- Department of Surgery, University of Vermont Larner College of Medicine, Burlington, VT, United States
Introduction: What is Translational Systems Biology?
The following are the Principles of Translational Systems Biology condensed from the definition stated in the book: “Translational Systems Biology: Concepts and Practice for the Future of Biomedical Research” (1), page 6-7:
“Primary Goal: Facilitate the translation of basic biomedical research to the implementation of effective clinical therapeutics.
Primary Design Strategies:
1. Utilize dynamic computational modeling to capture mechanism.
2. Develop a framework that allows “useful failure” à la [Karl] Popper.
3. Ensure that the framework is firmly grounded with respect to the history and philosophy of science.
Primary Methodological Strategies:
1. Use dynamic computational modeling to accelerate the pre-clinical Scientific Cycle by enhancing hypothesis testing, which will improve efficiency in developing better drug candidates…
2. Use simulations of clinical implementation via in silico clinical trials and personalized simulations to increase the efficiency of the terminal phase of the therapy development pipeline…
3. Use the power of abstraction provided by dynamic computational models to identify core, conserved functions and behaviors to bind together and bridge between different biological models and individual patients…
What Translational Systems Biology is not (which is not to say that the following are not laudable, or even necessary goals):
1. Translational Systems Biology is not using computational modeling to gain increasingly detailed information about biological systems.
2. Translational Systems Biology is not aiming to reproduce detail as the primary goal of modeling; level of detail included needs to be justified from a translational standpoint.
3. Translational Systems Biology is not aiming to develop the most quantitatively precise computational model of a pre-clinical, or sub-patient level system.
4. Translational Systems Biology is not just the collection and computational analysis of extensive data sets in order to provide merely a broad and deep description of a system, even if those data sets span a wide range of scales of organization spanning the gene to socio-environmental factors.”
This editorial is part of the Grand Challenge series for “Frontiers in Systems Biology”, specifically regarding the subsection “Translational Systems Biology and In silico Clinical Trials.” It represents my impressions, based on my own research experiences, on the challenges that face biomedical research moving forward. It is intended to be provocative and disruptive. With this in mind, this editorial will address what I consider the grandest of challenges facing biomedical research: crossing the Valley of Death in drug development. I assert that meeting this challenge is subject to a failure of imagination by a large sector of the biomedical research community, due in great degree to a lack of recognition of fundamental aspects of why the problem is so hard. I will address these aspects within the context of what is needed to cross the Valley of Death.
The Primary Goal of Biomedical Research: “True” Precision Medicine
My research career is grounded in the fact that I am first and foremost a clinician (I am a practicing trauma surgeon and surgical intensivist). As someone who treats critically-ill patients on a regular basis, no day goes by that I don’t wish that I had better drugs to treat my patients; where “better” means: more efficacious, fewer and less side effects, there are clear indications on who gets what drug and when, and that there is such a drug for every patient. I have formally defined this goal in the following Axioms of “True” Precision Medicine (An and Day et al., 2021):
Axiom 1. Patient A is not the same as Patient B (Personalization).
Axiom 2. Patient A at Time X is not the same as Patient A at Time Y (Precision).
Axiom 3. The goal of medicine is to treat, prognosis is not enough (Treatment).
Axiom 4. Precision medicine should find effective therapies for every patient and not only identify groups of patients that respond to a particular regimen (Inclusiveness)At some level these Axioms would seem to be self-evident, until one looks at the divergence present in the current practice of biomedical research (hence the quotes around “True”). For example:
1. The need to generate sufficient statistical power in clinical trials is necessarily in tension with the concept that individual patients can be (and often are) different (see Evidence Based Medicine*).
2. Emphasis on being able to prognose and forecast future disease progression is fine, but without the ability to change that potential future such information is much less useful (see emphasis on prediction in sepsis*).
3. While it is important to identify what existing therapies might be best suited for a particular subset of patients, there is no substantive improvement in the process of finding what can be done for those for whom no existing therapies are effective (see Precision Oncology*).
*Note: these refer to an entire corpus of literature that interested readers can readily survey.This is where the failure of imagination manifests: All too often the focus is on using tools currently employed and being limited by their existing capabilities instead of identifying the limitations of these tools and thinking about what new approaches are necessary to overcome them. We will return to these Axioms throughout this paper as they highlight key methodological limitations present in current biomedical research that inhibit our ability to achieve the goal of “True” Precision Medicine.The Barrier: The Valley of Death
Why do I not have better drugs to treat my patients, regardless of when I see them and how sick they are? I contend that the biggest hurdle to getting better drugs and therapies to treat each and every patient (Axiom 4) is the “Valley of Death”: The inability to efficiently translate basic science knowledge obtained from preclinical studies into effective therapies (Butler, 2008) (see Figure 1). The location of the Valley of Death (i.e., between pre-clinical suggestions of efficacy and clinical evaluation) is evidence that the main problem is not finding new drug candidates, e.g., identifying molecular compounds that effectively chemically interact with targeted biological components, but rather the inability to reliably determine: 1) If those targeted biological components should actually be targeted within the context of the entire human (which requires recontextualizing the putative action of a drug at a system level), and 2) if they should be targeted, how and when should such targeting be applied to have the desired effect (Axiom 1 and Axiom 2). It is worth noting that every compound that has failed in clinical trials started as a “promising” candidate, and successfully passed through all the phases of preclinical testing only to crash into the Valley of Death. My assertion is that technological advances in identifying potential drug candidate compounds (Jumper et al., 2021), while impressive and necessary, do not address the primary bottleneck in achieving our goal of getting better drugs that can treat all patients. To make an analogy: Even if you build a 6-lane superhighway that leads up to a cliff, if your bridge-building technology is limited to a wooden slat-rope bridge there will not be increased traffic across the chasm.
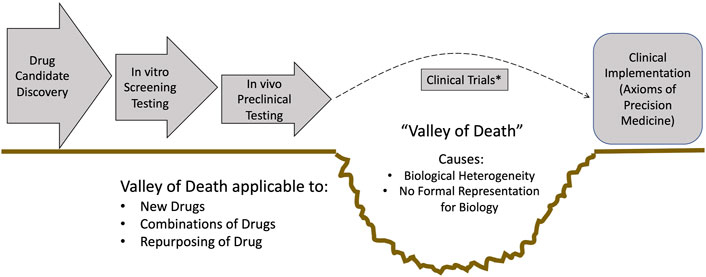
FIGURE 1. Schematic depicting the drug development pipeline and the location of the Valley of Death. Note that the steps to the left of the Valley of Death can be augmented with existing technological advances: Improved candidate identification via tools like AlphaFold (Jumper et al., 2021), high-throughput candidate screening using systems biology platforms, and improved, more clinically relevant pre-clinical models. However, none of these advances improve the actual step of translation to clinical populations: Clinical trials will still need to be done, and this remains the primary bottleneck in being able to provide clinicians with better drugs, optimal combinations of drugs and effective and efficient repurposing of drugs. *Note: I acknowledge that there have been developments in making clinical trials more productive, such as adaptive trials. But these approaches do not address the fundamental issue of how to better predict what drug, or combination of drugs, could prove to be effective.
So why does the Valley of Death exist? I assert that the Valley of Death exists for two main distinct but interrelated reasons:
1. Biological heterogeneity, namely the biological property that leads to intrinsic variability in how an organism responds to its environment (and changes thereof) (An, 2018). This is notably evident in virtually every experimental and clinical data set (e.g., “error” bars). The inability of currently employed statistical tools to account for the actual implications of such variability, i.e., the fact that the biological object was able to produce that particular measurement, is central to existence of the Valley of Death.
2. Current means of determining what is similar or conserved between one biological object and another are primarily descriptive and do not account for how the dynamics/behavior of a system arise (An, 2018).
Underlying these two properties are the following sequence of facts:
1. There is no formal unifying representation of how a biological organism behaves to produce the data that is collected (mechanistic dynamics).
2. Thus, there is no formal representation of what is similar and what is different between the behavior of biological organisms.
3. Therefore, there is an inability to rigorously and formally assess the effect of a perturbation or intervention on the behavior of that organism.
These features translate into the principal failings seen in the “Valley of Death”:
1. There is too much variation in the study population such that the signal-to-noise ratio regarding the efficacy of a particular compound cannot be statistically surmounted.
2. Attempts to narrow the definition of a study population intrinsically reduces the power of a planned clinical trial, which in turn makes it less likely that there will be statistically significant results and lessens the applicability of the potential drug.
3. The current means of narrowing the definition of a study population is ill-posed because there lacks a formal means of determining and characterizing what actually causes one patient to behave differently from another patient.
4. There is no process for “useful failure” in clinical trials. Since the reason an intervention did not work in a particular patient can only be indirectly inferred (since no formal representation of how that patient’s physiology behaved in response to the drug), that failure provides no meaningful insight into how it can be corrected in the future.
In short, the Valley of Death is a manifestation of the inability of the current biomedical research pipeline to achieve the goals of “True” Precision Medicine. It deals poorly with heterogeneity across individuals (Axiom 1), infrequently deals with dynamic heterogeneity of an individual over time (Axiom 2) and lacks an approach to correct these failings and better design effective therapies for every patient (Axiom 4).
Furthermore, biological heterogeneity presents challenges to data-centric approaches that use probabilistic/statistical models that attempt to correlate data to some underlying biological process. This is because of the sparsity of biological data relative to the number of features/variables present at each time point (e.g., the number of genes/proteins assayed for in a single -omics or biomarker sample). This data sparsity means that biological systems are almost invariably subject to the Curse of Dimensionality (Bellman, 2015), which refers to the combinatorial and exponential explosion when the number of samples needed to estimate an arbitrary function (i.e., mechanism) with a given level of accuracy grows with respect to the number of input variables (i.e., dimensionality) of the function (Chen et al., 2009). The Curse of Dimensionality suggests there is a hard limit to the ability of statistical, and by extension, most machine learning (ML) methods, which are correlative statistical tools at their core, to provide insight into how a biological system works (Jonas and Kording, 2017). I contend that knowing how a system works in integral to developing a rational approach to determining how to control (e.g., treat) that system. In fact, there is a term that describes this process, and that term is engineering.
Engineering a Way Across the Valley of Death: More Complicated Than You Think
The preceding text emphasized the lack of formal representation of biological systems as a recurrent insufficiency in most current approaches to biomedical research; what does this mean and why is it important? Essentially, formal representations equate to mathematics, providing access to all the tools available for using math to solve problems. The power of mathematics arises from its generalizability: The ability to cast a system into a particular mathematical framework allows any system so represented to be operated on/analyzed/reasoned over with the correspondingly appropriate mathematical tools. The combination of mathematical representation and identification of system constraints applied to that mathematical representation underlies the practice of engineering: problem solving and design using a set of formal tools. However, the engineering approach is predicated upon there being sufficient trust in the formal representation of the targeted system, and this leads to a significant challenge in the application of engineering principles to biology in cases where the biological system cannot be effectively represented as a physical system. Mathematical representations of physical systems are trustworthy because there are natural laws that provide grounding those representations. There are laws that govern the physics of mechanics, fluid flow, electrical properties; simulations of physical objects can be grounded by these laws and this provides trust in those simulations. This, however, is not true in much of biology, where the exceptions prove the rule. Mechanical implants (artificial joints and heart valves) can be engineered with respect to material properties (i.e., fatiguability and strength, etc.), but not in terms of the effect of their interfaces with biological tissue. Fluid dynamics models can provide information in planning cardiovascular procedures that alter the anatomic piping to correct abnormal flow, but they cannot represent the progression of the biological processes that result from, and subsequently influence, abnormalities in that flow. The vast majority of biomedical research focuses on generating knowledge in areas that cannot be effectively represented merely as a physical system: The behavior of cells and cellular populations. I contend that dealing with the uncertainties of what makes biology biological, namely the behavior of cells, represents a qualitatively different task (and set of solutions) compared with dealing with physical systems. This distinction is of critical importance because there is a tendency to equate discovering new drugs as an engineering problem, with attendant misleading transfer of methods and terms from the engineering field to biomedicine. One of the most current of these is the interest in “Medical” digital twins.
Medical “Digital Twins”: Why it Doesn’t Mean What People Think it Means
The appeal of a “digital twin” of an individual is obvious; what is less obvious is whether that appeal is grounded in facts about what a digital twin is, whether such a thing can be applied in a medical context, and if so, what are the limits of the capabilities of such an object. The term “digital twin” comes from the world of industrial applications. A digital twin is defined as (Grieves, 2019):
1. A data structure for the real-world system
2. Some process that links data together to form dynamics
3. Some link to the real world that feeds back data into the data-propagation/generation process
The advent and utility of Digital Twins for engineered objects relies on the fact that there exists a formal specification for the “twinned” real world object because those objects have been engineered/designed/constructed using some version of that specification (formal representation). Industrial Digital Twins are simulations of a particular individual example object drawn from a class of objects for which a common formal specification exists. There are several key points here:
1. There exists a common formal specification that is shared by and links different individual examples within a type/class of objects.
2. The goal of the Digital Twin is to examine the future state of the targeted specific physical object, meaning that accounting for dynamics and trajectories of behavior are necessary.
3. The common specification leads to the ability to simulate the behavior of the object, meaning that dynamics and behavior are intrinsic to the specification. This means that the specification includes information about how the system/object works. Because of this, the specification is not just a list of the components of the system/object, but rather a combination of the components and how they interact with each other to generate behavior.
To use the example of an aircraft engine: A digital twin of an aircraft engine is based on a specification of the engine that includes the needed components to make an engine and how they function together, and can be “personalized” to a specific instance of the object in the real world by populating the corresponding variables within its specification/simulation with real-world data extracted from the real-world object (i.e., “twinned”). Thus, a digital twin of a particular engine used to determine its failure points might have variables that account for the alterations in the material properties of the engine over time as measured by some set of metrics. Then simulations of this “twinned” version of the engine can project/forecast specific behavior under certain sets of potential future conditions and suggest interventions (i.e., maintenance or replacement) to forestall a projected failure point. The key here is that the representational capacity of the digital twin is specified at the time of development of the specification; there is a limit to the expressiveness of the specification that is determined by its use. For instance, a digital twin of an aircraft engine will tell you how the engine will wear and perform, but it will not tell you how fast the plane it is attached to will fly, unless the specification includes the properties of the entire airplane. The analogy in the medical field is to “limited” biomedical digital twins, i.e., those based on high level physiological models (fluid resus/pressors) or systems represented as mechanical objects (fluid dynamics or joints); these digital objects only reflect a limited and constrained aspect of the real-world/physical system but are able, despite that limited perspective, to generate information potentially useful for the specified use.
Conversely, the primary difference between a more general “medical” digital twin (i.e., general in the sense that it purports to capture underlying biological mechanisms, as would be needed to assess the effect of or design new drugs) and the traditional industrial digital twin goes back to the issue of heterogeneity and the lack of formal representations/natural laws in biology. Industrial digital twins start with an engineered object, for which, by definition, there exist specifications of the object from which the digital twin is constructed. Alternatively, the specification for a human being does not exist; it needs to be reverse engineered via basic research, with the attendant challenges that go with that entire process (e.g., the Valley of Death). Does this mean that a “medical” digital twin is impossible given our current state of knowledge? I would say “not exactly.” As noted above there are several aspects of biomedicine where a targeted biomedical problem can be effectively described either as a physical system (i.e., artificial joints) or with a sufficiently expressive mathematical model [i.e., fluid administration (Jin et al., 2018) or artificial pancreas (Bekiari et al., 2018)]. However, dealing with the complexity of cellular/multicellular behavior is a qualitatively different task, which is exactly the behavior that is targeted with drugs. In this case one must deal with considerable uncertainty with respect to what is “true” (again impacted by the dual issues of a lack of fundamental natural laws and a lack of formal, mathematical representation suitable for biology). I have previously proposed that sufficiently complex, mechanism-based simulation models can serve as translational objects that can serve as unifying specifications of biological systems (An, 2018); so too here this concept can be applied to the development and use of “medical” digital twins. The epistemic uncertainty associated with biological systems, in conjunction with the acknowledgment that knowledge of how the system works is necessarily and invariably incomplete, results in the concept that instead of “a” twin specification (as generally seen in industrial applications) there is an ensemble of candidate specifications that need to be refined, modified or discarded through a continuous process. This leads me to propose the following basic design principles for human digital twins to be used for drug development and testing (e.g., crossing the Valley of Death), principles that are in turn related to the ostensible goal of medicine reflected in the Axioms of Precision Medicine:
1. In order to create a Medical Digital Twin of a specific person you need to be able to simulate every possible person. This is a statement of generalizability with respect to the specification of the Medical Digital Twin, and an argument for conserved model structures (= candidate specifications) but with varied parameters reflecting differences in how individuals function (An, 2018) (this calls back to Axiom 1, Axiom 2 and Axiom 4).
2. In a related fashion, because the basic premise that a Medical Digital Twin is “twinned” to an individual, the development and use of Medical Digital Twins should stand in direct contrast to methods that attempt to statistically reduce heterogenous data into a “best fit” regressed line. All too often an experimental or clinical data set can be seen as a widely scattered series of points and a statistical analysis generates a line drawn through the cluster of points; this approach explicitly ignores differences in the individual biological objects that produced the data, reflected in Axiom 1 and Axiom 2. The process of “twinning” should be development of means of encompassing those varied trajectories from a common mechanistic specification [a fundamental property of Digital Twins (Grieves, 2019)].
3. People are not merely parts lists; they are composed of cells that interact and do things. The ostensible goals of a Medical Digital Twin, i.e., prediction, forecasting and design, reinforce the importance of dynamics and mechanisms; this calls back to Axiom 2 of Precision Medicine. The limitations and dangers of “data centric” digital twins are recognized in the industrial sector (Wright and Davidson, 2020). Therefore, the requirements of a Medical Digital Twin should place emphasis on the characterization and representation of functions, not just the descriptions of states (“snapshots”). This principle is a reflection of the famous quote from Richard Feynman: “What I cannot create, I cannot understand.”
4. The “medical” in a Medical Digital Twin should reflect the ostensible purpose of medicine: making ill people not ill anymore. This is reflected in Axiom 3 of Precision Medicine: being able to diagnose/prognose is not enough; we want to be able to figure out how to intervene to make people better. The desire to enhance our ability to improve patient care, by either optimizing existing therapies, repurposing existing therapies or accelerating development of new therapies, should be a central and driving feature to the design of Medical Digital Twins.
5. The intrinsic uncertainties and perpetual incompleteness of biological knowledge means that the process of developing Medical Digital Twins needs to mirror and account for those uncertainties: There is no “one” hypothesis (= model structure/specification) that is ontologically true (e.g., equivalent to a “natural law” in physics or chemistry), but rather an ensemble of sufficient hypotheses (= model structures) that need to undergo a constant cycle of testing, falsification and refinement. Some similarities can be drawn from weather forecasting, where an ensemble of models (albeit physics-based models, and therefore grounded in well-established physical laws) are all running in parallel, constantly being evaluated, constantly being improved. This adopts an evolutionary approach by which models, and the scientific knowledge associated with them, progresses (An, 2010).
In summary, there is considerable promise in the translation of the digital twin concept to biomedicine, but also significant pitfalls if the term, as it is applied to Medical Digital Twins, diverges from the original meaning and intent of the term (e.g., see “Precision” or “Personalized” Medicine). The implicit promise of a Medical Digital Twin is that it will enhance the ability of to fulfill the Axioms of Precision Medicine and enhance crossing the Valley of Death, and meeting that promise will require being cognizant of rebranding traditional approaches that do not meet the requirements of the aforementioned Axioms. This is most important in meeting Axiom 3 (prediction is not enough, the goal is therapy) and Axiom 4 (therapies should be discovered for everyone), and leads into concepts of control as a goal.
Complex Control Discovery and Artificial Intelligence
Complex problems call for complex solutions, and, as noted above, the Valley of Death demonstrates that dealing with the complexity of biomedical mechanisms challenges the boundaries of human intuition. Being able to represent biological complexity through dynamic, mechanism-based models is the first step towards understanding (An, 2004; An, 2008; An et al., 2008), but operating over that complexity requires additional methods. This is where modern advances in machine learning (ML) and artificial intelligence (AI) can be beneficial. “Modern” ML/AI, for all intents and purposes, involves the training of artificial neural networks (ANNs) to perform various types of pattern recognition tasks. This monograph is not intended to be a comprehensive review of biomedical ML/AI (there are many such summaries in the current literature), but I do want to highlight two fundamental aspects of ANNs that may be overlooked in those reviews, yet have significant implications regarding how biomedical ML/AI is marketed.
The first is the fact that ANNs are governed by the Universal Approximation Theorem (UAT), which essentially states that an arbitrarily complex ANN can be trained to approximate any function in order to replicate a large-enough data set (Minsky and Papert, 1988; Hornik et al., 1989). This property of ANNs is often not accounted for or acknowledged in the literature of biomedical uses of ML/AI, as the UAT virtually guarantees that the result of a particular ML task will provide an “answer” that meets acceptable performance based on statistical metrics using a traditional split of data into training and testing sets. Thus, reporting performance results of any biomedical ML/AI based on this type of standard internal validation is, to a great degree, meaningless; while this fact raises a series of issues about how biomedical ML should be evaluated, these issues are outside the scope of this article. However, it is important to note that the existence (and general lack of awareness of) the UAT is particularly impactful in biomedical applications with respect to overfitting and underspecification (D'Amour et al., 2020) due to the general and nearly ubiquitous sparsity of available training data [e.g., note how -omics data/biomarker panels manifest the Curse of Dimensionality (Chen et al., 2009)]. In the general, wider ML community training data is augmented by the use of synthetic data, where additional algorithms are used to generate “realistic” synthetic data points (Nikolenko, 2021). This has proved to be effective in image recognition tasks, but is challenging regarding attempts to predict/forecast (which require time series data) due to the UAT: Because of the UAT an ANN trained on synthetic time series data merely recapitulates the simulation model/algorithm used to generate the time series. This property of the UAT can be seen as a “feature” when applied to reconstituting known equations governing physical laws [i.e., deterministic chaos (Pathak et al., 2018), turbulence (Novati et al., 2021) and cosmology (Villaescusa-Navarro et al., 2021)], but leads to off-target training if the simulation model is used to generate synthetic time series data.
The second overlooked fundamental property regarding the capabilities of ML/AI in nearly all current biomedical applications (with one notable exception, which will be expanded upon below) is the fact that an arbitrarily complex ANN, despite being able to approximate any function, cannot predict the effects of interventions on that function based on data alone (Xia et al., 2021) i.e., it cannot be used to discover “new” means of controlling a system represented by that function based using data alone [see Causal Hierarchy Theorem (Xia et al., 2021)]. What this means is that while ML/AI can suggest new potential points/means of intervening on a system through traditional correlative methods, it is unable to evaluate what the potential effect of that intervention might actually be; this process still requires the traditional experimental pipeline that produces the Translational Dilemma and the Valley of Death, and intrinsically limits many claims as to how ML/AI will accelerate the delivery of new drugs to market [see AlphaFold (Jumper et al., 2021)].
The exception to this inability of ML/AI to pose and test new interventions is the use of Deep Reinforcement Learning (DRL), a form of training ANNs that utilizes time series/outcome data to identify combinations of actions that can alter/change the behavior/trajectories of a system (Lillicrap et al., 2015). The key requirement for DRL to function is the existence of counterfactuals with respect to any intervention; it is only when there is an ability to demonstrate the “what if?” alternative to a particular intervention that the true effect of that intervention can be evaluated. As such, when there is sufficient existing data regarding counterfactuals [as has been seen in DRL applied to the fluid and vasopressor management of sepsis (Komorowski et al., 2018), though with significant limitations well-described in (Jeter et al., 2019)], DRL can be used to train an AI agent to a management policy. But as is the case in nearly all aspects of drug development and testing, such information does not exist unless the trial has already been performed (in which the potential chasm of the Valley of Death has not been avoided). The solution to this problem can be seen in perhaps the most dramatic successes of modern AI: The game-playing AIs from Deep Mind which have mastered Go and other games (Silver et al., 2017; Silver et al., 2018; Vinyals et al., 2019). These systems have demonstrated the ability of DRL to train ANNs to execute dynamic policies of unfathomable complexity in order to achieve a specified goal (e.g., “win the game”). However, all these systems share the same requirement that there is a simulation of the “game” to be won; such simulations are needed in order to generate the synthetic data (which includes the necessary counterfactuals) to sufficiently train the ANN (and this is why this type of DRL is not bound by the Causal Hierarchy Theorem). We term this group of applications of DRL as simulation-based DRL; this describes the use of simulation-generated training data without a pre-defined structural model for the DRL agent.
We have demonstrated the feasibility of this approach by examining the use of simulation-based DRL to discover controls for a disease process for which no effective control strategy exists: Sepsis (Chousterman et al., 2017). In this work we demonstrated that a previously validated agent-based model of systemic inflammation (An, 2004; Cockrell and An, 2017), which replicated failed anti-cytokine therapies for sepsis (An, 2004), could be used to train a DRL AI to develop a complex, adaptive, multi-modal control policy to effectively steer sepsis simulations back to a state of health (Petersen et al., 2019; Larie et al., 2022). However, while these studies provided proof-of-concept for this approach, there is a critical reliance on the quality of the target simulations; because of the UAT there need to be approaches that can deal with the persistent danger of over-fitting to the simulation-generator of the synthetic training data. This is the point at which we need to discuss how to design and implement in silico trials to cross the Valley of Death and achieve True Precision Medicine.
From Populations to Populations of individuals: In Silico Trials
For the foreseeable future the gold-standard for determining the efficacy of a new drug or a new drug-combination or effective drug-repurposing will be a clinical trial; success is what lies on the other side of the Valley of Death. The goal, then, is to increase the efficiency and likelihood that a particular candidate will be able to cross the Valley of Death, e.g., a multi-lane bridge instead of a rope and slate one. This goal would normally call for the application of “engineering” principles of design to more rigorously pre-test potentially effective putative interventions, but we have also recognized that, currently, biomedical systems (or at least the cellular-molecular biology critical to drug development) do not meet the requirements needed for the application of engineering approaches, namely the lack the formal representations/specifications to robustly describe aggregated cellular behavior. The solution we have proposed is the use of multiscale mechanism-based simulation models that accept epistemic uncertainty as surrogate systems for understanding and control discovery (An, 2008; An, 2010; An et al., 2017; An, 2018; An and Day, 2021); these models essentially function as populations of Medical Digital Twins in order to perform in silico clinical trials (An, 2004; Petersen et al., 2019; Larie et al., 2022). As noted above, a key reason for the Translational Dilemma and the existence of the Valley of Death is intrinsic biological heterogeneity and the issues it causes in terms of the Curse of Dimensionality and the resulting perpetual data sparseness in clinical data. Therefore, overcoming this data sparseness through appropriate execution of in silico trials to achieve Precision Medicine requires that the synthetic population be able to capture/replicate the heterogeneity of the clinical population. This now links to Medical Digital Twins, where the ability to simulate any individual requires the ability to simulation every individual, and thus allows the generation of a synthetic population of Medical Digital Twins that can mirror the heterogeneity within a real clinical population. Our approach to accomplishing this, while in turn dealing with the perpetual epistemic insufficiency of the knowledge underlying the mechanism-based simulation models, is through a specific construction of the simulation models that utilizes the parameter space of the models to encompass this uncertainty (Cockrell and An, 2021). This approach involves a formal mathematical object termed the Model Rule Matrix (Cockrell and An, 2021). This object relies on a model construction formalism where the parameters of the model reflect the potential connectivity (existence and potential strength) between all the represented components of the targeted biological system; in this fashion the parameterizations of the model encompass all the potential factors (be they genetic, epigenetic or environmentally influenced) that would affect the responsiveness of the represented functions in the model (which is assumed to be comprehensive). This approach towards “parameterization” focuses the functional basis of inter-individual heterogeneity and can then capture the functional/response variances known to be present in a clinical population due to the above listed host of different factors. Then, identifying the clinically-relevant parameter space of the MRM through the application of ML methods such as Active Learning (Cockrell et al., 2021), can allow the generation of a synthetic population that encompasses the functional heterogeneity seen in a clinical population and allow the design and performance of a clinically relevant in silico trial.
Once such a synthetic population is generated, there can be different goals and uses that affect the design and execution of an in silico trial. The more traditional approach is to test a putative intervention derived by the standard method of designing clinical trials [(An, 2004; Clermont et al., 2004) for early examples of this]. This use of an in silico trial essentially serves as a plausibility check to see if a presumed intervention, derived from a sequence of reductionist experiments, actually behaves as expected in a clinically-relevant systemic context [this, in fact, is one of the foundational applications of Translational Systems Biology (Vodovotz et al., 2008)]. Alternatively, if there are existing effective therapies but the goal is to optimize them in combination, these types of in silico trials can guide how such multi-modal treatments could be designed (Wang et al., 2019). Finally, as described above, for diseases where no current effective therapy exists, ML methods such as DRL can be used to pose how these diseases can be controlled (Petersen et al., 2019; Larie et al., 2022). Notably, drug repurposing is particularly well suited to this approach (Larie et al., 2022).
Conclusion: The Grand Challenges for Translational Systems Biology and In Silico Trials
“Translational Systems Biology” (or TSB) was the term coined originally in 2008 (An et al., 2008; Vodovotz et al., 2008) and subsequently expanded into the book quoted at the beginning of this paper (Vodovotz and An, 2014). TSB was developed to describe how this translational challenge could be addressed by utilizing multi-scale, mechanism-based computational modeling with an explicit goal of representing clinically relevant phenomena, including the performance of in silico clinical trials (An, 2004; Clermont et al., 2004). I believe the durability of this program is evident in that the insights that led to TSB preceded the advent of “precision/personalized” medicine, where the “translational” aspect of TSB essentially describes a roadmap to “true” precision/personalized medicine (An and Day, 2021). Similarly, the emphasis on clinically relevant models and the clinically relevant use of those models in TSB presaged the recent interest in Medical Digital Twins (which are essentially mechanism-based TSB models). In the years since TSB was introduced “Big Data” has morphed into ML and AI (at least in terms of the correlative nature of the bulk of those applications in biomedicine), and now TSB has adapted to utilize the control discovery potential of DRL to further its goal of fulfilling the Axioms of Precision Medicine. I note these facts to emphasize the persistence of the TSB roadmap in achieving the Grandest Challenge of getting better drugs to treat every patient (e.g., “True” Precision Medicine), even as TSB has uncovered issues not identified in its original conception, i.e., the insufficiency of a direct engineering paradigm, the need to deal with perpetual epistemic uncertainty in biology and the critical role of biological heterogeneity, among others. The evolution of TSB has led to insights that suggest a series of Grand Challenges that I hope will spur investigations suitable for this journal. These are:
Grand Challenge #1: How to encompass and embrace the biological heterogeneity present in clinical populations in the design of in silico clinical trials? We recognize that biological heterogeneity is a feature, not a bug, in biology. While such heterogeneity presents significant challenges during the discovery phase of basic biological research, which primarily seeks to mitigate heterogeneity in order to achieve statistically significant results, it is essential to reconstruct how such heterogeneity manifests in the real world in order to represent the functional diversity seen in clinical populations. Accounting for the mechanistic bases for clinical heterogeneity in the generation of synthetic populations for in silico trials will be key in using in silico clinical trials to cross the Valley of Death. We have proposed one pipeline towards this goal (Cockrell and An, 2021; Cockrell et al., 2021) and hope this Grand Challenge will encourage the development of additional and alternative approaches. Being able to do this is critical if one is to apply ML or AI approaches to this simulated data (see next Grand Challenge).
Grand Challenge #2: How to generate synthetic data that “obscures” the generative simulation model from ML and AI approaches? As noted previously in this paper, the fact that ANNs are Universal Approximators means that they will extract a function that underlies a data set. If that data set is a synthetic one generated by an equation-based model, then the ANN will just recapitulate that model, with attendant consequences in terms of applicability and generalizability to the real world. The addition of stochasticity to these generative models can help, but only if how that stochasticity is applied matches how biological stochasticity is generated. For instance, a standard stochastic differential equation will not suffice because the ANN will “see through” the noise term generally added to make a deterministic differential equation model into a stochastic one. Our work has shown that bio-realistic stochasticity is not a constant function (Cockrell and An, 2017; Larie et al., 2021), a fact that should be evident by examining the error bars in virtually any sufficiently large experimental or clinical data set. The ability to generate effective synthetic time series data is crucial for the overall application of ML/AI to disease forecasting and control discovery, as biological data of this type is too invariably sparse for effective, generalizable learning.
Grand Challenge #3: How to design and use Translational Systems Biology models/Medical Digital Twins to inform the next generation of clinical sensors and controllers to achieve True Precision Medicine? I assert that the mechanism-based dynamic models utilized in TSB essentially meet the criteria for the shared specification inherent in the definition of a medical digital twin. However, what is missing to meet the actual definition of a digital twin is the linkage/interface of a simulation based on that specification to a real-world data stream that allows updating of the “twinned” simulation to a particular individual. It should be noted that the development of such sensors and controllers will provide the data that can falsify/invalidate and improve the underlying simulation models, resulting in an intrinsically iterative process that will enhance the trust in these systems.
Grand Challenge #4: How to integrate mechanism-based Translational Systems Biology simulation models with cutting edge developments in ML and AI to further control discovery and achieve the Axioms of Precision Medicine? The utility of modern ML and AI in furthering biomedical research is undeniable, but there are hard limits of those tools when it comes to crossing the Valley of Death, specifically noted in the Causal Hierarchy Theorem (Xia et al., 2021). We have proposed one method to integrate mechanism-based simulation with DRL/AI to foster control discovery (Petersen et al., 2019; Larie et al., 2022), and hope to see more researcher to develop additional methods that can leverage the benefits of both methods. As noted above in Grand Challenges #1 and #2, all of this is predicated upon being able to generate realistic synthetic data.
The vision and the promise of Translational Systems Biology can be seen in Figure 2.
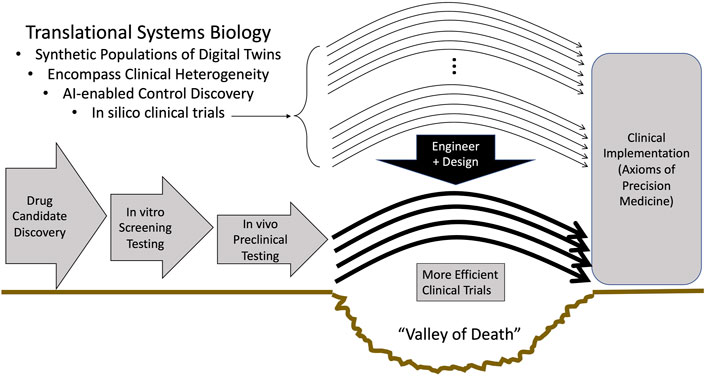
FIGURE 2. The role of Translational Systems Biology in enhancing the efficiency and success of clinical trials. The successful development of methods and approaches to address the Grand Challenges of: Enhanced development of mechanism-based simulations as specifications for digital twins, replicating clinical heterogeneity in synthetic populations, utilizing AI for control discovery, can lead to the use of in silico trials within an engineering paradigm of design and pretesting that can bring to biomedicine the same advances present in other technological fields.
As noted at the beginning of this editorial, the content presented herein is a compilation of my perspectives and experiences in the pursuit of finding better drugs to treat my patients; it is a journey that has lasted over 20 years and taken directions I could never have anticipated. This editorial is not intended to be a comprehensive review of the literature and is not exclusionary in intent; rather it is intended to illustrate the (my) rationale for a particular roadmap to address what I consider to be the key deficiency in modern biomedicine: increasing the effective translation of basic science knowledge into clinically effective therapeutics. I believe that the Challenges presented in this editorial represent necessary steps to move towards a goal of “true” Precision Medicine, but I also recognize that there are often multiple paths to getting to the same goal (much like biology itself). I look forward to seeing the work of those who take up the Challenges I offer, and especially those who challenge the tenets I present in order to expand the Universe of solutions to this Grandest of Challenges.
Author Contributions
GA is the sole author, having conceived, written and edited this manuscript.
Funding
This work was supported in part by the National Institutes of Health Award UO1EB025825. This research is also sponsored in part by the Defense Advanced Research Projects Agency (DARPA) through Cooperative Agreement D20AC00002 awarded by the U.S. Department of the Interior (DOI), Interior Business Center. The content of the information does not necessarily reflect the position or the policy of the Government, and no official endorsement should be inferred.
Conflict of Interest
The author declares that the research was conducted in the absence of any commercial or financial relationships that could be construed as a potential conflict of interest.
The handling editor YV declared a past collaboration with the author.
Publisher’s Note
All claims expressed in this article are solely those of the authors and do not necessarily represent those of their affiliated organizations, or those of the publisher, the editors and the reviewers. Any product that may be evaluated in this article, or claim that may be made by its manufacturer, is not guaranteed or endorsed by the publisher.
Acknowledgments
I would like to thank my colleagues Yoram Vodovotz (Journal Chief Editor), my co-developer of the concept of Translational Systems Biology, and Chase Cockrell.
References
An, G. (2010). Closing the Scientific Loop: Bridging Correlation and Causality in the Petaflop Age. Sci. Transl Med. 2 (41), 41ps34. doi:10.1126/scitranslmed.3000390
An, G. (2008). Introduction of an Agent-Based Multi-Scale Modular Architecture for Dynamic Knowledge Representation of Acute Inflammation. Theor. Biol. Med. Model. 5 (1), 11–20. doi:10.1186/1742-4682-5-11
An, G., and Day, J. (2021). Precision Systems Medicine: A Control Discovery Problem. In Systems Medicine: Integrative, Qualitative and Computational Approaches. Editors W. Olaf (Oxford: Elsevier). 3, 318–330. doi:10.1016/B978-0-12-801238-3.11513-2
An, G., Faeder, J., and Vodovotz, Y. (2008). Translational Systems Biology: Introduction of an Engineering Approach to the Pathophysiology of the Burn Patient. J. Burn Care Res. 29 (2), 277–285. doi:10.1097/bcr.0b013e31816677c8
An, G., Fitzpatrick, B. G., Christley, S., Federico, P., Kanarek, A., Neilan, R. M., et al. (2017). Optimization and Control of Agent-Based Models in Biology: A Perspective. Bull. Math. Biol. 79 (1), 63–87. doi:10.1007/s11538-016-0225-6
An, G. (2004). In Silico experiments of Existing and Hypothetical Cytokine-Directed Clinical Trials Using Agent-Based Modeling*. Crit. Care Med. 32 (10), 2050–2060. doi:10.1097/01.ccm.0000139707.13729.7d
An, G. (2018). The Crisis of Reproducibility, the Denominator Problem and the Scientific Role of Multi-Scale Modeling. Bull. Math. Biol. 80 (12), 3071–3080. doi:10.1007/s11538-018-0497-0
Bekiari, E., Kitsios, K., Thabit, H., Tauschmann, M., Athanasiadou, E., Karagiannis, T., et al. (2018). Artificial Pancreas Treatment for Outpatients with Type 1 Diabetes: Systematic Review and Meta-Analysis. BMJ 361, k1310. doi:10.1136/bmj.k1310
Butler, D. (2008). Translational Research: Crossing the Valley of Death. Nature 453 (7197), 840–842. doi:10.1038/453840a
Chen, L. (2009). “Curse of Dimensionality,” in Encyclopedia of Database Systems. Editors L. Liu, and M. T. ÖZsu (Boston, MA: Springer US), 545–546. doi:10.1007/978-0-387-39940-9_133
Chousterman, B. G., Swirski, F. K., and Weber, G. F. (2017). Cytokine Storm and Sepsis Disease Pathogenesis. Semin. Immunopathol. 39, 517. Springer. doi:10.1007/s00281-017-0639-8
Clermont, G., Bartels, J., Kumar, R., Constantine, G., Vodovotz, Y., and Chow, C. (2004). In Silico design of Clinical Trials: A Method Coming of Age. Crit. Care Med. 32 (10), 2061–2070. doi:10.1097/01.ccm.0000142394.28791.c3
Cockrell, C., and An, G. (2017). Sepsis Reconsidered: Identifying Novel Metrics for Behavioral Landscape Characterization with a High-Performance Computing Implementation of an Agent-Based Model. J. Theor. Biol. 430, 157–168. doi:10.1016/j.jtbi.2017.07.016
Cockrell, C., and An, G. (2021). Utilizing the Heterogeneity of Clinical Data for Model Refinement and Rule Discovery through the Application of Genetic Algorithms to Calibrate a High-Dimensional Agent-Based Model of Systemic Inflammation. Front. Physiol. 12, 662845. doi:10.3389/fphys.2021.662845
Cockrell, C., Ozik, J., Collier, N., and An, G. (2021). Nested Active Learning for Efficient Model Contextualization and Parameterization: Pathway to Generating Simulated Populations Using Multi-Scale Computational Models. Simulation 97 (4), 287–296. doi:10.1177/0037549720975075
D'Amour, A., Heller, K., Moldovan, D., Adlam, B., Alipanahi, B., Beutel, A., et al. (2020). Underspecification Presents Challenges for Credibility in Modern Machine Learning. arXiv preprint arXiv:201103395.
Grieves, M. W. (2019). Virtually Intelligent Product Systems: Digital and Physical Twins. Reston, VA: American Institute of Aeronautics and Astronautics.
Hornik, K., Stinchcombe, M., and White, H. (1989). Multilayer Feedforward Networks Are Universal Approximators. Neural Networks 2 (5), 359–366. doi:10.1016/0893-6080(89)90020-8
Jeter, R., Josef, C., Shashikumar, S., and Nemati, S. (2019). Does the “Artificial Intelligence Clinician” Learn Optimal Treatment Strategies for Sepsis in Intensive Care? arXiv preprint arXiv:190203271.
Jin, X., Bighamian, R., and Hahn, J-O. (2018). Development and In Silico Evaluation of a Model-Based Closed-Loop Fluid Resuscitation Control Algorithm. IEEE Trans. Biomed. Eng. 66 (7), 1905–1914. doi:10.1109/TBME.2018.2880927
Jonas, E., and Kording, K. P. (2017). Could a Neuroscientist Understand a Microprocessor? Plos Comput. Biol. 13 (1), e1005268. doi:10.1371/journal.pcbi.1005268
Jumper, J., Evans, R., Pritzel, A., Green, T., Figurnov, M., Ronneberger, O., et al. (2021). Highly Accurate Protein Structure Prediction with AlphaFold. Nature 596 (7873), 583–589. doi:10.1038/s41586-021-03819-2
Komorowski, M., Celi, L. A., Badawi, O., Gordon, A. C., and Faisal, A. A. (2018). The Artificial Intelligence Clinician Learns Optimal Treatment Strategies for Sepsis in Intensive Care. Nat. Med. 24 (11), 1716–1720. doi:10.1038/s41591-018-0213-5
Larie, D., An, G., and Cockrell, R. C. (2021). The Use of Artificial Neural Networks to Forecast the Behavior of Agent-Based Models of Pathophysiology: An Example Utilizing an Agent-Based Model of Sepsis. Frontiers in Physiology 12. doi:10.3389/fphys.2021.716434
Larie, D., An, G., and Cockrell, C. (2022). Preparing for the Next COVID: Deep Reinforcement Learning Trained Artificial Intelligence Discovery of Multi-Modal Immunomodulatory Control of Systemic Inflammation in the Absence of Effective Anti-Microbials. bioRxiv. doi:10.1101/2022.02.17.480940
Lillicrap, T. P., Hunt, J. J., Pritzel, A., Heess, N., Erez, T., Tassa, Y., et al. (2015). Continuous Control with Deep Reinforcement Learning. arXiv preprint arXiv:150902971.
Novati, G., de Laroussilhe, H. L., and Koumoutsakos, P. (2021). Automating Turbulence Modelling by Multi-Agent Reinforcement Learning. Nat. Mach. Intell. 3 (1), 87–96. doi:10.1038/s42256-020-00272-0
Pathak, J., Hunt, B., Girvan, M., Lu, Z., and Ott, E. (2018). Model-Free Prediction of Large Spatiotemporally Chaotic Systems from Data: A Reservoir Computing Approach. Phys. Rev. Lett. 120 (2), 024102. doi:10.1103/PhysRevLett.120.024102
Petersen, B. K., Yang, J., Grathwohl, W. S., Cockrell, C., Santiago, C., An, G., et al. (2019). Deep Reinforcement Learning and Simulation as a Path Toward Precision Medicine. J. Comput. Biol. 26 (6), 597–604. doi:10.1089/cmb.2018.0168
Silver, D., Hubert, T., Schrittwieser, J., Antonoglou, I., Lai, M., Guez, A., et al. (2018). A General Reinforcement Learning Algorithm that Masters Chess, Shogi, and Go Through Self-Play. Science 362 (6419), 1140–1144. doi:10.1126/science.aar6404
Silver, D., Schrittwieser, J., Simonyan, K., Antonoglou, I., Huang, A., Guez, A., et al. (2017). Mastering the Game of Go without Human Knowledge. Nature 550 (7676), 354–359. doi:10.1038/nature24270
Villaescusa-Navarro, F., Anglés-Alcázar, D., Genel, S., Spergel, D. N., S. Somerville, R., Dave, R., et al. (2021). The CAMELS Project: Cosmology and Astrophysics with Machine-Learning Simulations. ApJ 915 (1), 71. doi:10.3847/1538-4357/abf7ba
Vinyals, O., Babuschkin, I., Czarnecki, W. M., Mathieu, M., Dudzik, A., Chung, J., et al. (2019). Grandmaster Level in StarCraft II Using Multi-Agent Reinforcement Learning. Nature 575 (7782), 350–354. doi:10.1038/s41586-019-1724-z
Vodovotz, Y., and An, G. (2014). Translational Systems Biology: Concepts and Practice for the Future of Biomedical Research. Waltham, MA: Elsevier.
Vodovotz, Y., Csete, M., Bartels, J., Chang, S., and An, G. (2008). Translational Systems Biology of Inflammation. Plos Comput. Biol. 4 (4), e1000014. doi:10.1371/journal.pcbi.1000014
Wang, H., Milberg, O., Bartelink, I. H., Vicini, P., Wang, B., Narwal, R., et al. (2019). In Silico Simulation of a Clinical Trial with Anti-CTLA-4 and Anti-PD-L1 Immunotherapies in Metastatic Breast Cancer Using a Systems Pharmacology Model. R. Soc. Open Sci. 6 (5), 190366. doi:10.1098/rsos.190366
Wright, L., and Davidson, S. (2020). How to Tell the Difference Between a Model and a Digital Twin. Adv. Model. Simulation Eng. Sci. 7 (1), 1–13. doi:10.1186/s40323-020-00147-4
Xia, , , Lee, K., Bengio, K.-Z., Bareinboim, Y., and Elias, (2021). The Causal-Neural Connection: Expressiveness, Learnability, and Inference. Advances in Neural Information Processing Systems. Editors M. Ranzato, A. Beygelzimer, K. Nguyen, P. S. Liang, J. W. Vaughan, and Y. Dauphin. Curran Associates, Inc.. 34, 10823–10836. https://proceedings.neurips.cc/paper/2021/file/5989add1703e4b0480f75e2390739f34-Paper.pdf.
Keywords: in silico trials, multiscale modeling, machine learning, artificial intelligence, digital twin, agent based modeling (ABM), precision medicine, personalized medicine
Citation: An G (2022) Specialty Grand Challenge: What it Will Take to Cross the Valley of Death: Translational Systems Biology, “True” Precision Medicine, Medical Digital Twins, Artificial Intelligence and In Silico Clinical Trials. Front. Syst. Biol. 2:901159. doi: 10.3389/fsysb.2022.901159
Received: 21 March 2022; Accepted: 24 March 2022;
Published: 28 April 2022.
Edited and reviewed by:
Yoram Vodovotz, University of Pittsburgh, United StatesCopyright © 2022 An. This is an open-access article distributed under the terms of the Creative Commons Attribution License (CC BY). The use, distribution or reproduction in other forums is permitted, provided the original author(s) and the copyright owner(s) are credited and that the original publication in this journal is cited, in accordance with accepted academic practice. No use, distribution or reproduction is permitted which does not comply with these terms.
*Correspondence: Gary An, Z2FuQG1lZC51dm0uZWR1, ZG9jZ2NhQGdtYWlsLmNvbQ==