology, and the gut microbiome. Mol. Syst. Biol. 16, e8982. doi:10.15252/msb.20198982
Varma, A., and Palsson, B. O. (1994). Metabolic flux balancing: basic concepts, scientific and practical use. Bio/Technology 12, 994–998. doi:10.1038/nbt1094-994
Vendl, C., Taylor, M. D., Bräunig, J., Gibson, M. J., Hesselson, D., Neely, G. G., et al. (2021). Profiling research on PFAS in wildlife: protocol of a systematic evidence map and bibliometric analysis. Ecol. Solutions Evid. 2, e12106. doi:10.1002/2688-8319.12106
Villeneuve, D. L., and Garcia-Reyero, N. (2011). Vision & strategy: predictive ecotoxicology in the 21st century. Environ. Toxicol. Chem. 30, 1–8. doi:10.1002/etc.396
Wang, H., Robinson, J. L., Kocabas, P., Gustafsson, J., Anton, M., Cholley, P. E., et al. (2021). Genome-scale metabolic network reconstruction of model animals as a platform for translational research. Proc. Natl. Acad. Sci. U. S. A. 118, e2102344118. doi:10.1073/pnas.2102344118
Wang, Y.-M., Ong, S. S., Chai, S. C., and Chen, T. (2012). Role of CAR and PXR in xenobiotic sensing and metabolism. Expert Opin. Drug Metabolism Toxicol. 8, 803–817. doi:10.1517/17425255.2012.685237
Wang, Z., DeWitt, J. C., Higgins, C. P., and Cousins, I. T. (2017). A never-ending story of per- and polyfluoroalkyl substances (PFASs)? Environ. Sci. Technol. 51, 2508–2518. doi:10.1021/acs.est.6b04806
Waterman, C. L., Currie, R. A., Cottrell, L. A., Dow, J., Wright, J., Waterfield, C. J., et al. (2010). An integrated functional genomic study of acute phenobarbital exposure in the rat. BMC genomics 11, 9–2164. doi:10.1186/1471-2164-11-9
Westerfield, M. (2000). The zebrafish book. A guide for the laboratory use of zebrfaish (Danio rerio). Available at: https://zfin.org/zf_info/zfbook/zfbk.html (accessed on November 1, 2023).
Wolffe, T. A. M., Vidler, J., Halsall, C., Hunt, N., and Whaley, P. (2020). A survey of systematic evidence mapping practice and the case for knowledge graphs in environmental health and toxicology. Toxicol. Sci. 175, 35–49. doi:10.1093/toxsci/kfaa025
Keywords: systems toxicology, stoichiometric model, flux balance analysis, biomarkers, carnitine shuttle
Citation: Nolen RM, Petersen LH, Kaiser K, Quigg A and Hala D (2024) In silico biomarker analysis of the adverse effects of perfluorooctane sulfonate (PFOS) exposure on the metabolic physiology of embryo-larval zebrafish. Front. Syst. Biol. 4:1367562. doi: 10.3389/fsysb.2024.1367562
Received: 08 January 2024; Accepted: 14 March 2024;
Published: 27 March 2024.
Edited by:
Claudio Angione, Teesside University, United KingdomReviewed by:
Anze Zupanic, National Institute of Biology (NIB), SloveniaCopyright © 2024 Nolen, Petersen, Kaiser, Quigg and Hala. This is an open-access article distributed under the terms of the Creative Commons Attribution License (CC BY). The use, distribution or reproduction in other forums is permitted, provided the original author(s) and the copyright owner(s) are credited and that the original publication in this journal is cited, in accordance with accepted academic practice. No use, distribution or reproduction is permitted which does not comply with these terms.
*Correspondence: Rayna M. Nolen, bm9sZW4ucmF5bmFAZ21haWwuY29t
Disclaimer: All claims expressed in this article are solely those of the authors and do not necessarily represent those of their affiliated organizations, or those of the publisher, the editors and the reviewers. Any product that may be evaluated in this article or claim that may be made by its manufacturer is not guaranteed or endorsed by the publisher.
Research integrity at Frontiers
Learn more about the work of our research integrity team to safeguard the quality of each article we publish.