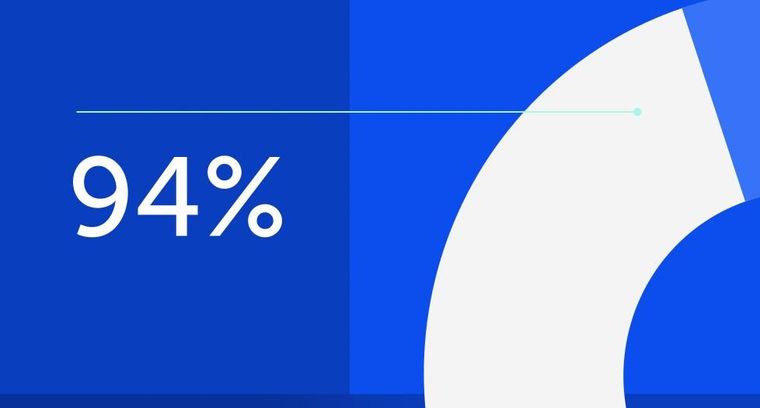
94% of researchers rate our articles as excellent or good
Learn more about the work of our research integrity team to safeguard the quality of each article we publish.
Find out more
ORIGINAL RESEARCH article
Front. Syst. Biol., 30 May 2022
Sec. Data and Model Integration
Volume 2 - 2022 | https://doi.org/10.3389/fsysb.2022.896265
This article is part of the Research TopicSystems Biology, Women in Science 2021/22: Data and Model IntegrationView all 9 articles
Astrocytes play an important role in various processes in the brain, including pathological conditions such as neurodegenerative diseases. Recent studies have shown that the increase in saturated fatty acids such as palmitic acid (PA) triggers pro-inflammatory pathways in the brain. The use of synthetic neurosteroids such as tibolone has demonstrated neuro-protective mechanisms. However, broad studies, with a systemic point of view on the neurodegenerative role of PA and the neuro-protective mechanisms of tibolone are lacking. In this study, we performed the integration of multi-omic data (transcriptome and proteome) into a human astrocyte genomic scale metabolic model to study the astrocytic response during palmitate treatment. We evaluated metabolic fluxes in three scenarios (healthy, induced inflammation by PA, and tibolone treatment under PA inflammation). We also applied a control theory approach to identify those reactions that exert more control in the astrocytic system. Our results suggest that PA generates a modulation of central and secondary metabolism, showing a switch in energy source use through inhibition of folate cycle and fatty acid β-oxidation and upregulation of ketone bodies formation. We found 25 metabolic switches under PA-mediated cellular regulation, 9 of which were critical only in the inflammatory scenario but not in the protective tibolone one. Within these reactions, inhibitory, total, and directional coupling profiles were key findings, playing a fundamental role in the (de)regulation in metabolic pathways that may increase neurotoxicity and represent potential treatment targets. Finally, the overall framework of our approach facilitates the understanding of complex metabolic regulation, and it can be used for in silico exploration of the mechanisms of astrocytic cell regulation, directing a more complex future experimental work in neurodegenerative diseases.
Astrocytes are involved in several functions that are essential to the maintenance of the brain’s homeostasis, as well as on the capture and a release of metabolites for neuronal protection (Buskila et al., 2019); in both physiological and pathological conditions, astrocytes play a crucial role in synaptogenesis, neurotransmitter release, neuroinflammation, elimination of toxic substances, buffering and spatial release of K+, among others (Volterra and Meldolesi 2005; Cabezas et al., 2012; Robertson 2018; González et al., 2020). An important role of the astrocytes and their interaction with neurons, is during the metabolism of fatty acids (FAs) (de Carvalho and Caramujo, 2018; Hashimoto and Hossain, 2018). One of the most common and predominant saturated fatty acids in the human body is the PA which in excess generates lipotoxicity. This metabolic condition induces the activation of pro-inflammatory pathways as NF-kB, resulting in an increased expression of pro-inflammatory cytokines like TNF, IL-1, IL-6. The above triggers pathological responses such as inflammatory processes, ceramide formation, oxidative stress (OS), among others (Carta et al., 2017; Frago et al., 2017; Fatima et al., 2019). In this context, recent studies have determined the association between PA and metabolic dysregulation in astrocytes. This generates modifications in the correct functioning of the central nervous system by the change of glial environment and increases the risk of developing dementia, such as amyotrophic lateral sclerosis (ALS), Alzheimer’s disease (AD), Huntington’s disease (HD), Parkinson’s disease (PD), among others (Luterman et al., 2000; Patil et al., 2006; Wong et al., 2014; Melo, Santos, and Ferreira 2019; Ortiz-Rodriguez et al., 2019).
Recent studies have described the crucial role of neuroactive estrogens, such as tibolone, as beneficial candidates for treating neurodegenerative diseases (Martin-jiménez and González 2020). In addition, tibolone has been a helpful treatment for osteoporosis in postmenopausal women and has had sound antidepressant effects (Arevalo et al., 2015; Crespo-Castrillo et al., 2020). Tibolone has been shown to reduce nuclear fragmentation and the production of reactive oxygen species, as well as activation of the Akt/GSK3ß signaling pathway that generates antioxidant activity in neuronal cultures, among other effects (Dhandapani et al., 2005; Martin-jiménez and González 2020). However, studies of astrocyte metabolism in association with lipotoxicity and tibolone treatment have focused on deciphering specific elements through experimental simulation, ignoring mechanisms that can occur at multiple levels of biological organization (omics) and creating a lack of understanding on the metabolic relationship between these interactions and pathological conditions (Ravindran et al., 2019). In this aspect, with the increase and availability of large-scale multi-omic data, there is a huge potential on the biological insights that can be drawn from integrating these data (Currais et al., 2015) as it has been done for other organisms in the study of response to changing environment conditions (Bardozzo et al., 2018). Therefore, developing a comprehensive view of the mechanisms implicated in brain behavior involves systemic approaches, which can be evaluated through mathematical representations of metabolism, such as genome-scale metabolic networks (GEMs) (Basler and Nikoloski 2011; Nielsen 2017). These models are typically built from several data sources (i.e., literature-based evidence, information of biological databases, among others), and allow the integration of multi-omics data that aims into the discovery of molecular pathways and molecular mechanisms affected by a disease state (Rezola et al., 2015; Ramon et al., 2018; Marttinen et al., 2019; Wörheide et al., 2021). GEMs have also demonstrated their efficiency in identifying essential metabolic reactions in a metabolic system and unraveling cellular control, leading to the prediction of genotype-phenotype relationships (Sweetlove and George Ratcliffe, 2011; Basler et al., 2016). However, conventional methods used to interrogate these models, such as flow balance analysis and its derivatives have shown to be usually biased, since they restrict the flow space to a reference state, and therefore running into an impartial computational approach (Terzer and Stelling 2008; Basler and Nikoloski 2011). Therefore, through the application of an unbiased approach, such as Control Theory, we are able to identify groups of reactions that are controlled directly or indirectly by the cell in order to further control its overall activity, without prior knowledge of the cellular objectives (Basler and Nikoloski 2011; Basler et al., 2016).
This approach allowed us to understand the mechanism in astrocytic metabolism under lipotoxic conditions and treatment with tibolone. Additionally, it proved to be key in order to propose new and better markers of astrocytic activation/injury, leading to a better understanding of the biological aspects involved in neuronal degeneration. Despite its usefulness, there are no previous studies that applied a multi-omic integration and control theory approach on metabolic networks to elucidate the mechanisms associated with phenomena of neurodegenerative diseases in astrocytic GEMs.
This study aimed to identify the controlling reactions for the human astrocytic response to PA in a multi-omic GEM, through the update of a published high-quality astrocyte model previously published by our group (Osorio et al., 2020) and the integration of transcriptome and proteome data under two treatments (control, low PA, and under PA/tibolone treatment). We used association rules and dimensionality reduction to identify reactions that are activated or deactivated in the context-specific model. We also applied a control theory approach to identify those controlling reactions that are key to switch between functional states in the system. Our results showed significant changes in cell metabolism under different treatments, activating inflammatory mechanisms in the case of PA and possible protective mechanisms in the case of tibolone. This research will lay the foundations for future pharmacological research, aimed at creating more effective therapies, and in the control field, aimed at extending its applications into the biological phenomena.
Transcriptome and proteome data was obtained by our laboratory from Lonza’s Normal Human Astrocytes cell line (NHA). For proteome and transcriptome data, three batches of NHA were cultivated in Astrocytes Basal Medium (ABM, Lonza) and were trypsinized. The cells were cultivated under 5% CO2 and 37 °C conditions until the cells reached a confluence near 80% to be later used under different treatments (palmitic acid, pretreatment with tibolone plus palmitic acid).
Pretreatment was performed by dissolving tibolone (Sigma–Aldrich, T0827, St. Louis, MO, United States) in 100% DMSO as a 40 mM stock solution; further dilutions were made with serum-free Dulbecco’s Modified Eagle Medium (DMEM) without phenol red. Concentrations between (100 uM–10 nM) were tested in cell culture. The optimal time and concentration of tibolone was 10 nM for 24 h. The final concentration of DMSO was <0.01%.
After tibolone treatment, cells were washed with 2x PBS1X and treated with serum-free DMEM containing palmitic acid (P0500, Sigma, St Louis, MO, United States), BSA (free bovine serum albumin of fatty acids; Sigma A2153) as a carrier protein and carnitine (C0283, Sigma, St Louis, MO, United States) to transport fatty acids to the mitochondrial matrix.
The cells were treated with different concentrations of palmitic acid (100–2000 µM). The final concentration of palmitic acid used was 2000 µM and the treatment time was 24 h. The control group included a final concentration of 1.35% BSA and 2 mM carnitine ((Martin-jiménez and González 2020).
After pre-treatment with tibolone (24 h) and treatment with palmitic acid (24 h), protein extraction and quantification were performed, using a lysis preparation composed of RIPA buffer (Thermo Scientific, Cat. 89,900) and inhibitor cocktail. of Halt Thermo 1X proteases (CAT-78425). Subsequently, centrifugation cycles were performed at 10,000 rpm at 4°C for 10 min to extract the proteins present in the supernatant. A sample of the solubilized proteins was used for protein quantification by the bicinchoninic acid method (BCA1 Sigma-Aldrich kit).
The protein pellet was submitted to UC Davis Proteomics Core for proteomics identification. For digestion, the samples were treated with dithiothreitol (DTT) and incubated at 37°C, to then be treated with iodoacetamide (IAA) and incubated at room temperature. DTT treatments must be performed to neutralize IAA. AMIC and trypsin were added to dilute the samples, which were desalted using the Macro Spin Column (Nest Group) (unpublished). The digested peptides were analyzed by LC-MS/MS together with Proxeon Easy-nLC II HPLC and Proxeon nanospray source. Data processing was carried out using cleavage parameters and a data mapping against SwissProt database to search for unique peptides. In addition, Sequest and AMANDA were used for label-free-quantification. The results were processed with MaxQuant v1.6.10.43 and Perseus v 1.6.10.45. The transformation of protein intensities was carried out in R from the variance normalization and stabilization method (VSN). The data imputation was carried out using k nearest neighbors (KNN). Differential expression analysis was performed using the optimized reproducibility test (ROTS), evaluating the following conditions: PA vs. vehicle, PA with tibolone pretreatment vs. vehicle, and PA with tibolone pretreatment vs. PA. The protein functional enrichment analysis was performed using g:Profiler. Finally, a weighted co-expression network analysis was performed to visualize protein-protein interactions given the treatments (unpublished).
Regarding the transcriptome, the libraries were prepared by extracting total RNA using the RNeasy mini kit (Qiagen, United States). RNase-free DNAse I was used to prevent contamination in the samples. Samples were stored at −80°C in a nuclease-free buffer for RNA sequencing on an Illumina HiSeq machine with a 2 × 150 bp paired-end configuration, producing a sequencing depth of ∼75 million reads per sample. The quality control of the sequencing was evaluated through QUARS (QUA of controlling for RNA-Seq; github.com/tluquez/QUARS). Saturation plots were made to assess the quality of sequencing. Differential expression analysis was performed by inspecting the log counts, using DESeq2 to normalize the library size and reduce the variance.
Differential expression analysis was performed by checking the record counts, using DESeq2 to normalize the library size and contract the variance. Differential expression between conditions (PA versus vehicle, PA with tibolone pretreatment versus vehicle, and PA with tibolone pretreatment versus PA) was evaluated using Wald tests with a Bonferroni correction. The gene pool enrichment analysis was performed using Gene Ontology, WikiPathways, and Reactome, using 24,893 genes with at least ten reads per treatment. The construction of a brain-specific gene regulatory network was made using the transcriptional regulatory network analysis (TReNA) package (rdrr.io/bioc/TReNA). Moreover, this network was built using the ENCODE atlas and data from 278 post-mortem temporal cortex samples from the Mayo Clinic. TReNA enumerates all TF-target pairs of genes where there is evidence that the TF binds the proximal promoter and ranks these candidate interactions based on co-expression evidence and Spearman correlation. The analysis of the gene regulatory network allowed elucidation of which ascendant transcription factors are differentially expressed that are potentially regulating the expressed genes. . The data obtained from the transcriptome and proteome were experimentally validated and integrated into the Osorio’s astrocyte model (Osorio et al., 2020), using the methodology described below.
Restriction-based metabolic models, such as the GEM used in this work, allow in-silico description and prediction of possible metabolic states through the mathematical representation of metabolic reactions in a stoichiometric matrix (
In the context of multi-omic data integration, GEMs are ideal frameworks that allow the association of these type of data with other layers of biological information such as that derived from the transcriptome and the proteome(Bordbar et al., 2014; Voillet et al., 2016; Pinu et al., 2019). In our case, we used the astrocyte GEM created by Osorio et al. (2020), which represents 1956 metabolites and 2747 metabolic reactions, associated to Gene-Protein-Reaction (GPR) association rules that play an important role in the integration of experimental data (transcriptome, proteome and their relationship with reactions) in the model, thus increasing the veracity of its predictions (Kim et al., 2016; Karahalil 2017; Gu et al., 2019).
Describing the GPRs associations into a GEM brings several advantages for modeling. For instance, they permit gene deletion simulations and ease the omic data integration. In our case, the reconstruction carried out by Osorio et al. (2020) excluded the EC numbers (Enzyme Commission Number), from which one can generate PR (Protein-Reaction) associations necessary to be linked with appropriate genes. Therefore, we further updated Osorio’s model by a manual construction of these associations: 1) the list of genes associated with the model was obtained, and the IDs were searched in UniProt (https://www.uniprot.org/uploadlists/); 2) from the list, the EC numbers were associated with each of the reactions, using the makeprules function (https://github.com/gibbslab/GEM-multiomic-integrator); 3) the mapping of abundance reactions was performed by associating the abundance values with the EC identifiers for each reaction of the model. This allowed us to obtain the abundance data for each scenario. Alternatively, for mapping data expression, the following methodology was performed: 1) the ID of the Ensembl reaction was converted to Entrez to map the expressions; 2) the expressions for each reaction were extracted using the GPR association, using the mapping gene expression methodology described below, obtaining the expression data for each scenario.
Association rules (GPR and PR) can include more than one gene or protein for each reaction. Therefore, reactions with multiple associations include logical rules (AND and OR) to indicate the order and essentiality of each association. These logical rules were used to map the expression and abundance values of the reactions, taking the minimum values when the associations were joined by AND and the maximum values when it was OR. COBRA toolbox was used for association rules and mapping gene expression and protein abundance (Hyduke et al., 2011).
Dimensionality reduction is a mathematical way to reduce the complexity of a data set while increasing the statistical power of analysis by reducing the burden of multiple tests (Zierer et al., 2016; Altenbuchinger et al., 2019; Wörheide et al., 2021), we accomplished this reduction by means of principal component analysis (PCA). In our case, PCA was applied to the transcriptome and proteome data sets, transforming the unique omic variables into a lower-dimensional subspace that maximizes the retention of variance within the data by finding orthogonal linear combinations of the original variables, saving as much information as possible. In this way, the population’s behavior can be observed in a smaller set.
The PCA requires that the variables are all quantitative and normalized, that is, with mean 0 and variance 1 for each variable, as described in the following equation:
Once all the variables are standardized, the covariance matrix is calculated, allowing us to observe how the variables are related to each other. The above is obtained by multiplying the variance and covariance matrix as shown below:
When the variance and covariance matrix is calculated, then the values of eigenvectors are estimated, and the principal components (PCs) are created. The methodology allows the creation of not correlated PCs, letting the information of linearly correlated variables to be represented in one PC. These permit the construction of an estimated biologically significant variable (Rodríguez-Mier et al., 2021) and provides a way to limit the potential for overfitting (Wörheide et al., 2021).
To test the metabolic effects of PA and tibolone during metabolic inflammation in astrocytes, we defined three scenarios considering previously obtained transcriptome and experimental proteome data: 1) a “healthy"/control scenario, which emulates normal metabolic conditions of astrocytes (Das et al., 2010; Osorio et al., 2020); 2) A palmitate-induced inflammatory scenario; 3) a treatment scenario with tibolone was defined after the induction of inflammation by PA. In order to restrict the GEM with each of this data sets, and thus obtain a model for each of the aforementioned scenarios, the Exp2flux algorithm was used (https://github.com/gibbslab/exp2flux). using the previously information obtained by the PCA as values for the expression data required for this tool. The limits of the flow of the exchange reactions were not changed.
Flux balance analysis (FBA) is a linear optimization method that selects flux values that can optimize (maximize or minimize) the objective function (Huang et al., 2020; Orth, Thiele, and Palsson 2010; Orth et al., 2011). Metabolic reactions are represented as
The FBA can be described from the following system of equations:
Where vector
The metabolic flux differences for each reaction between optimized scenarios were measured using the methodology proposed by Osorio et al. (2020) and Osorio et al. (2016), where the flux difference function (fluxDifferences) and the fold change function (foldchange) describe how much the flux changes. The function takes as argument two valid models and a threshold value of −0.5 to 0.5 for reactions with an absolute change between the metabolic scenarios evaluated (Osorio et al., 2020).
An analysis of essential reactions was performed in order to identify those reactions that were essential for growth and maintenance of the astrocytic cell, using the principle of enzymatic functionality by knockout of reactions (Jiang et al., 2015), modifying each reaction’s upper and lower limits to become zero Gelius-Dietrich et al. (2013). In order to calculate all flux distributions on the restricted models that were previously created, we applied the optimization algorithm lMOMA. This algorithm unlike regular FBA, simulates all possible suboptimal states associated with the metabolic model (Segre et al., 2002), according to equations (8)–(10):
Where
Although gene essentiality analysis is key for the understanding of the set of genes necessary for cell survival, there is still a lack of knowledge on the specific mechanisms that control the underlying predicted cell behaviour. Therefore in the multi omic model created we identified its control structure by characterizing 4 key network components: 1) the flow coupling graph, 2) the set of flow coupling profiles, 3) the driver reactions and 4) all the possible metabolic switches (Basler et al., 2016).
The flow coupling graph of a metabolic model is a labeled and directed graph, that uses five flow coupling profiles: complete, partial, anti, inhibitory, and directional, indicating the type of coupling between reactions i and j that reflect functional characteristics of the considered metabolic pathways (Larhlimi et al., 2012; Basler et al., 2016). Therefore, we use the flow coupling graph to calculate the control graph, where reactions i are converted to nodes, and a directed edge (i→j) is set between nodes according to the flow profile. Therefore it is possible to determine if i can control the state of j or if j can control the state of i (Basler et al., 2016). This approach led us to the identification of driver reactions; it is the set of reactions that control the states and the activity pattern (in)directly of reactions in the model. It has been determined that the controlling reactions may have a divergent role in the control model and the challenged models. Therefore, the differences in the coupling profile of these reactions are considered as metabolic switches (Basler et al., 2016).
Following the methodology proposed by Basler et al. (2016), we use the Fast Flux Coupling Calculator (F2C2) algorithm within the MATLAB environment (MATLAB and Statistics Toolbox Release 2021b, The MathWorks, Inc., Natick, Massachusetts, United States) to determine coupled reactions (Larhlimi et al., 2012). The F2C2 algorithm identifies dead-end metabolites, blocks the corresponding reactions, removes the blocked reactions from the stoichiometric matrix, and applies the Trivial Full Coupling (TFC) rule to determine reactions proportional to each other. The algorithm repeats this step until a dead-end metabolite cannot be identified and updates each reaction’s reversibility (Larhlimi et al., 2012). Then, F2C2 applies Trivial Directional Coupling (TDC) and Trivial Uncoupling (TUC) rules to determine (un)coupled reactions and determine the coupling flux between (pseudo-)irreversible reactions using linear programming (LP) (David et al., 2011; Larhlimi et al., 2012). We restricted the set of feasible flow distributions and defined anti-coupling according to the following equation:
Where
Since the flow coupling profile of a network is determined by a vector
The hypothesis test was performed according to the network restrictions based on the mass balance principle, through the randomization methodology with replacement of substrates and products of the network, changing the stoichiometric coefficients while preserving the mass balance proposed by (Basler and Nikoloski 2011) and (Basler, Grimbs, and Ebenho 2012).
The sampling of reaction activity patterns was performed according to the methodology proposed by Basler et al. (2016), using random sampling of flow distributions in the steady-state, ensuring non-zero values. A labeled flow coupling graph of the metabolic network was made:
Where
Regarding the control graph for a network with
1)
2)
3)
4)
5)
Therefore, the calculation of the controlling reactions is given by a smaller set of reactions (intermittent, critical, and redundant reactions), whose activities must be specified to activate all the reactions given the value of σ. To calculate the activity pattern, we kept following the methodology proposed by Basler et al. (2016). The overall procedure used to calculate these controlling rections (Figure 1, part 7) was deployed under a dedicated computational node using 72 Intel Xeon Gold 6240 CPU cores at 2.60 GHz and 512 GB of RAM.
FIGURE 1. General overview of methodology. 1) Raw data as obtained and deposited by our laboratory was retrieved from https://www.ncbi.nlm.nih.gov/geo/query/acc.cgi?acc=GSE166500. 2) Transcriptomics and proteomics data dimensionality was reduced and then 3) used to constrain Osorio’s Genome Scale Model. 4, 5 and 6) Constrained model was subjected to Flux Balance Analysis and other optimization based algorithms. 7) Control reactions on constrained model were identified by means of control theory, using a three steps approach: creation of flow coupling graph, control graph and then calculation of control reactions.
We present three context-specific multi-omic models that are a compilation of 2747 biochemical reactions, of which 1607 belong to intracellular reactions, 60 correspond to reactions that allow the intake and out take of metabolites from the environment into the cell (exchange reactions), and 1080 are transport reactions, it is reactions that allow the transport of metabolites between cell compartments. In order to model the toxic effects of palmitic acid and the protective effect of tibolone at the metabolic level in astrocytes, we performed an optimization of the model under three different metabolic scenarios, obtaining a metabolic phenotype for each scenario (Figure 2).
FIGURE 2. Distribution of reactions by subsystems associated with the metabolic phenotype for each metabolic model generated. Main activated subsystems are shown. (A) Metabolic phenotype for the control model, in which 41.17% of the reactions were activated; (B) Metabolic phenotype for the palmitate model, in which 41.68% of the reactions were activated; (C) Metabolic phenotype for the tibolone-palmitate model, in which 41.65% of the reactions were activated.
The metabolic simulation predicts a growth rate for astrocytes of 0.447,430 mMgWD−1h−1under normal conditions (supplemented ABM medium), with an activation of 41.17% of the model reactions, suggesting a preference for an energy-based metabolism on glucose and fatty acids (Figure 2A). This energy-based metabolism was observed within the extracellular transport subsystem, where the metabolites obtained are associated with the catabolism of glucose and fatty acids. Furthermore, our model also suggests an energy metabolism based on fatty acid oxidation, which is consistent with the results obtained by Osorio et al. (2020). The metabolite release rate and biomass growth were used as a reference to compare changes between the three metabolic scenarios.
In this scenario, we used experimental information in which the cells were cultivated in a microenvironment with an increased concentration of PA. The calculated growth on this simulation was 0.472,459 mMgWD−1h−1. Our model showed an activation of 41.68% of the model reactions (Figure 2B), increasing the uptake of l-asparagine, l-arginine, l-ascorbate, l-carnitine, l-serine, d-glucose, and l-glutamate. These results are also consistent with the results obtained by Osorio et al. (2020) (Figure 3A). This response is usual in astrocytes under an insult, where the inflammation generates homeostatic alterations (Bylicky et al., 2018; Hidalgo-Lanussa et al., 2020).
FIGURE 3. Summary of metabolites and pathways associated with biochemical reactions that presented high flow variability compared to palmitate. (A) Uptake rate of metabolites on the inflammatory scenario using the generic biomass reaction included in the multi-omic model as the objective function; (B) Reactions activated by palmitate treatment; (C) Main reactions inactivated by palmitate treatment; (D) Reactions with decreased metabolic flux by palmitate treatment.
A decrease in arginine has been associated with inflammation and oxidative stress. Its reduction was a biomarker of metabolic inflammation during obesity, showing inverse proportionality between arginine concentration and IL-6, CRP, and TNF-α in serum (Niu et al., 2012). Thus, predicted arginine increase uptake could be related to an anti-inflammatory mechanism activated in astrocytes under PA treatment (Moncada and Higgs 1993). Like glutamine, increasing concentrations of l-asparagine have shown an increased pH in astrocytes, predicting an activation of the H+ exchange transport mechanism (Chaudhry et al., 1999; Chaudhry et al., 2001). Furthermore, asparagine induces a Ca2+ response comparable to GABA-induced Ca2+ states (Doengi et al., 2009; Schousboe et al., 2013; Osorio et al., 2020). Therefore, this asparagine uptake could be a feedback mechanism to control glutamine uptake that would probably be increased in the extracellular medium, as predicted by our model (Supplementary Material 1) (Chaudhry et al., 2001). Moreover, l-carnitine acts on the degradation of fatty acids by β-oxidation, representing a neuroprotective biomarker, leading cells to reduce oxidative stress and improve their energy metabolism (increasing catabolism and the release of lactate and glucose) (Agostinho et al., 2010; Pardo et al., 2013; Schousboe et al., 2013). On the other hand, ascorbate is reported to have an anti-inflammatory effect in neuron/glia cocultures by inhibiting p38, ERK MAPK signaling, and NF-κB translocation(Huang et al., 2014).
Our simulations also suggest that astrocytic cells can increase the glutamate uptake under inflammatory conditions. Although astrocytes prevent high concentrations of glutamate and maintain the metabolic homeostasis of amino acids by converting glutamate to glutamine for its neuronal absorption (Flott and Seifert 1991; Haroon et al., 2017; Mahmoud et al., 2019), a high concentration of glutamate contributes to exocytoxicity and chronic activation (reactive astrocytosis) (Huang et al., 2014; Haroon et al., 2017; Mahmoud et al., 2019). Astrocyte survival mechanisms have been associated with increased l-serine uptake, described by authors such as Green et al. (2014) and Osorio et al. (2020). In other words, all the metabolite exchanges that were differentially obtained in the inflammatory scenario by our model showed an essential role in homeostasis, which was expected due to the known function of astrocytes.
In the inflammatory scenario, the metabolic phenotype activate 1145 reactions where 535 showed a high flow variability (activation, inactivation or flow change), affecting the oxidation of fatty acids (11.21%), extracellular transport (35.14%), mitochondrial transport (8.22%), nucleotide interconversion (6.36%), endoplasmic reticulum transport (3.36%), keratan sulfate synthesis (2.06%) and the citric acid cycle (2.06%). These results are also consistent with findings by Osorio et al. (2020), who suggested that astrocytes modified the flow rate of 586 reactions compared to the non-stimulated scenario. In this sense, it is important to highlight that Osorio et al. (2020) performed a sensitivity analysis to identify pro-inflammatory reactions, which by inhibiting them, improved the cellular metabolism of astrocytes. The two candidate reactions were associated with formimidoyltransferase cyclodeaminase (FTCD) and mitochondrial water transport, associated with aquaporin-9. The role of FTCD in high-fat diets as well as in the degradation of folate pool, glutamate synthesis and memory performance in young adults has been previously described (reviewed in Osorio et al., 2020). It is worth noticing that consistently in this work we predict this same activation, and further analysis are needed in order to establish the exact role of FTCD in the contect of neuroinflamation. Our results also predicted the activation of additional reactions related to the keratan sulfate subsystem (Table 1). In response to injury, reactive astrocytes lead to scar formation (glial scar) by upregulation of sulfate proteoglycans and keratan sulfate proteoglycans (KSPG) for the inhibition of axonal growth of injured neurons (Patil et al., 2007; Hilton et al., 2012; Verkhratsky and Butt 2018; Dupuis et al., 2019; Li et al., 2019; Li et al., 2020). Recently it has been determined that the down-regulation of N-acetylglucosamine 6-O-sulfotransferase (GlcNAc6ST) is involved in the keratan sulfate pathway in the central nervous system, leading to the loss of reactive keratan sulfate, causing a reduction in glial scar formation after injury (Zhang et al., 2006b; Ito et al., 2010; Zhang et al., 2006a). Therefore, there is a consistency between our model predictions and the keratan sulfate subsystem as key reactions during astrocyte inflamation, whose variation would possibly improve the astrocyte metabolism during a lipotoxic scenario.
An essentiality analysis was performed to determine which of the 535 reactions variates during inflammation were vital for cell survival. We identified 136 essential reactions that may be associated with pro-inflammatory processes. The majority reactions belonged to extracellular transport (27.12%), endoplasmic reticulum transport (10.17%), Fatty acid oxidation (25.42%) and folate metabolism (3.39%). Within these reactions, PA generates the activation of 59 reactions (Figure 3B). Some of them are extracellular transport reactions associated with the uptake of l-arginine, l-alanine, l-asparagine, l-threonine, l-methionine, l-isoleucine, l-phenylalanine, l-valine, and l-histidine. As mentioned above, many of these metabolites have been previously shown to participate in the inflammatory response. The increase in histidine uptake is associated with the uptake of free radicals, and they are negatively related together with threonine, glycine, lysine, and serine with IL-6, TNF-α, CRP, and IL-8 secretion (Son et al., 2005; Niu et al., 2012). Hence, these identified essential reactions have an experimentally demonstrated importance in inflammation, being crucial for homeostasis maintenance.
PA scenario simulations generated the inactivation of 46 reactions. The majority of these reactions are associated with: Golgi apparatus transport (10.87%), monosaccharide metabolism (2.17%), folate metabolism (2.17%) (Table 2), and degradation of keratan sulfate (2.17%) (Figure 3C). Folate metabolism supports carbon metabolism, which activates and transfers carbon units for biosynthetic processes (Souders et al., 2021). In astrocytes, both serine and tetrahydrofolate (THF) decrease induce astrocytic stress, reducing NADPH synthesis due to NAD+ deficiency (Balsa et al., 2020; Rose et al., 2020). When the mitochondrial folate pathway is lost, the directionality of cytosolic carbon flux reverses to compensate for NADPH synthesis (Field et al., 2014; Rose et al., 2020). Methylenetetrahydrofolate dehydrogenase (MTHFD) in healthy or normal tissues of the central nervous system is low or even absent. However, the expression of MTHFD in pathological states increases and generates a decrease in the availability of methyltetrahydrofolate, creating high demand on the part of the methyl groups’ donors for the formation of methionine (Coppedè et al., 2006; Coppedè 2021; Shi et al., 2021). This mechanisms affects neuronal communication and signaling and antioxidant processes that supports mitochondrial function in cells (Coppedè et al., 2006; Field et al., 2014; Rose et al., 2020). As stated above, our model identified folate metabolism as a set of essential reactions, and the inactivation of some of them exhibits the stress produced by PA in astrocytic metabolism. On the other hand, 24 reactions decreased metabolic flux, among which the majority are keratan sulfate synthesis (33.33%), extracellular transport (12.5%), monosaccharide metabolism (8.33%), and synthesis of CoA (8.33%) (Figure 3D). As mentioned earlier, the alterations in metabolism associated with these subsystems are associated with pro-inflammatory processes. According to our model, the keratan sulfate pathway was detected in the sensitivity analysis, its degradation is inhibited, its synthesis is reduced, and high variability was observed in this group. Our findings highlight the importance that keratan sulfate may have in the astrocytic response to PA, making it a potential target for further studies.
TABLE 2. Set of reactions of folate metabolism with inflammatory potential and changes, identified through an essentiality and variability analysis on the inflammatory scenario with palmitate.
Under the tibolone treatment scenario, simulations showed a cell growth of 0.605,193 mMgWD−1h−1. After tibolone in silico treatment, the model activated 41.65% of biochemical reactions (Figure 2C). When contrasting the palmitate model against the tibolone model, tibolone generated a variation of 747 reactions. We found important metabolic changes associated with the activation, inactivation or flow change of protective pathways in astrocytes, which agrees with the simulation carried out by Osorio et al. (2020). In this scenario was found 374 essential reactions whose variability may be associated with inflammatory or protective processes. Within these reactions, the majority is contemplated in the oxidation of fatty acids (21.93%), degradation of keratan sulfate (12.03%), synthesis of keratan sulfate (9.63%), extracellular transport (7.22%), mitochondrial transport (3.74%) and chondroitin synthesis (3.74%). Of these reactions, 17 were activated (Supplementary Figure S1A), and 60 increased their flux value (Supplementary Figure S1D) under tibolone treatment. Among these 60 reactions, the most representative biological systems are the oxidation of fatty acids (28.33%), synthesis of keratan sulfate (28.33%), and extracellular transport (8.33%). Under tibolone treatment, keratan sulfate synthesis is shown to be upregulated, probably allowing glial scars to form by activating the glia, microglia, and oligodendrocytes (Supplementary Table S1) (Jones and Tuszynski 2002; Zhang et al., 2006a; Zhang et al., 2006b; Rose et al., 2020). Reactive astrogliosis is proven to be beneficial for neural protection and regulation of inflammation in multiple conditions(Sofroniew, 2009). However, our model found the inactivation of reactions associated with N-acetylglucosamine 6-O-sulfotransferase-1, an enzyme related to the keratan sulfate synthesis (Zhang et al., 2006a). It is possible that the tibolone pre-treatment is activating a protective mechanism in astrocytes in order to compensate for the lipotoxic effect of PA and that this is a very controlled activation, similar to the expected in a protective scenario (Sofroniew, 2009).
Additionally, this scenario showed the inactivation of 197 reactions (Supplementary Figure S1B) and decreased flux of 16 reactions (Supplementary Figure S1C), that correspond to oxidation of fatty acids (31.47%), synthesis of chondroitin (7.11%), and degradation of keratan sulfate (5.58%) among others. As with the results obtained by Osorio et al. (2020), the rate of absorption and release of l-glutamine and l-glutamate mediated by tibolone was found to be reduced, which is associated with neuroprotective effects (Flott and Seifert 1991). Furthermore, as mentioned above, tibolone could positively affect protective pathways by inhibiting the degradation of keratan, allowing a possible increase in cell viability by protecting cell homeostasis. These results suggest that tibolone exerts a significant modulation on inflammatory reactions by activating protective pathways. Likewise, it generates the deactivation of neuroinflammatory pathways, which agrees with experimental results (Schuller-levis and Park, 2003; Ávila et al., 2014; Hidalgo-lanussa et al., 2017; Martín-Jiménez et al., 2017; González-giraldo et al., 2019).
The previous results allowed us to have a global vision of the possible target reactions according to our models. We use control theory which combines the efficiency of previously performed methods with the breadth of unbiased approaches by not using objective functions and thus allows for systematic metabolism studies. According to the control theory and the principle of steady-state, the reactions operate through coupling relations and the state of the reactions (McCloskey et al., 2013). Burgard et al. (2004) and (Basler et al., 2016) have proposed five flow coupling relationships: directional, partial, complete, inhibitory, and anticoupling. The coupling implies that the fluxes can be controlled by regulating enzyme activities, concentrations, and the principle of steady-state, which suggests that the gene-protein-reaction relationship must be coordinated according to its imposed state (Schuetz et al., 2007; Basler et al., 2016).
To determine if the flow coupling reflects the functional principles of metabolism of the astrocyte model, we analyzed the three models studied previously (control, lipotoxic, and tibolone). For each model, we first compute the five coupling types and their frequencies according to the flux binding profile for each model (Figures 4A,B). We found that the coupling profiles for the three networks are similar. However, the reactions that participate in each model vary according to the metabolic characteristics in each scenario, which is consistent with the previous analysis. According to the results obtained by Basler et al. (2016), anticoupling is rarely found in this type of model, so the anticoupling frequencies found (<0.1) for our multi-omics networks are consistent with their results.
FIGURE 4. Clustering of flux coupling profiles and change in coupling frequency of metabolic switches in control vs. lipotoxic models. We used three clustering methods: hierarchical, k-means, and kmedoids for coupling profile classification. (A) Flux coupling profiles of “healthy” multi-omic model of astrocyte; (B) Flux coupling profiles of lipotoxic multi-omic model of astrocyte; (C) Box plot showing the comparison of the coupling profiles of critical driver reactions in inflammatory vs. “healthy”/control scenario. The critical driver reactions in the lipotoxic model and redundant and intermittent reactions in the healthy model are considered metabolic switches. Negative values indicate a low number of couplings in metabolic models, and positive values indicate many couplings. The number of anti and full couplings of critical driver reactions in the lipotoxic model is similar in the healthy model, while inhibitory, partial, and directional reactions vary.
Considering the five coupling relationships, we applied the methodology used by Basler et al. (2016), in which the activity patterns are considered, through a control graph. The control graph indicates which reactions control the state of other reactions for the activity pattern considering the described sampling scheme and calculating the average of driver reactions over 1000 activity patterns (Terzer and Stelling 2008; Basler et al., 2016). Therefore, we determined that the fraction of reactions that are drivers of central metabolism for the astrocyte model is approximately 41.95%. This is consistent with the fractions ranging from 35.8 to 49.2% for the eukaryotes by Basler et al. (2016). It is important to highlight that most of the reactions found are part of the central metabolism, indirectly controlling secondary metabolism reactions, as observed in our previous analyses. It is also important to highlight that this is a time and computer resources consuming step. In our case, for a network this size and with the hardware resources described in the Methods section, this analysis took us 2 weeks of dedicated computing resources to be completed.
As described above, increased uptake of l-serine, d-glucose, l-glutamate, and the release of l -glutamine and lactate, is a usual response to the inflammatory response. As well as the activation and deactivation of metabolic pathways associated with inflammation and oxidative stress. However, it remains challenging to identify the relationship of genes and enzymes that serve as possible activation factors of the astrocytic lesion in a lipotoxic scenario and its modulation with tibolone. Therefore, we analyze the gene-enzyme-reaction associations by classifying the controller nodes according to their roles in each scenario in the model (Devkota and Wuchty, 2020), grouping them into three classes according to the number of activity patterns (critical, redundant, and intermittent reactions) carried out by Basler et al. (2016) (Supplementary Figure S2). For the three evaluated models, we found 84 critical reactions associated with central metabolism: carbohydrate metabolism (i.e., glycolysis, gluconeogenesis), pentose phosphate pathways, tricarboxylic acid cycle, amino acid biosynthesis, and amino acid exchange pathways (Supplementary Table S2).
To identify flux control patterns that underlie healthy astrocytic cell metabolism changes, we identified critical driver reactions in the palmitic acid model, which are redundant or intermittent in the control model. We refer to these reactions as metabolic switches because of their divergent role in controlling the lipotoxic effect on the astrocytic cell but not in healthy metabolism. In total, 25 metabolic switches were found, where the coupling profile differs between the control and AP models, mainly concerning directional, inhibitory, and partial couplings (Figure 4C).
Considering the above, we focused our analysis on the 25 metabolic switches and its influence on astrocytic metabolic phenotype when modulated by PA (Figure 5). As mentioned above, astrocytes use glucose through glycolysis as the primary energy source, generating pyruvate as the main product. Of course, there was a tight coupling of pyruvate production by oxidative reactions of glucose in the healthy astrocyte model. However, in the presence of PA, the reactions associated with glycolysis: catalysis of glyceraldehyde 3-phosphate to d-glycerate 1,3-bisphosphate (GAPD, EC 0.1.2.1.12) by Glyceraldehyde 3-phosphate dehydrogenase and catalysis of the reversible transfer of a phosphate group from 1,3-bisphosphoglycerate to ADP producing 3-phosphoglycerate and ATP (PGK, EC. 2.7.2.3) by Phosphoglycerate kinase, they may be partially inhibited, especially by the active coupling interaction due to their role in the co-production of pyruvate (Supplementary Figure S4). This result suggests that PA increasing the dependence of fatty acid oxidation in astrocytes (Schafer et al., 2004; Fell 2005; Yang and Vousden 2016; Rose et al., 2020). The inhibitory profiles described above alter reactions associated with folate metabolism FTCD (GluForTx, EC. 2.1.2.5; 4.3.1.4) discussed before and the catalysis reaction of argininosuccinate by argininosuccinate synthase (ARGSS, EC. 6.3.4.5) associated with urea metabolism. This confirms the predicted role of these reactions as a critical driver in a scenario within PA, but not in the control scenario.
FIGURE 5. The presence of 25 metabolic switches in the lipotoxic model allows identifying critical reactions. (1) In astrocytes, glucose is used through glycolysis as the primary energy source; however, PA negatively regulates reactions associated with this metabolic pathway through GAPD and PGK. It has further been shown that recurrent exposure to low glucose levels increases astrocyte FAO dependency. In our model, the presence of PA can modulate and alter COAtm, affecting the oxidative decarbonization of pyruvate. (2) Fatty acids (FA) with less 12-C can enter the mitochondria directly; however, fatty acids with 13–21 carbons need the carnitine shuttle to pass into the mitochondria. In the presence of PA, the reactions of carnitine transport C4tcx, C4tmc, and C4x showed downregulation. (3) β-oxidation is the preferable route for AC-CoA synthesis. In the presence of PA, it can be altered by the negative regulation of C4CRNCPT2, which transports carnitine for the final hydrolysis of fatty acids and modulates the reactions FAOXC16080x, FAOXC6C4x, FAOXC8C6x, and r0735. (4) PA upregulates HMGCOASim and HMGCOAtm, probably generating a high production of ketone bodies, which act as an alternative fuel. (5) Cysteine Ex_cys_L together with the glutamate Ex_glu_L allows the synthesis of glutathione (GSH). In the case of the oxidative stress generated by PA, the production of ROS dependent on NADPH is affected, deregulating the redox state. In our model, the reactions associated with the biosynthesis of RNA pyrimidines, OROTGLUt and r0839, demonstrated a critical role in the presence of PA; Ex_cys_L was presented as a critical reaction due to its different roles, especially in the production of GSH and palmitoylation. (6) Reactions associated with the folate pathway have been determined as essential in the lipotoxic stage. These reactions are FTHFLm, MTHFD, and GHMT2r. We also found metabolic switches involved in cell maintenance that, in the presence of PA, modulate central and secondary metabolic pathways, including EX_pro_L(e), FORt2m (for mitochondrial formate transport), DGTPtn (DGTP diffusion in the nucleus), and H2O transport.
The flux change in the lipotoxic model generates a partial or total inhibitory modulation of mitochondrial CoA transport (COAtm), affecting the oxidative decarboxylation of pyruvate to acetyl-CoA and the esterification of fatty acids (ATP-dependent process) (Rose et al., 2020). In this process, the carnitine shuttle needs to cross the mitochondrial membrane and be oxidized to metabolize fatty acids. Therefore, and as mentioned above, carnitine represents a biomarker and, at the same time, it is completely coupled with reactions of the β-oxidation cycle. This could indicate that reactions associated with its transport (C4tcx, C4tmc, C4x, and C4CRNCPT2, EC. 2.3.1.21) are essential in astrocytic metabolism since they can modulate the coenzyme A thioesters (Acyl-CoA) transport. In the presence of PA, we found that the reactions associated with carnitine transport are not completely inhibited, but they generate a downward modulation of the fully coupled reactions: related to the β-oxidation of long-chain acids (FAOXC16080x, EC. 2.3 0.1.16; 2.3.1.155; 2.3.1.9), β-oxidation of fatty acids C8- > C6 (FAOXC8C6x, EC. 4.2.1.17; 5.3.3.8; 1.1.1.35), β-oxidation of fatty acids C6- > C4 (FAOXC6C4x, EC. 1.1.1.n12; 4.2.1.107; 4.2.1.119) and r0735, thus affecting the oxidation of fatty acids and inducing their accumulation in the endoplasmic reticulum (Yang et al., 1987; Piccolis et al., 2019; Souza et al., 2019). The preceding leads to the upregulation of ketone bodies formation through directional coupling and change in metabolic flow to reactions associated with mitochondrial enzymes HMG-CoA synthase, in our case hydroxymethylglutaryl-CoA synthase (HMGCOASim, EC. 2.3.3.10) and 3-hydroxy-3-methylglutaryl coenzyme A (HMG-CoA) (HMGCOAtm) (Foll and Levin 2016; Farmer et al., 2020).
On the other hand, the glucose carbons can also be used to synthesize cysteine, which, together with the glutamate (Ex_glu_L in the model) used for the synthesis of glutamine, allows the synthesis of glutathione (GSH), which is essential for redox buffering(M. Yang and Vousden 2016). Additionally, the oxidative stress generated by PA can be regulated by repressing glucose metabolism, decreasing NADPH-dependent extracellular ROS production (Arnedo et al., 2011; Vicente-Gutierrez et al., 2019; Rose et al., 2020).
As mentioned before, reactions associated with the folate pathway have been previously determined to be essential in the lipotoxic scenario. This pathway supports the transfer of carbon units from serine for biosynthetic processes. However, when this pathway is negatively modulated, a decrease in NADPH biosynthesis is generated due to NAD + deficiency (Bailey and Gregory 1999; Field et al., 2014). Consistently with those findings, our model predicts the regulation of formate-tetrahydrofolate ligase reaction (FTHFLm, EC. 1.1.1.300), which participates in the transfer of carbon units, essential for several biosynthetic pathways. Additionally, it is directly related to the above-discussed MTHFD, which in the presence of an insult can affect mitochondrial maintenance functions and cellular homeostasis (Bailey and Gregory 1999; Coppedè et al., 2006; Coppedè 2021; Shi et al., 2021).
As we showed previously, one of the metabolic pathways that has more directional and inhibitory couplings with other pathways is the folate cycle. Other metabolic switches associated with different NADPH-dependent metabolic pathways are also altered, such as the reaction related to GMP reductase (Field et al., 2014; Rose et al., 2020). GMP reductase catalyzes the irreversible deamination of GMP for the conversion of guanosine nucleotides into inosine nucleotides, precursors of adenosine nucleotides, which maintain intracellular nucleotide balance (Ipata and Tozzi 2006). We found that the reaction associated with Glycine hydroxymethyltransferase (GHMT2r, EC. 2.1.2.1) is represented as critical since it is part of the polyglutamylation of folate, but it is also fully and directionally coupled to purine, serine, alanine, and threonine metabolism (Fell 2005; Yin 2015; Rose et al., 2020). Glycine is an important source for transferring carbon units through folate intermediates because a downward modulation would represent a negative modulation in the folate cycle (Yang and Vousden 2016; Rose et al., 2020). Finally, other reactions such as EX_pro_L(e), FORt2m (for mitochondrial formate transport), DGTPtn (Deoxyguanosine-triphosphate diffusion in the nucleus), and H2O transport are metabolic pathways that are indirectly modulated by palmitic acid and are essential for the maintenance of brain homeostasis (Leanza et al., 2008; Badaut 2010; Nagelhus and Ottersen 2013; Pietzke et al. 2020). It is important to take into account that the intermediates associated with each metabolic pathway provide precursors for the biosynthesis of several classes of molecules. Thus, any alteration that can modify biosynthetic processes alters astrocytic cellular homeostasis and neuronal-metabolic cooperation processes.
Although or model showed the control role (inhibitory) of the reactions associated with the glutamate-orotate antiporter (OROTGLUt), it is important to mention that in eukaryotic cells, the absence of this antiporter or its modulation prevents the uptake of orotic acid (OA), demonstrating a limiting capacity to use OA, and therefore, generating an inhibitory modulation of orotate transport (r0839) for the biosynthesis of RNA pyrimidines (Sonnewald et al., 1998; Fumagalli et al., 2017). Our model also found a modulation of the cysteine exchange (Ex_cys_L), which has several crucial roles in astrocytic cells by contributing to the production of GSH and palmitoylation (Young et al., 2012; Butland et al., 2014). In addition, palmitoylation also allows the adjustment of protein functions (Young et al., 2012).
Finally, within these 25 metabolic switches, we compared the metabolic changes between the PA metabolic model and the astrocytic model under tibolone pre-treatment, i.e., critical driver reactions in the PA model and redundant ones in the tibolone-treated cells and healthy astrocytes. In total, we found nine reactions of this type, including nuclear, peroxisomal, and extracellular transport, as well as glycolysis/gluconeogenesis and amino acid metabolism (Table 3). The fact that these reactions could be controlled by other reactions in the tibolone scenario but not in the inflammatory one implies that they would be key for protective treatment. Therefore, the possible role of decreasing inhibitory or competitive coupling of most reactions and the protective capacity of tibolone probably lay in these nine reactions. However, further research will be necessary to asses the importance of the metabolic switches proposed here.
TABLE 3. Set of critical reactions of the PA model that have a divergent role in the healthy and tibolone models.
In this study, we have focused on PA and its regulaory role in astrocytic cells at the multi-omic level. Updating a previously published genome-scale metabolic model, we performed an integration of transcriptomics and proteomics data by anovel approach. This approach allowed us to identify controller-type reactions that have previously been experimentally characterized as critical. These reactions showed an essential role in astrocytic metabolism, not only at the local level (where they play their primary function) but also by directly or indirectly controlling other reactions. We characterized these reactions as metabolic switches in the lipotoxic model, being key points for astrocyte homeostasis in a healthy state. These promising results highlight the feasibility of applying control theory to either postulate previously identified reactions or suggest new ones as possible study or treatment targets. This approach facilitated the understanding of the mechanistic aspects of control inside of the astrocytic cell, such as the inhibition of energy production through fatty acids β-oxidation or folate cycle (FTHFL and MTHFD reactions), switching to the formation of ketone bodies by upregulation of HMGCOASim, highly described mechanisms in stress processes generated by PA. Additionally, we found nuclear, peroxisomal, and extracellular transport, as well as glycolysis/gluconeogenesis and amino acid metabolism to be critical only in the PA condition but not in healthy or treated with tibolone astrocytes. Such information not only would provide a better understanding of the systemic level of the astrocytic response to PA and tibolone, but will also lay the foundations for future pharmacological investigations, guided to create more effective therapies, and in the control field, aimed to extend their applications in the biological phenomena,, such as the possible role of these metabolic switches as effectors of multi-omics oscillations, as it has been suggested for organisms such as Escherichia coli (Bardozzo et al., 2018). Finally, our results along with this study framework can be used for in silico exploring the mechanisms of astrocytic cell regulation, directing a more complex future experimental work in neurodegenerative diseases.
The data presented in the study are deposited in the Gene Expression Omnibus database repository, accession number GSE166500. Raw data used in this research was deposited at NCBI's SRA archive database. It is accessible through the following URL: https://www.ncbi.nlm.nih.gov/geo/query/acc.cgi?acc=GSE166500.
JG, AP and GB conceived of the presented idea. AP, AA-R, NM-M, and JR-M developed the theory and performed the computations. AP, AA, OH-L and AA-R verified the analytical methods. JG and AP encouraged AA, GB, NM-M, OH-L, JR-M and AA-R to investigate conceptual and critical advances in astrocyte omic integration-level computational models for utilizing control theory for identification. of controlling reactions and supervised the findings of this work. All authors discussed the results and contributed to the final manuscript.
This work was supported by the Pontificia Universidad Javeriana, Bogotá, Colombia, and Minciencias IDs 8845 and 20,304 to JG.
The authors declare that the research was conducted in the absence of any commercial or financial relationships that could be construed as a potential conflict of interest.
All claims expressed in this article are solely those of the authors and do not necessarily represent those of their affiliated organizations, or those of the publisher, the editors and the reviewers. Any product that may be evaluated in this article, or claim that may be made by its manufacturer, is not guaranteed or endorsed by the publisher.
The Supplementary Material for this article can be found online at: https://www.frontiersin.org/articles/10.3389/fsysb.2022.896265/full#supplementary-material
Agostinho, P., A. CunhaCunha, R., and Oliveira, C. (2010). Neuroinflammation, Oxidative Stress and the Pathogenesis of Alzheimers Disease. Cpd 16, 2766–2778. doi:10.2174/138161210793176572
Altenbuchinger, M., Zacharias, H. U., Solbrig, S., Schäfer, A., Büyüközkan, M., Schultheiß, U. T., et al. (2019). A Multi-Source Data Integration Approach Reveals Novel Associations between Metabolites and Renal Outcomes in the German Chronic Kidney Disease Study. Sci. Rep. 9 (1), 1–13. doi:10.1038/s41598-019-50346-2
Arevalo, M.-A., Azcoitia, I., and Garcia-Segura, L. M. (2015). The Neuroprotective Actions of Oestradiol and Oestrogen Receptors. Nat. Rev. Neurosci. 16 (1), 17–29. doi:10.1038/nrn3856
Arnedo, M., Ramos, M., Puisac, B., Concepcion, M., Teresa, E., Pie, A., et al. (2011). “Mitochondrial HMG-CoA Synthase Deficiency,” in Advances in the Study of Genetic Disorders. London: IntechOpen. doi:10.5772/22151
Ávila Rodriguez, M., Garcia-segura, L. M., Cabezas, R., Torrente, D., Capani, F., Gonzalez, J., et al. (2014). Tibolone Protects T98G Cells from Glucose Deprivation. J. Steroid Biochem. Mol. Biol. 144, 294–303. doi:10.1016/j.jsbmb.2014.07.009
Badaut, J. (2010). Aquaglyceroporin 9 in Brain Pathologies. Neuroscience 168 (4), 1047–1057. doi:10.1016/j.neuroscience.2009.10.030
Bailey, L. B., and Gregory, J. F. (1999). Folate Metabolism and Requirements. J. Nutr. 129, 779–782. doi:10.1093/jn/129.4.779
Balsa, E., Perry, E. A., Bennett, C. F., Jedrychowski, M., Gygi, S. P., Doench, J. G., et al. (2020). Defective NADPH Production in Mitochondrial Disease Complex I Causes Inflammation and Cell Death. Nat. Commun. 11, 2714. doi:10.1038/s41467-020-16423-1
Bardozzo, F., Lió, P., and Tagliaferri, R. (2018). A Study on Multi-Omic Oscillations in Escherichia coli Metabolic Networks. BMC Bioinform. 19 (Suppl. 7), 194. doi:10.1186/s12859-018-2175-5
Basler, G., Grimbs, S., Ebenhöh, O., Selbig, J., and Nikoloski, Z. (2012). Evolutionary Significance of Metabolic Network Properties. J. R. Soc. Interface. 9, 1168–1176. doi:10.1098/rsif.2011.0652
Basler, G., and Nikoloski, Z. (2011). JMassBalance: Mass-Balanced Randomization and Analysis of Metabolic Networks. Bioinformatics 27 (19), 2761–2762. doi:10.1093/bioinformatics/btr448
Basler, G., Nikoloski, Z., Larhlimi, A., Barabási, A.-L., and Liu, Y.-Y. (2016). Control of Fluxes in Metabolic Networks. Genome Res. 26 (7), 956–968. doi:10.1101/gr.202648.115
Bordbar, A., Monk, J. M., King, Z. A., and Palsson, B. O. (2014). Constraint-based Models Predict Metabolic and Associated Cellular Functions. Nat. Rev. Genet. 15, 107–120. doi:10.1038/nrg3643
Brunk, E., Sahoo, S., Zielinski, D. C., Altunkaya, A., Dräger, A., Mih, N., et al. (2018). Recon3D Enables a Three-Dimensional View of Gene Variation in Human Metabolism. Nat. Biotechnol. 36 (3), 272–281. doi:10.1038/nbt.4072
Burgard, A. P., Nikolaev, E. V., SchillingNikolaev, C. H., Maranas, C. D., and Maranas, Costas. D. (2004). Flux Coupling Analysis of Genome-Scale Metabolic Network Reconstructions. Genome Res. 14, 301–312. doi:10.1101/gr.1926504
Buskila, Y., Bellot-Saez, A., and Morley, J. W. (2019). Generating Brain Waves, the Power of Astrocytes. Front. Neurosci. 13 (October), 1–10. doi:10.3389/fnins.2019.01125
Butland, S. L., Sanders, S. S., Schmidt, M. E., Riechers, S.-P., Lin, D. T. S., Martin, D. D. O., et al. (2014). The Palmitoyl Acyltransferase HIP14 Shares a High Proportion of Interactors with Huntingtin: Implications for a Role in the Pathogenesis of Huntington's Disease. Hum. Mol. Genet. 23 (15), 4142–4160. doi:10.1093/hmg/ddu137
Bylicky, M. A., Mueller, G. P., and Day, R. M. (2018). Mechanisms of Endogenous Neuroprotective Effects of Astrocytes in Brain Injury. Oxidative Med. Cell. Longev. 2018, 1–16. doi:10.1155/2018/6501031
Cabezas, R., El-Bachá, R. S., González, J., and Barreto, G. E. (2012). Mitochondrial Functions in Astrocytes: Neuroprotective Implications from Oxidative Damage by Rotenone. Neurosci. Res. 74 (2), 80–90. doi:10.1016/j.neures.2012.07.008
Carta, G., Murru, E., Banni, S., and Manca, C. (2017). Palmitic Acid: Physiological Role, Metabolism and Nutritional Implications. Front. Physiol. 8 (NOV), 1–14. doi:10.3389/fphys.2017.00902
Chaudhry, F. A., Krizaj, D., Larsson, P., Reimer, R. J., Wreden, C., Storm-Mathisen, J., et al. (2001). Coupled and Uncoupled Proton Movement by Amino Acid Transport System N. EMBO J. 20 (24), 7041–7051. doi:10.1093/emboj/20.24.7041
Chaudhry, F. A., Reimer, R. J., Krizaj, D., Barber, D., Storm-Mathisen, J., Copenhagen, D. R., et al. (1999). Molecular Analysis of System N Suggests Novel Physiological Roles in Nitrogen Metabolism and Synaptic Transmission. Cell. 99 (7), 769–780. doi:10.1016/S0092-8674(00)81674-8
Coppedè, F., Mancuso, M., Siciliano, G., Migliore, L., and Murri, L. (2006). Genes and the Environment in Neurodegeneration. Biosci. Rep. 26 (5), 341–367. doi:10.1007/s10540-006-9028-6
Coppedè, F. (2021). One-Carbon Epigenetics and Redox Biology of Neurodegeneration. Free Radic. Biol. Med. 170 (October), 19–33. doi:10.1016/j.freeradbiomed.2020.12.002
Crespo-Castrillo, A., Garcia-SeguraGarcia-Segura, L.-M., and Arevalo, M.-A. (2020). The Synthetic Steroid Tibolone Exerts Sex-specific Regulation of Astrocyte Phagocytosis under Basal Conditions and after an Inflammatory Challenge. J. Neuroinflammation 17 (1), 1–14. doi:10.1186/s12974-020-1719-6
Currais, A., Goldberg, J., Farrokhi, C., Chang, M., Prior, M., Dargusch, R., et al. (2015). A Comprehensive Multiomics Approach toward Understanding the Relationship between Aging and Dementia. Aging 7 (11), 937–955. doi:10.18632/aging.100838
Das, A., Banik, N. L., and Ray, S. K. (2010). Flavonoids Activated Caspases for Apoptosis in Human Glioblastoma T98G and U87MG Cells but not in Human Normal Astrocytes. Cancer 116 (1), 164–176. doi:10.1002/cncr.24699
David, L., Marashi, S.-A., Larhlimi, A., Mieth, B., and Bockmayr, A. (2011). FFCA: A Feasibility-Based Method for Flux Coupling Analysis of Metabolic Networks. BMC Bioinforma. 12 (1), 236. doi:10.1186/1471-2105-12-236
de Carvalho, C., and Caramujo, M. J. (2018). The Various Roles of Fatty Acids. Molecules 23 (10), 2583. doi:10.3390/molecules23102583
Devkota, P., and Wuchty, S. (2020). Controllability Analysis of Molecular Pathways Points to Proteins that Control the Entire Interaction Network. Sci. Rep. 10 (1), 1–9. doi:10.1038/s41598-020-59717-6
Dhandapani, K. M., Wade, F. M., Mahesh, V. B., and Brann, D. W. (2005). Astrocyte-Derived Transforming Growth Factor-β Mediates the Neuroprotective Effects of 17β-Estradiol: Involvement of Nonclassical Genomic Signaling Pathways. Endocrinology 146 (6), 2749–2759. doi:10.1210/en.2005-0014
Doengi, M., Hirnet, D., Philippe, C., Pape, H., Deitmer, J. W., and Lohr, C. (2009). GABA Uptake-dependent Ca (2+) Signaling in Developing Olfactory Bulb Astrocytes. Proc. Natl. Acad. Sci. U. S. A. 106 (41), 17570–17575. doi:10.1073/pnas.0809513106
Duarte, N. C., Becker, S. A., Jamshidi, N., Thiele, I., Mo, M. L., ThuyVo, D., et al. (2007). Global Reconstruction of the Human Metabolic Network Based on Genomic and Bibliomic Data. Proc. Natl. Acad. Sci. U. S. A. 104 (6), 1777–1782. doi:10.1073/pnas.0610772104
Dupuis, J. R., Ruiz‐Arce, R., Barr, N. B., Thomas, D. B., and Geib, S. M. (2019). Range‐wide Population Genomics of the Mexican Fruit Fly: Toward Development of Pathway Analysis Tools. Evol. Appl. 12 (8), 1641–1660. doi:10.1111/eva.12824
Farmer, B. C., WalshWalsh, A. E., Kluemper, J. C., and Johnson, L. A. (2020). Lipid Droplets in Neurodegenerative Disorders. Front. Neurosci. 14 (July), 1–14. doi:10.3389/fnins.2020.00742
Fatima, S., Hu, X., Gong, R.-H., Huang, C., Chen, M., Wong, H. L. X., et al. (2019). Palmitic Acid Is an Intracellular Signaling Molecule Involved in Disease Development. Cell. Mol. Life Sci. 76 (13), 2547–2557. doi:10.1007/s00018-019-03092-7
Fell, D. A. (2005). Enzymes, Metabolites and Fluxes. J. Exp. Bot. 56 (410), 267–272. doi:10.1093/jxb/eri011
Field, M. S., Kamynina, E., Agunloye, O. C., Liebenthal, R. P., Lamarre, S. G., Brosnan, M. E., et al. (2014). Nuclear Enrichment of Folate Cofactors and Methylenetetrahydrofolate Dehydrogenase 1 (MTHFD1) Protect De Novo Thymidylate Biosynthesis during Folate Deficiency. J. Biol. Chem. 289 (43), 29642–29650. doi:10.1074/jbc.M114.599589
Flott, B., and Seifert, W. (1991). Characterization of Glutamate Uptake Systems in Astrocyte Primary Cultures from Rat Brain. Glia 4 (3), 293–304. doi:10.1002/glia.440040307
Frago, L. M., Canelles, S., Freire-Regatillo, A., Argente-Arizón, P., Barrios, V., Argente, J., et al. (2017). Sandra Canelles, Alejandra Freire-Regatillo, Pilar Argente-Arizón, Vicente Barrios, Jesús Argente, Luis M. Garcia-Segura, and Julie A. ChowenEstradiol Uses Different Mechanisms in Astrocytes from the Hippocampus of Male and Female Rats to Protect against Damage Induced by Palmitic Acid. Front. Mol. Neurosci. 10 (October), 1–17. doi:10.3389/fnmol.2017.00330
Fumagalli, M., Lecca, D., Abbracchio, M. P., and Ceruti, S. (2017). Pathophysiological Role of Purines and Pyrimidines in Neurodevelopment: Unveiling New Pharmacological Approaches to Congenital Brain Diseases. Front. Pharmacol. 8 (DEC), 1–18. doi:10.3389/fphar.2017.00941
Gelius-Dietrich, G., Desouki, A. A., Fritzemeier, C. J., and Lercher, M. J. (2013). Sybil--Efficient Constraint-Based Modelling in R. BMC Syst. Biol. 7 (November), 125. doi:10.1186/1752-0509-7-125
Gianchandani, E. P., Chavali, A. K., PapinChavali, J. A., and Papin, Jason. A. (2010). The Application of Flux Balance Analysis in Systems Biology. WIREs Mech. Dis. 2, 372–382. doi:10.1002/wsbm.60
González, J., Pinzón, A., Angarita-Rodríguez, A., Aristizabal, A. F., Barreto, G. E., and Martín-Jiménez, C. (2020). Advances in Astrocyte Computational Models: From Metabolic Reconstructions to Multi-Omic Approaches. Front. Neuroinform. 14 (August), 1–13. doi:10.3389/fninf.2020.00035
González-Giraldo, Y., Forero, D. A., Echeverria, V., Garcia-SeguraBarreto, L. M., and Barreto, G. E. (2019). Tibolone Attenuates Inflammatory Response by Palmitic Acid and Preserves Mitochondrial Membrane Potential in Astrocytic Cells through Estrogen Receptor Beta. Mol. Cell. Endocrinol. 486 (February), 65–78. doi:10.1016/j.mce.2019.02.017
Green, D. R., Galluzzi, L., and Kroemer, G. (2014). Cell Biology. Metabolic Control of Cell Death. Science 345, 1250256. doi:10.1126/science.1250256
Gu, C., Kim, G. B., Kim, W. J., Kim, H. U., and LeeLee, S. Y. (2019). Current Status and Applications of Genome-Scale Metabolic Models. Genome Biol. 20 (1), 1–18. doi:10.1186/s13059-019-1730-3
Haroon, E., Miller, A. H., and Sanacora, G. (2017). Inflammation, Glutamate, and Glia: A Trio of Trouble in Mood Disorders. Neuropsychopharmacol 42, 193–215. doi:10.1038/npp.2016.199
Hashimoto, M., and Hossain, S. (2018). “Fatty Acids: From Membrane Ingredients to Signaling Molecules,” in Biochemistry and Health Benefits of Fatty Acids. London: IntechOpen. doi:10.5772/intechopen.80430
Hidalgo‐Lanussa, O., Baez‐Jurado, E., Echeverria, V., Ashraf, G. M., Sahebkar, A., Garcia‐Segura, L. M., et al. (2020). Lipotoxicity, Neuroinflammation, Glial Cells and Oestrogenic Compounds. J. Neuroendocrinol. 32 (1), 1–15. doi:10.1111/jne.12776
Hidalgo-lanussa, O., Ávila-Rodriguez, M., Baez-jurado, E., Zamudio, J., Echeverria, V., Garcia-segura, L. M., et al. (2017). Tibolone Reduces Oxidative Damage and Inflammation in Microglia Stimulated with Palmitic Acid through Mechanisms Involving Estrogen Receptor Beta. Mol. Neurobiol. 55, 5462–5477. doi:10.1007/s12035-017-0777-y
Hilton, B. J., Lang, B. T., and CreggCregg, J. M. (2012). Keratan Sulfate Proteoglycans in Plasticity and Recovery after Spinal Cord Injury. J. Neurosci. 32 (13), 4331–4333. doi:10.1523/JNEUROSCI.0333-12.2012
Huang, J., Hou, J., Li, L., and Wang, Y. (2020). Flux Balance Analysis of Glucose Degradation by Anaerobic Digestion in Negative Pressure. Int. J. Hydrogen Energy 45 (51), 26822–26830. doi:10.1016/j.ijhydene.2020.07.053
Huang, Y.-N., Lai, C.-C., Chiu, C.-T., Lin, J.-J., and Wang, J.-Y. (2014). L-ascorbate Attenuates the Endotoxin-Induced Production of Inflammatory Mediators by Inhibiting MAPK Activation and NF-Κb Translocation in Cortical Neurons/Glia Cocultures. PLoS ONE 9 (7), e97276–12. doi:10.1371/journal.pone.0097276
Hyduke, D., Hyduke, D., Schellenberger, J., Que, R., Fleming, R., Thiele, I., et al. (2011). COBRA Toolbox 2.0. Protoc. Exch. doi:10.1038/protex.2011.234
Ipata, P. L., and Tozzi, M. G. (2006). Recent Advances in Structure and Function of Cytosolic IMP-GMP Specific 5′nucleotidase II (cN-II). Purinergic Signal. 2 (4), 669–675. doi:10.1007/s11302-006-9009-z
Ito, Z., Sakamoto, K., Imagama, S., Matsuyama, Y., Zhang, H., Hirano, K., et al. (2010). N-acetylglucosamine 6-O-Sulfotransferase-1-Deficient Mice Show Better Functional Recovery after Spinal Cord Injury. J. Neurosci. 30 (17), 5937–5947. doi:10.1523/JNEUROSCI.2570-09.2010
Jiang, P., Wang, H., Li, W., Zang, C., Li, B., Wong, Y. J., et al. (2015). Network Analysis of Gene Essentiality in Functional Genomics Experiments. Genome Biol. 16 (1), 1–10. doi:10.1186/s13059-015-0808-9
Jones, L. L., and Tuszynski, M. H. (2002). Spinal Cord Injury Elicits Expression of Keratan Sulfate Proteoglycans by Macrophages, Reactive Microglia, and Oligodendrocyte Progenitors. J. Neurosci. 22 (11), 4611–4624. doi:10.1523/jneurosci.22-11-04611.2002
Karahalil, B. (2017). Overview of Systems Biology and Omics Technologies. Curr. Med. Chem. 23 (37), 4221–4230. doi:10.2174/0929867323666160926150617
Kim, M., Rai, N., Zorraquino, V., and Tagkopoulos, I. (2016). Multi-omics Integration Accurately Predicts Cellular State in Unexplored Conditions for Escherichia coli. Nat. Commun. 7, 1–12. doi:10.1038/ncomms13090
Larhlimi, A., David, L., Selbig, J., and Alexander, B. (2012). F2C2 : A Fast Tool for the Computation of Flux Coupling in Genome-Scale Metabolic Networks. BMC Bioinforma. 13, 57. doi:10.1186/1471-2105-13-57
Le Foll, C., and Levin, B. E. (2016). Fatty Acid-Induced Astrocyte Ketone Production and the Control of Food Intake. Am. J. Physiology-Regulatory, Integr. Comp. Physiology 310 (11), R1186–R1192. doi:10.1152/ajpregu.00113.2016
Leanza, L., Ferraro, P., Reichard, P., and Bianchi, V. (2008). Metabolic Interrelations within Guanine Deoxynucleotide Pools for Mitochondrial and Nuclear DNA Maintenance. J. Biol. Chem. 283 (24), 16437–16445. doi:10.1074/jbc.M801572200
Li, K., Li, J., Zheng, J., and Qin, S. (2019). Reactive Astrocytes in Neurodegenerative Diseases. Aging Dis. 10 (3), 664. doi:10.14336/ad.2018.0720
Li, X., Meng, L., Tian, L., Chen, J., Liu, R., and Ning, B. (2020). Review Article Reactive Astrogliosis : Implications in Spinal Cord Injury Progression and Therapy. Oxid. Med. Cell. Longev. 2020, 9494352. doi:10.1155/2020/9494352
Luterman, J. D., Haroutunian, V., Yemul, S., Ho, L., Purohit, D., AisenAisen, P. S., et al. (2000). Cytokine Gene Expression as a Function of the Clinical Progression of Alzheimer Disease Dementia. Arch. Neurol. 57 (8), 1153–1160. doi:10.1001/archneur.57.8.1153
Maarleveld, T. R., Khandelwal, R. A., Olivier, B. G., Teusink, B., and Bruggeman, F. J. (2013). Basic Concepts and Principles of Stoichiometric Modeling of Metabolic Networks. Biotechnol. J. 8 (9), 997–1008. doi:10.1002/biot.201200291
Mahmoud, S., Gharagozloo, M., Simard, C., and Gris, D. (2019). Astrocytes Maintain Glutamate Homeostasis in the CNS by Controlling the Balance between Glutamate Uptake and Release. Cells 8, 184. doi:10.3390/cells8020184
Martín-Jiménez, C. A., Salazar-Barreto, D., Barreto, G. E., González, J. B., and González, J. (2017). Genome-Scale Reconstruction of the Human Astrocyte Metabolic Network. Front. Aging Neurosci. 9 (FEB), 1–17. doi:10.3389/fnagi.2017.00023
Martin-jiménez, C., and González, J. (2020). Tibolone Ameliorates the Lipotoxic Effect of Palmitic Acid in Normal Human Astrocytes. Neurotox. Res. 38 (3), 585–595. doi:10.1007/s12640-020-00247-4
Marttinen, M., Paananen, J., Neme, A., Mitra, V., Takalo, M., Natunen, T., et al. (2019). A Multiomic Approach to Characterize the Temporal Sequence in Alzheimer's Disease-Related Pathology. Neurobiol. Dis. 124, 454–468. doi:10.1016/j.nbd.2018.12.009
McCloskey, D., Palsson, B. Ø., and Feist, A. M. (2013). Basic and Applied Uses of Genome‐scale Metabolic Network Reconstructions of Escherichia coli. Mol. Syst. Biol. 9 (1), 661. doi:10.1038/msb.2013.18
Melo, H. M., Santos, L. E., and Ferreira, S. T. (2019). Diet-Derived Fatty Acids, Brain Inflammation, and Mental Health. Front. Neurosci. 13 (March), 1–12. doi:10.3389/fnins.2019.00265
Moncada, S., and Higgs, A. (1993). The L-Arginine-Nitric Oxide Pathway. N. Engl. J. Med. 329, 2002–2012. doi:10.1056/NEJM199312303292706
Nagelhus, E. A., and Ottersen, O. P. (2013). Physiological Roles of Aquaporin-4 in Brain. Physiol. Rev. 93 (4), 1543–1562. doi:10.1152/physrev.00011.2013
Nielsen, J. (2017). Systems Biology of Metabolism: A Driver for Developing Personalized and Precision Medicine. Cell. Metab. 25 (3), 572–579. doi:10.1016/j.cmet.2017.02.002
Niu, Y.-C., Feng, R.-N., Hou, Y., Li, K., Kang, Z., Wang, J., et al. (2012). Histidine and Arginine Are Associated with Inflammation and Oxidative Stress in Obese Women. Br. J. Nutr. 108 (1), 57–61. doi:10.1017/S0007114511005289
Orth, J. D., Conrad, T. M., Na, J., Lerman, J. A., Nam, H., Feist, A. M., et al. (2011). A Comprehensive Genome‐scale Reconstruction of Escherichia coli Metabolism-2011. Mol. Syst. Biol. 7 (535), 535–539. doi:10.1038/msb.2011.65
Orth, J. D., Thiele, I., and Palsson, B. Ø. (2010). What Is Flux Balance Analysis? Nat. Biotechnol. 28 (3), 245–248. doi:10.1038/nbt.1614
Ortiz-Rodriguez, A., Acaz-Fonseca, E., Boya, P., Arevalo, M. A., and Garcia-Segura, L. M. (2019). Ana, Estefania Acaz-Fonseca, Patricia Boya, Maria Angeles Arevalo, and Luis M Garcia-SeguraLipotoxic Effects of Palmitic Acid on Astrocytes Are Associated with Autophagy Impairment. Mol. Neurobiol. 56 (3), 1665–1680. doi:10.1007/s12035-018-1183-9
Osorio, D., Botero, K., Gonzalez, J., and Pinzon, A. (2016). ‘exp2flux’ Convierte Datos de Gene EXPression a FBA FLUXes. Package Version 0.1. doi:10.13140/RG.2.2.14401.56168
Osorio, D., Pinzón, A., Martín-Jiménez, C., Barreto, G. E., and González, J. (2020). Multiple Pathways Involved in Palmitic Acid-Induced Toxicity: A System Biology Approach. Front. Neurosci. 13 (January), 1–14. doi:10.3389/fnins.2019.01410
Papin, J. A., Hunter, T., Palsson, B. O., and Subramaniam, S. (2005). Reconstruction of Cellular Signalling Networks and Analysis of Their Properties. Nat. Rev. Mol. Cell. Biol. 6 (2), 99–111. doi:10.1038/nrm1570
Pardo, B., Contreras, L., and Satrústegui, J. (2013). De Novo Synthesis of Glial Glutamate and Glutamine in Young Mice Requires Aspartate provided by the Neuronal Mitochondrial Aspartate-Glutamate Carrier Aralar/AGC1. Front. Endocrinol. 4 (October), 15–18. doi:10.3389/fendo.2013.00149
Patil, S., Melrose, J., and Chan, C. (2007). Involvement of Astroglial Ceramide in Palmitic Acid-Induced Alzheimer-like Changes in Primary Neurons. Eur. J. Neurosci. 26 (8), 2131–2141. doi:10.1111/j.1460-9568.2007.05797.x
Patil, S., Sheng, L., Masserang, A., and Chan, C. (2006). Palmitic Acid-Treated Astrocytes Induce BACE1 Upregulation and Accumulation of C-Terminal Fragment of APP in Primary Cortical Neurons. Neurosci. Lett. 406 (1–2), 55–59. doi:10.1016/j.neulet.2006.07.015
Piccolis, M., Bond, L. M., Kampmann, M., Pulimeno, P., Chitraju, C., JaysonJayson, C. B. K., et al. (2019). Probing the Global Cellular Responses to Lipotoxicity Caused by Saturated Fatty Acids. Mol. Cell. 74 (1), 32–44. e8. doi:10.1016/j.molcel.2019.01.036
Pietzke, M., Meiser, J., and Vazquez, A. (2020). Formate Metabolism in Health and Disease. Mol. Metab. 33, 23–37. doi:10.1016/j.molmet.2019.05.012
Pinu, F. R., Beale, D. J., Paten, A. M., Kouremenos, K., Swarup, S., Schirra, H. J., et al. (2019). Systems Biology and Multi-Omics Integration: Viewpoints from the Metabolomics Research Community. Metabolites 9 (4), 76–31. doi:10.3390/metabo9040076
Ramon, C., Gollub, M. G., and Stelling, J. (2018). Integrating -Omics Data into Genome-Scale Metabolic Network Models: Principles and Challenges. Essays Biochem. 62 (4), 563–574. doi:10.1042/EBC20180011
Ravindran, V., Nacher, J. C., Akutsu, T., Ishitsuka, M., Osadcenco, A., Sunitha, V., et al. (2019). Network Controllability Analysis of Intracellular Signalling Reveals Viruses Are Actively Controlling Molecular Systems. Sci. Rep. 9 (1), 1–11. doi:10.1038/s41598-018-38224-9
Rezola, A., PeyTobalina, J., Tobalina, L., Rubio, A., Beasley, J. E., and Planes, F. J. (2015). Advances in Network-Based Metabolic Pathway Analysis and Gene Expression Data Integration. Briefings Bioinforma. 16 (2), 265–279. doi:10.1093/bib/bbu009
Rodríguez-Mier, P., Poupin, N., de Blasio, C., Le Cam, L., and Jourdan, F. (2021). DEXOM: Diversity-Based Enumeration of Optimal Context-specific Metabolic Networks. PLoS Comput. Biol. 17, e1008730. doi:10.1371/journal.pcbi.1008730
Rose, J., Brian, C., Pappa, A., Panayiotidis, M. I., and Franco, R. (2020). Mitochondrial Metabolism in Astrocytes Regulates Brain Bioenergetics, Neurotransmission and Redox Balance. Front. Neurosci. 14 (November), 1–20. doi:10.3389/fnins.2020.536682
Schafer, J. R. A., Fell, D. A., Rothman, D., and Shulman, R. G. (2004). Protein Phosphorylation Can Regulate Metabolite Concentrations rather Than Control Flux: The Example of Glycogen Synthase. Proc. Natl. Acad. Sci. U.S.A. 101 (6), 1485–1490. doi:10.1073/pnas.0307299101
Schousboe, A., Bak, L. K., and Waagepetersen, H. S. (2013). Astrocytic Control of Biosynthesis and Turnover of the Neurotransmitters Glutamate and GABA. Front. Endocrinol. 4 (AUG), 1–11. doi:10.3389/fendo.2013.00102
Schuetz, R., Kuepfer, L., and Sauer, U. (2007). Systematic Evaluation of Objective Functions for Predicting Intracellular Fluxes in Escherichia Coli. Mol. Syst. Biol. 3 (119), 119. doi:10.1038/msb4100162
Schuller-levis, G. B., and Park, E. (2003). , 226, 195–202. doi:10.1016/S0378-1097(03)00611-6Taurine : New Implications for an Old Amino AcidFEMS Microbiol. Lett.
Segrè, D., Vitkup, D., and Church, G. M. (2002). Analysis of Optimality in Natural and Perturbed Metabolic Networks. Proc. Natl. Acad. Sci. U.S.A. 99 (23), 15112–15117. doi:10.1073/pnas.232349399
Shi, L.-f., Zhang, Q., ShouShou, X.-y., and Niu, H.-j. (2021). Expression and Prognostic Value Identification of Methylenetetrahydrofolate Dehydrogenase 2 (Mthfd2) in Brain Low-Grade Glioma. Ijgm Vol. 14, 4517–4527. doi:10.2147/IJGM.S323858
Shlomi, T., Berkman, O., and Ruppin, E. (2005). Regulatory On/off Minimization of Metabolic Flux Changes after Genetic Perturbations. Proc. Natl. Acad. Sci. U.S.A. 102 (21), 7695–7700. doi:10.1073/pnas.0406346102
Sofroniew, M. V. (2009). Molecular Dissection of Reactive Astrogliosis and Glial Scar Formation. Trends Neurosci. 32 (12), 638–647. doi:10.1016/j.tins.2009.08.002.Molecular
Son, D. O., Satsu, H., and Shimizu, M. 2005, Histidine Inhibits Oxidative Stress- and TNF-α-Induced Interleukin-8 Secretion in Intestinal Epithelial Cells. FEBS Lett. 579, 4671–4677. doi:10.1016/j.febslet.2005.07.038
Sonnewald, U., Akiho, H., Koshiya, K., and Iwai, A. (1998). Effect of Orotic Acid on the Metabolism of Cerebral Cortical Astrocytes during Hypoxia and Reoxygenation: An NMR Spectroscopy Study. J. Neurosci. Res. 51 (1), 103–108. doi:10.1002/(sici)1097-4547(19980101)51:1<103:aid-jnr11>3.0.co;2-c
Souders, C. L., Zubcevic, J., and Martyniuk, C. J. (2021). Tumor Necrosis Factor Alpha and the Gastrointestinal Epithelium: Implications for the Gut-Brain Axis and Hypertension. Cell. Mol. Neurobiol. 42 (419)–437. doi:10.1007/s10571-021-01044-z
Souza, D. G., Almeida, R. F., SouzaSouza, D. O., and Zimmer, E. R. (2019). The Astrocyte Biochemistry. Seminars Cell. & Dev. Biol. 95 (April), 142–150. doi:10.1016/j.semcdb.2019.04.002
Sweetlove, L. J., and Ratcliffe, R. G. (2011). Flux-Balance Modeling of Plant Metabolism. Front. Plant Sci. 2 (AUG), 1–10. doi:10.3389/fpls.2011.00038
Terzer, M., and Stelling, J. (2008). Large-Scale Computation of Elementary Flux Modes with Bit Pattern Trees. Bioinformatics 24 (19), 2229–2235. doi:10.1093/bioinformatics/btn401
Verkhratsky, A., and Butt, A. (2018). The History of the Decline and Fall of the Glial Numbers Legend. Neuroglia 1 (1), 188–192. doi:10.3390/neuroglia1010013
Vicente-Gutierrez, C., Bonora, N., Bobo-Jimenez, V., Jimenez-Blasco, D., Lopez-Fabuel, I., Fernandez, E., et al. (2019). Astrocytic Mitochondrial ROS Modulate Brain Metabolism and Mouse Behaviour. Nat. Metab. 1 (2), 201–211. doi:10.1038/s42255-018-0031-6
Voillet, V., Besse, P., Liaubet, L., San Cristobal, M., and González, I. (2016). Handling Missing Rows in Multi-Omics Data Integration: Multiple Imputation in Multiple Factor Analysis Framework. BMC Bioinforma. 17, 402. doi:10.1186/s12859-016-1273-5
Volterra, A., and Meldolesi, J. (2005). Astrocytes, from Brain Glue to Communication Elements: The Revolution Continues. Nat. Rev. Neurosci. 6 (8), 626–640. doi:10.1038/nrn1722
Wong, K.-L., Wu, Y.-R., Cheng, K.-S., Chan, P., Cheung, C.-W., Lu, D.-Y., et al. (2014). Palmitic Acid-Induced Lipotoxicity and Protection by (+)-Catechin in Rat Cortical Astrocytes. Pharmacol. Rep. 66 (6), 1106–1113. doi:10.1016/j.pharep.2014.07.009
Wörheide, M. A., Krumsiek, J., Kastenmüller, G., and Arnold, M. (2021). Multi-omics Integration in Biomedical Research - A Metabolomics-Centric Review. Anal. Chim. Acta 1141, 144–162. doi:10.1016/j.aca.2020.10.038
Yang, M., and Vousden, K. H. (2016). Serine and One-Carbon Metabolism in Cancer. Nat. Rev. Cancer 16 (10), 650–662. doi:10.1038/nrc.2016.81
Yang, S. Y., He, X. Y., and Schulz, H. (1987). Fatty Acid Oxidation in Rat Brain Is Limited by the Low Activity of 3-Ketoacyl-Coenzyme A Thiolase. J. Biol. Chem. 262 (27), 13027–13032. doi:10.1016/s0021-9258(18)45161-7
Yin, K. (2015). Positive Correlation between Expression Level of Mitochondrial Serine Hydroxymethyltransferase and Breast Cancer Grade. Ott 8, 1069–1074. doi:10.2147/OTT.S82433
Young, F. B., ButlandButland, S. L., Sanders, S. S., Sutton, L. M., and Hayden, M. R. (2012). Putting Proteins in Their Place: Palmitoylation in Huntington Disease and Other Neuropsychiatric Diseases. Prog. Neurobiol. 97 (2), 220–238. doi:10.1016/j.pneurobio.2011.11.002
Zhang, H., Muramatsu, T., Murase, A., Yuasa, S., Uchimura, K., and Kadomatsu, K. (2006a). N-acetylglucosamine 6-O-Sulfotransferase-1 Is Required for Brain Keratan Sulfate Biosynthesis and Glial Scar Formation after Brain Injury. Glycobiology 16 (8), 702–710. doi:10.1093/glycob/cwj115
Zhang, H., Uchimura, K., and Kadomatsu, K. (2006b). Brain Keratan Sulfate and Glial Scar Formation. Ann. N. Y. Acad. Sci. 1086, 81–90. doi:10.1196/annals.1377.014
Keywords: astrocytes, data integration, palmitic acid, computational model, multi-omics, control theory, genome scale model, neurodegeneration
Citation: Angarita-Rodríguez A, Mendoza-Mejía N, Gonzalez J, Aristizabal AF, Hidalgo-Lanussa O, Rubio-Mesa JJ, Barreto GE and Pinzon A (2022) Multi-Omics Integrative Analysis Coupled to Control Theory and Computational Simulation of a Genome-Scale metabolic Model Reveal Controlling Biological Switches in Human Astrocytes Under Palmitic Acid-Induced Lipotoxicity. Front. Syst. Biol. 2:896265. doi: 10.3389/fsysb.2022.896265
Received: 14 March 2022; Accepted: 25 April 2022;
Published: 30 May 2022.
Edited by:
Angelyn Lao, De La Salle University, PhilippinesReviewed by:
Francesco Bardozzo, University of Salerno, ItalyCopyright © 2022 Angarita-Rodríguez, Mendoza-Mejía, Gonzalez, Aristizabal, Hidalgo-Lanussa, Rubio-Mesa, Barreto and Pinzon. This is an open-access article distributed under the terms of the Creative Commons Attribution License (CC BY). The use, distribution or reproduction in other forums is permitted, provided the original author(s) and the copyright owner(s) are credited and that the original publication in this journal is cited, in accordance with accepted academic practice. No use, distribution or reproduction is permitted which does not comply with these terms.
*Correspondence: Andres Pinzon, YW1waW56b252QHVuYWwuZWR1LmNv
Disclaimer: All claims expressed in this article are solely those of the authors and do not necessarily represent those of their affiliated organizations, or those of the publisher, the editors and the reviewers. Any product that may be evaluated in this article or claim that may be made by its manufacturer is not guaranteed or endorsed by the publisher.
Research integrity at Frontiers
Learn more about the work of our research integrity team to safeguard the quality of each article we publish.