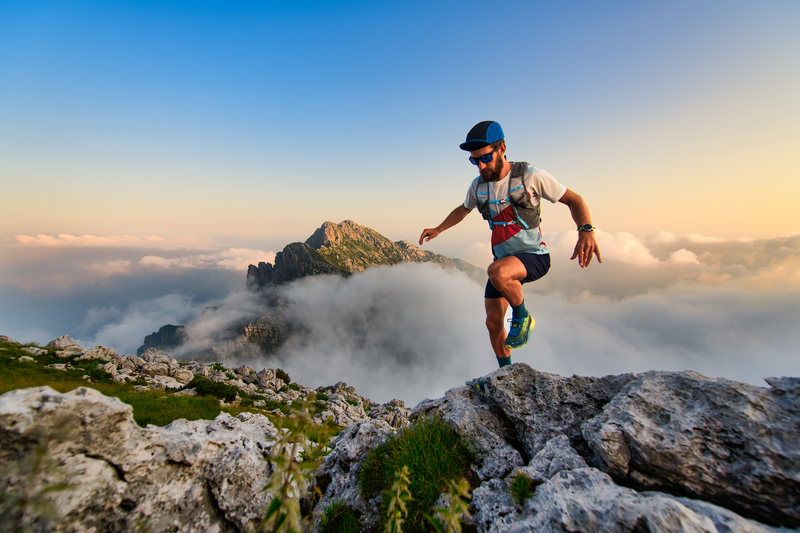
94% of researchers rate our articles as excellent or good
Learn more about the work of our research integrity team to safeguard the quality of each article we publish.
Find out more
ORIGINAL RESEARCH article
Front. Syst. Biol. , 10 January 2023
Sec. Data and Model Integration
Volume 2 - 2022 | https://doi.org/10.3389/fsysb.2022.926618
Introduction: Systemic acute inflammation accompanies and underlies the pathobiology of sepsis but is also central to tissue healing. We demonstrated previously the in vivo feasibility of modulating the key inflammatory mediator tumor necrosis factor-alpha (TNF-α) through the constitutive production and systemic administration of soluble TNF-α receptor (sTNFR) via a biohybrid device.
Methods: We have now created multiple, stably transfected human HepG2 cell line variants expressing the mouse NF-κB/sTNFR. In vitro, these cell lines vary with regard to baseline production of sTNFR, but all have ∼3.5-fold elevations of sTNFR in response to TNF-α.
Results: Both constitutive and TNF-α-inducible sTNFR constructs, seeded into multicompartment, capillary-membrane liver bioreactors could reprogram dynamic networks of systemic inflammation and modulate PaO2, a key physiological outcome, in both endotoxemic and septic rats.
Discussion: Thus, Control of TNF-α may drive a new generation of tunable biohybrid devices for the rational reprogramming of acute inflammation.
Sepsis is a major health problem driven by the host’s inflammatory response to infection (Seymour et al., 2016), which can be replicated in various experimental paradigms including bolus or infusion administration of the pathogen-derived molecular pattern (PAMP) molecule endotoxin, cecal ligation and puncture to generate a polymicrobial infection, or exogenous administration of live bacteria (Parker and Watkins, 2001). Acute inflammation in the setting of sepsis and endotoxemia can elicit a set of inter-related immuno-inflammatory, neural, metabolic, and physiological responses that can lead to severe organ dysfunction and ultimately death (Namas et al., 2012a; Scheff et al., 2013), though with different time courses based on the experimental setting given the dynamics of bolus vs. intravenous infusion of endotoxin vs. the release of pathogen-associated molecular pattern molecules from dividing bacteria (Parker and Watkins, 2001). This inflammatory process occurs at multiple scales and involves the activation of signaling pathways that mobilize inflammatory cells and stimulate the secretion of multiple inflammatory mediators (Namas et al., 2015) rippling across multiple organs as well as the systemic circulation (Zamora et al., 2018; Zamora et al., 2021). The complexity of this inflammatory response has stymied attempts at therapeutic modulation, resulting in a dearth of therapeutic options.
Our group and others have tried to address this complexity through systems and computational biology approaches, and this work has highlighted the importance of multiple positive feedback loops that serve to ramp up inflammation (Vodavotz and An, 2013). We hypothesize that this feed-forward behavior is necessary to respond to perturbations such as infection or injury; however, when overly activated or insufficiently damped, these positive feedback loops can result in harm to the host (as we demonstrated recently for the case of human blunt trauma) (Abboud et al., 2016). We seek to modulate inflammation in a rational fashion; thus, our goal is to attenuate the vicious positive feedback cycle, by allowing the body to re-equilibrate its inflammatory response through an iterative and incremental reduction of pro-inflammatory drivers.
Inflammation is driven and regulated by chemokines, cytokines, free radicals, along with damage-associated molecular pattern (DAMP) molecules, which are context-dependent regulatory molecules (Nathan, 2002). A central aspect of the negative regulation of pro-inflammatory cytokines that exhibit this type of feed-forward behavior involves the production of cytokine antagonists. Examples include: 1) the canonical pro-inflammatory cytokine tumor necrosis factor (TNF-α) and its anti-inflammatory endogenous inhibitor, soluble TNF-α receptor (sTNFR) (Van Zee et al., 1992); 2) interleukin-1 (IL-1β) and IL-1 receptor antagonist (IL-1ra) as well as soluble IL-1 receptor type II (Arend et al., 1994; Symons et al., 1995), 3) transforming growth factor-β1 (TGF-β1) and TGF-β1 latency-associated peptide (LAP) (Khalil, 1999). The basic concept of our rational approach to reprogramming inflammation is to create a biohybrid device that produces negative feedback proportional to the degree of pro-inflammatory stimulus by invoking the production of these natural antagonists. More precisely, in response to a given inflammatory cytokine, the device would produce or release the neutralizing protein. This would in theory reduce inflammation in a manner that echoes the natural mechanisms of inflammation control, and thus may have fewer detrimental side effects. On a practical level, such interventions could be achieved by a blood-perfused, extracorporeal, cell-seeded biohybrid device that is connected via a pump to the venous blood circulation and operated similarly to established clinical plasma perfusion devices.
One of the earliest recognized drivers of systemic acute inflammation in endotoxemia and sepsis is TNF-α (Strieter et al., 1993). However, antagonism of TNF-α using neutralizing antibodies has failed to show benefit in clinical trials of sepsis, a fact that we have attributed to the individual-specific combination of beneficial and detrimental effects of this cytokine (Clermont et al., 2004). Accordingly, as an initial prototype of a device for self-adaptive control of systemic acute inflammation, we generated a human hepatocyte (HepG2) cell line that was stably transfected with the mouse sTNFR gene driven by the constitutive cytomegalovirus promoter. In that initial study, we demonstrated that biohybrid devices seeded with these sTNFR-producing cells could reprogram the pro-inflammatory response of rats subjected to an infusion of Gram-negative bacterial endotoxin, as well as reducing organ damage (Namas et al., 2012b).
The present study extends this prior work, from a system that delivers sTNFR constitutively to one that is designed to adapt to individual TNF-α levels. Our results suggest that sTNFR is a central node in dynamic networks of inflammation in both endotoxemia and bacterial sepsis in rats; that sTNFR can be produced in vitro in a manner that is dependent on exogenous TNF-α; and that provision of sTNFR via a biohybrid device in vivo can reprogram dynamic networks of inflammation and improve sepsis-related pathophysiologic parameters.
All restriction endonucleases were purchased from New England BioLabs (Ipswich, MA). All oligonucleotides were ordered from Invitrogen (Waltham, MA).
To create a system sensitive to stimulation by TNF-α, we focused on signaling via the NF-κB enhancer pathway, which is activated by TNF-α (Van Zee et al., 1992; Siebenlist et al., 1994; Li and Verma, 2002). NF-κB dimers consist of five different gene products, all of which have a conserved region called the Rel homology region. NF-κB dimers are held in the inactive state by a family of inhibitors called I-κB. Receptor signaling leads to activation of a multisubunit I-Bκ kinase (IKK) complex which phosphorylates I-κB on two key serine residues. Phosphorylation of I-κB marks it for degradation by the ubiquitin pathway, so that the NF-κB dimer is liberated to translocate to the nucleus, bind to DNA, and activate transcription (Siebenlist et al., 1994; May and Ghosh, 1998; Li and Verma, 2002).
To generate this molecular system, we utilized lentiviral transfection methodology. The vector for producing sTNFR in response to TNF-α (pLenti6-NFkB-sTNFR) was produced by LR reaction from ViraPower™ Promoterless Lentiviral Gateway® technology (Invitrogen). The integrity of this construct was verified by DNA sequencing. The vector for producing sTNFR constitutively was constructed in order to provide for the constitutive production of mouse sTNFR protein as has been described previously (Namas et al., 2012b).
HepG2 (ATCC, Manassas, VA) cells were cultured in Dulbecco’s modified Eagle’s medium (Lonza, Walkersville, MD) containing 10% fetal bovine serum (Invitrogen), 1% Antibiotic-Antimicotic (Gibco, Waltham, MA), 1% Glutamax™ (Gibco), and 1.5% Hepes (Lonza). Cell culture was performed in flasks/plates treated with 0.1% Gelatin (Sigma, St. Louis, MO) in calcium-free PBS for ∼45 min.
Stably transfected HepG2 clones were established by transfection of cells in 6-well plates with serially diluted lentivirus solution. The virus was packaged using the Lenti-X-HTX packaging system (Clontech, Mountain View, CA) with the pLenti6-NFkB-sTNFR vector, according to the manufacturer’s protocol. Ten colonies were picked manually after 2 weeks of selection in standard media with supplied 1.5 μg/mL Blasticidin (Gibco).
The negative control and sTNFR-producing HepG2 variants were seeded in a scaled-down four-compartment hollow-fiber culture bioreactor (Gerlach, 2006; Schmelzer et al., 2009; Zeilinger et al., 2011) (StemCell Systems, Berlin, Germany). In this class of bioreactors, cells are cultured in the interstitial spaces between the fibers. Culture medium or blood circulate from the lumens of the microfiltration fibers to the cell compartment and back to the fiber lumens, and most proteins produced by the cells seeded in the bioreactor can freely diffuse out, as the microfiltration fibers have a cutoff of 0.2 µm. Medium or blood is pumped through the two-microfiltration fiber bundles in opposing directions (counter-current flow). This complex flow pattern mimics an arterial and venous flow in the liver tissue.
The stably transfected HepG2 hepatocytes (10 million cells) were injected in the cell compartment, allowing the cells to spontaneously reassemble into tissue-like structures in a 3-D perfused cell compartment. The bioreactor (total volume = 0.8 mL) was then integrated into a processor-controlled perfusion device with electronic pressure, flow, temperature, and pH regulation. The production of mouse sTNFR was assessed daily in a bioreactor culture by obtaining medium samples, including after the removal of the bioreactor for in vivo experiments in either endotoxemia or sepsis rats (see below). To assess the viability of the seeded HepG2 cells, and while the bioreactor is connected to the perfusion device, daily samples were taken from the culture media for the measurement of pH, lactate dehydrogenase, lactate, albumin, PaO2, PaCO2, HCO3, and glucose.
The study was approved by the University of Pittsburgh Institutional Animal Care and Use Committee and conforms to the National Institutes of Health (NIH) guidelines for the care and use of laboratory animals. Adult male Sprague-Dawley rats (24–28 weeks old, 430–480 g body weight from Harlan Laboratories, Madison, WI) were anesthetized with pentobarbital sodium (50 mg/kg intraperitoneally). Both the femoral artery and the ipsilateral internal jugular vein (IJV) were isolated by dissection and cannulated with 0.97-mm polyethylene 50 tubing, for use in the extracorporeal circuit. All the tubing in the extracorporeal circulation was flushed with heparinized saline (5 units/mL).
The rats were then cannulated to a bioreactor seeded with either: 1) HepG2 cells that do not produce sTNFR (Clone nine cells) i.e., negative control bioreactor; 2) HepG2 cells producing mouse sTNFR constitutively (CMV-driven bioreactor); and 3) HepG2 cells that produce sTNFR in a TNF-α-dependent manner (Clone three cells) (see below). The bioreactor was placed over a heating pad during the treatment period to maintain the bioreactor temperature at 37°C. Blood flow through the bioreactor was maintained for 6 h by a peristaltic infusion pump (Instech Laboratories, Inc., Plymouth Meeting, PA) at a rate of 1.5 mL/min. To monitor the mean arterial pressure (MAP), the arterial blood (from the previously cannulated femoral artery) was connected to a blood pressure analyzer (Blood Pressure Analyzer™ 400; Micro-Med, Inc., Louisville, KY). Arterial blood gases, blood electrolytes, hematocrit, hemoglobin concentration, and glucose were analyzed using i-STAT® Handheld blood analyzer (Abbott Point of Care Inc., Princeton, NJ). After 6 h of bioreactor treatment, the extracorporeal circuit was stopped, and the rats were euthanized by administering an overdose of pentobarbital sodium followed by cervical dislocation.
The bioreactor was then disconnected from the rat and flushed with HEPES buffer solution and 1% antibiotic-antimycotic solution to clear blood from the inlet and outlet portals of the medium compartments. The bioreactor was then reintegrated to the perfusion device (see above) for 2–3 days. After 3 days of maintaining the bioreactor with the perfusion device, another extracorporeal circuit was established, and the bioreactor was connected to another rat using the experimental procedures mentioned below.
To induce endotoxemia, 13 μg/kg/h of Gram-negative bacterial LPS solution (6.5 μg/mL LPS in saline, catalog #L2630; Sigma-Aldrich, St. Louis, MD) was infused intravenously (i.v.) through the rats’ internal jugular vein at a rate of 1 mL/h via the peristaltic infusion pump. The i. v. Infusion of LPS was initiated at 0 h (immediately after establishing the extracorporeal circuit with the bioreactor) and continued to 4 h of the bioreactor treatment. At 4 h, the LPS infusion was discontinued while continuing treatment with the bioreactor for an additional 2 h; thus, the total bioreactor treatment time was 6 h. Three groups of experimental animals were studied: 1) control animals (n = 4), i.e., without bioreactor treatment; 2) bioreactor negative control animals (n = 4), i.e., rats treated with bioreactor seeded with Clone nine cells; and 3) animals (n = 7) treated with a CMV-driven bioreactor. All animals were subjected to the same surgical procedure mentioned above.
To induce peritonitis, an Escherichia coli (E. coli)-impregnated fibrin clot was formed from a bacterial culture of a single isolated colony of E. coli bacteria (strain ATCC 25922; American Type Culture Collection, Manassas, VA). The culture was grown to a specified optical density using a spectrophotometer (DU 530 UV/VIS; Beckman Coulter, Brea, CA, United States) equivalent to a concentration of 1.5 × 108 to 2 × 108 colony-forming units. After the addition of Fibrinogen (1%) and Thrombin (15u), the clot was placed in the peritoneum via laparotomy, as per our previously published work with extracorporeal hemoadsorption in rat Gram-negative sepsis (Namas et al., 2012c). After closing the abdomen, a topical anesthetic was applied over the surgical wound, and the rats were returned to their cages and allowed food and water ad libitum. Twenty-four hours later, the rats were re-anesthetized, cannulated, and treated with either CMV-driven bioreactor seeded with HepG2 cells that produce sTNFR constitutively (n = 4) or bioreactor seeded with HepG2 cells that produce sTNFR in a TNF-α-dependent manner (referred to hereafter as “Clone three bioreactor”; n = 3), for 6 h, i.e., a total of 30 h of experimental sepsis. For the control animals, a separate group of rats (n = 8) were subjected to the same surgical procedure mentioned above but without treatment.
Blood (0.4 mL) was withdrawn from the IJV line at time 0 (before either starting the LPS i. v. Infusion and 24 h after implantation of the E. coli–impregnated fibrin clot) and then hourly up to 6 h. Blood samples were centrifuged, and plasma aliquots were stored at −80°C for subsequent analysis of inflammatory mediators. Plasma cytokines/chemokines were measured using Luminex™ technology (Millipore, Billerica, MA). The assays included granulocyte/macrophage colony-stimulating factor (GM/CSF), keratinocyte-derived chemokine (GRO/KC, CINC-1, CXCL-1), interferon (IFN)-γ, interleukin (IL)-1α, IL-1β, IL-2, IL-4, IL-5, IL-6, IL-10, IL-12p70, IL-18, monocyte chemoattractant protein-1 (MCP-1/CCL2), and TNF-α. Plasma NO2−/NO3− was measured by the nitrate reductase method using a commercially available kit (Cayman Chemical, Ann Arbor, MI). Plasma sTNFR (as well as sTNFR collected during the bioreactor culture) was assessed using a kit from R&D Systems (Minneapolis, MN). Arterial blood gases, blood electrolytes, hematocrit, hemoglobin concentration, and glucose were analyzed using i-STAT® Handheld blood analyzer (Abbott Point of Care Inc., Princeton, NJ).
All data are expressed as mean ± SEM. Statistical analysis was performed by One-way analysis of variance (ANOVA) or Two-way ANOVA, as appropriate, followed by the Tukey post hoc test using SigmaStat software (Systat Software, San Jose, CA), with p < 0.05 considered significant.
Principal Component Analysis (PCA) was carried out to identify the subsets of mediators (in the form of orthogonal normalized linear combinations of the original mediator variables, called principal components) that are most strongly correlated with the inflammatory response in each experimental group, and that thereby might be considered principal characteristics of each response. We have previously utilized PCA in this fashion in the setting of mouse trauma/hemorrhage (Mi et al., 2011), human pediatric acute liver failure (Azhar et al., 2013), and human blunt trauma (Namas et al., 2016a). PCA is a non-parametric statistical method of reducing a multidimensional dataset to a few principal components. These are the components that account for the most variability in the dataset. The underling hypothesis is that a mediator that varies extensively during a specific process is important to that process. This method allows us to identify the mediators that account for the most change, or variance, in the dataset. The limitation is that some principal components may lack biological relevance (Janes and Yaffe, 2006). In the present study, we utilized a variant of PCA in which data were segmented into defined time intervals (three time intervals), as we have described recently (Zamora et al., 2018). To perform this analysis, the raw data from all time points (0–6 h) were first normalized for each inflammatory mediator (i.e., in a given inflammatory mediator, a given value from each experimental rat divided by the Euclidean norm of this experimental rat’s values from its available time points). In this way, any artifactual effects on variance due to the different ranges of concentration observed for different experimental rats and inflammatory mediators were eliminated. Next, these normalized data were segmented into defined time intervals and PCA was performed on each segment of the normalized data. Only components that captured at least 70% of the total variance in the data were considered. From these leading principal components, the coefficient (weight) associated with each mediator was multiplied by the eigenvalue associated with that principal component. This product represented the contribution of a given inflammatory mediator to the variance accounted for in that principal component. The overall score given to each mediator is the sum of its scores in each component. This gives a measure of a mediator’s contribution to the overall variance of the system. The mediators with the largest scores are the ones who contributed most to the variance of the process being studied. More specifically, the overall PCA score was calculated in the following way:
Dynamic Bayesian network (DyBN) inference was carried out to define the most likely single network structure that best characterizes the dynamic interactions among systemic inflammatory mediators across all time points, in the process suggesting likely feedback structures that define central nodes. DyBNs depict the entire time progression of a given network as a single graph to outline the patterns and relationships/feedbacks prevalent throughout the entire experimental course. Given time-series data, DyBN inference provides a way of inferring causal relationships among variables (e.g., inflammatory mediators) based on probabilistic measure. Unlike standard correlative approaches, DyBNs consider the joint distribution of the entire dataset when making inferences about the dependencies between variables or nodes in the network. The networks might also suggest possible feedbacks governing mechanisms by which progression of the inflammatory response differs within a given experimental group. The values of each node are assumed to be distributed according to a chosen model (e.g., Gaussian) and the relationships among nodes are defined by the structure of the directed network and the corresponding conditional probability distributions of the interacting nodes. Network structure is inferred by a sampling technique that iteratively proposes candidate structures and evaluates them based on how well they fit the observed data using a specified scoring criterion, until reaching convergence on a network structure with the highest score. The algorithm uses an inhomogeneous dynamic changepoint model, with a Bayesian Gaussian with score equivalence (BGe) scoring criterion. In this analysis, time courses of unprocessed inflammatory mediator measurements from each rat were used as input for a DyBN inference algorithm, implemented in Matlab® essentially as described previously for gene array data (Grzegorczyk and Husmeier, 2011) and modified by our group for the study of systemic acute inflammation (Azhar et al., 2013; Emr et al., 2014). Inflammatory mediators are shown as nodes, and the arrows connecting them suggest an influence of one mediator on the one(s) to which it is connected. The arrows do not distinguish positive from negative influences of one mediator on another. Semi-circular arrows suggest either positive or negative feedback of a given mediator on itself. The thickness of each edge denotes the relative algorithmic confidence in a given interaction between nodes.
Dynamic network analysis (DyNA) was carried out to define, in a granular fashion, the dynamic changes of the central inflammatory network nodes as a function of both time and experimental group. The mathematical formulation of this method is essentially to calculate the correlation among variables (inflammatory mediators) by which we can examine their dependence and visually observe the associations among the inflammatory mediators. The main difference between DyNA and DyBN is that DyNA allows for granular temporal resolution of networks over distinct time intervals, while DyBN helps suggest feedback structures. Using inflammatory mediator measurements, networks were created over three consecutive time intervals (0–2, 2–4, 4–6 h or 0–1, 1–3, 3–6 h, based on the experimental setting) using Matlab® software, as described previously (Ziraldo et al., 2013; Namas et al., 2016b). Network edges/connections, defined as the trajectories of serum inflammatory mediators that move in parallel (same or opposite direction), were created if the absolute value of the Pearson correlation coefficient between any two nodes (inflammatory mediators) at the same time interval was greater or equal to a threshold of 0.7. Connections with black color indicate Pearson correlation coefficient greater or equal to 0.7, whereas the red color connections indicate Pearson correlation coefficient less than or equal to -0.7. The network complexity for each time interval was calculated using the following formula: sum (N1 + N2 + ... + Nn)/(n-1), where N represents the number of connections for each mediator and n is the total number of mediators analyzed. The total number of connections represents the sum of the number of connections across all time intervals for all experimental rats in a given group.
We have reported previously on the generation of a HepG2 variant that produces sTNFR constitutively under the control of the CMV promoter (Namas et al., 2012b). The central goals of the present study were 1) to compare the effects of constitutively delivered sTNFR to those of sTNFR produced in response to TNF-α, and 2) to compare the impact of these two approaches on endotoxemia vs. true Gram-negative bacterial peritonitis/sepsis. To create variants of HepG2 that could respond to TNF-α by producing sTNFR, we utilized a lentiviral delivery system. Based on the Invitrogen ViraPower™ Promotorless Lentiviral Gateway® system, we created the vector pLenti6-NFkB-sTNFR as described in the Materials and Methods. Using this vector, we established 10 stably transfected clones of HepG2 cells. All of these clones produced mouse sTNFR to various degrees at baseline, ranging from 0 to 376 pg/mL. Upon stimulation with mouse TNF-α, these HepG2 clones produced 0–1,200 pg/mL mouse sTNFR (Supplementary Figure S1). From this group of HepG2 variants, we chose three with different properties (clones 2, 3, and 9) for further characterization. Clone nine exhibited no production of sTNFR, either with or without stimulation with mouse TNF-α. Clones two and three exhibited different degrees of sTNFR production in response to stimulation, but with similar fold induction (Supplementary Figure S2).
A major goal of this study was to test the constitutive (CMV-driven) sTNFR HepG2 clone, as well as Clones three and nine in experimental paradigms of endotoxemia and Gram-negative sepsis. To achieve this goal, it was first necessary to define the dynamic properties of inflammation in these two experimental paradigms in the absence of any intervention. We used one-way ANOVA to define inflammatory mediators that were altered significantly over time in each experimental paradigm, Time-Interval Principal Component Analysis (TI-PCA) (Zamora et al., 2018) to define the principal characteristics/drivers of LPS infusion vs. true Gram-negative sepsis, and DyNA to define the progression of systemic inflammatory networks in these two related, but distinct (Parker and Watkins, 2001), inflammatory challenges. The results of these studies are summarized in Table 1 and detailed below.
TABLE 1. Summary of key results inferred from the six experimental conditions. One--way ANOVA was used to define the inflammatory mediators that were altered significantly over time. Principal component analysis (PCA) was used to define principal characteristics of the systemic inflammatory response in each experimental condition. Dynamic network analysis (DyNA) was used to visualize the dynamic progression of systemic inflammatory networks in each experimental group. Dynamic Bayesian network (DyBN) inference was used to suggest the most likely feedback structures that could define central nodes.
Following stimulus with LPS, the following inflammatory mediators were altered significantly: TNF-α, IL-6, MCP-1, IL-5, IL-2, IL-10, IL-1β, IL-18, IFN-γ, and GRO/KC (p < 0.05 by one-way ANOVA) (Table 1; Supplementary Figure S3). These results are in agreement with well-known pathways of inflammation in endotoxemia, including NF-κB (e.g. TNF-α, IL-6), Th1 (IFN-γ), Th2 (IL-5, IL-10), and the NLRP3 inflammasome (leading to activation of IL-1β and IL-18).
We next sought to define the principal characteristics/drivers of LPS-induced inflammation using TI-PCA. In this implementation of PCA, the data were segmented into defined time intervals and the overall score given to each mediator is the sum of its scores in each principal component, depicted as a stacked bar graph. This gives a measure of a given inflammatory mediator’s contribution to the overall variance of the system. The mediators with the largest scores are the ones which contribute most to the variance of the process being studied. This analysis suggested that the circulating inflammatory response during the first hour in the control animals (LPS infusion without treatment) was characterized primarily by GRO/KC, IFN-γ, IL-1α, TNF-α, and IL-10 (Table 1; Supplementary Figure S4A). Over the 1–3 h time interval, the inflammatory response was characterized primarily by TNF-α, MCP-1, IL-18, IL-5, and IL-1β. Over the 3–6 h interval, IFN-γ, TNF-α, IL-2, IL-6, and GM-CSF were the primary characteristics of the inflammatory response to LPS (Table 1; Supplementary Figure S4A). Thus, LPS-induced inflammation was characterized by two distinct components during the 0–1 h time frame, decreasing in complexity to one component from 1 to 3 h, and then returning to two components in the 3–6 h time frame (Table 1; Supplementary Figure S4A). Also, confirming the results of one-way ANOVA, PCA suggested a role for activation of NF-κB (TNF-α, IL-6), Th1 (IFN-γ), Th2 (IL-5), and the NLRP3 inflammasome (IL-1β and IL-18).
We further examined the dynamics of inflammation induced by LPS infusion by defining dynamic networks of inflammation (Table 1; Supplementary Figure S4B). Dynamic Network Analysis (DyNA), a network inference algorithm that we developed previously (Mi et al., 2011; Ziraldo et al., 2013; Abboud et al., 2016; Namas et al., 2016b), allows us to define the progression over time of correlated (black edges) or anti-correlated (red edges) inflammatory mediators. This analysis suggested that an initially high network complexity following LPS infusion, which decreased in complexity through 6 h. Furthermore, anti-correlated edges in the 3–6 h time frame suggested the initiation of resolution of inflammation. Taken together with the PCA results, this analysis suggests a rapid ramp-up of inflammation induced by LPS, with a follow-on resolution phase, in agreement with the known dynamics of inflammation in endotoxemia (Parker and Watkins, 2001).
We further sought to define potential feedback structures in dynamic networks of inflammation, and for this purpose we utilized a Dynamic Bayesian Network (DyBN) inference algorithm (Grzegorczyk and Husmeier, 2011). In endotoxemic rats, this analysis suggested that central nodes (i.e., nodes exhibiting self-feedback and feedback onto other nodes) consisted of the chemokines MCP-1 and GRO/KC along with the cytokines IL-6 and IL-10 (Table 1; Supplementary Figure S4C). These central nodes, known to participate in the inflammatory response to LPS, were upstream of multiple inflammatory mediators, suggestive of activation of the NLRP3 inflammasome (IL-1β and IL-18) as well as Th1 (IFN-γ) and Th2 (IL-4) pathways.
We next compared the response to LPS with that observed in true Gram-negative sepsis. One-way ANOVA suggested that TNF-α, IL-6, MCP-1, and GM-CSF changed significantly (p < 0.05) over the duration of the study (Table 1; Supplementary Figure S5). During the first hour in the control E. coli fibrin clot peritonitis animals without treatment, TI-PCA suggested similar principal inflammatory characteristics to those observed in the control LPS animals: TNF-α, IL-6, GRO/KC, IL-10, and IFN-γ (Table 1; Supplementary Figure S6A). Subsequently, TI-PCA suggested that IL-6, TNF-α, MCP-1, and IL-10 were principal characteristics during the 1–3 h interval. Over the 3–6 h of interval of experimental sepsis, the principal, characteristic mediators were TNF-α, IL-6, and MCP-1 (Table 1; Supplementary Figure S6A). Taken together, these analyses again suggest a role for activation of NF-κB and NLRP3 pathways in the setting of Gram-negative sepsis.
In contrast to dynamic networks in endotoxemia, the DyNA-inferred networks after established sepsis were all quite sparse (Table 1; Supplementary Figure S6B); this likely reflects the fact that the networks we delineated in endotoxemia were defined during the ramp-up and initial resolution phases, while our Gram-negative sepsis model involved an initial period of 18 h of infection. Based on DyBN, GRO/KC and IL-6 were central nodes in this sepsis model, largely similar to the inferred networks in endotoxemia (Table 1; Supplementary Figure S6C). Likewise, downstream pathways (NLRP3 inflammasome, Th1, Th2) inferred in septic rats were similar to those in endotoxemic rats, with the addition of the neutrophil-activating cytokine GM-CSF.
We have demonstrated previously the ability to reprogram the acute inflammatory responses of endotoxemic rats by cannulating them to bioreactors containing HepG2 cells stably modified to produce sTNFR constitutively (Namas et al., 2012b). We also showed that systemic sTNFR was elevated and several inflammatory mediators were suppressed relative to cannulation with bioreactors containing the inactive Clone nine cells (Namas et al., 2012b). To gain further insight into how these biohybrid devices reprogram inflammation, we sought to characterize the impact of CMV-driven (constitutive) sTNFR vs. TNF-α-dependent sTNFR vs. control treatment on dynamic networks of systemic inflammation in the setting of experimental sepsis. Notably, sTNFR was measured in these animals in order to be able to define the inflammatory responses of animals cannulated to sTNFR-producing bioreactors.
We initially sought to define the impact of a control bioreactor (seeded with Clone nine cells). One-way ANOVA identified TNF-α, IL-6, MCP-1, IL-10, IL-1β (as has been reported previously (Namas et al., 2012b)), IL-2, IL-5, IL-18, IFN-γ, and GRO/KC as being altered significantly (p < 0.05) in endotoxemic rats treated with the Clone nine bioreactor (which does not produce sTNFR) (Table 1; Supplementary Figure S7). Based on TI-PCA, the inflammatory response in this experimental group was characterized by TNF-α, IL-18, GRO/KC, sTNFR, and IL-5 during the initial 2 h time interval (Figure 1A). The principal mediators, however, changed subsequently to include IFN-γ, IL-6, GM-CSF, IL-10, and TNF-α during the 2–4 h interval and to IL-10, IFN-γ, IL-5, IL-2, and GM-CSF during the 4–6 h interval (Figure 1A). These findings suggest the expected interplay between TNF-α and sTNFR.
FIGURE 1. Impact of the negative control bioreactor seeded with Clone nine HepG2 cells on the inflammatory response in endotoxemic rats. Rats (n = 4) subjected to LPS infusion for 4 h were cannulated for a further 2 h (total of 6 h experimental time) to bioreactor that does not produce sTNFR. (A) Principal component analysis (PCA) was carried out over three time intervals (0–2, 2–4, and 4–6 h) using time course data of circulating inflammatory mediators. PCA pointed to a differential role of inflammatory mediators that characterizes the inflammatory response over the three time intervals. (B) Dynamic network analysis (DyNA) of circulating inflammatory mediators was performed during three time frames, 0–2, 2–4, and 4–6 h, as described in Materials and Methods. Inflammatory mediators encircled with a red circle represent mediators (TNF--α, IL--6, MCP--1, IL--2, IL--5, IL--1α, IL--1β, IL--10, IL--18, IFN--γ, GM--CSF, and GRO/KC) that were statistically significantly altered by One--way ANOVA (p < 0.05) with at least one connection to another mediator. (C) Dynamic Bayesian Network (DyBN) inference of the inflammatory mediators over the 6 h study period suggested that central nodes consisted of the GRO/KC, MCP--1, IL--6, and sTNFR. The thickness of each arrow denotes the relative algorithmic confidence in a given interaction between nodes.
Dynamic inflammation networks inferred by DyNA suggested an increasing complexity over the duration of the experiment (Figure 1B). Notably, DyNA revealed a highly connected network during the initial 2 h interval which declined thereafter, consisting of sparsely connected nodes that included sTNFR, IL-18, IL-1α, IL-12p70, and IL-4 by 6 h (Figure 1B). DyBN inference (Figure 1C) pointed to GRO/KC, MCP-1, IL-6, and sTNFR as central nodes, with key cytokines including TNF-α being connected to sTNFR. As expected, this latter finding suggests that sTNFR is involved in the regulation of TNF-α. Taken together, these results suggest an overall similarity to the inflammatory responses of rats undergoing endotoxemia in the absence of cannulation to a bioreactor.
We next examined the impact on inflammation of constitutively delivered sTNFR on the dynamics of systemic inflammation induced by LPS. One-way ANOVA suggested that TNF-α, IL-6, MCP-1, IL-2, IL-5, IL-10, IL-18, IL-1β, IFN-γ, GRO/KC, and sTNFR changed significantly (p < 0.05) over the duration of the study (Table 1; Supplementary Figure S8). In contrast to the LPS control animals (Supplementary Figure S4A) and endotoxemic animals cannulated to negative control bioreactors (Clone 9; Figure 1A), TI-PCA of systemic inflammatory mediators in endotoxemic rats treated with the CMV-driven sTNFR bioreactor suggested that the inflammatory response over the initial 2 h is primarily characterized/driven by IL-10, TNF-α, IL-1α, sTNFR, and GRO/KC (Figure 2A). Subsequently, and over 2–4 h of treatment, TI-PCA pointed to IL-1β, IL-6, IL-18, MCP-1, and IL-1α as being the most characteristic of this group. With the discontinuation of LPS infusion at 4 h and continuation of treatment for an additional 2 h, PCA revealed that the inflammatory response was characterized by IL-1α, IL-6, IFN-γ, IL-1β, and IL-12p70 at 6 h (Figure 2A).
FIGURE 2. Early role of constitutively delivered sTNFR on the dynamics of systemic inflammation in endotoxemic rats. Rats (n = 7) subjected to LPS infusion for 4 h were cannulated for a further 2 h (total of 6 h experimental time) to bioreactor producing sTNFR constitutively (CMV--driven). (A) Principal component analysis (PCA) was carried out over three time intervals (0–2, 2–4, and 4–6 h) using time course data of circulating inflammatory mediators. PCA pointed to a differential role of inflammatory mediators that characterizes the inflammatory response over the three time intervals. (B) Dynamic network analysis (DyNA) of circulating inflammatory mediators was performed during three time frames, 0–2, 2–4, and 4–6 h, as described in Materials and Methods. Inflammatory mediators encircled with a red circle represent mediators (TNF--α, IL--6, MCP--1, IL--2, IL--5, IL--10, IL--18, IL--1β, IFN--γ, GRO/KC, and sTNFR) that were statistically significantly altered by One--way ANOVA (p < 0.05) with at least one connection to another mediator. (C) Dynamic Bayesian Network (DyBN) inference of the inflammatory mediators over the 6 h study period suggested that central nodes consisted of the GRO/KC, MCP--1, and sTNFR. The thickness of each arrow denotes the relative algorithmic confidence in a given interaction between nodes.
DyNA-inferred inflammatory networks in endotoxemic animals cannulated to CMV-sTNFR bioreactors increased in complexity over the 6 h duration of the experiment (Figure 2B), in contrast to the decreasing network complexity seen in endotoxemic rats cannulated to the negative control (Clone 9) bioreactor (Figure 1B). These networks consisted of TNF-α, GRO/KC, IL-18, IL-5, IL-12p70, IL-10, IL-6, IL-1β, and MCP-1. These networks evolved progressively into much larger, highly connected networks by 6 h (Figure 2B). In this experimental group, DyBN identified a primary network driven by three central feedback nodes, GRO/KC, MCP-1, and sTNFR, with the later having a direct effect on the downstream production of IL-18, IFN-γ, TNF-α, IL-2, IL-4, IL-12p70, IL-5, IL-10, and IL-1α (Figure 2C). Overall, these results suggest an early role for sTNFR in modulating TNF-α, with later responses involving the activation of the NLRP3 inflammasome.
A key question we sought to address was whether inflammation reprogramming that adapted to the degree of inflammation in a given animal resulted in better outcomes in true sepsis as compared to constant delivery of an anti-inflammatory molecule. To that end, we compared the performance of the CMV-sTNFR bioreactor vs. that of the TNF-α-dependent bioreactor (seeded with Clone three cells) in our experimental paradigm of Gram-negative sepsis in rats. One-way ANOVA identified sTNFR and IL-12p70 in E. coli fibrin clot peritonitis rats treated with the CMV-driven bioreactor (Table 1; Supplementary Figure S9), and IL-2 in rats treated with Clone three bioreactor (Table 1; Supplementary Figure S10), respectively, as being altered significantly (p < 0.05). Group-time interactions of plasma inflammatory mediator levels determined by Two-way ANOVA suggested that IL-5 and IL-18 were statistically significantly elevated (p = 0.013 and p = 0.006, respectively) in rats treated with Clone three bioreactor vs. rats treated with the CMV-driven bioreactor (Table 1; Supplementary Figures S11A, B). Rats treated with Clone three bioreactor exhibited statistically significantly lower TNF-α levels (p < 0.001) when compared to rats treated with the CMV-driven bioreactor (Supplementary Figure S11C), as might be expected from the statistically elevated levels of sTNFR in rats treated with the CMV-driven bioreactor over the duration of the study when compared to rats treated with Clone three bioreactor (p < 0.001; Supplementary Figure S11D).
TI-PCA of the data from E. coli fibrin clot peritonitis rats treated with the CMV-driven bioreactor suggested that IL-18, sTNFR, IL-12p70, MCP-1, and IL-10 characterize the inflammatory response over the initial 2 h of treatment (Figure 3A), and this remained relatively unchanged during the 2–4 h time interval (Figure 3A). By 6 h, i.e., 30 h of experimental sepsis, TI-PCA suggested that the inflammatory response is primarily driven by IL-6, IL-1α, IL-1β, IL-18, and IL-10. During this experimental period, DyNA networks remained largely constant in complexity.
FIGURE 3. Constitutively delivered sTNFR reprograms systemic inflammation induced by E. coli--impregnated fibrin clot peritonitis (Gram--negative sepsis). Rats (n = 4) subjected to E. coli fibrin peritonitis for 24 h (time = 0 h) were cannulated for a further 6 h (total of 30 h of experimental sepsis) to bioreactor producing sTNFR constitutively (CMV--driven). (A) Principal component analysis (PCA) was carried out over three time intervals (0–2, 2–4, and 4–6 h) using time course data of circulating inflammatory mediators. PCA pointed to a differential role of inflammatory mediators that characterizes the inflammatory response over the three time intervals. (B) Dynamic network analysis (DyNA) of circulating inflammatory mediators was performed during three time frames, 0–2, 2–4, and 4–6 h, as described in Materials and Methods. Inflammatory mediators encircled with a red circle represent mediators (sTNFR and IL--12p70) that were statistically significantly altered by One--way ANOVA (p < 0.05) with at least one connection to another mediator. (C) Dynamic Bayesian Network (DyBN) inference of the inflammatory mediators over the 6 h study period suggested that central nodes consisted of the MCP--1, GRO/KC, and sTNFR. The thickness of each arrow denotes the relative algorithmic confidence in a given interaction between nodes.
Dynamic Network Analysis of rats subjected to E. coli fibrin clot peritonitis and cannulated to CMV-driven bioreactors revealed a sparsely connected network during the initial 2 h consisting of MCP-1, TNF-α, IL-6, GRO/KC, and IL-10 (Figure 3B). These connections persisted over time, with the addition of IL-18 and IL-1β to the network by 4–6 h of bioreactor treatment. Interestingly, two nodes, namely IL-2 and IL-1α, exhibited a negatively bidirectional correlation at 6 h (indicated by red edges in Figure 3B). Based on DyBN, MCP-1, GRO/KC, and sTNFR were retained as the central feedback nodes in rats with E. coli peritonitis treated with CMV-driven bioreactor, with IL-1β, IL-18, NO2−/NO3−, IL-10, and IL-2 (Figure 3C). These results suggest a key role for sTNFR in reprogramming inflammation induced in the course of Gram-negative sepsis. In addition, these data (especially the inferred role for IL-1β and IL-18) again implicate an early upregulation of NLRP3 inflammasome.
TI-PCA of the E. coli fibrin clot peritonitis rats treated with Clone three bioreactor suggested that IL-12p70, IL-18, GRO/KC, IL-5, and IL-1β were most characteristic during the initial 2 h of treatment (Figure 4A). As the study progressed in time, TI-PCA pointed to IL-6, IL-5, IL-18, IL-1β, and GRO/KC during the 2–4 h interval and to IL-6, IL-5, IL-1β, IL-10, and IL-12p70 during the 4–6 h interval. This analysis again reinforced the hypothesis for a key role for the NLRP3 inflammasome.
FIGURE. 4. Role of TNF--α--dependent delivery of sTNFR in reprograming systemic inflammation induced by E. coli--impregnated fibrin clot peritonitis (Gram--negative sepsis). Rats (n = 3) subjected to E. coli fibrin peritonitis for 24 h (time = 0 h) were cannulated for a further 6 h (total of 30 h of experimental sepsis) to bioreactor producing sTNFR NF--κB--dependent manner (seeded with Clone three cells). (A) Principal component analysis (PCA) was carried out over three time intervals (0–2, 2–4, and 4–6 h) using time course data of circulating inflammatory mediators. PCA pointed to a differential role of inflammatory mediators that characterizes the inflammatory response over the three time intervals. (B) Dynamic network analysis (DyNA) of circulating inflammatory mediators was performed during three time frames, 0–2, 2–4, and 4–6 h, as described in Materials and Methods. Inflammatory mediators encircled with a red circle represent mediators (IL--2) that were statistically significantly altered by One--way ANOVA (p < 0.05) with at least one connection to another mediator. (C) Dynamic Bayesian Network (DyBN) inference of the inflammatory mediators over the 6 h study period suggested that central nodes consisted of the MCP--1 and GRO/KC. The thickness of each arrow denotes the relative algorithmic confidence in a given interaction between nodes.
DyNA inference of rats with E. coli fibrin clot peritonitis treated with Clone three bioreactor suggested the activation of inflammatory networks with a high degree of network connectivity over the initial 2 h, consisting of sTNFR, MCP-1, TNF-α, IL-1β, IL-6, GRO/KC, IL-10, IL-12p70, IL-2, and NO2−/NO3− (Figure 4B). These network connections persisted over the 2–4 h interval (Figure 4B). During the 4–6 h interval, multiple negatively correlated connections were observed, notably between IL-12p70, IL-18, IL-2, IL-10, GRO/KC, IL-6, IL-1β, TNF-α, and MCP-1 (Figure 4B). DyBN inference suggested a primary network driven by MCP-1 and GRO/KC, which was inferred to affect the downstream production of IL-5, IL-1β, IL-12p70, IL-10, IL-18, IL-2, and sTNFR (Figure 4C).
We finally sought to determine if these differential principal characteristics and dynamic networks were associated with different physiological responses. To do so, physiological and biochemical parameters (see Materials and Methods), were measured hourly over the 6-h time period in both experimental groups. Eichacker et al had shown that animals subjected to TNF- α infusion exhibited a significant decrease in arterial partial pressure of oxygen (PaO2) as a result of acute pulmonary dysfunction similar to that seen in human septic shock (Eichacker et al., 1991). In addition, Windsor et al had shown that PaO2 is reduced in septic swine, and that neutralizing TNF-α raises PaO2 (Windsor et al., 1993). Notably, PaO2 was elevated significantly (p < 0.05 by Two-Way ANOVA) in septic rats cannulated to the Clone three bioreactor compared to the rats cannulated to the CMV-driven bioreactor and control rats (Figure 5), suggesting a physiologic benefit of adaptive sTNFR administration over constitutive sTNFR administration. However, PaO2 was significantly higher in rats treated with either the Clone three or CMV-driven bioreactors when compared to the control septic rats without treatment, suggesting the presence of other factors in addition to sTNFR that might impact PaO2.
FIGURE 5. Effects of constitutively delivered sTNFR and TNF--α--dependent delivery bioreactors on arterial partial pressure of oxygen (PaO2) in the context of fibrin clot peritonitis (Gram--negative sepsis). Rats subjected to E. coli fibrin peritonitis for 24 h (time = 0 h) were cannulated for a further 6 h (total of 30 h of experimental sepsis) to bioreactors producing sTNFR either constitutively (CMV--driven: n = 4) or in a NF--κB--dependent manner (Clone 3: n = 3). Control animals (Gram--negative sepsis without treatment: n = 8). PaO2 was measured using the ISTAT system. p < 0.05 by Two--way ANOVA.
Managing acute inflammation remains a key translational challenge in multiple diseases, since inflammation can be harmful and yet is also necessary for proper healing and regeneration (Medzhitov, 2008; Eming et al., 2017). Nowhere is the challenge of balancing the beneficial and detrimental effects of acute inflammation more relevant than in the setting of sepsis and critical illness (Namas et al., 2012a). Gram-negative infection can result in sepsis and endotoxemia, and can be replicated to various degrees in rodent models in which TNF-α is a crucial early mediator (Parker and Watkins, 2001). In prior work, we demonstrated that we could modify key inflammatory and physiological parameters of endotoxin-induced systemic acute inflammation through the constitutive delivery of sTNFR via a biohybrid device (Namas et al., 2012b). Though that study served as a proof of principle, it lacked several key elements. This initial biohybrid device could not produce sTNFR in a manner proportional to circulating levels of TNF-α; rather, it functioned in an always-on fashion. This was a limitation since we hypothesize that an optimal treatment would allow sufficient bioactive TNF-α to be available to drive inflammatory responses that help kill invading bacteria as well as driving the early tissue healing responses necessary for recovery from sepsis, while blocking self-sustaining TNF-α production that would damage tissues. A second limitation of our original study was the fact that the device was tested experimentally in a model of inflammation induced by endotoxin (LPS), rather than in a true bacterial sepsis model. This is a limitation since, as mentioned above, TNF-α is needed to drive antibacterial innate immune mechanisms in the setting of infection as well as tissue-damaging inflammation but drives only the latter in endotoxemia. Finally, our initial study lacked a broad characterization of the dynamic inflammatory milieu in both endotoxemia and bacterial sepsis models, and the impact thereon of the constitutive and self-adaptive production of sTNFR.
In the present study, we focused on addressing these issues by modulating the production of sTNFR in a manner dependent on the production of TNF-α. To drive TNF-α-dependent activation of sTNFR, we employed the NF-κB promoter/enhancer element (Leitman et al., 1991; Lavrovsky et al., 1994). We created a DNA construct carrying the mouse sTNFR gene under the control of a gene promoter-enhancer element (consisting of multiple repeats of the mouse NF-κB TNF-α-responsive enhancer followed by a minimal thymidine kinase promoter). We created stably transformed variants of the HepG2 cell line using a lentiviral system, because lentiviruses could infect both dividing and non-dividing cells (unlike retroviral systems) (Naldini et al., 1996; Dull et al., 1998). This approach resulted in a roughly 3-fold stimulation of sTNFR in vitro in response to exogenous TNF-α. In vivo, this circuit could reprogram the systemic inflammatory response as assessed both by standard statistical analyses of altered mediators over time, as well as by PCA and dynamic network inference.
Our computational analyses point to both similarities and differences in experimental endotoxemia and sepsis, as well as differential impact of sTNFR delivered constitutively vs. in a TNF-α-dependent manner. Our results support numerous prior studies showing key roles for TNF-α and chemokines such as MCP-1, along with activation of the NLRP3 inflammasome and subsequent production of bioactive IL-1β and IL-18 in endotoxemia. Interestingly, established Gram-negative sepsis is characterized by the production of TNF-α and MCP-1, but not inflammasome activation, but this may be a limitation of our study in which did not, for technical reasons, obtain samples earlier than 18 h after implantation of a fibrin clot containing E. coli.
Treatment with sTNFR-producing bioreactors reprograms these dynamic inflammation programs. A key aspect of this reprogramming appears to lie in the emergence of the NLRP3 inflammasome pathway following either constitutive or TNF-α-dependent provision of sTNFR to septic rats. The NLRP3 inflammasome is one of several multi-protein complexes that play important roles in inflammation (Thornberry et al., 1992; Kono et al., 2010). Interestingly, either deficiency or over-activation of the NLRP3 inflammasome is detrimental in a variety of inflammatory disorders (Becker and O'Neill, 2007). Importantly, inflammasome activation of part of a well-established cascade of responses to severe infection (Wiersinga, 2011; Witzenrath et al., 2011). We speculate that this is a protective effect, given recent studies that pointed to a protective role for NLRP3 inflammasome activation in the setting of endotoxemia and burn injury (Osuka et al., 2012), as well as studies showing that caspase-1–the end-product of inflammasome activation–as well as IL-1β are diminished in peripheral blood mononuclear cells from sepsis patients (Giamarellos-Bourboulis et al., 2011).
Interestingly, our results showed lower circulating TNF-α in the plasma and better improvement in PaO2 in rats treated with the Clone 3 (TNF-α-dependent) bioreactor relative to the CMV-regulated (constitutive) bioreactor. These results appear counter-intuitive from the point of view of total amount of sTNFR produced, since the CMV-regulated bioreactor led to higher circulating levels of sTNFR as compared to levels observed in animals treated with the Clone three bioreactor. Within the context of these results, we hypothesize that adaptive production of sTNFR is either more efficient at neutralizing TNF-α or leads to lower production of TNF-α by some mechanism other than neutralization. Prior studies of multiple TNF-α antagonists, including the sTNFR drug etanercept, suggest that in addition to TNF-α inhibition via binding of sTNFR to soluble TNF-α, another mechanism of action involves binding of sTNFR to membrane-bound TNF-α on cells and subsequent suppression of further TNF-α production (Mitoma et al., 2018). We therefore hypothesize that the sTNFR produced by Clone three bioreactor is produced in a manner more like that of physiologic sTNFR production and is thus present at lower circulating levels, but binds to membrane-bound TNF-α and prevents further secretion of TNF-α. We further hypothesize that with the much greater production of sTNFR via the CMV bioreactor, the majority of sTNFR remains in the circulation and does not bind to membrane TNF-α. We speculate that this difference underlies the better physiologic function in septic rats treated with the Clone three bioreactor as compared to animals treated with the CMV-regulated device, but further mechanistic studies are needed to address this issue.
Our study demonstrates the potential utility of synthetic biology approaches to the control of systemic acute inflammation, via the combined use of engineered cells and bioreactors. There have been multiple reports of engineering cells to create specific behaviors in vivo. For example, Siciliano et al reported on the generation of CHO cell variants that expressed a circuit designed specifically to create positive feedback (Siciliano et al., 2011). Kemmer et al described modifying mice using a synthetic circuit to control of urate homeostasis (Kemmer et al., 2010). Recently, Xie et al reported on the generation of minimally engineered of human cells, in which glucose responsiveness was obtained via a synthetic circuit that couples glycolysis-mediated calcium entry to an excitation-transcription system controlling therapeutic transgene expression. These cells could correct insulin deficiency and abolished persistent hyperglycemia in type 1 diabetic mice (Xie et al., 2016). To our knowledge, however, the current study is the first reported example of an adaptive circuit for the control of systemic acute inflammation in vivo.
Our study has several limitations. One limitation concerns the potential for secretion of mediators other than sTNFR by the various cell lines utilized in this study, which may impact inflammation and/or physiology. Although Clone nine HepG2 cells did not secrete sTNFR above the detection limit and hence were used as negative control, these cells may well secrete other mediators and thus may have contributed to some degree to the distinct dynamics of the inflammatory response between the LPS + Clone nine bioreactor vs. LPS alone and Gram-negative sepsis + Clone 3 vs. Gram-negative sepsis alone. Other limitations include the assessment of a limited number of protein-level mediators (vs. extensive transcriptomic, metabolomic, or other additional analyses that might yield additional insights into the impact on inflammation of the various biohybrid constructs), as well as the inclusion of additional experimental repeats and concomitantly larger datasets may increase confidence in the output of the various machine learning analyses utilized in these studies. Finally, we note that given the complex, in vivo biological responses to LPS and Gram-negative E. coli, it is difficult to compare in vitro results from gene-modified HepG2 cells to in vivo results directly.
Our long-term goal is to create a self-adaptive device for temporary extracorporeal application modulating the inflammatory response. If successful, this device would help solve the current need for a personalized (yet standardized) inflammation-modulating therapy. The device would be standardized since, for a given disease, a single bioreactor would be used. This feature should reduce regulatory hurdles. The treatment would be personalized, since a given patient’s individual production of cytokines would be counteracted in a precise fashion and only as required. Furthermore, our initial studies reported herein demonstrate the feasibility of the components required to create such a device (capability of engineering molecular circuits and the use of gene-modified cells to modulate inflammation in vivo), and, thus, represent a key step toward the concept of a self-adaptive, biohybrid device for the control of systemic inflammation. In order to proceed toward clinical use, we will need to generate GMP-grade HepG2 (or other) cell lines expressing the human versions of the gene constructs and test them in a device applied to small animals, large animals (possibly non-human primates), and studies in humans culminating in Phase III clinical trials. Regulatory challenges include the fact that such a biohybrid, adaptive device currently has no known predicates (though predicate devices using extracorporeal filtration for sepsis do exist (Namas et al., 2012c; Panagiotou et al., 2013; Girardot et al., 2019)).
Importantly, there must be a rational process by which to tailor the specific characteristics of such a device. For example, the specific cytokines to be antagonized and the timing and magnitude of such manipulation will vary depending on the nature of the inflammatory disease targeted. It is therefore highly likely that computational modeling of inflammation will be a necessary part of this rational inflammation reprogramming approach in the context of personalized or precision medicine (Mi et al., 2010). The present study is a first step in that direction, using data-driven modeling of dynamic networks and principal drivers to suggest possible adjunct targets, such as the NLRP3 inflammasome, that could be engineered into future variants of our biohybrid device. Future studies will also require mechanistic modeling of disease pathways, likely combined with approaches such as model-based dynamic control (Day et al., 2010), that could be leveraged to further optimize our approach to dynamic, self-adaptive regulation of acute inflammation. Finally, we note that the inflammation-regulating device described herein is only a proof of concept; future iterations could target multiple inflammatory pathways and be tuned to other signals, thus targeting diverse disease processes.
The original contributions presented in the study are included in the article/Supplementary Material, further inquiries can be directed to the corresponding author.
The animal study was reviewed and approved by Institutional Animal Care and Use Committee (IACUC) of the University of Pittsburgh.
RN: designed experiments, carried out experiments, analysed data, wrote and edited manuscript, MM: designed experiments, carried out experiments, analysed data, wrote and edited manuscript, JY: carried out experiments, analysed data, wrote and edited manuscript, DB: carried out experiments, BJ: carried out experiments, QM: analysed data, wrote and edited manuscript, TB: provided funding, edited manuscript, RZ: analysed data, wrote and edited manuscript, JG: provided funding, designed experiments, wrote and edited manuscript, YV: provided funding, designed experiments, wrote and edited manuscript.
This work was supported by NIH grant RO1-GM107231-01A1 as well as DARPA grant D20AC00002.
JG owns shares of StemCell Systems that provided bioreactors used in this study. YV and TB are co-founders and stakeholders in Immunetrics, Inc. A patent on the device described in this manuscript has been granted. These financial interests are managed by the University of Pittsburgh under all applicable institutional and federal guidelines.
The remaining authors declare that the research was conducted in the absence of any commercial or financial relationships that could be construed as a potential conflict of interest.
All claims expressed in this article are solely those of the authors and do not necessarily represent those of their affiliated organizations, or those of the publisher, the editors and the reviewers. Any product that may be evaluated in this article, or claim that may be made by its manufacturer, is not guaranteed or endorsed by the publisher.
The Supplementary Material for this article can be found online at: https://www.frontiersin.org/articles/10.3389/fsysb.2022.926618/full#supplementary-material
Abboud, A. N., Namas, R. A., Ramadan, M., Mi, Q., Almahmoud, K., Abdul-Malak, O., et al. (2016). Computational analysis supports an early, type 17 cell-associated divergence of blunt trauma survival and mortality. Crit. Care Med. 44, e1074–e1081. doi:10.1097/CCM.0000000000001951
Arend, W. P., Malyak, M., Smith, M. F., Whisenand, T. D., Slack, J. L., Sims, J. E., et al. (1994). Binding of IL-1 alpha, IL-1 beta, and IL-1 receptor antagonist by soluble IL-1 receptors and levels of soluble IL-1 receptors in synovial fluids. J. Immunol. 153 (10), 4766–4774.
Azhar, N., Ziraldo, C., Barclay, D., Rudnick, D. A., Squires, R. H., Vodovotz, Y., et al. (2013). Analysis of serum inflammatory mediators identifies unique dynamic networks associated with death and spontaneous survival in pediatric acute liver failure. PLoS One 8 (11), e78202. doi:10.1371/journal.pone.0078202
Becker, C. E., and O'Neill, L. A. (2007). Inflammasomes in inflammatory disorders: the role of TLRs and their interactions with NLRs. Semin. Immunopathol. 29 (3), 239–248. doi:10.1007/s00281-007-0081-4
Clermont, G., Bartels, J., Kumar, R., Constantine, G., Vodovotz, Y., and Chow, C. (2004). In silico design of clinical trials: a method coming of age. Crit. Care Med. 32, 2061–2070. doi:10.1097/01.ccm.0000142394.28791.c3
Day, J., Rubin, J., and Clermont, G. (2010). Using nonlinear model predictive control to find optimal therapeutic strategies to modulate inflammation. Math. Biosci. Eng. 7 (4), 739–763. doi:10.3934/mbe.2010.7.739
Dull, T., Zufferey, R., Kelly, M., Mandel, R. J., Nguyen, M., Trono, D., et al. (1998). A third-generation lentivirus vector with a conditional packaging system. J. Virol. 72 (11), 8463–8471. doi:10.1128/JVI.72.11.8463-8471.1998
Eichacker, P. Q., Hoffman, W. D., Farese, A., Banks, S. M., Kuo, G. C., MacVittie, T. J., et al. (1991). TNF but not IL-1 in dogs causes lethal lung injury and multiple organ dysfunction similar to human sepsis. J. Appl. Physiol. 71 (5), 1979–1989. doi:10.1152/jappl.1991.71.5.1979
Eming, S. A., Wynn, T. A., and Martin, P. (2017). Inflammation and metabolism in tissue repair and regeneration. Science 356 (6342), 1026–1030. doi:10.1126/science.aam7928
Emr, B., Sadowsky, D., Azhar, N., Gatto, L. A., An, G., Nieman, G. F., et al. (2014). Removal of inflammatory ascites is associated with dynamic modification of local and systemic inflammation along with prevention of acute lung injury: In vivo and in silico studies. Shock 41 (4), 317–323. doi:10.1097/SHK.0000000000000121
Gerlach, J. C. (2006). Bioreactors for extracorporeal liver support. Cell Transpl. 15, S91–S103. doi:10.3727/000000006783982296
Giamarellos-Bourboulis, E. J., van de Veerdonk, F. L., Mouktaroudi, M., Raftogiannis, M., Antonopoulou, A., Joosten, L. A., et al. (2011). Inhibition of caspase-1 activation in Gram-negative sepsis and experimental endotoxemia. Crit. Care 15 (1), R27. doi:10.1186/cc9974
Girardot, T., Schneider, A., and Rimmelé, T. (2019). Blood purification techniques for sepsis and septic AKI. Semin. Nephrol. 39 (5), 505–514. doi:10.1016/j.semnephrol.2019.06.010
Grzegorczyk, M., and Husmeier, D. (2011). Improvements in the reconstruction of time-varying gene regulatory networks: dynamic programming and regularization by information sharing among genes. Bioinformatics 27 (5), 693–699. doi:10.1093/bioinformatics/btq711
Janes, K. A., and Yaffe, M. B. (2006). Data-driven modelling of signal-transduction networks. Nat. Rev. Mol. Cell Biol. 7 (11), 820nrm2041–828. doi:10.1038/nrm2041
Kemmer, C., Gitzinger, M., Daoud-El Baba, M., Djonov, V., Stelling, J., and Fussenegger, M. (2010). Self-sufficient control of urate homeostasis in mice by a synthetic circuit. Nat. Biotechnol. 28 (4), 355–360. doi:10.1038/nbt.1617
Khalil, N. (1999). TGF-[beta]: from latent to active. Microbes Infect. 1 (15), 1255–1263. doi:10.1016/S1286-4579(99)00259-2
Kono, H., Chen, C. J., Ontiveros, F., and Rock, K. L. (2010). Uric acid promotes an acute inflammatory response to sterile cell death in mice. J. Clin. Invest. 120 (6), 1939–1949. doi:10.1172/JCI40124
Lavrovsky, Y., Schwartzman, M. L., Levere, R. D., Kappas, A., and Abraham, N. G. (1994). Identification of binding sites for transcription factors NF-kappa B and AP-2 in the promoter region of the human heme oxygenase 1 gene. Proc. Natl. Acad. Sci. 91 (13), 5987–5991. doi:10.1073/pnas.91.13.5987
Leitman, D. C., Ribeiro, R. C., Mackow, E. R., Baxter, J. D., and West, B. L. (1991). Identification of a tumor necrosis factor-responsive element in the tumor necrosis factor alpha gene. J. Biol. Chem. 266 (15), 9343–9346. doi:10.1016/s0021-9258(18)92822-x
Li, Q., and Verma, I. M. (2002). NF-[kappa]B regulation in the immune system. Nat. Rev. Immunol. 2 (10), 725–734. doi:10.1038/nri910
May, M. J., and Ghosh, S. (1998). Signal transduction through NF-kappa B. Immunol. Today 19 (2), 80–88. doi:10.1016/S0167-5699(97)01197-3
Medzhitov, R. (2008). Origin and physiological roles of inflammation. Nature 454 (7203), 428–435. doi:10.1038/nature07201)
Mi, Q., Li, N. Y. K., Ziraldo, C., Ghuma, A., Mikheev, M., Squires, R., et al. (2010). Translational systems biology of inflammation: Potential applications to personalized medicine. Pers. Med. 7, 549–559. doi:10.2217/pme.10.45
Mi, Q., Constantine, G., Ziraldo, C., Solovyev, A., Torres, A., Namas, R., et al. (2011). A dynamic view of trauma/hemorrhage-induced inflammation in mice: principal drivers and networks. PLoS One 6 (5), e19424. doi:10.1371/journal.pone.0019424
Mitoma, H., Horiuchi, T., Tsukamoto, H., and Ueda, N. (2018). Molecular mechanisms of action of anti-TNF-α agents - comparison among therapeutic TNF-α antagonists. Cytokine 101, 56–63. doi:10.1016/j.cyto.2016.08.014
Naldini, L., Blömer, U., Gallay, P., Ory, D., Mulligan, R., Gage, F. H., et al. (1996). In vivo gene delivery and stable transduction of nondividing cells by a lentiviral vector. Science 272 (5259), 263–267. doi:10.1126/science.272.5259.263
Namas, R. A., Zamora, R., Namas, R., An, G., Doyle, J., Dick, T. E., et al. (2012). Sepsis: Something old, something new, and a systems view. J.Crit Care 27, 314.e1–314.e11. doi:10.1016/j.jcrc.2011.05.025
Namas, R. A., Mikheev, M., Yin, J., Over, P., Young, M., Constantine, G., et al. (2012). A biohybrid device for the systemic control of acute inflammation. Disrupt.Science Technol. 1, 20–27. doi:10.1089/dst.2012.0001
Namas, R. A., Namas, R., Lagoa, C., Barclay, D., Mi, Q., Zamora, R., et al. (2012). Hemoadsorption reprograms inflammation in experimental Gram-negative septic fibrin peritonitis: Insights from in vivo and in silico studies. Mol. Med. 18, 1366–1374. doi:10.2119/molmed.2012.00106
Namas, R. A., Mi, Q., Namas, R., Almahmoud, K., Zaaqoq, A., Abdul Malak, O., et al. (2015). Insights into the role of chemokines, damage-associated molecular patterns, and lymphocyte-derived mediators from computational models of trauma-induced inflammation. Antiox. Redox Signal. 10, 1370–1387. doi:10.1089/ars.2015.6398
Namas, R. A., Almahmoud, K., Mi, Q., Ghuma, A., Namas, R., Zaaqoq, A., et al. (2016). Individual-specific principal component analysis of circulating inflammatory mediators predicts early organ dysfunction in trauma patients. J. Crit. Care 36, 146–153. doi:10.1016/j.jcrc.2016.07.002
Namas, R. A., Vodovotz, Y., Almahmoud, K., Abdul-Malak, O., Zaaqoq, A., Namas, R., et al. (2016). Temporal patterns of circulating inflammation biomarker networks differentiate susceptibility to nosocomial infection following blunt trauma in humans. Ann. Surg. 263 (1), 191–198. doi:10.1097/sla.0000000000001001
Nathan, C. (2002). Points of control in inflammation. Nature 420 (6917), 846–852. doi:10.1038/nature01320
Osuka, A., Hanschen, M., Stoecklein, V., and Lederer, J. A. (2012). A protective role for inflammasome activation following injury. Shock (Augusta, Ga.) 37 (1), 47–55. doi:10.1097/SHK.0b013e318234f7ff
Panagiotou, A., Gaiao, S., and Cruz, D. N. (2013). Extracorporeal therapies in sepsis. J. Intensive Care Med. 28 (5), 281–295. doi:10.1177/0885066611425759
Parker, S. J., and Watkins, P. E. (2001). Experimental models of gram-negative sepsis. Br. J. Surg. 88 (1), 22–30. doi:10.1046/j.1365-2168.2001.01632.x
Scheff, J. D., Mavroudis, P. D., Foteinou, P. T., An, G., Calvano, S. E., Doyle, J., et al. (2013). A multiscale modeling approach to inflammation: A case study in human endotoxemia. Shock 244, 279–289. doi:10.1016/j.jcp.2012.09.024
Schmelzer, E., Mutig, K., Schrade, P., Bachmann, S., Gerlach, J. C., and Zeilinger, K. (2009). Effect of human patient plasma ex vivo treatment on gene expression and progenitor cell activation of primary human liver cells in multi-compartment 3D perfusion bioreactors for extra-corporeal liver support. Biotechnol. Bioeng. 103 (4), 817–827. doi:10.1002/bit.22283
Seymour, C. W., Liu, V. X., Iwashyna, T. J., Brunkhorst, F. M., Rea, T. D., Scherag, A., et al. (2016). Assessment of clinical criteria for sepsis: For the third international consensus definitions for sepsis and septic shock (Sepsis-3). Jama 315 (8), 762–774. doi:10.1001/jama.2016.0288
Siciliano, V., Menolascina, F., Marucci, L., Fracassi, C., Garzilli, I., Moretti, M. N., et al. (2011). Construction and modelling of an inducible positive feedback loop stably integrated in a mammalian cell-line. PLoS Comput. Biol. 7 (6), e1002074. doi:10.1371/journal.pcbi.1002074
Siebenlist, U., Franzoso, G., and Brown, K. (1994). Structure, regulation and function of NF-kappa B. Annu. Rev. Cell Biol. 10, 405–455. doi:10.1146/annurev.cb.10.110194.002201
Strieter, R. M., Kunkel, S. L., and Bone, R. C. (1993). Role of tumor necrosis factor-alpha in disease states and inflammation. Crit. Care Med. 21, S447–S463. doi:10.1097/00003246-199310001-00006
Symons, J. A., Young, P. R., and Duff, G. W. (1995). Soluble type II interleukin 1 (IL-1) receptor binds and blocks processing of IL-1 beta precursor and loses affinity for IL-1 receptor antagonist. Proc. Natl. Acad. Sci. 92 (5), 1714–1718. doi:10.1073/pnas.92.5.1714
Thornberry, N. A., Bull, H. G., Calaycay, J. R., Chapman, K. T., Howard, A. D., Kostura, M. J., et al. (1992). A novel heterodimeric cysteine protease is required for interleukin-1 beta processing in monocytes. Nature 356 (6372), 768–774. doi:10.1038/356768a0
Van Zee, K. J., Kohno, T., Fischer, E., Rock, C. S., Moldawer, L. L., and Lowry, S. F. (1992). Tumor necrosis factor soluble receptors circulate during experimental and clinical inflammation and can protect against excessive tumor necrosis factor alpha in vitro and in vivo. Proc. Natl. Acad. Sci. 89 (11), 4845–4849. doi:10.1073/pnas.89.11.4845
Vodavotz, Y., and An, G. (2013). Complex systems and computational biology approaches to acute inflammation. New York, NY: Springer.
Wiersinga, W. J. (2011). Current insights in sepsis: from pathogenesis to new treatment targets. Curr. Opin. Crit. Care 17 (5), 480–486. doi:10.1097/MCC.0b013e32834a4aeb
Windsor, A. C., Walsh, C. J., Mullen, P. G., Cook, D. J., Fisher, B. J., Blocher, C. R., et al. (1993). Tumor necrosis factor-alpha blockade prevents neutrophil CD18 receptor upregulation and attenuates acute lung injury in porcine sepsis without inhibition of neutrophil oxygen radical generation. J. Clin. Invest. 91 (4), 1459–1468. doi:10.1172/JCI116351
Witzenrath, M., Pache, F., Lorenz, D., Koppe, U., Gutbier, B., Tabeling, C., et al. (2011). The NLRP3 inflammasome is differentially activated by pneumolysin variants and contributes to host defense in pneumococcal pneumonia. J. Immunol. 187 (1), 434–440. doi:10.4049/jimmunol.1003143
Xie, M., Ye, H., Wang, H., Charpin-El Hamri, G., Lormeau, C., Saxena, P., et al. (2016). β-cell-mimetic designer cells provide closed-loop glycemic control. Science 354 (6317), 1296–1301. doi:10.1126/science.aaf4006
Zamora, R., Korff, S., Mi, Q., Barclay, D., Yin, J., Schimunek, L., et al. (2018). A computational analysis of dynamic, multi-organ inflammatory crosstalk induced by endotoxin in mice. PLoS Comput. Biol. 6, e1006582. doi:10.1371/journal.pcbi.1006582
Zamora, R., Chavan, S., Zanos, T., Simmons, R. L., Billiar, T. R., and Vodovotz, Y. (2021). Spatiotemporally specific roles of TLR4, TNF, and IL-17A in murine endotoxin-induced inflammation inferred from analysis of dynamic networks. Mol. Med. 27 (1), 65. doi:10.1186/s10020-021-00333-z
Zeilinger, K., Schreiter, T., Darnell, M., Soderdahl, T., Lubberstedt, M., Dillner, B., et al. (2011). Scaling down of a clinical three-dimensional perfusion multicompartment hollow fiber liver bioreactor developed for extracorporeal liver support to an analytical scale device useful for hepatic pharmacological in vitro studies. Tissue Eng. Part C Methods 17 (5), 549–556. doi:10.1089/ten.TEC.2010.0580
Keywords: endotoxemia, sepsis, lipopolysaccharide, tumor necrosis factor, sTNFR, HepG2 cells
Citation: Namas RA, Mikheev M, Yin J, Barclay D, Jefferson B, Mi Q, Billiar TR, Zamora R, Gerlach J and Vodovotz Y (2023) An adaptive, negative feedback circuit in a biohybrid device reprograms dynamic networks of systemic inflammation in vivo. Front. Syst. Biol. 2:926618. doi: 10.3389/fsysb.2022.926618
Received: 22 April 2022; Accepted: 22 December 2022;
Published: 10 January 2023.
Edited by:
Xiaogang Wu, University of Texas MD Anderson Cancer Center, United StatesReviewed by:
Wei Cheng, Dalian Medical University, ChinaCopyright © 2023 Namas, Mikheev, Yin, Barclay, Jefferson, Mi, Billiar, Zamora, Gerlach and Vodovotz. This is an open-access article distributed under the terms of the Creative Commons Attribution License (CC BY). The use, distribution or reproduction in other forums is permitted, provided the original author(s) and the copyright owner(s) are credited and that the original publication in this journal is cited, in accordance with accepted academic practice. No use, distribution or reproduction is permitted which does not comply with these terms.
*Correspondence: Yoram Vodovotz, dm9kb3ZvdHp5QHVwbWMuZWR1
†These authors have contributed equally to this work and share first authorship
Disclaimer: All claims expressed in this article are solely those of the authors and do not necessarily represent those of their affiliated organizations, or those of the publisher, the editors and the reviewers. Any product that may be evaluated in this article or claim that may be made by its manufacturer is not guaranteed or endorsed by the publisher.
Research integrity at Frontiers
Learn more about the work of our research integrity team to safeguard the quality of each article we publish.