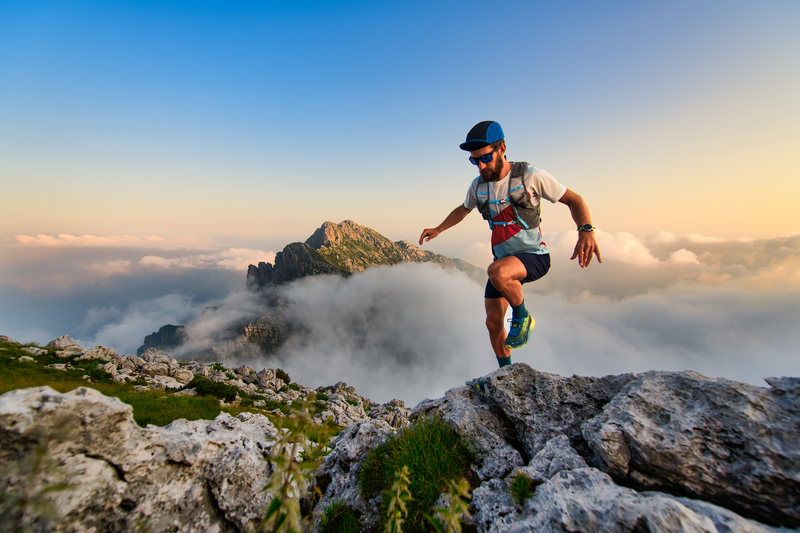
95% of researchers rate our articles as excellent or good
Learn more about the work of our research integrity team to safeguard the quality of each article we publish.
Find out more
ORIGINAL RESEARCH article
Front. Syst. Biol. , 29 April 2022
Sec. Data and Model Integration
Volume 2 - 2022 | https://doi.org/10.3389/fsysb.2022.893007
This article is part of the Research Topic Integrative Systems Biology and Big Data for Agricultural Improvement and Understanding View all 5 articles
Maple syrup, an emblematic food product of Canada is produced from the concentration of sap collected from maple trees during spring. During this season, the trees come out of dormancy, which modifies sap composition. Meanwhile, microorganisms that contaminate sap as it is collected can also modify its composition. As these two factors can impact the quality of maple syrup, we aimed to better understand how microbial communities vary along dormancy release. We estimated the absolute abundance of bacteria and fungi in maple sap along a dormancy release index using high-throughput amplicon sequencing and digital droplet PCR (ddPCR). Several members were identified as indicators of maple sap composition, syrup organoleptic conformity and color, some of which are also hubs in the microbial association networks. We further explored bacterial communities by performing a predictive functional analysis, revealing various metabolic pathways correlated to dormancy release. Finally, we performed an experimental investigation of maple sap carrying capacity and limiting nutrients along dormancy release and found that maple sap composition variation influences its carrying capacity. Taken together, our results indicate that an increase in nitrogen supply in the form of allantoate combined with possible metabolite excretion could lead microbial communities towards different paths. Indeed, we observed a greater heterogeneity during late dormancy release which in turn could explain the variation in maple syrup quality. Further experimental investigation into the contribution of microbial, vegetal, environmental, technological, and processing factors to the final composition of maple syrup will be required to improve our understanding of this complex and flavorful food matrix and to develop quality control strategies.
Maple sap is a natural nutrient matrix extracted mostly from sugar maple trees (Acer saccharum Marsh.) and sometimes from related species (Acer rubrum, Acer nigrum). Maple sap is used to produce maple syrup, an emblematic food product of Canada. Maple sap is mainly constituted of water and sucrose (∼2%), but its minor composition is complex (Lagacé et al., 2015). Among minor constituents, numerous organic compounds such as simple and complex carbohydrates, organic acids, ureides, amino acids, phenolic compounds, and vitamins as well as inorganic compounds including minerals, sulfate, ammonium, and phosphate have been reported in maple sap (Filteau et al., 2011; Lagacé et al., 2015; Filteau et al., 2017; N’guyen et al., 2018; van den Berg et al., 2019; Garcia et al., 2020). Variations in sap composition were observed as the harvesting season progresses, sucrose and phenolic compounds tend to decrease, while reducing sugars, organic acids, nitrogen and sulfur containing compounds increase (Morselli and Whalen, 1986; Filteau et al., 2011; Lagacé et al., 2015; N’guyen et al., 2018; Garcia et al., 2020).
The variation in maple sap composition can be influenced by environmental, vegetal, and microbial factors or their interactions. Indeed, in response to environmental cues, trees come out of their dormancy during spring, and their metabolism changes prior to bud break, influencing sap composition (Millard et al., 1998; Cooke et al., 2012; N’guyen et al., 2018). In turn, microorganisms contaminating the sap during collection and developing during its storage can alter its composition (Morselli and Whalen, 1991; Lagacé et al., 2002; Filteau et al., 2011; Lagacé et al., 2018). Indeed, sap is now mostly collected through networks of tubing in which mixed biofilms develop (Lagacé et al., 2006). Moreover, microorganisms may also respond to environmental conditions as the contamination levels increase during spring (Filteau et al., 2010; Lagacé et al., 2015). Finally, temperature could directly affect maple sap composition by influencing enzymatic activity. However, the environmental cues influencing dormancy release, sap flow and microbial responses may not be the same. The mechanism and factors underlying maple sap exudation are still not fully understood, but it is acknowledged that continuous freezing and thawing events after an extended period of sub-zero temperatures are required (Driller et al., 2020). Meanwhile, bud break in sugar maple trees can be predicted independently of freezing and thawing events (Raulier and Bernier, 2000). Therefore, we recently introduced the Sbb index, calculated from meteorological data to estimate the metabolic state of trees at the time of harvest (N’guyen et al., 2018). Because this index is based on environmental parameters influencing tree metabolism independently from sap flow, which was traditionally used as a referential for sample binning and comparison (Lagacé et al., 2004; Lagacé et al., 2006; Filteau et al., 2010; Lagacé et al., 2015), it provides a better referential to compare samples between different locations and years. Ultimately, this index helped to better understand maple composition variation with respect to tree dormancy release and associated maple syrup flavor defects (N’guyen et al., 2018). Here, we aim to investigate how microbial communities of maple sap vary along this index.
Maple sap is a favorable environment for some microorganisms with total viable count increasing over spring and reaching 5.5 to 7 log CFU/mL on average by the end of the flow period depending on the harvest year (Lagacé et al., 2004; Filteau et al., 2010; Lagacé et al., 2015). Whether the increasing temperatures during spring or changes in maple sap nutrient composition during dormancy release, or both underly the increase in microbial load is still unclear. Although, experimental evidence with a model yeast suggests that the ureide content, mostly allantoate, could play a pivoting role as the main nitrogen source (Filteau et al., 2017). The microbial communities growing in tubing biofilms and maple sap have been previously investigated with respect to the sap flow period with molecular profiling methods (Lagacé et al., 2004; Lagacé et al., 2006; Filteau et al., 2010; Filteau et al., 2011). The main bacteria observed forming biofilms in the plastic tubing were Pseudomonas spp. (Lagacé et al., 2006). In maple sap, the main group of bacteria identified belonged to the Pseudomonas, Rahnella, Janthinobacterium, Leuconostoc, Epilithonimonas, Chryseobacterium and Sphingomonas genera (Filteau et al., 2010; Filteau et al., 2011). The fungal community was dominated by yeast related to Mrakia spp., Mrakiella spp., Tausonia (Guehomyces) pullulans, Cryptococcus victoriae and Williopsis saturnus (Filteau et al., 2011).
Microorganisms found in maple sap can contribute to maple syrup properties such as color, flavor, and texture. For instance, enzymatic hydrolysis of sucrose caused by microbial activity leads to the presence of glucose and fructose in sap (Naghski et al., 1957; Morselli and Whalen, 1991; Lagacé et al., 2002). Those two reducing sugars react in Maillard reactions during the heating process, which contribute to color and flavor development of maple syrup (Alli et al., 1990; Nursten, 2007; Nimalaratne et al., 2020). However, beyond light transmittance, which is the official method used for maple syrup grade classification, little is known about the factor influencing the color components of maple syrup. Also, so far, few studies have explored the relationship between syrup flavors and microorganisms (Naghski et al., 1957; Willits et al., 1961; Filteau et al., 2012). Correlations between bacterial and fungal groups and major flavor families have been reported, including positive relationships between the relative abundance of Pseudomonas fluorescens group and Mrakia and maple and vanilla flavor intensity, along with vanillin concentration in syrup (Filteau et al., 2012). Vanillin, coniferol, and syringaldehyde in maple sap at the beginning of the flow period have been correlated to the relative abundance of Janthinobacterium lividum (Filteau et al., 2011). Microorganisms capable of causing syrup ropiness, a type of syrup defect, have also been recently identified as belonging to Leuconostoc mesenteroides species and Enterobacteriaceae family (Lagacé et al., 2018). Our previous work also suggests that microbial activity plays a role in the buddy defect appearance during late season production (N’guyen et al., 2018). However, the maple sap microbiota associated with downgraded syrups has not been explored yet. Altogether, microorganisms seem to be able to contribute both positively and negatively to maple syrup properties, but further research is necessary to fully understand this complex system.
The aim of this study was to describe how microbial communities vary along dormancy release and its impact on maple syrup quality. We performed a metataxonomic analysis on bacteria and fungi using next generation sequencing and digital droplet PCR (ddPCR) to estimate absolute abundance of microorganisms in maple sap along dormancy release as measured by the Sbb index. We explored microbial communities by performing an inter-kingdom association network and a predictive functional analysis in bacteria. Finally, we performed an experimental investigation of maple sap carrying capacity and limiting nutrients along dormancy release.
A first set of 47 concentrated maple sap from 11 sites harvested during the flow period in 2015, 2016, and 2017, and previously characterized in Filteau et al. (2017), N’guyen et al. (2018) were used in this study to explore sap bacterial and fungal communities. Those samples were collected on a voluntary basis from maple sap producers in Québec and New Brunswick. As is typical for maple syrup production, sap was collected from hundreds to thousands of trees, mainly Acer saccharum. Sap collection was achieved using a network of plastic tubing lines under vacuum, then concentrated by membrane processes. Sap concentrate samples were then collected right before the evaporation process and the corresponding syrup was sampled after the batch was processed. Samples were frozen on site at −20°C. Supplementary Data S1 summarizes the samples used in this study and their characteristics. The concentration (°Brix) was measured in laboratory with a digital refractometer and the average density of sap concentrate samples was 13.2 °Brix. To obtain the concentrated microbial biomass 50 mL aliquots of sap concentrate were rapidly thawed in a cold-water bath and then centrifugated for 5 min × 915 g at 4°C. The supernatant was partially removed to conserve 5 mL of the final solution and stored at −80°C until analysis. For these samples, we used the reported data for ammonium, organic sulfur, sulfate and phosphate quantification in sap (N’guyen et al., 2018). When available, we also used the corresponding syrup quality classification which was performed by trained analysts following the standard organoleptic assessment procedures officially used by the industry as described in N’guyen et al. (2018). Since the classification nomenclature is not informative for our purposes, we grouped samples by organoleptic conformity: standard (standard and allowing for light off-flavors (v), N = 11) and non-standard (1, 4, 5 and 6 defect types leading to product downgrading or rejection, N = 21).
For follow up experiments, a second set of 42 samples were collected in 2018 and 2019 from five other production sites following similar handling methods. Maple sap concentrated by membrane processing at the farm was frozen on site at −20°C until analysis. After thawing, saps were sterilized by 0.2 μM filtration before analysis.
Colorimetric analysis of available corresponding syrups (N = 37) using the CIELAB color space (L*a*b*) were performed in laboratory with a Spectrophotometer UV-2700 (Shimatzu, Kyoto, Japan) and the UVPC Color Analysis Software, version 3.10.
We previously introduced a dormancy release index representing the remaining Sum of cumulative temperature necessary to reach Bud Break (Sbb) as a temporal and meteorological reference to compare sap samples (N’guyen et al., 2018). It is important to note that this index is independent of the temperature occurring after the sap sample collection date, and thus allows for comparison between samples of different years. Meteorological records from the nearest stations were obtained from the Canadian government public records (http://climate.weather.gc.ca) and missing data were estimated using data from the nearest station available.
The DNA extraction was performed as in (Bleuven et al., 2020). A volume of 1 mL of sap concentrate was used for DNA extractions. Bacterial and fungal contamination levels were quantified by the number of 16S and ITS amplicon copy numbers by droplets digital PCR (ddPCR) with the QX200 droplet generator (Bio-Rad). The procedure was done according to the manufacturer recommendation (Taylor et al., 2015). Briefly, to adjust the DNA concentration of samples into the detection range of the ddPCR, a reference sample was made by pooling 2 µl of each sample. Then, 2 µl pooled sample was then amplified by RT-PCR in 7500 Real-Time PCR system (Life Technologies) with a mix made with 17 µl of PowerUp SYBR Green Master mix (Life Technologies) and 1 µl of 10 µM primers targeting the 16S V3-V4 region (Klindworth et al., 2013) or ITS2 region (Tedersoo et al. (2015). Each sample were diluted by a factor determined by adjusting the reference Ct value to 25Ct. After dilution, the ddPCR protocol (Taylor et al., 2015) was followed for each target, 16S or ITS. The PCR cycle was a first step at 95°C for 5 min followed by 30 cycles of denaturation at 95°C for 30 s, annealing at 56°C for 30 s, extension at 60°C for 30 s and a final stabilisation step at 4°C for 5 min and at 90°C for 5 min. The total DNA copy number of 16S and ITS were then used to estimate the absolute abundance of bacteria and fungi in each sample, respectively.
To explore the microbial community diversity, we amplified and sequenced the 16S subunit gene with primers covering the V3-V4 region (Klindworth et al., 2013) and ITS2 sequence specific regions described in Tedersoo et al. (2015) for bacteria and fungi, respectively. Amplification was performed in a two-step dual-index PCR approach specifically designed for Illumina instruments by the Plateforme d’Analyses Génomiques (IBIS, Université Laval, Quebec City, Canada) as in Bleuven et al. (2020). Briefly, primer sequences were designed by the fusion of the gene specific sequence and the Illumina TruSeq sequencing primers and PCR was carried out in a total volume of 25 µl that contains 1X Q5 buffer (NEB), 1X high CG content buffer, 0.25 µM of each primer, 200 µM of each dNTPs, 1 U of Q5 High-Fidelity DNA polymerase (NEB) and 1 µl of template DNA. The PCR started with an initial denaturation at 98°C for 30 s followed by 35 cycles of denaturation at 98°C for 10 s, annealing at 55°C for 10 s, extension at 72°C for 30 s and a final extension at 72°C for 2 min. Quality of the purified PCR product were checked on a 1% agarose gel. Fifty-fold dilution of this purified product was used as a template for a second PCR step with the goal of adding barcodes (dual-indexed) and the specific sequence required for Illumina sequencing. Cycling for the second PCR were identical to the first PCR with 12 cycles. PCR reactions were checked for quality on a DNA7500 Bioanalyzer chip (Agilent). Barcoded Amplicons were then pooled in equimolar concentration based on agarose gel band intensity, purified with the Axygen PCR cleanup kit (Axygen) and sequenced on the illumina Miseq (paired–end 300 bases with two index reads) at the Plateforme d’analyses génomiques (IBIS, Université Laval, Quebec City, Canada). Please note that primers used in this work contain Illumina specific sequences protected by intellectual property (Oligonucleotide sequences © 2007–2013 Illumina, Inc. All rights reserved. Derivative works created by Illumina customers are authorized for use with Illumina instruments and products only. All other uses are strictly prohibited.) The 16S and ITS amplicons were sequenced in two independent Illumina runs.
The bioinformatics workflow applied for this study was similar to (Bleuven et al., 2020). Raw sequences obtained from the Illumina sequencing were first demultiplexed and sequences with low length were removed following the Illumina default parameters. For amplicon assignation to amplicon sequence variant (ASV), several steps were followed based on Dada2 package version 1.5.0 developed by Callahan et al. (2016) and workflow (3) in R version 3.1.2 (http://www.R-project.org). The resulting ASVs were obtained with the following parameters. For the filtration step and quality check, sequences were trimmed at 255 pb for forward reads and 225 pb for reverse reads. The first 10 base pairs were truncated for all reads. Ambiguous bases and more than two expected errors were filtered out for all reads. After filtration, the average of reads count for library was 31,538 (min: 4020; max: 75,505) for 16S and 75,641 (min: 33,291; max: 112,897) for ITS. An average of reads filtered out was 27% +/− 4 for 16S and 57% +/− 14 for ITS mostly due to low length sequences. Then, the amplicon error learning steps for the forward and reverse reads were performed on 100M nucleotides using the DADA2 algorithm with default parameters. Finally, the denoised output reads were generated by dereplication using the derepFastq function from the DADA2 package and all reads with any mismatches were removed. Then forward and reverse reads were merged, and chimera sequences were removed with the uchime_denovo function from the vsearch package with a minimum difference set at 2. Purged ASV sequences with more than 4 read counts were kept. For 16S corresponding ASV, 3928 unique ASV sequences were found with 2100 (53.5%) considered as chimeras representing 7.6%) in 1175149 total sequences. For ITS, 463 unique ASV sequences were found with 103 (40.6%) considered as chimeras representing 1.4% in 1965903 total sequences.
ASVs sequences were then annotated by BLAST matching the NCBI database, excluding the Uncultured/environmental sample sequences. From the top 50 hits, the best score was kept. If several species had a similar score, the sequence was renamed as “Genus.sp”. To exclude potential mislabeling, if several Genus has a similar score, genus represented in more that 1/5 of the best scores were concatenated and the ASV renamed as Genus.species_1-Genus.species_2. Finally, ASVs associated to the same species names were aligned and sequences presenting less that 1% difference were grouped into Operational Taxonomic Units (OTUs). This last step was performed to reduce false positives and to decrease sparsity in the abundance matrix. The relative abundance of each OTU based on sequence counts was converted to absolute abundance estimates using the 16S and ITS total amplicon copy number evaluated by ddPCR in each library. Abundance is presented as the log10 (amplicon copy numbers +1), so that null values can remain informative.
We used the web platform microbiome analyst to compare alpha and beta diversity between samples (Dhariwal et al., 2017). Absolute abundances were used as the input and Shannon diversity index was calculated with unfiltered data. Principal coordinates analysis (PCoA) ordination was performed on OTUs with at least 10% occurrence, using the Bray-Curtis distance. Homogeneity of group dispersions (PERMDISP) and analysis of group similarities (ANOSIM) were performed to compare Sbb and organoleptic conformity groups.
To model the relationships in our high-dimensional dataset, a partial least square approach to model fitting was selected. This approach is particularly useful when there are more explanatory variables than observations or when the explanatory variables are highly correlated, which is the case with our OTU data. We applied a nonlinear iterative partial least squares analysis (JMP14, SAS institute) to OTUs as predictor of sap composition (ammonium, organic sulfur, sulfate and phosphate) and syrup L*a*b* values. Because of missing values, separate models were fitted for each variable and the number of factors was cross validated using the Root Mean PRESS (predicted residual sum of squares) approach. OTUs that were detected in a minimum of five samples were included in the analysis and ranked by Variable Importance for Projection (VIP) to identify the top predictors for each parameter tested.
Further, we used bootstrap forest partitioning (JMP14) to evaluate the contribution of OTUs as predictors of maple syrup conformity (standard or non-standard). The bootstrap forest models (100 decision trees each) were built on multiple predictors; therefore, the method can identify OTUs that might be weak predictors alone but strong when used in combination with other OTUs. The analysis was run ten times on OTUs occurring in at least five samples and the contribution of each OTUs was ranked to identify the consistently best predictors.
The estimated abundance adjusted by ddPCR was used to generate a networks for the Early and Late Sbb groups using the CoNet algorithm (Faust and Raes, 2016) and SparCC (Sparse Correlations for Compositional data) (Friedman and Alm, 2012). OTUs with less than three occurrences were not considered and the Spearman correlation threshold was set at 0.6. Associations observed with both methods were used to construct the final networks. The network characteristics and figures were generated with Cytoscape v3.8.2.
We used the web-based application Piphillin (Narayan et al., 2020) to predict the metagenomic content of maple sap bacterial communities. For greater precision, we used as input the ddPCR-adjusted abundances of each ASV and selected a conservative 99% identity cutoff for genome annotation with the Biocyc 24.0 database. The annotations of 686 (out of 1598) ASV were inferred from 181 genomes yielding 5988 Biocyc inferred features. Spearman’s non-parametric correlations of each feature abundance with the Sbb index and FDR adjusted Pvalues were computed with JMP14.
Allantoin and allantoate were quantified as described in Filteau et al. (2017). Briefly, the maple sap concentration of solid content measured with a digital refractometer was adjusted to 10 °Brix. Then samples were diluted 4 × in 90:10 (acetonitrile:water) containing allantoin-13C2,15N4 as an internal standard (1ppm), vortexed for 1 min and centrifuged at 16,000 g for 5 min at 4°C. Samples were then filtered through a 0.22 µm nylon filter prior to injection in a Acquity H-Class Ultra-Performance LC system coupled to a Xevo TQD mass spectrometer (Waters, Milford, MA, United States).
Ammonium and sulfate were quantified as described in N’guyen et al. (2018), by FIA with Quikchem 8500 series2 using the Quikchem method 10-107-06-2-B ammonia in surface water, wastewater, and the Quikchem method 10-116-10-1-C (tubidimetric method), respectively. Data acquisition was carried out with Omnion 3.0 from Lachat Instruments and quantification was performed based on external calibration.
A yeast strain from the prototrophic deletion collection (MATα hoΔ0::KanMX can1Δ:: STE2pr-SpHIS5 his3Δ1 lyp1Δ0) (VanderSluis et al., 2014), a Pseudomonas spp. strain (MJ020) isolated from sap (N’guyen et al., 2020) and the Leuconostoc mesenteroides strain ATCC 23386 were used in growth experiments. Each strain was individually precultured at room temperature overnight without agitation in allantoin media (Allantoin: 1.25 g/L, Yeast Nitrogen Base without amino acid and without nitrogen source (YNB): 1.75 g/L, Sucrose: 20 g/L).
To test the maximum growth capacity of microorganisms in the saps, we used filtered saps harvested in 2018 and 2019. The three strains were individually inoculated in filtered sap adjusted to 2 °Brix. Initial Optical Density (OD) was adjusted for each strain at 0.05 OD in 300 μl in a 96 well microplate and measured in triplicates, including non-innoculated negative controls for each sap. After 4 days incubation at room temperature, final OD was recorded using a Tecan Spark plate reader (Zürich, Switzerland). To assess which nutrient may be limiting, the same growth assays were performed in a subset of nine sap samples, which were spiked with either methionine (15uM), allantoate (1.25 g/L), sulfate (0.5 g/L) or sucrose (20 g/L). Corresponding non-supplemented sap were used as a control to compare each strain maximum growth.
Based on previous observations of maple sap biochemical variation using yeast functional mutants as biological reporter (N’guyen et al., 2018), we classified Sbb groups as Early (Sbb >35.5) or Late (Sbb ≤35.5). In these groups, the probability that the corresponding syrups were of acceptable quality (standard or light off-flavor) was significatively greater for the Early than the Late group (Fisher’s Exact Test, N = 32, Pvalue = 0.0004). We also characterized the syrup colors in the L*a*b* color space (N = 37). We found significant correlations between the L* (Spearman ρ = 0.68, Pvalue <0.0001) and a* (Spearman ρ = -0.55, Pvalue = 0.0004). These result shows that factors predictive of dormancy release in maple trees are also associated with syrup quality and color. Next, we characterized maple sap microbial communities to investigate how they vary along the Sbb index.
Microbial communities of 47 sap samples harvested in 2015, 2016 and 2017 were analyzed. We estimated bacterial and fungal contamination levels through 16S rDNA and ITS amplicon copy numbers determined by ddPCR, respectively. A significant correlation was observed between the Sbb index and overall microbial contamination levels (Figure 1) (Spearman ρ = -0.4243, Pvalue = 0.003). Although, bacterial and fungal contamination were correlated with each other (Spearman ρ = 0.48, Pvalue = 0.0005), only 16S rDNA copy numbers showed a significant correlation with the Sbb index (Spearman ρ = −0.43, Pvalue = 0.0026). Microbial composition and relative abundances were determined by amplicon sequencing and the total copy number measured by ddPCR was used to find estimates of absolute abundance of each community member (Supplementary Data S2). Analysis at the phylum level revealed that Proteobacteria were the most abundant, reaching 7.8 log amplicon copies/mL, but that the strongest association with the Sbb index was with Firmicutes, followed by Actinobacteria (Figure 2). For the 47 saps samples, we identified 550 bacterial and 161 fungal OTUs belonging to 77 and 64 genera, respectively (Figure 3, see Supplementary Figure S1 for an overview by sample). The most frequent and abundant bacteria were Pseudomonas, Rahnella, Janthinobacterium, Leuconostoc, Carnobacterium, Cryobacterium and Sanguibacter. Concurrently, Mrakia, Tausonia, Cadophora, Leucosporidium and Rhodosporidiobolus were the most frequently encountered fungal genera with over 70% prevalence and were also the most abundant. Molds such as Fusarium and Penicillium were also identified, but with a lower prevalence. In general, fungi ranked as high as bacteria in occurrence, but not in abundance.
FIGURE 1. Microbial contamination levels in sap along dormancy release. Sap contamination levels are reported as the sum of 16S rDNA and ITS amplicon copy numbers measured by ddPCR. The horinzontal axis shows decreasing Sbb values to reflect the temporal change from dormancy to bud burst. Shapes reflect maple syrup quality while colors reflect the a* component of the L*a*b* colorspace of maple syrups. The vertical dotted line separates the samples belonging to the Early and Late Sbb groups.
FIGURE 2. Variation of bacterial and fungal phylum abundance in maple sap with tree dormancy release. Spearman ρ and Pvalues (N = 47) are provided for each phylum. The vertical dotted line delineates the Early and Late Sbb groups.
FIGURE 3. Bacterial and fungal genus ranked by their occurrence and abundance in maple sap. (A) All 141 genus, colored by kingdom. Upper right square is magnified in (B). (B) Most frequent and abundant genus, colored by phylum.
Microbial community diversity varied with the Sbb index. The alpha diversity, as measured with the Shannon’s diversity was significatively correlated to the Sbb index (Spearman ρ = −0.3820, Pvalue = 0.0081), but was not different between organoleptic conformity groups (T-test Pvalue = 0.28, Figure 4A). To compare the beta diversity between Sbb and organoleptic conformity groups, we performed a PCoA ordination (Figure 4B) and multivariate statistical tests. We found that group dispersion was significatively different between Sbb groups (PERMDISP F-value: 8.7979, N = 47, Pvalue = 0.0048133), the Late group being more heterogenous. Because of this heterogeneity, we performed a non-parametric test to evaluate similarity between groups. We found that the similarity between Sbb groups is significatively lower than the similarity within the groups (ANOSIM R: 0.27239, N = 47, Pvalue <0.001). When comparing syrup organoleptic conformity, no difference in heterogeneity was detected (PERMDISP F-value: 1.0409, N = 32, Pvalue = 0.31577) and the similarity between groups was only marginally lower than similarity within groups (ANOSIM R: 0.10986, N = 32, Pvalue = 0.099). Altogether, these results indicate that microbial community profiles vary along factors influencing the tree dormancy release, but their relationship with maple syrup flavor defects may be more subtle than whole community profiles and binary groups or may require more statistical power to be supported.
FIGURE 4. The diversity of maple sap microbial community varies with the Sbb index. (A) Shannon’s diversity in relation to the Sbb index. The horizontal axis shows high to low Sbb values to logically depict the change from dormancy to bud burst. (B) Ordination plot (PCoA) of maple sap microbial communities. Shapes refer to syrup organoleptic conformity, while colors reflect the Sbb group.
Because microorganisms can alter their environment, in this case maple sap composition, the abundance of some species or combinations may be predictive of the maple syrup quality and color produced. To further investigate the relationship between OTU abundance and quantitative descriptors of sap composition, we performed a nonlinear iterative partial least squares analysis of OTUs as predictor (Figure 5). The models were generated for sap composition descriptors that are correlated with the Sbb index; ammonium, organic sulfur compounds, sulfate. For phosphate, which was not correlated to the Sbb index, the cross validation did not identify a number of factors minimizing the Root Mean PRESS. Restricting our focus on OTUs negatively correlated with the Sbb index, the strongest predictor OTUs belonged to the Proteobacteria, then Basidiomycota, Ascomycota, Firmicutes and Actinobacteria phyla. We performed the same analysis for L*a*b* values. The best predictors OTUs of maple syrup color descriptors belonged to Proteobacteria, then Basidiomycota, Ascomycota, and Firmicutes, but variable importance for projection were in general smaller than for sap composition descriptors. A few OTUs were found among the best predictors for both sap composition and syrup color parameters, namely Leuconostoc.sp_1, Pseudomonas.sp_19, Pseudomonas.sp_62 and, Pseudomonas.sp_65.
FIGURE 5. Nonlinear iterative partial least squares analysis of OTUs as predictor of sap composition and syrup color. For each factor, the top ten OTUs based on variable importance for projection (VIP) for OTU negatively correlated to the Sbb index are shown and boldface labels indicate that this correlation is significant (Spearman P FDR adjusted Pvalue <0.05). OTUs are colored by phylum.
To identify the top ten predictors of maple syrup organoleptic conformity, we used a bootstrap forest partitioning approach (Figure 6A). Eight of these OTUs were more abundant in the sap of non-standard syrups (Leuconostoc.sp_1, Pseudomonas.syringae_7, Curvibasidium.cygneicollum_2, Cadophora.malorum_2, Cystofilobasidium.capitatum_1, Pseudomonas.sp_48, Carnobacterium.sp_2 and Pseudomonas.sp_62) while two where more abundant in the sap of standard syrups (Pseudomonas.sp_27, Chryseobacterium.polytrichastri_1) (Figure 6B). The fact that OTUs belonging to the Pseudomonas genus are found in both cases highlights that effect may be species- or even strain-dependent.
FIGURE 6. OTUs identified as the best indicators of maple syrup organoleptic conformity. OTUs are colored by phylum. (A) Box plot of the contribution of the top ten OTUs predictor to maple syrup conformity partition models. Bold labels indicate that the OTU has a significant correlation to the Sbb index (Spearman P FDR adjusted Pvalue <0.05) and underlined labels indicate that the OTU is also present in Figure 5B) OTU average abundance in maple sap samples corresponding to standard vs. non-standard syrups. The top ten OTUs predictor of syrup organoleptic conformity identified in (A) are labeled.
Among the identified top predictor OTUs of maple sap composition, syrup color and quality (Figures 5, 6A), 16 out of 45 were also significatively negatively correlated to the Sbb index (Spearman correlation, FDR adjusted Pvalue <0.05, boldface in Figures 5, 6A). Thus, only part of the microbial contaminants associated with syrup quality appears to vary along factors influencing the tree dormancy release, leaving room for other factors influencing their abundance patterns, such as microbial interactions.
To better grasp how microorganisms associate and interact in the changing maple sap environment, we constructed microbial association networks of the Early and Late groups of samples (Figure 7A). The Early network was smaller, with 270 nodes and 4211 edges compared to 485 nodes and 6332 edges for the Late network. However, the density was higher in the Early network (0.116) than in the Late network (0.055). Conversely, network heterogeneity was 0.948 in the Late network, compared to 0.651 in the Early network. Most of the OTUs present in the Early network were also present in the Late network, but few edges were shared, indicating circumstantial or context-dependent associations (Figure 7B). Although the ratios of Bacteria:Fungi were similar between networks (3:1), inter- and intra-kingdom associations proportions were significatively different (likelihood ratio Pvalue = 2.8e-131) (Figure 7C). The Early network harbored a higher proportion of inter-kingdom correlations, while the Late network had more Bacteria-Bacteria associations.
FIGURE 7. Microbial associations and influent microbial community members differ between the Early and Late Sbb groups. (A) Microbial association networks constructed using Spearman correlations of OTU abundance. Node size and color reflects average abundance and phylum, respectively. Edge colors indicate positive (blue) and negative (orange) correlations. (B) Venn diagram showing the overlap of nodes and edges between networks. (C) Donut chart comparing the proportion of inter- and intra-kingdom association types in the Early and Late networks. Bacteria-Bacteria associations (blue) are the most common, while Fungi-Fungi (yellow) have the lowest proportion in both networks. Bacteria-Fungi associations (green) are more abundant in the Early than in the Late network.
The prevalence of negative association was higher in the Late network (149, 2.4%) compared to the Early network (18, 0.4%) implicating mostly bacteria. The few negative hubs in the Early network belonged to the Pseudomonas genus, while in the Late network, members of Caulobacter, Mesorhizobium, Phyllobacterium, Bradyrhizobium, Delftia and Sphingomonas had the most negative associations. In the Early network, the abundant OTUs ranked high in betweenness centrality, but not necessarily in degree (Figure 8). In contrast, in the Late network the most abundant OTUs tended to also be the most connected, with intermediate ranks of betweenness centrality. Interestingly, among the top predictors associated with non-standard syrups, Leuconostoc.sp_1 and Pseudomonas.syringae_7 occupied hub positions in the Late network, but not in the Early network. Conversely, Pseudomonas.sp-27, which is associated with standard samples, was highly connected in the Early, but not in the Late network. These results show that microorganisms, including OTUs predictor of syrup organoleptic conformity, associate with each other’s differently in the two Sbb groups.
FIGURE 8. OTUs ranked by degree and betweenness centrality in the (A) Early and (B) Late network. Circle color and size refer to phylum and average abundance, respectively. The top ten OTUs predictor of syrup organoleptic conformity are labeled.
To complement the microbial community composition and structure analysis and identify functional features associated with the Sbb index, we performed a predictive functional analysis of maple sap bacterial communities. The results revealed 469 features strongly negatively correlated to the Sbb index (Spearman ρ < −0.50, FDR adjusted Pvalue <0.01) (Supplementary Data S3). Positive correlations with the Sbb index were all comparatively weaker (Spearman ρ < −0.36, FDR >0.01) and are thus not reported. For ease of interpretation and visualization, annotated features were grouped into functional categories (Supplementary Data S3). The top functions belonging to degradation and biosynthesis categories are shown in Figure 9. The results show that features correlated to the Sbb index are mostly unidirectional, that is they involve either biosynthesis or degradation of various metabolites, but rarely both. The strongest correlation is for sucrose degradation, the main carbon source in maple sap. The various other carbohydrates also mostly figure in the degradation categories, except for levan and peptidoglycan. Interestingly, genomic features associated with the biosynthesis of sulfur-containing secondary compounds are negatively correlated to the Sbb index. Since the abundance of organic sulfur compounds has been previously measured in these samples (N’guyen et al., 2018) and shown to be negatively correlated with the Sbb index, the predictive functional association with their biosynthesis revealed in Figure 9 suggest that some bacteria contribute to their presence in maple sap. A few Pseudomonas.sp OTUs shown in Figure 5 are correlated to both the Sbb index and organic sulfur compounds and thus are likely candidates. As for amino acids, they are split between both categories; tryptophan, leucine, isoleucine, arginine and methionine degradation, vs. asparagine, lysin, alanine and citrulline biosynthesis. For other nitrogen sources, the results include significant correlations for features related to urate and allantoin degradation as well as ammonia assimilation but also peptidases.
FIGURE 9. Functional prediction analysis reveals bacterial gene abundance of specific biosynthesis and degradation pathways correlated to the Sbb index. The genomic features significatively correlated to the Sbb index were grouped by functional category and ordered by their average Spearman ρ value (color scale). Only the top 50 biosynthesis and degradation categories are shown (FDR adjusted Pvalue ≤0.002). Results for all categories are available in Supplementary Data S3.
The increase in microbial contamination levels along the Sbb index could be explained by increasing temperatures which allow for faster growth, or by the variation in sap composition along the Sbb index. To distinguish between these two factors, we performed growth experiments with representative microorganisms (a Pseudomonas, Leuconostoc and a yeast strain) in 42 sterilized sap samples from five production sites collected in 2018 and 2019 to determine their carrying capacity at controlled temperature. The optical density measurement for the yeast (Spearman ρ = −0.55, Pvalue = 0.0002) and Pseudomonas (Spearman ρ = −0.50, Pvalue = 0.0007) strain indeed significatively increased at lower Sbb values, but not for Leuconostoc (Spearman ρ = −0.13, Pvalue = 0.40) (Figure 10A). These results demonstrate that as the tree undergoes dormancy release, the sap collected can support more growth for two of the three microorganisms tested.
FIGURE 10. Maple sap carrying capacity and nitrogen content increases rapidly in the Late Sbb group. (A) Growth of representative microorganisms in filtered maple sap measured by optical density after 4 days relative to the Sbb index. (B) Quantification of allantoate, allantoin and ammonium in filtered maple sap relative to the Sbb index. Values are reported as uM normalized to 2 °Brix sap.
In light of the current results and previous work showing that allantoate was an important nutrient for yeast in maple sap and that its quantity increased over the harvest period at the one production site tested (Filteau et al., 2017), we quantified allantoate, allantoin and ammonium in the 2018–2019 samples. We observed significant negative correlations between the Sbb index and allantoate (Spearman ρ = −0.56, Pvalue <0.0001) and ammonium (Spearman ρ = −0.54, Pvalue = 0.0002) (Figure 10B). Their concentration was relatively low and stable in the Early Sbb group and increased rapidly in the Late group. Comparatively, allantoin concentration was low and positively correlated to the Sbb index (Spearman ρ = 0.50, Pvalue = 0.0008), but this correlation was driven mostly by samples from only one production site. Therefore, the change in nitrogen content occurring after Sbb = 35.5 could explain the increased carrying capacity of maple sap.
To further understand which nutrient may influence the growth of Pseudomonas, Leuconostoc and yeast, we performed experiments with a subset of saps from three production sites supplemented with either sucrose, sulfate, methionine or allantoate (Figure 11). The growth of the Pseudomonas strain consistently increased when adding a nitrogen source (allantoate or methionine), except in the latest sample. Meanwhile, the growth of Leuconostoc was almost always increased by methionine supplementation, but not allantoate or sulfate, indicating a requirement that is more complex than sulfur or nitrogen limitation. Interestingly, the addition of sulfate to saps in the Early Sbb group, partly inhibited Pseudomonas growth. As for the yeast strain, results varied between samples, but sulfur appeared as a limiting nutrient in some cases. Altogether, these results demonstrate that Pseudomonas can degrade and use allantoate as a nitrogen source while Leuconostoc can benefit from an organic sulfur source, i.e., methionine, two metabolites that become more abundant when Sbb < 35.5. These observations thus contribute to a mechanistic understanding of the associations between the Sbb index, maple sap microbial contamination and maple syrup quality.
FIGURE 11. Limiting nutrients in maple sap vary by microorganism. Growth of a Leuconostoc, a Pseudomonas and a yeast strain in filtered maple sap samples supplemented with either sucrose, sulfate, methionine or allantoate is represented in the heatmap. Cell colors reflect the mean OD values expressed as % of control (N = 3). Only significant differences are shown (Dunnet’s test, Pvalues <0.05). The horizontal axis reports the Sbb index values and groups.
Around 200 million pounds of maple syrup is produced each year of which over 85% is sold in bulk and each barrel produced goes through a standardized classification system to determine its commercial value (AAC, 2021; MAPAQ, 2021; PPAQ, 2021). Maple syrup properties are known to vary, particularly along the flow period (Filteau et al., 2012; Lagacé et al., 2015; Nimalaratne et al., 2020). Recently, a marked transition in commercial quality and maple sap composition was described along dormancy release (N’guyen et al., 2018; Garcia et al., 2020). We also observed a relationship between the level of red color (positive a* values in CIELAB color space) and the Sbb index. Thus, we sought to investigate the maple sap microbiota associated with these changes. We used a next generation sequencing approach coupled to ddPCR to estimate the absolute abundance of maple sap contaminants. Although this approach does not distinguish between viable and dead cells, the quantitative results show a general increase of total contaminant along dormancy release coherent with the increase in microbial counts reported in previous studies as the flow period progresses (Lagacé et al., 2004; Filteau et al., 2010; Lagacé et al., 2015). However, only some phyla appear to increase during both Early and Late dormancy release, namely Firmicutes, Actinobacteria, and Proteobacteria. Trends for Bacteroidetes, Ascomycota, and Basidiomycota suggest that members of these phylum do not increase during the Late dormancy release period. Thus, the general shift in maple sap properties around Sbb = 30–40 observed previously (N’guyen et al., 2018) is mirrored by changes in microbial contamination.
Our results are in good agreement with previous reports of maple sap predominant contaminants, both bacterial and fungal (Lagacé et al., 2004; Lagacé et al., 2006; Filteau et al., 2010; Filteau et al., 2011). However, Carnobacterium was found to be more predominant compared to previous studies (Filteau et al., 2010; Filteau et al., 2011), possibly because of the emphasis on late season samples. A noteworthy discrepancy is that we did not identify Mrakiella among our fungal OTUs, which is likely owed to the reference database used for OTU annotations and the relatedness between Mrakia and Mrakiella. Aside from this difference, in previous work some contaminants could not be identified, due to some limitations of the method (Filteau et al., 2011). Here our use of next generation sequencing allowed to identify multiple prevalent fungal genera not previously reported in maple sap, including Rhodosporidiobolus, Cyberlindnera, Curvibasidium, Cystofilobasidium, Itersonilia, Phenoliferia, Phaffia and Vishniacozyma. On top of this yeast diversity, molds were detected including some belonging to the Penicillium and Fusarium genus. Molds have previously been isolated from tapholes (Sheneman and Costilow, 1959), and they can sometimes develop in collection tubes, highlighting possible sanitations issues that should be given further attention.
In previous studies, the highest microbial diversity was observed at 50% (Lagacé et al., 2004) and 100% (Filteau et al., 2010) flow periods in sap, and a diminishing trend was reported for tubing biofilms in main lines (Lagacé et al., 2006). Here, when considering both bacteria and fungi, diversity in maple sap is negatively correlated with the Sbb index and samples are more heterogenous in the Late group. This result indicates that contamination occurred throughout the collection period and/or that the changes in environmental conditions such as changes in amino acids and inorganic content (Lagacé et al., 2015; N’guyen et al., 2018; Garcia et al., 2020) enabled the growth of a higher diversity of microorganisms towards bud burst. However, diversity did not vary significatively between organoleptic conformity groups, perhaps because of a lack of statistical power, or because maple syrup quality is not related to broad microbial community patterns, but to specific members.
We then screened for the top OTUs predictors of sap composition (ammonium, organic sulfur, and sulfate), maple syrup color descriptors (L*a*b* values) and syrup organoleptic conformity. The top predictors of ammonia in maple sap mostly belonged to Proteobacteria and the strongest OTUs were assigned to Pseudomonas and Rahnella, two dominant bacterial genera in maple sap that include members capable of fixing molecular nitrogen and producing ammonia (Pratiwi et al., 2020; Singh et al., 2022). Multiple OTUs belonging to predominant genera in maple sap (Pseudomonas, Rahnella, Leuconostoc) were also associated with the presence of organic sulfur. The strongest predictors of organic sulfur were also associated with the presence of sulfate suggesting metabolic activity. Also, two predictors associated with sulfate, Leuconostoc.sp_1 and Carnobacterium.sp_2 were also identified among the top predictors of syrup organoleptic conformity. Taken together, these results support the hypothesis that microbial activity plays a role in the appearance of defects, such as buddy off-flavors, towards bud burst (N’guyen et al., 2018).
Microorganisms occur in communities and can influence each other’s activity directly using targeted mechanisms or indirectly by modify their environments (Tyc et al., 2014; Grandclement et al., 2016; Ratzke and Gore, 2018; Gu et al., 2020; Gupta et al., 2021). In some cases, microbial interactions may be as important as the presence of an individual species for community function (Gould et al., 2018) and microorganisms can depend on metabolite production by other community members for survival (Ponomarova et al., 2017; Ratzke et al., 2020). To grasp how microorganism associate in Early and Late groups, we constructed two microbial association networks and compared their general properties. In these types of networks, the observed associations mostly reflect parallel variations along an environmental gradient but can sometimes also capture ecological interactions (Faust et al., 2015; Faust and Raes, 2016). Most associations were positive, but a larger proportion of negative associations was observed in the Late network where nutrients are more abundant. This result aligns with the recently proposed hunger games hypothesis stating that cooperation prevails in bacterial communities, but that competition is more frequent in nutrient-rich (copiotrophic) conditions (Dai et al., 2022). This hypothesis also predicts a greater number of bacterial interactions in copiotrophic conditions than in oligotrophic conditions which contrast with the higher density observed in the Early network where nutrients are more limiting. However, their hypothesis was constructed based on bacterial data and the same principles may not fully apply to multi-kingdom microbial communities. Also, the results indicate that interkingdom associations could play a more important role in the Early group, which is supported by the observation that fungal phylum do not appear to increase in the Late group, whereas associations between bacteria form the vast majority in the Late group. Interestingly, some predictors of maple syrup organoleptic conformity were identified as hubs in the Early or Late network indicating that not only their abundance, but also their associations with other microorganisms may be relevant for maple syrup quality. Considering, the modest number of samples in our datasets, further studies are needed to validate these conclusions.
To better understand how microorganisms may impact maple sap composition, we identified predicted function of bacterial communities associated with the Sbb index. As expected, functions associated with sucrose and allantoate degradation were strongly negatively correlated to the Sbb index, meaning that gene content in these pathways increased in late spring, indicating an increased genetic ability to use these main sources of carbon and nitrogen, respectively. Also, degradation of tryptophan, methionine, leucine, isoleucine, arginine, and choline is associated with dormancy release, in agreement with the previously reported increase of these metabolites in maple sap at low Sbb (N’guyen et al., 2018; Garcia et al., 2020). Moreover, an interesting result is the increase of the degradation pathways of galacturonate and xylose towards late spring as these two compounds are constituents of the hemi-cellulose of Acer saccharum (Timell, 1959). Components of degradation pathways of fatty acids and lipids also increased. In angiosperms, xylem cell walls contain cellulose, hemicelluloses, lignin, proteins, glycoproteins, and lipids, including phospholipids (Schenk et al., 2018). Therefore, our results suggest an increase in bacteria that can degrade subunits of xylem cell wall components in late spring. Such components could be transported as building blocks in the sap by the tree. Xylose for example has been reported to peak in maple sugar wood tissue during spring (Wong et al., 2003). Alternatively, xylem cell wall components could be released by the action of phytopathogens on tree structures. For instance, proteins can be present in xylem sap (Schenk et al., 2018) and the various predicted peptidases increasing with dormancy release could in part explain the highly variable amino acids composition reported in maple sap (Dumont, 1994; N’guyen et al., 2018; Garcia et al., 2020). Also, the yeast Tausonia (Guehomyces) pullulans has been reported to produce cold-active xylanase (Tasselli et al., 2017), an enzyme that breaks down hemi-cellulose. The fact that we find notorious plant pathogens, namely Pseudomonas syringae and Cadophora malorum among maple syrup quality predictors further supports this hypothesis. Moreover, a vast majority of Pseudomonas syringae strains isolated from maple trees were pathogenic in maple seedlings (Malvick, 1988) underlining that their detection in maple sap deserves further attention.
In contrast, for lysine, asparagine, and sulfur-containing secondary compounds, it is the biosynthetic pathways that increases in late spring, suggesting that bacteria may contribute to the presence of these compounds in maple sap. Therefore, we hypothesize that microorganisms are at least partly responsible, directly, or indirectly, for the increase in amino acids and organic sulfur compounds observed during late spring. However, there is still much to decipher about the maple sap microbiota and its metabolism since the functional prediction analysis was able to predict the metagenome content of only 43% of the bacterial members. Another limitation of the functional prediction analysis is that the fungal contribution cannot be estimated at this point because the currently available tools provide only for the prediction of bacterial metagenome content. Moreover, further studies to disentangle the maple tree contribution to maple sap composition and its relevance for maple syrup quality are needed since few studies were performed on aseptically harvested sap (Whalen and Morselli, 1986; Morselli and Whalen, 1991).
Taken together, the predicted functional properties revealed that microbial metabolism is intertwined with specific maple sap metabolites which may influence the resulting syrup properties including color, flavor, viscosity, and nutritional functionality. For instance, lysine and xylose are highly reactive compounds in Maillard reactions (Kwak and Lim, 2004) and their product possess antioxidative properties (Yen and Hsieh, 1995). Maple syrup is well known for its antioxidant potential, especially in darker colored syrups (Singh et al., 2014; FPAQ, 2018). Galacturonic acid is also involved in non-enzymatic browning reactions, and its thermal degradation products produce a typical red-brown color (positive a* values in CIELAB color space) (Bornik and Kroh, 2013) which we also find to be negatively correlated to the Sbb index. One of the main thermal degradation products of Galacturonic acid is 4-hydroxy-5-methylfuran-3-one (norfuraneol), of which the flavor descriptors include: sweet, caramellic, cotton candy, maple, burnt sugar and roasted coffee (http://www.thegoodscentscompany.com/). Also, bacterial fermentation of sap in particular by Leuconostoc mesenteroides has been shown to influence maple syrup viscosity (Lagacé et al., 2018). Here we find an association between Leuconostoc sp. in maple sap and maple syrup quality. Moreover, we find a functional prediction for levan biosynthesis increasing towards bud break. Many bacteria can synthesize levan from sucrose including Leuconostoc mesenteroides (Öner et al., 2016), however the feature (3.2.1.64-RXN) in our dataset is specific to some Actinobacteria (according to KEGG annotations of EC 3.2.1.64). Another instance where microbial metabolism may influence maple syrup properties is that maple syrup is a nutritional source of thiamine and riboflavin (FPAQ, 2018), and the bacterial metabolic capacity related to these compounds is predicted to increase with dormancy release. Also, the pathways related to thiamine, methionine, sulfoquinovose and other sulfur-containing secondary metabolites revealed in the functional prediction associations are of particular interest since organic sulfur compounds in sap and dimethyldisulfide in syrup have been associated with buddy off-flavors in maple syrup (N’guyen et al., 2018; Camara et al., 2019). Thus, further investigations into the role of specific microorganisms and their metabolism in maple sap could help improve our understanding of the mechanisms underlying some characteristics of maple syrup.
In previous work, we observed that maple sap composition undergoes important biochemical changes around Sbb = 30–40 (N’guyen et al., 2018). We hypothesized that the increase of allantoate in sap marks this turning point in dormancy release and impacts microbial communities. Thus, we quantified allantoin, allantoate and ammonium in an independent set of maple sap samples. Our results show that indeed an increase in allantoate occurs after this point. The ureide allantoate is a nitrogen storage molecule, which is accumulated in the roots of some deciduous trees during winter (Conn et al., 1990). However, maple sap exudate comes from reserves stored aboveground (Driller et al., 2020). Thus, the sharp increase of allantoate in maple sap could indicate that the exudate collected has shifted from aboveground to underground reserves. This distinction may have empirically been made by producers in Québec, as they refer to sap exudate as “maple water” and associate the term “sap” to late harvest and a distinct syrup flavor. Altogether, the correlated increase in allantoate, ammonium concentration and microbial growth may reflect a mechanism with critical consequences for maple syrup characteristics development. Indeed, allantoate, its catabolic intermediate urea, and ammonium can all influence the outcome of Maillard reactions. The presence of urea and ammonium may favor the formation of pyrazine, including 2,5-dimethylpyrazine (Chen et al., 2000), which has been suggested as responsible for a certain type of off-flavor in maple syrup (van den Berg et al., 2009).
Finally, seasonal temperatures could directly impact microbial communities by favoring some microorganisms while prohibiting the growth of others. For instance, most Mrakia species cannot grow at temperatures above 20°C (Thomas-Hall et al., 2010). As our Sbb index is ultimately based on temperatures, the microbial composition variation observed reflects both this seasonal temperature effect and the sap composition changes resulting from the metabolic modifications occurring in response to dormancy release in trees. To disentangle these effects on relevant microorganisms, we performed growth experiments in sterilized sap at constant temperature. Pseudomonas and yeast growth correlated with the Sbb index indicating that variation in maple sap composition during dormancy release can influence microbial contamination levels, independently of the impact of meteorological conditions on microbial growth. Moreover, spiked sap growth experiments indicate that nitrogen is a limiting nutrient for Pseudomonas and that the increase of allantoate will favor their growth. Similarly, methionine supplementation increased the growth of the Leuconostoc strain in maple sap. Lactic acid bacteria are often auxotrophic and methionine is one of the most common requirement, but auxotrophies can be species and strain-dependent (Canon et al., 2020). Based on our results, we can surmise that the identification of Leuconostoc sp._1 as the top predictor of maple syrup quality is because of the presence of methionine and/or a related sulfur compound which favors its growth, while acting as a flavor defect precursor.
Maple syrup is a unique sugaring product with distinctive sensorial and functional properties rooted not only in its vegetal origin, but also in its unique production process in which microorganism play an integral part. In this study, we investigated the relationships between maple sap microbial communities and a dormancy release index which shed a new light to the multiple ways microorganisms could contribute to maple syrup properties. Altogether our results suggest that the increase in nutrient supply and metabolite excretion lead microbial communities towards different paths (Dai et al., 2022), explaining the greater heterogeneity observed during late dormancy release, and in turn the variation in maple syrup quality. Our results highlight that further experimental investigation is needed to disentangle the contribution of microbial, vegetal, environmental, and technological factors, and their interaction with the heating process to the final characteristics of maple syrup.
The datasets presented in this study can be found in online repositories. The names of the repository/repositories and accession number(s) can be found in the article/Supplementary Material. Raw sequencing data are available at Bioproject number PRJNA808740 at http://www.ncbi.nlm.nih.gov/bioproject/.
GN, CR, LL, and MF designed the experiments and contributed to sample collection. GN performed the experiments. GN and MF performed the data analysis and wrote the manuscript. MF prepared the figures. GN, CR, LL, and MF revised the manuscript.
This work was funded by a Mitacs accelerate grant to MF in partnership with the Centre ACER Inc. (IT10507), a NSERC Engage grant to MF and Fruit d’Or (536599-18) and a NSERC Discovery grant to MF (RGPIN-2017-04771).
Author CR was employed by Fruit d'Or.
The remaining authors declare that the research was conducted in the absence of any commercial or financial relationships that could be construed as a potential conflict of interest.
All claims expressed in this article are solely those of the authors and do not necessarily represent those of their affiliated organizations, or those of the publisher, the editors and the reviewers. Any product that may be evaluated in this article, or claim that may be made by its manufacturer, is not guaranteed or endorsed by the publisher.
We are grateful to the maple syrup producers for providing samples and to Raymond Nadeau and Joël Boutin for logistical help and discussions. We are grateful to Nathalie Martin for providing comments on the manuscript.
The Supplementary Material for this article can be found online at: https://www.frontiersin.org/articles/10.3389/fsysb.2022.893007/full#supplementary-material
Supplementary Figure S1 | Heatmap of bacterial and fungal abundance by genus in each maple sap sample, ordered by decreasing value of Sbb index. The color gradient reflects the 16S or ITS amplicon copy number per mL estimated from ddPCR and sequencing data. Taxa occurring in at least 10% of samples and representing at least 1% relative abundance in any sample are shown. Sample labels are colored by Sbb group and flavor conformity is indicated by symbols.
Supplementary Data S1 | Description of all samples used in this study and their characteristics.
Supplementary Data S2 | Matrix of the absolute abundance estimate of each OTU per sample.
Supplementary Data S3 | Predicted functional features negatively correlated to the Sbb index and corresponding functional categories.
AAC (2021). Statistical Overview of the Canadian Maple Industry 2020. Agriculture and Agri-Food Canada. Available at: https://agriculture.canada.ca/en/canadas-agriculture-sectors/horticulture/horticulture-sector-reports.
Alli, I., Bourque, J., Metussin, R., Liang, R., and Yaylayan, V. (1990). Identification of Pyrazines in maple Syrup. J. Agric. Food Chem. 38 (5), 1242–1244. doi:10.1021/jf00095a019
Bleuven, C., Nguyen, G. Q., Després, P. C., Filteau, M., and Landry, C. R. (2020). Competition Experiments in a Soil Microcosm Reveal the Impact of Genetic and Biotic Factors on Natural Yeast Populations. ISME J. 14 (6), 1410–1421. doi:10.1038/s41396-020-0612-8
Bornik, M.-A., and Kroh, L. W. (2013). D-Galacturonic Acid as a Highly Reactive Compound in Nonenzymatic Browning. 1. Formation of Browning Active Degradation Products. J. Agric. Food Chem. 61 (14), 3494–3500. doi:10.1021/jf303855s
Callahan, B. J., McMurdie, P. J., Rosen, M. J., Han, A. W., Johnson, A. J. A., and Holmes, S. P. (2016). DADA2: High-Resolution Sample Inference from Illumina Amplicon Data. Nat. Methods 13 (7), 581–583. doi:10.1038/nmeth.3869
Camara, M., Cournoyer, M., Sadiki, M., and Martin, N. (2019). Characterization and Removal of Buddy Off‐Flavor in Maple Syrup. J. Food Sci. 84 (6), 1538–1546. doi:10.1111/1750-3841.14618
Canon, F., Nidelet, T., Guédon, E., Thierry, A., and Gagnaire, V. (2020). Understanding the Mechanisms of Positive Microbial Interactions that Benefit Lactic Acid Bacteria Co-Cultures. Front. Microbiol. 11, 2088. doi:10.3389/fmicb.2020.02088
Chen, Y., Xing, J., Chin, C.-K., and Ho, C.-T. (2000). Effect of Urea on Volatile Generation from Maillard Reaction of Cysteine and Ribose. J. Agric. Food Chem. 48 (8), 3512–3516. doi:10.1021/jf991076l
Conn, M. P., Stumpf, W., Miflin, B. J., and Lea, P. J. (1990). The Biochemistry of Plants: A Comprenhensive Treatise Intermediary Nitrogen Metabolism. San Diego, CA: Academic Press 16.
Cooke, J. E. K., Eriksson, M. E., and Junttila, O. (2012). The Dynamic Nature of Bud Dormancy in Trees: Environmental Control and Molecular Mechanisms. Plant Cel Environ 35 (10), 1707–1728. doi:10.1111/j.1365-3040.2012.02552.x
Dai, T., Wen, D., Bates, C. T., Wu, L., Guo, X., Liu, S., et al. (2022). Nutrient Supply Controls the Linkage between Species Abundance and Ecological Interactions in marine Bacterial Communities. Nat. Commun. 13 (1), 175. doi:10.1038/s41467-021-27857-6
Dhariwal, A., Chong, J., Habib, S., King, I. L., Agellon, L. B., and Xia, J. (2017). MicrobiomeAnalyst: A Web-Based Tool for Comprehensive Statistical, Visual and Meta-Analysis of Microbiome Data. Nucleic Acids Res. 45 (W1), W180–W188. doi:10.1093/nar/gkx295
Driller, T., Holland, D. J., and Watson, M. J. (2020). A Detailed Look at the Mechanisms Involved in Maple Sap Exudation. Acta Hortic. 1300, 121–130. doi:10.17660/actahortic.2020.1300.16
Dumont, J. (1994). Caractéristique chimiques et nutritives du sirop d'érable. Les acides aminés de la sève et du sirop d'érable. St-Hyacinthe, QC: Centre Acer Inc.
Faust, K., Lima-Mendez, G., Lerat, J.-S., Sathirapongsasuti, J. F., Knight, R., Huttenhower, C., et al. (2015). Cross-Biome Comparison of Microbial Association Networks. Front. Microbiol. 6, 1200. doi:10.3389/fmicb.2015.01200
Faust, K., and Raes, J. (2016). CoNet App: Inference of Biological Association Networks Using Cytoscape. F1000Res 5, 1519. doi:10.12688/f1000research.9050.2
Filteau, M., Charron, G., and Landry, C. R. (2017). Identification of the Fitness Determinants of Budding Yeast on a Natural Substrate. ISME J. 11 (4), 959–971. doi:10.1038/ismej.2016.170
Filteau, M., Lagacé, L., LaPointe, G., and Roy, D. (2011). Correlation of maple Sap Composition with Bacterial and Fungal Communities Determined by Multiplex Automated Ribosomal Intergenic Spacer Analysis (MARISA). Food Microbiol. 28 (5), 980–989. doi:10.1016/j.fm.2011.01.008
Filteau, M., Lagacé, L., LaPointe, G., and Roy, D. (2012). Maple Sap Predominant Microbial Contaminants Are Correlated with the Physicochemical and Sensorial Properties of maple Syrup. Int. J. Food Microbiol. 154 (1-2), 30–36. doi:10.1016/j.ijfoodmicro.2011.12.007
Filteau, M., Lagacé, L., LaPointe, G., and Roy, D. (2010). Seasonal and Regional Diversity of maple Sap Microbiota Revealed Using Community PCR Fingerprinting and 16S rRNA Gene Clone Libraries. Syst. Appl. Microbiol. 33 (3), 165–173. doi:10.1016/j.syapm.2010.02.003
FPAQ (2018). Quebec maple Syrup Industry Sheet. Longueuil, QC: Federation of Quebec Maple Syrup Producers.
Friedman, J., and Alm, E. J. (2012). Inferring Correlation Networks from Genomic Survey Data. Plos Comput. Biol. 8 (9), e1002687. doi:10.1371/journal.pcbi.1002687
Garcia, E. J., McDowell, T., Ketola, C., Jennings, M., Miller, J. D., and Renaud, J. B. (2020). Metabolomics Reveals Chemical Changes in Acer Saccharum Sap Over a Maple Syrup Production Season. Plos One 15 (8), e0235787. doi:10.1371/journal.pone.0235787
Gould, A. L., Zhang, V., Lamberti, L., Jones, E. W., Obadia, B., Korasidis, N., et al. (2018). Microbiome Interactions Shape Host Fitness. Proc. Natl. Acad. Sci. U.S.A. 115 (51), E11951–E11960. doi:10.1073/pnas.1809349115
Grandclément, C., Tannières, M., Moréra, S., Dessaux, Y., and Faure, D. (2016). Quorum Quenching: Role in Nature and Applied Developments. Fems Microbiol. Rev. 40 (1), 86–116. doi:10.1093/femsre/fuv038
Gu, S., Wei, Z., Shao, Z., Friman, V.-P., Cao, K., Yang, T., et al. (2020). Competition for Iron Drives Phytopathogen Control by Natural Rhizosphere Microbiomes. Nat. Microbiol. 5 (8), 1002–1010. doi:10.1038/s41564-020-0719-8
Gupta, G., Ndiaye, A., and Filteau, M. (2021). Leveraging Experimental Strategies to Capture Different Dimensions of Microbial Interactions. Front. Microbiol. 12, 700752. doi:10.3389/fmicb.2021.700752
Klindworth, A., Pruesse, E., Schweer, T., Peplies, J., Quast, C., Horn, M., et al. (2013). Evaluation of General 16S Ribosomal RNA Gene PCR Primers for Classical and Next-Generation Sequencing-Based Diversity Studies. Nucleic Acids Res. 41 (1), e1. doi:10.1093/nar/gks808
Kwak, E.-J., and Lim, S.-I. (2004). The Effect of Sugar, Amino Acid, Metal Ion, and NaCl on Model Maillard Reaction under pH Control. Amino Acids 27 (1), 85–90. doi:10.1007/s00726-004-0067-7
Lagacé, L., Camara, M., Leclerc, S., Charron, C., and Sadiki, M. (2018). Chemical and Microbial Characterization of Ropy maple Sap and Syrup. Maple Syrup Digest 57 (4), 9–19.
Lagacé, L., Girouard, C., Dumont, J., Fortin, J., and Roy, D. (2002). Rapid Prediction of Maple Syrup Grade and Sensory Quality by Estimation of Microbial Quality of Maple Sap Using ATP Bioluminescence. J. Food Sci. 67 (5), 1851–1854. doi:10.1111/j.1365-2621.2002.tb08734.x
Lagacé, L., Jacques, M., Mafu, A., and Roy, D. (2006). Compositions of Maple Sap Microflora and Collection System Biofilms Evaluated by Scanning Electron Microscopy and Denaturing Gradient Gel Electrophoresis. Int. J. Food Microbiol. 109 (1-2), 9–18. doi:10.1016/j.ijfoodmicro.2006.01.004
Lagacé, L., Leclerc, S., Charron, C., and Sadiki, M. (2015). Biochemical Composition of Maple Sap and Relationships Among Constituents. J. Food Compost. Anal. 41, 129–136. doi:10.1016/j.jfca.2014.12.030
Lagacé, L., Pitre, M., Jacques, M., and Roy, D. (2004). Identification of the Bacterial Community of Maple Sap by Using Amplified Ribosomal DNA (rDNA) Restriction Analysis and rDNA Sequencing. Appl. Environ. Microbiol. 70 (4), 2052–2060. doi:10.1128/aem.70.4.2052-2060.2004
Malvick, D. K. (1988). Population Dynamics and Diversity of Pseudomonas S on Maple and Pear Trees and Associated Grasses. Phytopathology 78 (10), 1366. doi:10.1094/phyto-78-1366
MAPAQ (2021). Portrait diagnostic sectoriel de l'industrie acéricole du Québec. MAPAQ, Gouvernement du Québec.
Millard, P., Wendler, R., Hepburn, A., and Smith, A. (1998). Variations in the Amino Acid Composition of Xylem Sap of Betula Pendula Roth. Trees Due to Remobilization of Stored N in the spring. Plant Cel Environ 21 (7), 715–722. doi:10.1046/j.1365-3040.1998.00313.x
Morselli, M. F., and Whalen, M. L. (1986). Amino Acids Increase in Xylem Sap of Acer saccharum Prior to Bud Break. Am. J. Bot. 73 (5), 722–723.
Morselli, M. F., and Whalen, M. L. (1991). Aseptic Tapping of Sugar maple (Acersaccharum) Results in Light Color Grade Syrup. Can. J. For. Res. 21 (7), 999–1005. doi:10.1139/x91-137
Naghski, J., Reed, L. L., and Willits, C. O. (1957). Maple Sirup. X. Effect of Controlled Fermentation of Maple Sap on the Color and Flavor of Maple Sirup. J. Food Sci. 22 (2), 176–181. doi:10.1111/j.1365-2621.1957.tb16998.x
Narayan, N. R., Weinmaier, T., Laserna-Mendieta, E. J., Claesson, M. J., Shanahan, F., Dabbagh, K., et al. (2020). Piphillin Predicts Metagenomic Composition and Dynamics from DADA2-Corrected 16S rDNA Sequences. Bmc Genomics 21 (1), 56. doi:10.1186/s12864-019-6427-1
N’guyen, G. Q., Jain, M., Landry, C. R., and Filteau, M. (2020). Mapping Gene-Microbe Interactions: Insights from Functional Genomics Co-Culture Experiments between Saccharomyces Cerevisiae and Pseudomonas Spp. Biorxiv [Preprint]. doi:10.1101/2020.06.01.127472
N’guyen, G. Q., Martin, N., Jain, M., Lagacé, L., Landry, C. R., and Filteau, M. (2018). A Systems Biology Approach to Explore the Impact of maple Tree Dormancy Release on Sap Variation and maple Syrup Quality. Sci. Rep. 8 (1), 14658. doi:10.1038/s41598-018-32940-y
Nimalaratne, C., Blackburn, J., and Lada, R. R. (2020). A Comparative Physicochemical Analysis of maple (Acer Saccharum Marsh.) Syrup Produced in North America with Special Emphasis on Seasonal Changes in Nova Scotia maple Syrup Composition. J. Food Compost. Anal. 92, 103573. doi:10.1016/j.jfca.2020.103573
Nursten, H. (2007). The Maillard Reaction : Chemistry, Biochemistry and Implications. Cambridge, United Kingdom: Royal Society of Chemistry. doi:10.1039/9781847552570-fp001
Öner, E. T., Hernández, L., and Combie, J. (2016). Review of Levan Polysaccharide: From a Century of Past Experiences to Future Prospects. Biotechnol. Adv. 34 (5), 827–844. doi:10.1016/j.biotechadv.2016.05.002
Ponomarova, O., Gabrielli, N., Sévin, D. C., Mülleder, M., Zirngibl, K., Bulyha, K., et al. (2017). Yeast Creates a Niche for Symbiotic Lactic Acid Bacteria through Nitrogen Overflow. Cel Syst. 5 (4), 345–357. doi:10.1016/j.cels.2017.09.002
PPAQ (2021). Quality Control of Our Bulk Maple Syrup. Longueuil, QC: Producteurs et productrices acéricoles du Québec.
Pratiwi, E. R., Ardyati, T., Ardyati, T., and Suharjono, S. (2020). Plant Growth Promoting Endophytic Bacteria of Coffea Canephora and Coffea Arabica L. In UB Forest. J. Exp. Life Sci. 10 (2), 119–126. doi:10.21776/ub.jels.2020.010.02.07
Ratzke, C., Barrere, J., and Gore, J. (2020). Strength of Species Interactions Determines Biodiversity and Stability in Microbial Communities. Nat. Ecol. Evol. 4 (3), 376–383. doi:10.1038/s41559-020-1099-4
Ratzke, C., and Gore, J. (2018). Modifying and Reacting to the Environmental pH Can Drive Bacterial Interactions. Plos Biol. 16 (3), e2004248. doi:10.1371/journal.pbio.2004248
Raulier, F., and Bernier, P. Y. (2000). Predicting the Date of Leaf Emergence for Sugar Maple across its Native Range. Can. J. For. Res. 30, 1429–1435. doi:10.1139/x00-064
Schenk, H. J., Espino, S., Rich‐Cavazos, S. M., and Jansen, S. (2018). From the Sap's Perspective: The Nature of Vessel Surfaces in Angiosperm Xylem. Am. J. Bot. 105 (2), 172–185. doi:10.1002/ajb2.1034
Sheneman, J. M., and Costilow, R. N. (1959). Identification of Microorganisms from Maple Tree TAPHOLESb. J. Food Sci. 24 (1), 146–151. doi:10.1111/j.1365-2621.1959.tb17643.x
Singh, A. S., Jones, A. M. P., and Saxena, P. K. (2014). Variation and Correlation of Properties in Different Grades of maple Syrup. Plant Foods Hum. Nutr. 69 (1), 50–56. doi:10.1007/s11130-013-0401-x
Singh, P., Singh, R. K., Zhou, Y., Wang, J., Jiang, Y., Shen, N., et al. (2022). Unlocking the Strength of Plant Growth Promoting Pseudomonas in Improving Crop Productivity in Normal and Challenging Environments: A Review. J. Plant Interactions 17 (1), 220–238. doi:10.1080/17429145.2022.2029963
Tasselli, G., Filippucci, S., Sannino, C., Turchetti, B., and Buzzini, P. (2017). Psychrophiles: From Biodiversity to Biotechnology. Berlin: Springer, 555–584. doi:10.1007/978-3-319-57057-0_24
Taylor, S. C., Carbonneau, J., Shelton, D. N., and Boivin, G. (2015). Optimization of Droplet Digital PCR from RNA and DNA Extracts with Direct Comparison to RT-qPCR: Clinical Implications for Quantification of Oseltamivir-Resistant Subpopulations. J. Virol. Methods 224, 58–66. doi:10.1016/j.jviromet.2015.08.014
Tedersoo, L., Anslan, S., Bahram, M., Põlme, S., Riit, T., Liiv, I., et al. (2015). Shotgun Metagenomes and Multiple Primer Pair-Barcode Combinations of Amplicons Reveal Biases in Metabarcoding Analyses of Fungi. MycoKeys 10, 1–43. doi:10.3897/mycokeys.10.4852
Thomas-Hall, S. R., Turchetti, B., Buzzini, P., Branda, E., Boekhout, T., Theelen, B., et al. (2010). Cold-Adapted Yeasts from Antarctica and the Italian Alps—Description of Three Novel Species: Mrakia Robertii Sp. nov., Mrakia Blollopis Sp. Nov. And Mrakiella Niccombsii Sp. Nov. Extremophiles 14 (1), 47–59. doi:10.1007/s00792-009-0286-7
Timell, T. E. (1959). The Constitution of a Hemicellulose from Sugar Maple (Acer Saccharum). Can. J. Chem. 37 (5), 893–898. doi:10.1139/v59-122
Tyc, O., van den Berg, M., Gerards, S., van Veen, J. A., Raaijmakers, J. M., de Boer, W., et al. (2014). Impact of Interspecific Interactions on Antimicrobial Activity Among Soil Bacteria. Front. Microbiol. 5, 567. doi:10.3389/fmicb.2014.00567
van den Berg, A. K., Perkins, T. D., Godshall, M. A., and Lloyd, S. W. (2009). Metabolism Off-Flavor in Maple Syrup Part I: Identification of the Compound Responsible for Metabolism Off-Flavor. Maple Syrup Digest 21A (1), 15–18.
van den Berg, A. K., Perkins, T. D., and Isselhardt, M. L. (2019). Composition and Properties of Maple Sap, Concentrate, and Permeate. Agric. Sci. 10 (01), 32–45. doi:10.4236/as.2019.101004
VanderSluis, B., Hess, D. C., Pesyna, C., Krumholz, E. W., Syed, T., Szappanos, B., et al. (2014). Broad Metabolic Sensitivity Profiling of a Prototrophic Yeast Deletion Collection. Genome Biol. 15 (4), R64. doi:10.1186/gb-2014-15-4-r64
Whalen, M. L., and Morselli, M. F. (1986). Microbial Ecology of Xylem Sap of Acer saccharum Marsh. Am. J. Bot. 73 5, 694.
Willits, C., Bell, R., and Frank, H. (1961). Maple Sirup. 19. Flavor and Color through Controlled Fermentation of Maple Sap. Food Technol. 15 (11), 473.
Wong, B. L., Baggett, K. L., and Rye, A. H. (2003). Seasonal Patterns of reserve and Soluble Carbohydrates in Mature Sugar maple (Acer Saccharum). Can. J. Bot. 81 (8), 780–788. doi:10.1139/b03-079
Keywords: maple syrup, maple sap, 16S, ITS, predictive functional analysis, allantoate, food quality
Citation: N’guyen GQ, Roblet C, Lagacé L and Filteau M (2022) A Metataxonomic Analysis of Maple Sap Microbial Communities Reveals New Insights Into Maple Syrup Complexity. Front. Syst. Biol. 2:893007. doi: 10.3389/fsysb.2022.893007
Received: 09 March 2022; Accepted: 07 April 2022;
Published: 29 April 2022.
Edited by:
Liliana Fadul, University of Wisconsin-Madison, United StatesReviewed by:
Hang Ma, University of Rhode Island, United StatesCopyright © 2022 N’guyen, Roblet, Lagacé and Filteau. This is an open-access article distributed under the terms of the Creative Commons Attribution License (CC BY). The use, distribution or reproduction in other forums is permitted, provided the original author(s) and the copyright owner(s) are credited and that the original publication in this journal is cited, in accordance with accepted academic practice. No use, distribution or reproduction is permitted which does not comply with these terms.
*Correspondence: Marie Filteau, bWFyaWUuZmlsdGVhdUBmc2FhLnVsYXZhbC5jYQ==
†ORCID ID:Guillaume Quang N’guyenorcid.org/0000-0001-5302-5859
Disclaimer: All claims expressed in this article are solely those of the authors and do not necessarily represent those of their affiliated organizations, or those of the publisher, the editors and the reviewers. Any product that may be evaluated in this article or claim that may be made by its manufacturer is not guaranteed or endorsed by the publisher.
Research integrity at Frontiers
Learn more about the work of our research integrity team to safeguard the quality of each article we publish.