- 1Metabolic Engineering and Systems Biology Laboratory, Institute of Chemical Engineering Sciences, Foundation for Research and Technology-Hellas (FORTH/ICE-HT), Patras, Greece
- 2Department of Chemical Engineering, University of Patras, Patras, Greece
Genome-scale metabolic network models are of great importance in systems biology research, as they are used in metabolic activity dynamics studies and provide the metabolic level representation in multi-omic investigations. Especially for human, accurate metabolic network reconstruction is important in biomedical research and drug discovery. Today, there exist many instances of the human metabolic network as a whole and in its tissue-specific versions. Some are improved updates of models reconstructed from the same research team, while others are combinations of models from various teams, in an effort to include all available information from genome annotation and omic datasets. A major challenge regarding the human stoichiometric models in particular is the standardization of the reconstruction methods, representation formats and model repositories. Stoichiometric model standardization will enable the educated selection of the model that better fits the goals of a study, the direct comparison of results from various flux analysis studies and the identification of model sections that require reconsideration and updating with respect to the annotation of the human genome and proteome. Standardized human metabolic models aligned to the human genome will be a very useful tool in multi-omic studies, enabling the direct and consistent integration of the metabolic with the gene regulation and protein interaction networks. In this work, we provide a thorough overview of the current collection of human metabolic stoichiometric models, describe the current issues regarding their direct comparison and alignment in the context of the various model repositories, exposing the standardization needs, and propose potential solutions.
The Importance of Metabolic Modeling in Systems Biology
In systems biology, cellular physiology is viewed as a complex dynamic biomolecular network of interacting genes, transcripts, proteins, and metabolites. The interplay of biomolecular networks describes the relationship between genotype and phenotype (Oberhardt et al., 2009). These networks are subjected to multi-scale regulatory mechanisms (Aon, 2014), enabling the cell to optimize its resources, to carry out its functions in an efficient manner, to interact with its environment and respond to any perturbations exhibiting adaptability and robustness (Barabási and Oltvai, 2004; Abedpour and Kollmann, 2015). Thus, the individual and integrated analysis of these networks is essential for understanding the underlying mechanisms characterizing a particular (patho)-physiology.
Metabolism is a significant biological process, producing all biomolecules and energy for the cellular functions, while also interacting with the extracellular environment. The metabolic pathways are series of enzyme-catalyzed reactions of certain stoichiometry. Metabolites are also regulatory molecules of proteins, while the metabolic activity is affected by changes in all other molecular levels of cellular function, genomic, transcriptional and translational (Nielsen, 2017b). Thus, elucidating the structure and regulation of metabolic pathways is an essential objective of systems biology for obtaining a comprehensive perspective of cellular function (Vasilopoulou et al., 2016; Masid and Hatzimanikatis, 2021). Especially for human, metabolic modeling is essential for the development of methodologies for accurate and sensitive diagnosis, the design of drugs, therapeutic treatments, nutrition and exercise regimes, and the advancement of cell and tissue engineering.
Genome-scale metabolic network reconstruction takes collectively advantage of the genome annotation and omic data to identify the potentially active reactions inside a cell, and of metabolic reaction stoichiometry information to “fill any gaps” due to incomplete annotation (Thiele and Palsson 2010). Systemic analysis of the in vivo activity and regulation of metabolic networks is carried out based on data from metabolic profiling (metabolomics), quantifying free metabolite abundances. This analysis does not require extensive knowledge of the metabolic network, on the contrary, it contributes data for the network reconstruction, and can be applied under transient physiological conditions too (Nielsen 2017b). However, comparison of metabolic profiles can provide mainly qualitative information about the in vivo metabolic activity and pathway fluxes. The flux distribution in a metabolic network provides a measure of the degree of engagement of the various pathways (Klapa, 2009). Comparative analysis of the metabolic flux distribution under various physiological conditions can reveal metabolic regulatory mechanisms and indicate the optimized direction for genetic modifications. However, an accurate representation of the metabolic reaction network is an essential part of metabolic modeling, along with comprehensive kinetic information, in the case of kinetic models. Extensive kinetic models are not readily available for large complex networks, especially in human, but rather for targeted well-studied small-scale networks, for which in vivo kinetic information is accessible or at least good approximations can be made. The stoichiometric metabolic models bypass this issue, based only on the balancing of fluxes in and out of the metabolite pools according to the stoichiometry of the metabolic reactions. Thus, the internal fluxes are estimated by the external metabolite net excretion rates based on the stoichiometric (or metabolite) balances.
A major challenge regarding the stoichiometric models of metabolic networks in general, and in human in particular, is the standardization of the reconstruction methods, the representation formats and the model repositories. At the moment, direct comparison between models is not possible, hindering the selection of the most appropriate model for a particular application, and it is not clear how the human metabolic network reconstruction evolves. In this perspective, we support the importance of the standardization of stoichiometric metabolic models, focusing on human metabolism. We provide an overview of the current collection of human metabolic stoichiometric models, describe the issues regarding their direct comparison and alignment, exposing the standardization needs, and propose potential solutions.
Stoichiometric Modeling of Metabolic Network Activity
Metabolic flux analysis based on stoichiometric models of comprehensive metabolic networks was developed in the 1980s as a methodology of metabolic engineering to analyze the metabolic network activity and regulation and identify targets of genetic modification, mainly in the context of industrial microbial biotechnology (Stephanopoulos et al., 1998). Later, it was expanded to cell culture engineering in pharmaceutical industry (e.g. Goudar et al., 2014) and biomedical research, as holistic network-wide analyses were promoted in the context of systems biology and multi-omic studies and information for the metabolic network reconstruction was becoming available from genome sequencing and annotation (Nielsen, 2017a).
The stoichiometric model of a given metabolic network is described by the system of the metabolite balance equations. At metabolic steady- (or pseudo-steady-) state conditions, at which metabolite balancing analysis is usually applied, fluxes are estimated as the solution of a weighted least-squares problem on the measured external metabolite net excretion rates based on the stoichiometric and flux boundary constraints:
where
Reconstruction and Validation of Genome-Scale Stoichiometric Models
When genomic information was not available, metabolic network reconstruction was mainly based on biochemical information about major pathways and enzyme characterization along with experimental information about the substrates and products of the particular biological system/organism under various growth conditions and targeted genetic modifications. In this case, data reconciliation analysis through redundancies was a way to identify inconsistencies in the metabolic network reconstruction and discover new pathways. In the post-genomic era, genome annotation contributes to the genome-scale metabolic network reconstruction of the target organism (Thiele and Palsson, 2010). The nonlinear genetic information flow between genome, transcriptome and proteome, where one gene may correspond to multiple proteins and vice versa adds to the complexity of metabolic network reconstruction of complex organisms, and the human in particular. Obviously, compartmentalized and tissue-specific metabolic networks are an additional challenge for eukaryotic and multi-tissue organisms, respectively. Metabolic models should follow the updates of the genome, transcriptome and proteome collections. As genome annotations are not complete, genome-scale metabolic network reconstructions have usually “gaps” that are filled based on biochemical knowledge. Most common issues are the “dead-end” metabolites, considered to be intracellular, which have only producing or only consuming reactions, and the “orphan” reactions that are known or expected to exist, but the respective gene(s) has/have not been identified on the genome. Orphan, transport and spontaneous reactions implied from metabolic activity data and experimental observations are then added to the reconstruction to ensure that the model is functional for optimization analyses. Still, genome annotation cannot provide direct information about the direction and reversibility of a reaction nor about its cellular or tissue localization. To gain further insight for these, integration with enzymatic and metabolic databases is necessary along with available omic data at various molecular levels of cellular function. Omic data can be used for the gap-filling process, the validation of the current metabolic models, and the (re-) annotation of genes and their cellular and tissue localization. Consistent integration of omic data in the metabolic network reconstruction requires alignment between the genome and the metabolic model. Algorithms modifying generic metabolic models into tissue-specific based on omic data have been proposed in the literature (e.g. Zur et al., 2010; Agren et al., 2012; Wang et al., 2012; Heirendt et al., 2019). However, in tissue-specific model reconstructions, there are open questions about the transport reactions from and into the tissue, about which molecules are produced in other tissues and become available to the particular tissue only through the blood and about the reversibility of the metabolic reactions (e.g. Shlomi et al., 2008; Jerby et al., 2010). To date, there are issues with the harmonization and interoperability between different metabolic reconstructions of the same target organism or tissue that limit their comparability, and with the regular model updating along with the evolution of genome annotation. Below, we focus on the current challenges regarding the human metabolic stoichiometric models.
Human Metabolic Stoichiometric Models and Reactomes
The first genome-scale human metabolic stoichiometric models were published in 2007 by two separate efforts: the Edinburgh Human Metabolic Network (EHMN, Ma et al., 2007) and Recon1 (Duarte et al., 2007). These reconstructions served as basis for the reconstruction of metabolic networks of other organisms, such as mouse and rat, through gene orthologues. The human metabolic models have evolved through different instances and also tissue-specific reconstructions became available (Ferreira et al., 2021). An overview of the human metabolic model landscape today led to the reconstruction of the evolution and connectivity map between the various human models since 2007, which is shown in Figure 1. As depicted, some models have evolved through different versions, while some others started from the combination of existing reconstructions along with omic and other biological data. Actually, there are four main reconstruction lines: 1) the EHMN, which stopped its updates in 2010 after the compartmentalized model, 2) the Recon series, which has evolved from Recon1 to Recon3D (Brunk et al., 2018), while its Recon 2.02 version (Thiele et al., 2013) formed the basis for 3) the Recon 2.1–2.2 reconstructions (Smallbone, 2013; Swainston et al., 2016), and 4) the HMR (Human Metabolic Reaction) models (Agren et al., 2012; Mardinoglu et al., 2013; 2014), which evolved to Human1 in 2020 after inclusion of tissue-specific reconstructions (Robinson et al., 2020). The human metabolic models are available from different portals, as the consortia behind the main reconstruction lines have developed their own resource (Figure 2A). Recon1 and Recon3D are available from BiGG Models (King et al., 2016), which includes reconstructions from other organisms too, mainly microbial. Virtual Metabolic Human (VMH) (Noronha et al., 2019) hosts Recon 2.02–2.04 (Haraldsdóttir et al., 2014) and Recon3D models. The HMR series and Human 1 are available from Metabolic Atlas (Robinson et al., 2020), which contains also reconstructions of yeast, fruitfly, mouse, rat, zebrafish and worm metabolic networks. All portals allow users to access and download the included instances. However, each portal provides different formats, hindering the direct model comparison. Most popular formats are XML and SBML (Figure 2B). SBML has been accepted as the default language for biochemical reactions by consortia promoting community-led standardization efforts in computational biology, such as the European Infrastructure ELIXIR (https://elixir-europe.org/) and the Computational Modeling in Biology Network (COMBINE; https://co.mbine.org).
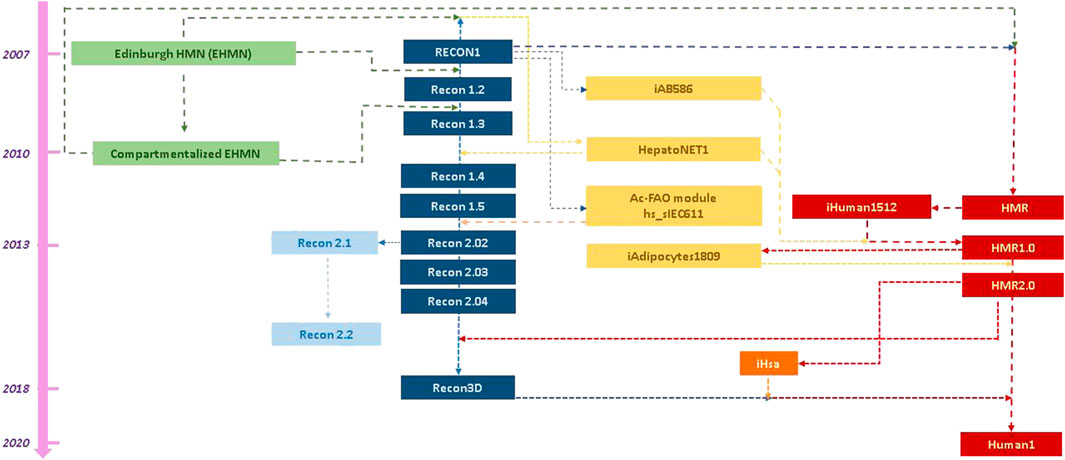
FIGURE 1. Literature-deduced schematic representation of the evolution of and interconnectivity between the main reconstruction lines of human stoichiometric models. Literature search indicated four main reconstruction lines of the generic model, depicted, respectively, in green, blue, light blue and red boxes, aligned chronologically based on the respective publication(s). Yellow boxes are used for the tissue-specific reconstructions, which were derived and/or have been incorporated in the generic stoichiometric models. Orange box is used to depict a model from a distinct research team from the main four, which had not published a human metabolic stoichiometric model before. The arrows and colors of the lines between the boxes indicate the “information flow” between the models.
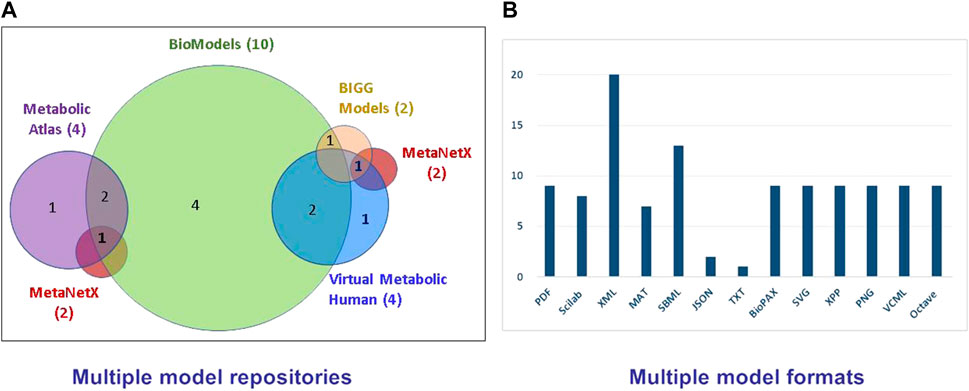
FIGURE 2. (A) The presently available repositories of human metabolic stoichiometric models and their model intersections and (B) the distribution of the various model formats. A human stoichiometric metabolic model may be available in multiple repositories and multiple formats, confounding its consistent and comparable use.
Recently, vaster repositories were developed, aiming at collecting metabolic models from different resources and organisms, and storing them in a common standardized way, enabling their direct comparison and interoperability. Main resource of this type is the EMBL-EBI BioModels (Glont et al., 2018; Malik-Sheriff et al., 2020), which stores both kinetic and stoichiometric models. Biomodels requires for a model to be uploaded in SBML format and other formats are automatically generated from the original submission, so that the model can be accessible by different bioinformatic tools. So far, the BioModels team has undertaken the standardization task for the kinetic models, however, standardization and thus direct comparability is not yet available for the stoichiometric models, including human. The need for stoichiometric model standardization has been largely discussed in relevant scientific communities and consortia. BioModels includes the EHMN (original and compartmentalized), Recon 1, 2.02–2.03, 2.1–2.2 and all HMR models (Figure 2A). The standardized SIB (Swiss Institute of Bioinformatics) MetaNetX model repository and analysis tool (Moretti et al., 2021) includes HMR2.0 and Recon3D models and the expert curated human metabolic reactome of the extended metabolic database, Rhea (Alcántara et al., 2012). Rhea includes the metabolic reactions, which are likely to occur in the human cell, based on the human proteome annotation as defined in UniProt and the enzyme-reaction relationships defined by the Nomenclature Committee of the IUBMB (NC-IUBMB) (https://iubmb.qmul.ac.uk/), as stored in ExplorEnz database (McDonald et al., 2009). The chemical species (metabolites) are extracted from the standardized database ChEBI (Hastings et al., 2016). Using a standardized identifier scheme enables MetaNetX to consistently store metabolic models and reactomes, so that they are readily comparable and interoperable.
Traditionally, human metabolic reactome collections have been available through metabolic databases, with most popular the Kyoto Encyclopedia of Genes and Genomes (KEGG) (Kanehisa et al., 2019), HumanCyc, part of the BioCyc metabolic database collection (Karp and Caspi, 2011), the expert curated Reactome Knowledgebase (Gillespie et al., 2022) and Rhea. All collections refer to the human genome annotation and connect metabolic reactions to genes, transcripts, enzymes, chemical compounds and localization information through database cross-referencing. These collections do not directly constitute balanced stoichiometric models, gap-filled with transport and “technical” reactions to enable their use in flux balance optimization studies. However, HumanCyc, which runs pathway prediction tools based on genome annotation, adds transport reactions, and fills pathway gaps that are not yet supported by the human genome. Transport reactions are also included in the Reactome Knowledgebase and Rhea through MetaNetX to facilitate their use in stoichiometric modeling.
Standardization Challenges in Human Stoichiometric Modeling
The overview of the available human metabolic stoichiometric models and reactome collections indicates a plurality of reconstructions and utilized formats, which are not directly comparable and interoperable. This situation may confuse a user as to which reconstruction is the most suitable for a particular application, as there is no clear information of their differences. Moreover, instances of the same model in different resources and formats might not be identical. Comparison of the same reconstruction (Recon1) between different formats and resources with respect to the number of the included metabolites indicated that this could indeed be the case for some models (Supplementary Table S1). Then, we compared various models in MATLAB format with respect to the number of included reaction IDs; each model was collected from the main resource of the respective reconstruction line (Supplementary Figure S1). We observed a small overlap between the reconstructions, which cannot be attributed solely to the inclusion of different reactions, but, and potentially mainly, to the use of different identifiers for the same reactions between the models. Each of the main reconstruction series shown in Figure 1 tends to use its own IDs. The problem with different identifiers between models was also indicated in a recent thorough comparison of the SBML files of Recon and HMR series, HepatoNet and EHMN models (Vieira et al., 2018). In addition, some models include the Ensembl gene IDs of the involved enzymes instead of their Enzyme Commission (EC) number, further hindering their direct comparison, while alignment to more recent genome annotations may be necessary. It becomes apparent that standardized ID systems and direct connection of enzyme identifiers to the genome annotation are of value for the comparability and interoperability of the various stoichiometric models and their consistent updating along with new genomic information. The MetaNetX framework succeeds towards this direction using the standardized schema of Rhea database. BridgeDb (van Iersel et al., 2010) is a tool that enables the mapping between various biological databases, providing the ontological framework for the direct comparison between models and reactomes.
Challenges of the metabolic network reconstruction for specific tissues and compartments remain the reversibility and localization of certain reactions, and the transport of certain metabolites through membranes (Lewis et al., 2014). The direction of the transport reactions is also an issue. Incorporation of omic, and when possible, isotopic labeling data into the models could enhance our knowledge in these matters. Reconstruction of secondary metabolism could be challenging too. Balancing both sides of a reaction is relatively straightforward for most reactions in primary metabolism, however, it may be challenging for pathways involving macromolecules with unspecified number of carbon atoms. To avoid errors related to limited knowledge of certain parts of secondary metabolism, stoichiometric models may be occasionally simplified by lumping secondary metabolism into one biomass equation, which stoichiometrically connects primary metabolism intermediates with biomass constituents. Defining the biomass equation in human generic and tissue-specific models has not yet been a standardized process.
Conclusion and Future Directions
Standardization of human stoichiometric models is necessary as it will enable the consistent integration of metabolomic and metabolic flux data with other omic and biological data. Currently, there exist many human metabolic network reconstructions in various repositories and multiple formats, using different identifiers and schemas that hinder their direct comparability and interoperability, while they cannot be readily updated along with the genome annotation. Standardization will enable the educated selection of the model that better fits the goals of a study and the direct comparison of results from various studies. The use of standardized IDs will enable the alignment of the existing instances with biological databases, including genome annotation resources. In this way, we could identify sections of the metabolic models that need reconsideration or updating based on the genome annotation evolution, while, on the other hand highlighting gene functions that need re-evaluation, incorporating thus metabolic knowledge into functional genomics. Finally, standardization of human metabolic stoichiometric models is expected to consistently add metabolomics and especially fluxomics in the systems biology and medicine toolbox.
Data Availability Statement
The original contributions presented in the study are included in the article/Supplementary Material, further inquiries can be directed to the corresponding author.
Author Contributions
MIK conceptualized the perspective and supervised the work; MDAP and MIK designed the review; MDAP carried out the literature and data/model resource review; MDAP and MIK contributed towards the proposed directions; MDAP drafted and MIK edited and finalized the manuscript; MIK attracted the funding for this work.
Funding
This work was supported mainly by the project “ELIXIR-GR: Hellenic Research Infrastructure for the Management and Analysis of Data from the Biological Sciences” (MIS 5002780), and ELIXIR “Standardizing the fluxomic workflows” Implementation Study. Partial funding was also provided by Stavros Niarchos Foundation (FORTH/ICE-HT ARCHERS project), the project “Infrastructure for preclinical and early-phase clinical development of drugs, therapeutics and biomedical devices” (MIS 5028091) and the European Commission H2020 Framework Program projects: SC1-BHC-07-2019 JointPromise (Complex joint implants to prevent osteoarthritis; Grant Agreement ID: 874837) and DT-ICT-12-2020 AIDPATH (AI for smart therapy provision in hospitals; Grant Agreement ID: 874837).
Conflict of Interest
The authors declare that the research was conducted in the absence of any commercial or financial relationships that could be construed as a potential conflict of interest.
Publisher’s Note
All claims expressed in this article are solely those of the authors and do not necessarily represent those of their affiliated organizations, or those of the publisher, the editors and the reviewers. Any product that may be evaluated in this article, or claim that may be made by its manufacturer, is not guaranteed or endorsed by the publisher.
Supplementary Material
The Supplementary Material for this article can be found online at: https://www.frontiersin.org/articles/10.3389/fsysb.2022.899980/full#supplementary-material
References
Abedpour, N., and Kollmann, M. (2015). Resource Constrained Flux Balance Analysis Predicts Selective Pressure on the Global Structure of Metabolic Networks. BMC Syst. Biol. 9, 88. doi:10.1186/s12918-015-0232-5
Agren, R., Bordel, S., Mardinoglu, A., Pornputtapong, N., Nookaew, I., and Nielsen, J. (2012). Reconstruction of Genome-Scale Active Metabolic Networks for 69 Human Cell Types and 16 Cancer Types Using INIT. PLOS Comput. Biol. 8, e1002518. doi:10.1371/journal.pcbi.1002518
Alcántara, R., Axelsen, K. B., Morgat, A., Belda, E., Coudert, E., Bridge, A., et al. (2012). Rhea-a Manually Curated Resource of Biochemical Reactions. Nucleic Acids Res. 40, D754–D760. doi:10.1093/nar/gkr1126
Aon, M. A. (2014). Complex Systems Biology of Networks: The Riddle and the Challenge. Syst. Biol. Metabolic Signal. Networks, Springer Ser. Biophysics 16, 19–35. doi:10.1007/978-3-642-38505-6_2
Barabási, A.-L., and Oltvai, Z. N. (2004). Network Biology: Understanding the Cell's Functional Organization. Nat. Rev. Genet. 5, 101–113. doi:10.1038/nrg1272
Brunk, E., Sahoo, S., Zielinski, D. C., Altunkaya, A., Dräger, A., Mih, N., et al. (2018). Recon3D Enables a Three-Dimensional View of Gene Variation in Human Metabolism. Nat. Biotechnol. 36, 272–281. doi:10.1038/nbt.4072
Duarte, N. C., Becker, S. A., Jamshidi, N., Thiele, I., Mo, M. L., Vo, T. D., et al. (2007). Global Reconstruction of the Human Metabolic Network Based on Genomic and Bibliomic Data. Proc. Natl. Acad. Sci. 104 (6), 1777–1782. doi:10.1073/pnas.0610772104
Ferreira, J., Vieira, V., and Rocha, M. (2021). Genome-Scale Metabolic Models. Syst. Med. 2, 420–428. doi:10.1016/B978-0-12-801238-3.11514-4
Foguet, C., Jayaraman, A., Marin, S., Selivanov, V. A., Moreno, P., Messeguer, R., et al. (2019). p13CMFA: Parsimonious 13C Metabolic Flux Analysis. PLOS Comput. Biol. 15, e1007310. doi:10.1371/journal.pcbi.1007310
Gillespie, M., Jassal, B., Stephan, R., Milacic, M., Rothfels, K., Senff-Ribeiro, A., et al. (2022). The Reactome Pathway Knowledgebase 2022. Nucleic Acids Res. 50, D687–D692. doi:10.1093/nar/gkab1028
Glont, M., Nguyen, T. V. N., Graesslin, M., Hälke, R., Ali, R., Schramm, J., et al. (2018). BioModels: Expanding Horizons to Include More Modelling Approaches and Formats. Nucleic Acids Res. 46, D1248–D1253. doi:10.1093/nar/gkx1023
Goudar, C. T., Biener, R. K., Piret, J. M., and Konstantinov, K. B. (2014). Metabolic Flux Estimation in Mammalian Cell Cultures. Methods Mol. Biol. 1104, 193–209. doi:10.1007/978-1-62703-733-4_13
Haraldsdóttir, H. S., Thiele, I., and Fleming, R. M. (2014). Comparative Evaluation of Open Source Software for Mapping between Metabolite Identifiers in Metabolic Network Reconstructions: Application to Recon 2. J. Cheminform. 6, 2. doi:10.1186/1758-2946-6-2
Hastings, J., Owen, G., Dekker, A., Ennis, M., Kale, N., Muthukrishnan, V., et al. (2016). ChEBI in 2016: Improved Services and an Expanding Collection of Metabolites. Nucleic Acids Res. 44, D1214–D1219. doi:10.1093/nar/gkv1031
Heirendt, L., Arreckx, S., Pfau, T., Mendoza, S. N., Richelle, A., Heinken, A., et al. (2019). Creation and Analysis of Biochemical Constraint-Based Models Using the COBRA Toolbox v.3.0. Nat. Protoc. 14, 639–702. doi:10.1038/s41596-018-0098-2
Jerby, L., Shlomi, T., and Ruppin, E. (2010). Computational Reconstruction of Tissue‐specific Metabolic Models: Application to Human Liver Metabolism. Mol. Syst. Biol. 6, 401–409. doi:10.1038/msb.2010.56
Kanehisa, M., Sato, Y., Furumichi, M., Morishima, K., and Tanabe, M. (2019). New Approach for Understanding Genome Variations in KEGG. Nucleic Acids Res. 47, D590–D595. doi:10.1093/nar/gky962
Karp, P. D., and Caspi, R. (2011). A Survey of Metabolic Databases Emphasizing the MetaCyc Family. Arch. Toxicol. 85, 1015–1033. doi:10.1007/s00204-011-0705-2
King, Z. A., Lu, J., Dräger, A., Miller, P., Federowicz, S., Lerman, J. A., et al. (2016). BiGG Models: A Platform for Integrating, Standardizing and Sharing Genome-Scale Models. Nucleic Acids Res. 44, D515–D522. doi:10.1093/nar/gkv1049
Klapa, M. I. (2009). “Chapter 15: Metabolic Flux Analysis,” in The Metabolic Pathway Engineering Handbook Volume 1: Fundamentals. Editors Christina Smolke (CRC Press).
Lewis, C. A., Parker, S. J., Fiske, B. P., McCloskey, D., Gui, D. Y., Green, C. R., et al. (2014). Tracing Compartmentalized NADPH Metabolism in the Cytosol and Mitochondria of Mammalian Cells. Mol. Cell 55, 253–263. doi:10.1016/j.molcel.2014.05.008
Ma, H., Sorokin, A., Mazein, A., Selkov, A., Selkov, E., Demin, O., et al. (2007). The Edinburgh Human Metabolic Network Reconstruction and its Functional Analysis. Mol. Syst. Biol. 3, 135. doi:10.1038/msb4100177
Malik-Sheriff, R. S., Glont, M., Nguyen, T. V. N., Tiwari, K., Roberts, M. G., Xavier, A., et al. (2020). BioModels-15 Years of Sharing Computational Models in Life Science. Nucleic Acids Res. 48, D407–D415. doi:10.1093/nar/gkz1055
Mardinoglu, A., Agren, R., Kampf, C., Asplund, A., Nookaew, I., Jacobson, P., et al. (2013). Integration of Clinical Data with a Genome‐scale Metabolic Model of the Human Adipocyte. Mol. Syst. Biol. 9, 649. doi:10.1038/msb.2013.5
Mardinoglu, A., Agren, R., Kampf, C., Asplund, A., Uhlen, M., and Nielsen, J. (2014). Genome-scale Metabolic Modelling of Hepatocytes Reveals Serine Deficiency in Patients with Non-alcoholic Fatty Liver Disease. Nat. Commun. 5, 3083. doi:10.1038/ncomms4083
Masid, M., and Hatzimanikatis, V. (2021). Quantitative Modeling of Human Metabolism: A Call for a Community Effort. Curr. Opin. Syst. Biol. 26, 109–115. doi:10.1016/j.coisb.2021.04.008
McDonald, A. G., Boyce, S., and Tipton, K. F. (2009). ExplorEnz: the Primary Source of the IUBMB Enzyme List. Nucleic Acids Res. 37, D593–D597. doi:10.1093/nar/gkn582
Moretti, S., Tran, V. D. T., Mehl, F., Ibberson, M., and Pagni, M. (2021). MetaNetX/MNXref: Unified Namespace for Metabolites and Biochemical Reactions in the Context of Metabolic Models. Nucleic Acids Res. 49, D570–D574. doi:10.1093/nar/gkaa992
Nielsen, J. (2017b). Systems Biology of Metabolism. Annu. Rev. Biochem. 86, 245–275. doi:10.1146/annurev-biochem-061516-044757
Nielsen, J. (2017a). Systems Biology of Metabolism: A Driver for Developing Personalized and Precision Medicine. Cell Metab. 25, 572–579. doi:10.1016/j.cmet.2017.02.002
Noronha, A., Modamio, J., Jarosz, Y., Guerard, E., Sompairac, N., Preciat, G., et al. (2019). The Virtual Metabolic Human Database: Integrating Human and Gut Microbiome Metabolism with Nutrition and Disease. Nucleic Acids Res. 47, D614–D624. doi:10.1093/nar/gky992
Oberhardt, M. A., Palsson, B. Ø., and Papin, J. A. (2009). Applications of Genome‐scale Metabolic Reconstructions. Mol. Syst. Biol. 5, 320. doi:10.1038/msb.2009.77
Orth, J. D., Thiele, I., and Palsson, B. Ø. (2010). What Is Flux Balance Analysis? Nat. Biotechnol. 28, 245–248. doi:10.1038/nbt.1614
Robinson, J. L., Kocabaş, P., Wang, H., Cholley, P.-E., Cook, D., Nilsson, A., et al. (2020). An Atlas of Human Metabolism. Sci. Signal. 13, 1482. doi:10.1126/scisignal.aaz1482
Shlomi, T., Cabili, M. N., Herrgård, M. J., Palsson, B. Ø., and Ruppin, E. (2008). Network-based Prediction of Human Tissue-specific Metabolism. Nat. Biotechnol. 26, 1003–1010. doi:10.1038/nbt.1487
Smallbone, K. (2013). Striking a Balance with Recon 2.1. arXiv. 1311.5696v2. doi:10.48550/arXiv.1311.5696
Stephanopoulos, G., Aristidou, A. A., and Nielsen, J. H. (1998). Metabolic Engineering. San Diego: Academic Press.
Swainston, N., Smallbone, K., Hefzi, H., Dobson, P. D., Brewer, J., Hanscho, M., et al. (2016). Recon 2.2: from Reconstruction to Model of Human Metabolism. Metabolomics 12, 109. doi:10.1007/s11306-016-1051-4
Thiele, I., and Palsson, B. Ø. (2010). A Protocol for Generating a High-Quality Genome-Scale Metabolic Reconstruction. Nat. Protoc. 5, 93–121. doi:10.1038/nprot.2009.203
Thiele, I., Swainston, N., Fleming, R. M. T., Hoppe, A., Sahoo, S., Aurich, M. K., et al. (2013). A Community-Driven Global Reconstruction of Human Metabolism. Nat. Biotechnol. 31, 419–425. doi:10.1038/nbt.2488
van Iersel, M. P., Pico, A. R., Kelder, T., Gao, J., Ho, I., Hanspers, K., et al. (2010). The BridgeDb Framework: Standardized Access to Gene, Protein and Metabolite Identifier Mapping Services. BMC Bioinforma. 11, 5. doi:10.1186/1471-2105-11-5
Vasilopoulou, C. G., Margarity, M., and Klapa, M. I. (2016). Metabolomic Analysis in Brain Research: Opportunities and Challenges. Front. Physiol. 7, 183. doi:10.3389/fphys.2016.00183
Vieira, V., Ferreira, J., Rodrigues, R., Liu, F., and Rocha, M. (2018). A Model Integration Pipeline for the Improvement of Human Genome-Scale Metabolic Reconstructions. J. Integr. Bioinform. 16, 1–11. doi:10.1515/jib-2018-0068
Wang, Y., Eddy, J. A., and Price, N. D. (2012). Reconstruction of Genome-Scale Metabolic Models for 126 Human Tissues Using mCADRE. BMC Syst. Biol. 6, 153. doi:10.1186/1752-0509-6-153
Keywords: metabolic modeling, stoichiometric models, human metabolic network reconstruction, fluxomics, metabolomics, network biology, systems biology
Citation: Pantziri MDA and Klapa MI (2022) Standardization of Human Metabolic Stoichiometric Models: Challenges and Directions. Front. Syst. Biol. 2:899980. doi: 10.3389/fsysb.2022.899980
Received: 19 March 2022; Accepted: 24 May 2022;
Published: 24 June 2022.
Edited by:
Edoardo Saccenti, Wageningen University and Research, NetherlandsReviewed by:
Miguel Rocha, University of Minho, PortugalCopyright © 2022 Pantziri and Klapa. This is an open-access article distributed under the terms of the Creative Commons Attribution License (CC BY). The use, distribution or reproduction in other forums is permitted, provided the original author(s) and the copyright owner(s) are credited and that the original publication in this journal is cited, in accordance with accepted academic practice. No use, distribution or reproduction is permitted which does not comply with these terms.
*Correspondence: Maria I. Klapa, bWtsYXBhQGljZWh0LmZvcnRoLmdy