- 1Divison of Microbial and Environmental Biotechnology, ASPEE SHAKILAM Biotechnology Institute, Navsari Agricultural University, Navsari, Gujarat, India
- 2Shri Alpesh N. Patel PG Institute of Science and Research, Sardar Patel University, Anand, Gujarat, India
- 3Division of Plant Biotechnology, ASPEE SHAKILAM Biotechnology Institute, Navsari Agricultural University, Navsari, Gujarat, India
Metagenomics is an approach for directly analyzing the genomes of microbial communities in the environment. The use of metagenomics to investigate novel enzymes is critical because it allows researchers to acquire data on microbial diversity, with a 99% success rate, and different kinds of genes encode an enzyme that has yet to be found. Basic metagenomic approaches have been created and are widely used in numerous studies. To promote the success of the advance research, researchers, particularly young researchers, must have a fundamental understanding of metagenomics. As a result, this review was conducted to provide a thorough insight grasp of metagenomics. It also covers the application and fundamental methods of metagenomics in the discovery of novel enzymes, focusing on recent studies. Moreover, the significance of novel biocatalysts anticipated from varied microbial metagenomes and their relevance to future research for novel industrial applications, the ramifications of Next-Generation Sequencing (NGS), sophisticated bio-informatic techniques, and the prospects of the metagenomic approaches are discussed. The current study additionally explores metagenomic research on enzyme exploration, specifically for key enzymes like lipase, protease, and cellulase of microbial origin.
1 Introduction
Applications for biological enzymes and catalysts in biotechnology are quite promising. They can be utilized for a wide range of things, including making chiral SYNTHONS in the bio-pharmaceutical business, pulp and feed industry, and active components for laundry detergents (Nazir and Harinarayanan, 2016). Further enhancing their use in the biodegradation of natural polymers like starch, cellulose, proteins, and other compounds is the flexible character of these biological enzymes. Beyond yeast and filamentous fungus, there is currently little to no access to industrial enzymes and biocatalysts. It is possible to look for acceptable natural biocatalysts while taking into account enzymatic limitations by combining the discovery of microbial diversity with in vitro evolution technologies (Ghosh et al., 2019).
Every ecosystem on Earth is dominated by microbes. The largest terrestrial and oceanic biomass are made up of microbial communities, which include bacteria, fungi, archaea, and protists. This prodigious microbial diversity can be seen in the presence of approximately 166,244/24,249 (bacteria/fungi) and 49,102 bacteria operational taxonomic units (OTU) in the Dryland and Scotland data sets, respectively (Alves et al., 2018), and 25, 000 different microbial genotypes in just 1 mL. The most intricate microbiome ecology is found in the rumen of ruminants. It consists of several bacterial, fungal, archaeal, and protozoan communities. Gram-negative and Gram-positive bacteria make up around 1011 cells/ml of the rumen contents among them. These obligate anaerobes’ enzymatic activity is crucial for the disintegration of plant polymers into their monomeric constituents, which then yield volatile fatty acids. About 8%–20% of the total rumen microbial biomass is made up of fungi. Hydrolytic enzymes are involved in the digestion of plant fiber and are produced by anaerobic fungi found in the rumen of herbivores. The diversity of microbial species worldwide is thought to be enormous, although just 1% out of which is laboratory cultivable. In this sense, such microbial biomass should be seen as an endless source of genetic innovation (Ghosh et al., 2019).
Unfortunately, a large variety of microorganisms still go unnamed and are incapable of being grown in growth media. Less than 1% of all the environmental microorganisms are represented in the diversity data obtained by the culture-based method. The drawback of the culture-based technique, which has seen a dramatic rise in its use in recent years, has been addressed by metagenomics. In metagenomics, DNA is directly retrieved from the environmental sample without going through a laboratory culturing process. A representative and thorough result is obtained when the diversity of bacteria is analyzed using DNA. Research on the microbial communities in the human gut, sugarcane bagasse waste, and hypersaline environments have all made use of metagenomics (Prayogo et al., 2020).
To improve the insights into metagenomics, the concept of metagenomics must be examined further. Increased research into the knowledge of the microbial community and natural enzymes is anticipated to be influenced by a thorough grasp of metagenomics and its application. Therefore, the purpose of this review is to talk about how metagenomics is being used to discover new enzymes in nature. This review’s main goal is to give readers a thorough understanding of metagenomics, its fundamental methodology, and how it may be used to explore enzymes, particularly in light of recent findings.
2 Metagenomics
Metagenomics, also known as ecological or community genomics, has the unending potential to have a significant impact on the development of bioactive, biomarkers, polymers, medicines, and other different biotech products. The functional screening and sequence-based analysis of microbial bulk DNA from the moderate to extreme environment are notably integrated into metagenomics (Bragg and Tyson, 2014). The majority of environmental microbes cannot be cultured. In addition, most of the enzyme and bio-catalytic potential in these uncatalogued ambient microbial consortia remains unavailable (Handelsman et al., 1998). Only 1%–10% of the microbial genomes are now accessible to us due to the lack of metagenomics methods.
In fact, the development of the molecular metagenomic approach gave scientists the ability to discover the genetic insights into microbes that are present in various habitats, regardless of cultivability, to use the target genes and genomes for biotechnological purposes, and to functionally screen microbial genome sequences in metagenomic libraries. This could act as a collection of fresh enzymes and useful proteins (Lear et al., 2018). In a metagenomic approach, bulk microbial DNA is extracted from arbitrary environmental samples or enrichment cultures, metagenomes are archived or cloned in heterologous hosts, metagenomic libraries are produced, and these libraries are then functionally screened for genes of interest or DNA expression before being screened for interesting enzymatic activities (Pucker et al., 2019). In this context, well established metagenomic technologies are used to enable or increase the inherent limitation of culture-based procedures during isolation and cultivation.
Basic strategy to implement metagenomics study on any aspect is depicted in Figure 1, which mainly comprises structural metagenomics and functional metagenomics (Prayogo et al., 2020). The study of structural metagenomics is concerned with the composition of microbial communities. Understanding the interactions between the various elements that go into creating a community in a given context is the main goal of the study of community structure. For the study of ecology and biological processes, relationships between community members provide crucial information (Woese et al., 1985). Basic structural metagenomics techniques include microbial community analysis such as taxonomic profiling, gene prediction, and metabolic pathways, as well as assembly and binning (Ghosh et al., 2019). A unique challenge in discovering natural substances that can be used in the biotechnology sector is the study of functional metagenomics. To find novel enzymes, functional metagenomics uses several fundamental techniques, including gene construction, screening, and gene expression. These techniques can be followed by rigorous bioinformatic analysis, including sequence digging, Pfam, structure prediction, and phylogenetic analysis, as well as protein product characterization, including analysis of protein activity and optimal pH and temperature ranges.
Around late 1998, the groundwork for the metagenomics approach solidified due to advances in genomics and molecular biology (Handelsman et al., 1998). The microbiologists did not agree unanimously with Staley and Konopka (1985) conclusions that majority of microorganisms were unreachable. Later research by Torsvik et al. (1990) gave compelling evidence that methodologies relying on culturing could not capture the whole spectrum of microorganisms. Further research by Torsvik et al. (2002) has shown that just 1% of the world’s microbial communities are represented by microorganisms that have been cultivated and isolated to date. The development of microbiology during that period was impacted by Woese’s hypothesis, which was made in 1985 and claimed that the 16S rRNA transmits a molecular clock with a high degree of functional permanence (Woese et al., 1985). The 16s rRNA gene has been extensively used for molecular characterization because of its size, multigene content, and prevalence in all bacteria. The complete genomic DNA was isolated, fractionated, and cloned into a bacteriophage lambda vector using this method (Schmidt et al., 1991a). Despite the extensive sophistication of the metagenomics technique, the utilization of the metabolic and catalytic activities of microbial consortia was insufficient. The enzymes, cellulase and xylanase genes were discovered in 1995 by Healy et al. (1995) who built functional-based screening of metagenomics libraries, sometimes known as “zoo libraries”.
Metagenomic screening is a well-established method for studying the genomic DNA of uncultured microorganisms (Rondon et al., 2000; Cowan et al., 2005). The exploitation of hidden microbial communities has been made possible by the incorporation of potential advanced functional genomics, bio-informatics tools, system and synthetic biology, and functional screening methods like SIGEX (substrate induced gene expression) (Uchiyama et al., 2005), METREX (metabolite regulated expression) (Changhui, Jo, 2005), Next-Generation Sequencing (NGS), and High Throughput Screening (HTS) in a metagenomic study (Kumar et al., 2015). In other words, these are essential additions to completely comprehend how microbial communities function and how they interact within niches (Woese et al., 1985). Globally accessible generation of megabases of sequence data is now possible, thanks to the NGS’s dramatically reduced operational costs. This has made metagenomics possible everywhere.
As the first of its sort, Tyson et al., 2004 reported the sequencing of 76 Mbp of DNA from an acid mine drainage biofilm in 2004. The metabolic route of the biofilm community was further illuminated by this work. It was discovered to be more difficult to sequence metagenomic DNA from the Sargasso Sea that was over one GBP in size (Tyson et al., 2004; Venter et al., 2004). The Sargasso Sea metagenomic sequencing project identified over 1.2 million putative genes, which further demonstrated the innovative approach to gene discovery (Cowan et al., 2005). However, the Sargasso Sea’s abundant biodiversity and limited sequencing coverage made it difficult to assemble the entire genome. Whole genome assembly may be made possible by increased sequencing coverage and the use of small, medium, and large insert libraries (Schmidt et al., 1991b; Béjà, 2004; Cowan et al., 2005).
According to Madhavan et al. (2017), Function-based and sequence-based metagenomic library screening are the two fundamental methods that metagenomic technology uses to screen biomolecules from environmental materials. In all situations, the metagenomic libraries are created by cloning genomic DNA fragments into the proper expression vectors, such as plasmids, cosmids, lambda phages, or fosmids, depending on the intended target gene size which allow the necessary genes to be expressed (Figure 2); (Madhavan et al., 2017).
Sanger sequencing has been largely replaced as the primary source of sequence data for metagenomics by the extensive use of sophisticated, high-throughput NGS technology. NGS, in contrast to Sanger Sequencing, may identify even extremely rare and low-abundance bacteria from different metagenomes (Albertsen et al., 2013; Sims et al., 2014). Previously, classic approaches used in metagenomics research and analysis included denaturing gradient gel electrophoresis (DGGE) (Muyzer et al., 1993), terminal restriction fragment length polymorphism (T-RFLP) analysis (Fierer and Jackson, 2006), and Sanger sequencing of a library of 16s rRNA gene clones (Lear et al., 2018). The latter method was largely dominant in gaining access to knowledge of the genetic makeup of microbes from varied natural habitats. The potential of this strategy is increased by using E. coli as the host cell (Kumar et al., 2015).
Sanger sequencing method span for more than three decades followed by the development of the second-generation sequencing technique called as next-generation sequencing” (NGS). The second-generation sequencing approach uses several technological platforms, including Roche/454, Ion torrent, and Ilumina (Torsvik et al., 1990). Second-generation sequencing has advantages over first-generation sequencing, according to Bragg and Tyson (Torsvik et al., 2002), including 1) faster throughput, 2) lower operating costs, and 3) rapid detection of sequencing findings without the need for electrophoresis (Staley and Konopka, 1985). Despite being adequately advanced, the second generation of sequencing technology still has issues with costs, outcomes, and time that should be improved which ultimately lead to the creation of third generation of sequencing technology. Third-generation sequencing is superior to second-generation sequencing in many aspects, as it is quicker, less expensive, and requires no PCR processing (Torsvik et al., 1990). The third-generation sequencing approach uses the PacBio RS (Pacific Bioscience) and Oxford Nanopore technology platforms (Staley and Konopka, 1985). (Supplementary Table S1 shows a brief comparison between the different sequencing platforms).
The lack of appropriate enzymes and an appropriate host for effective gene expression were some of the limitations for various bio-transformation processes until recently, even though this metagenomics approach has greatly proven to be effective in unlocking the microbial world and obtaining an arsenal of multi-functional enzymes. Similarly, some of the crucial key problems with activity-based metagenomics screening when dealing with natural genomic heterogeneity and cross-strain assemblies are low sensitivity and low throughput (Staley and Konopka, 1985). The timely analysis is being overcome by the sequencing data extracted from the metagenomic database (Rashid and Stingl, 2015). To gain a deeper understanding of biological identity within a single cell, fluorescence-activated cell sorting (FACS), phenotypic micro-array (PM) (Culligan et al., 2014), community isotype array (CIArray) (Tourlousse et al., 2013a), fluorescence in situ hybridization (FISH), and fluorescence microscopy are helpful tools (Mircea et al., 2007). High throughput screening techniques like SIGEX (substrate-induced gene expression) (Schmidt et al., 1991b), PIGEX (product-induced gene expression) (Taku and Kentaro, 2010), and METREX (metabolite-regulated expression) (Healy et al., 1995) have also shown to be highly effective in overcoming the aforementioned restrictions. There is currently no established gold standard for the analysis of metagenomic data.
3 Various tactics for metagenomics analysis
In metagenomics study, method choice is a very crucial strategy. In essence, the method is split into two categories: molecular methods and bioinformatics methods.
3.1 Molecular approach
The study of metagenomics uses the metagenome, or genome, of an environmental community or specific environmental niches as its research problem focusing on specific biological question which differs marginally from genome-based individual investigations (single genome). In metagenome, direct DNA extraction from ambient samples is being done and the process/techniques could be varied depending on the kind of research sample being employed. (Tourlousse et al., 2013b). The simplest way is to extract metagenomic DNA using commercial kits, which simply require reagents and the standard procedure to be followed that the manufacturer has provided and it consumes less time (Diniz and Canduri, 2017). Researchers most frequently use the PowerSoil and DNeasy PowerMax (Qiagen) kits to analyze soil samples, whereas the DNeasy Blood and Tissue Kits (Qiagen) kits to analyze seawater and groundwater samples. As a result of their greater time efficiency, kits are preferred by researchers, though certain research framework that follows their lab established protocols leads to greater findings with specific objectivity. The success of the subsequent stage will depend on the outcome of the metagenomic DNA extraction process, which is a critical step. A precise DNA size must be produced during metagenome extraction. Fragments utilized for metagenome analysis typically range in size from 600 bp to 25 kbp. The extracted sample will not be suitable for additional metagenomic analysis due to poor results. Therefore, the following needs to be taken into consideration: avoid physical interference with genetic material and avoid protein, humic acid, and metal contamination. The pH, mineral content, and type of soil are other variables that could influence the outcome of DNA extraction.
The purity and concentration of extracted metagenomic DNA plays a very important role in further experimentation part. Three techniques are widely utilized, i.e., UV absorbance, fluorescence staining, and the di-phenylamine reaction, to determine the DNA concentrations and purity values (Mehmood et al., 2014). The most common and frequently used technique by researchers to determine the DNA’s content and purity is the UV absorbance method due to its simplicity, applicability, and low cost (Healy et al., 1995). To confer the proper metagenomic DNA standards, researchers usually judge the ratio of 260/230 which detect the proteins, guanidine HCL, EDTA, lipids, phenols, and salt compounds. Results for DNA purity can be worsened by contaminants, i.e., humic acid and protein which are the most frequent contaminants in metagenome samples (Lucena-Aguilar et al., 2016). Protein and phenol pollutants typically have absorption values that are less than 1.6, at 260/280. Meanwhile, if the 260/280 absorption ratio value is more than 2.0, RNA contamination of DNA is present (Escuder-Rodríguez et al., 2018). A common qualitative technique for separating, determining sizes and purifying nucleic acids is gel electrophoresis (Schröder et al., 2014; Tiwari et al., 2018; Paula et al., 2019).
The 16S rRNA genes are quite conservative and frequently used as a benchmark when creating taxonomies. Prokaryotes typically include 35% protein and 65% rRNA (ribosome-ribonucleic Acid). The large subunits (LSU) (the 50S), which include two rRNA molecules (5S and 23S), and small subunits (SSU) (30S), which contain a single rRNA molecule (16S), make up each bacterial ribosome (Tavano et al., 2018). Prokaryotic organisms are classified, identified, and grouped employing the 16S rRNA region as a reference for taxonomy profiling studies (Razzaq et al., 2019). The 16S rRNA have nine sections known as hypervariable regions (V1-V9) with a combined length of roughly 1,500 bp to make up this gene and the variety of prokaryotic organisms can be distinguished by these nine zones. Genus and species readings can typically be distinguished at a minimum level of 95% for the genus and 97% for the species, whereas strain readings can typically be distinguished at a minimum level of 99% (Devi et al., 2016). In metagenomic research, the V2-V3 region is typically a great place to employ a gene marker. However, several scientists have used alternative target regions in the 16S rRNA gene’s V region to study the diversity of microorganisms. There are three factors that make 16 rRNAs an acceptable marker for taxonomy profiling: 1) all prokaryotic organisms have 16 rRNA genes; 2) lateral gene transfer is virtually unheard of; and 3) the conservative ribosomal protein structure makes the sequence very durable (Tavano et al., 2018).
3.2 Bioinformatics approach
The analysis of metagenomics relies heavily on profound bioinformatics analysis. Utilization of bioinformatics tools depends on exploration goals; a proper biological question and the type of analysis being hypothesized. Various online tools, standalone software and server are employed for the assessment of microbial diversity, network analysis, metabolic pathway mapping and functional analysis. Some of the handy examples includes, MG-RAST, EBI, RDP, QIIME, MOTHUR, SqueezeMeta etc., as well as the approach to analyze the metagenome sequence data is also different depending on what type of output or results that researchers are looking up to materialize. Several approaches which are used frequently during analysis includes assembly generation, binning, sequence analysis, Pfam and many more.
3.2.1 Assembly
Assembly is the process of reassembling small meta-genome readings into a lengthy sequence called Contigs. Assembly employs one of two commonly used approaches, overlap–layout–consensus (OLC) or the de Bruijn graph (López-López et al., 2014), although some researchers have created and successfully employed assembly methods such as hybrid and iterative joining (Kaur et al., 2016). The de Bruijn graph, on the other hand, is the most widely used approach, moreover it is less expensive than OLC since it does not require pairwise comparisons. BBAP, Genovo, MegaGT, and MEGAHIT etc., are some of the bioinformatic tools that can be utilized in assembly (Hårdeman and Sjöling, 2007).
3.2.2 Binning
Binning is the process of clustering sequences created during the assembly process. Binning classifies sequences known as contigs to represent a biological taxon. This approach is used after raw sequence reads have been assembled into contigs. MetaWatt and CONCOCT are two software alternatives for binning analysis. MetaWatt provides more accuracy than existing approaches and is easier to use, while CONCOCT, has great precision and can group together complex microbial communities (Prayogo et al., 2020).
3.2.3 Sequence analysis
Brief understanding reveals that the process of detecting sections of the same biological sequence is known as sequence analysis which includes simple alignment and multiple alignments. A simple alignment is defined as the alignment of two sequences, whereas multiple alignments are defined as the alignment of more than two sequences (Tourlousse et al., 2013b). Most versatile example is application of BLAST (Basic Local Alignment Tool), a technique for comparing sequences from distinct organisms and alignment’s score is assigned an expectation value (E value), which is a statistical significance metric (Mircea et al., 2007).
3.2.4 Pfam analysis
Pfam is a database of curated protein families, which is defined by double alignments and a profile hidden Markov model (HMM). Profile HMMs are probabilistic models used for the statistical inference of homology built from an aligned set of curator-defined family-representative sequences. The common purpose of the Pfam database is to provide a complete and accurate classification of protein families and domains. (Taku and Kentaro, 2010).
3.2.5 Protein structure prediction analysis
Sequence of amino acids are central to the design of proteins which is essentially divided into secondary, tertiary, and quaternary structures. The basic structure is dictated by the sequence of genes that encode it. Perception of protein structure greatly helps to understand its crucial function and its significance. Bioinformatics prediction study of protein structure can aid in understanding the physical properties of a protein and its functions at advance level (Jia et al., 2013).
3.2.6 Phylogenetic analysis
The term “phylogenetic analysis of functional metagenomics” refers to processes that are used to rebuild the evolutionary links between groups of protein molecules and to anticipate specific properties of a molecule. The likelihood approaches, parsimony methods, and distance methods are used to create phylogenetic trees. There is no perfect method, and each has its own set of advantages and disadvantages. MEGA (Molecular Evolutionary Genetics Analysis), MOLPHY, and PHYLIP are examples of phylogenetic analysis tools (Lappalainen et al., 2013).
4 Novel enzyme exploration using a metagenomic method
Specially designed organic molecules are produced by all living organisms, ranging from large gene-encoded peptides to small volatile chemicals, which have evolved over the years. As natural products, they are highly desirable in the field of medicine, agriculture, nutrition, and industries. The tremendous progress in the field of genomics has revealed that the metabolic capacity of virtually all organisms is hugely underappreciated. Focusing mainly in bacteria and fungi, genome mining technologies have now accelerated towards metabolite discovery. Recent efforts are now towards all life forms, including protists, plants and animals, and advanced integrative omics technologies are enabling effective mining of this molecular diversity.
Plethora of information can be extracted from a genome sequence. It can range from function to conserved patterns or signatures and even the (predicted) structure. It is assumed that many valuable enzymatic details still have to be found among wild-type enzymes resulting from years of natural evolution. To explore these uncharacterized enzymes, new ways are required. In this context, modern bioinformatic tools and genome mining can help for the discovery of new biocatalysts. Moreover, the profiling and characterization of new enzymes will provide more activity-attributed sequences, thereby increasing the data of reference sequences available to determine novel biocatalysts by sequence comparison methods. The interdisciplinary nature of natural products will culminate the fields of chemistry, biology, informatics, and medicine and help to expand the role of genome mining in discovery of novel organic substances. In the past, research on enzyme exploration still relied on conventional techniques, such as cultivating microorganisms on growth media. But as technology advances, scientists are now able to investigate novel enzymes without cultivating them on growth media. The enzymes like lipase, protease, and cellulose etc., are crucial for industrial operations (Culligan et al., 2014).
The discovery of novel enzymes is valuable as it helps to perceive enzyme evolution, enzyme structure, function, basic mechanisms of catalysis, as well as identification of novel protein folds. Novel enzymes can be pillars in catalyzing industrial chemical synthesis reactions, ultimately providing ‘clean’ options for chemical synthesis at large-scale. Presently there is need of highly robust enzymes in many different industries like pharmaceutical and agricultural industries. The expansion of the repertoire of known enzymes, both for research and industrial applications, is currently the subject of immense interest. Additionally, the vast amount of DNA sequence data generated can be exploited for other areas such as medicinal chemistry, characterization of human physiological processes, the identification and validation of new drug targets in human pathogens, and the discovery of new chemical entities (NCEsa) from natural sources. It can also become a steppingstone for synthesizing new drugs.
4.1 Lipase
The hydrolytic breakage of ester bonds between carboxylic acids and alcohol groups is catalyzed by the enzymes known as lipases (Culligan et al., 2014). The detergent, food, biodiesel, and bioremediation sectors all use this enzyme. The most well-known producers of bacterial lipases are Bacillus spp. including B. acidophilus, B. licheniformis, B. pumilus, and B. subtilis (Cowan et al., 2005; Uchiyama et al., 2005; Tourlousse et al., 2013b). Currently, scientists are competing to investigate more organisms that may be able to produce stronger lipase. Using a functional metagenomic approach, Hardeman and Sjoling (Mircea et al., 2007) discovered the h1Lip1 gene, which shares a 54% similarity with the lipase of Pseudomonas putida and optimally active at 35°C (low temperature). In general, the highest lipase character at low temperatures is appropriate for the cold washing process in detergents, according to Lopez-López et al. (Culligan et al., 2014). Additionally, many additional enzymes found in the metagenome library have special biochemical properties that make them useful for commercial applications like a solvent-resistant enzyme is one illustration, and soil detergents are tainted with petroleum hydrocarbons (Lear et al., 2018).
4.2 Protease
Enzymes called proteases break down peptide bonds in amino acid chains. The detergent, pharmaceutical, food, and beverage sectors all use this enzyme (López-López et al., 2014). Bacillus sp. is currently the addressed as the most well-known protease producer in the industrial sector (Kaur et al., 2016). The growth of metagenomic technology makes it possible to look for additional creatures/sources that may be potentially more effective. Devi et al. (2016) reported the protease enzyme from organic waste, encoded by the Prt1A gene and it behaves best around 55°C. Later on, Pessoa et al. (2017) identified a gene that produces proteases that are most active at 60°C and favorable to work at most o the industrial process.
4.3 Cellulase
A set of enzymes known as cellulases catalyze the breakdown of cellulose polymers into simpler sugars (Tavano et al., 2018). The detergents, processing of cotton, and the paper sector can all be benefited from this enzyme (Tavano et al., 2018). Aspergillus sp. Has been identified as an entity with strong cellulase activity through standard exploration of cellulase enzymes (Pessoa et al., 2017). However, metagenomic techniques show that cellulase enzymes are broadly distributed in a variety of creature types. Many genera were discovered in bamboo paper manufacturing facilities with high cellulose conditions. While, previous investigations showed that the genes producing the cellulase enzyme were also present in the microbial communities of bagasse waste and the human intestinal microbial community. Currently, many researchers are digging the hot springs, other high-temperature situations and harsh conditions (Pessoa et al., 2017) to obtain the cellulase enzyme which worked best at high temperature (thermostable).
4.4 Other enzymes
Researchers have discovered several enzymes from metagenome sources that are commercially available and on processes. Table 1 depicts a list of different enzymes that are mined using the functional metagenomic analysis approach. A new bleomycin resistance dioxygenase (BRPD) was recently identified from polluted agricultural soil through metagenomic approach (Taku and Kentaro, 2010) which catalyze the breakdown of hydrocarbon substrates like pesticides, it serves a purpose in the bioremediation process. Furthermore, Berini et al. (Jia et al., 2013) discovered the 53D1 gene, which encodes chitinases that may be exploited to manage plant pests, Lepidoptera Bombyx mori. AHL-lactonase (Zhang et al., 2021), a transaminase used in the pharmaceutical industry (Madhavan et al., 2017), oxoflavin-degrading enzyme used in agriculture (Lappalainen et al., 2013) as well many other enzymes originating from metagenome sources have also been revealed in recent investigations.
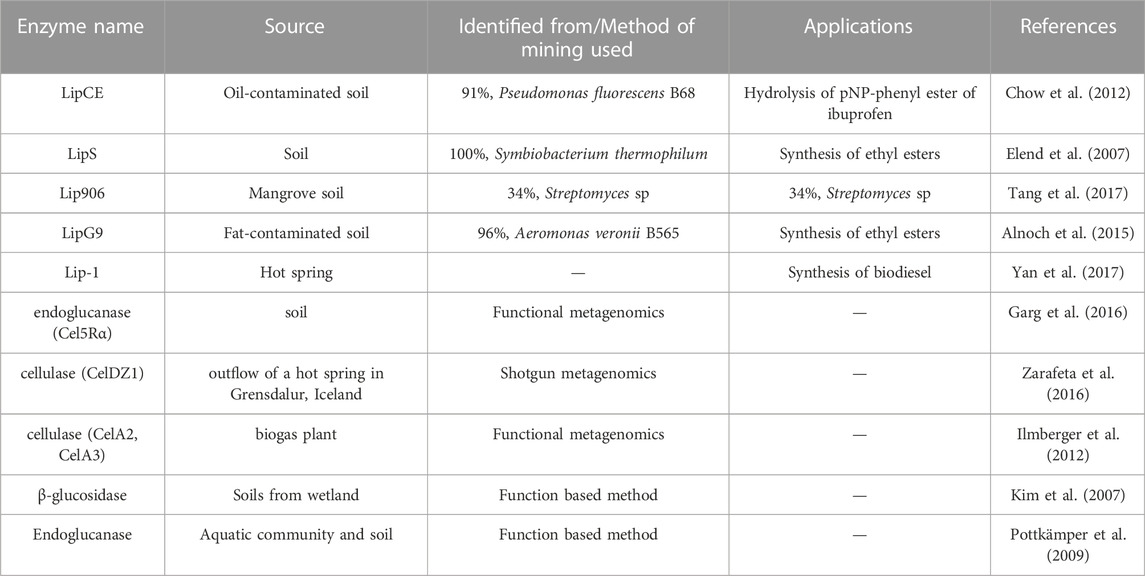
TABLE 1. Depicts a list of different enzymes that are mined using the functional metagenomic analysis approach.
5 Conclusion
Metagenomics is a potential area of research, and to study in vivo imprints of microbial genomics. The information acquired from the metagenomic library is critical for investigating the potential of diverse microbial enzymatic networks and correlating sequence data to molecular structure and functional features. The multifunctional properties of novel biocatalysts discovered using a metagenomic approach will undeniably fascinate the scientific community and industrial specialists interested in white and red biotechnology. The ease of access to numerous methods for extracting DNA from varied environments, the reduction in sequencing costs, advancements in NGS platforms, and readily available bio-analytical algorithms and simulation tools have propelled metagenomics into an exciting new phase. Using a metagenomic method, researchers discovered several unique enzymes from nature, including cellulases, proteases, lipases, and other enzymes such as BRPD, chitinases, oxoflavin-degrading enzymes, transaminases, and AHL-lactonase that are valuable to industry. Despite significant developments in functional screening capabilities, the characterization of most biocatalysts at an industrial scale remains a key hurdle to their discovery. To some extent, the introduction of a diverse variety of different host vectors could relieve the issue of heterologous expression of metagenomic DNA in functional screening. Metagenomic understanding and application are predicted to have an impact on the development of technology that is valuable to humanity.
Author contributions
Conceptualization VP, HGC; Writing original draft TP, NS, VP, and SP; Editing and revision of subsequent drafts VP, SP and VM; Revision of final draft: All authors. All authors have read and agreed to the publisned version of th manuscript.
Conflict of interest
The authors declare that the research was conducted in the absence of any commercial or financial relationships that could be construed as a potential conflict of interest.
Publisher’s note
All claims expressed in this article are solely those of the authors and do not necessarily represent those of their affiliated organizations, or those of the publisher, the editors and the reviewers. Any product that may be evaluated in this article, or claim that may be made by its manufacturer, is not guaranteed or endorsed by the publisher.
Supplementary material
The Supplementary Material for this article can be found online at: https://www.frontiersin.org/articles/10.3389/fsysb.2022.1046230/full#supplementary-material
References
Albertsen, M., Hugenholtz, P., Skarshewski, A., Nielsen, K. L., Tyson, G. W., and Nielsen, P. H. (2013). Genome sequences of rare, uncultured bacteria obtained by differential coverage binning of multiple metagenomes. Nat. Biotechnol 31 (6), 533–538. doi:10.1038/nbt.2579
Alnoch, R. C., Martini, V. P., Glogauer, A., Costa, A. C., Piovan, L., and Muller-Santos, M. (2015). Immobilization and characterization of a new regioselective and enantioselective lipase obtained from a metagenomic library. PLoS One. 10(2):e0114945. doi:10.1371/journal.pone.0114945
Alves, L. de F., Westmann, C. A., Lovate, G. L., de Siqueira, G. M. V., Borelli, T. C., and Guazzaroni, M. E. (2018). Metagenomic approaches for understanding new concepts in microbial science. Heng H, editor. Int. J. Genomics. 2018. 1-15. doi:10.1155/2018/2312987
Bashir, Y., Pradeep Singh, S., and Kumar Konwar, B. (2014). “Metagenomics: An application based perspective,”. Chin. J. Biol., 2014. 1-7. doi:146030doi:10.1155/2014/146030
Béjà, O. (2004). To BAC or not to BAC: Marine ecogenomics. Curr. Opin. Biotechnol. 15 (3), 187–190. doi:10.1016/j.copbio.2004.03.005
Bragg, L., and Tyson, G. W. (2014). “Metagenomics Using next-generation sequencing,” in Environmental microbiology: Methods and protocols (New York, NY, USA: Springer Science), 1096. doi:10.1007/978-1-62703-712-9
Chow, J., Kovacic, F., Dall Antonia, Y., Krauss, U., Fersini, F., and Schmeisser, C. (2012) The metagenome-derived enzymes LipS and LipT increase the diversity of known lipases. PloS One. 7(10):e47665. doi: doi:10.1371/journal.pone.0047665
Cowan, D., Meyer, Q., Stafford, W., Muyanga, S., Cameron, R., and Wittwer, P. (2005) Metagenomic gene discovery: Past, present and future. Trends Biotechnol. Jun 1;23(6):321–329. doi:10.1016/j.tibtech.2005.04.001
Culligan, E. P., Marchesi, J. R., Hill, C., and Sleator, R. D. (2014). Combined metagenomic and phenomic approaches identify a novel salt tolerance gene from the human gut microbiome. Front. Microbiol. 5, 189. doi:10.3389/fmicb.2014.00189
Devi, S. G., Fathima, A. A., Sanitha, M., Iyappan, S., Curtis, W. R., and Ramya, M. (2016). Expression and characterization of alkaline protease from the metagenomic library of tannery activated sludge. J. Biosci. Bioeng. 122 (6), 694–700. doi:10.1016/j.jbiosc.2016.05.012
Diniz, W. J. S., and Canduri, F. (2017). REVIEW-ARTICLE bioinformatics: An overview and its applications. Genet. Mol. Res. 16. doi:10.4238/gmr16019645
Elend, C., Schmeisser, C., Hoebenreich, H., Steele, H. L., and Streit, W. R. (2007). Isolation and characterization of a metagenome-derived and cold-active lipase with high stereospecificity for (R)-ibuprofen esters. J. Biotechnol. 130(4):370–377. doi:10.1016/j.jbiotec.2007.05.015
Escuder-Rodríguez, J., DeCastro, M. E., Cerdán, M. E., Rodríguez-Belmonte, E., Becerra, M., and González-Siso, M. I. (2018). Cellulases from thermophiles found by metagenomics. Microorganisms 6, 66–26. doi:10.3390/microorganisms6030066
Fierer, N., and Jackson, R. B. (2006). The diversity and biogeography of soil bacterial communities. Proc. Natl. Acad. Sci. U. S. A. 103 (3), 626–631. doi:10.1073/pnas.0507535103
Garg, R., Srivastava, R., Brahma, V., Verma, L., Karthikeyan, S., and Sahni, G. (2016). Biochemical and structural characterization of a novel halotolerant cellulase from soil metagenome. Sci. Rep. 6 (1), 39634–39635. doi:10.1038/srep39634
Ghosh, A., Mehta, A., and Khan, A. M. (2019). “Metagenomic analysis and its applications,” in Encyclopedia of bioinformatics and computational biology [internet]. Editors S. Ranganathan, M. Gribskov, K. Nakai, and C. Schönbach (Oxford, UK: Academic Press), 184–193.
Handelsman, J., Rondon, M. R., Brady, S. F., Clardy, J., and Goodman, R. M. (1998). Molecular biological access to the chemistry of unknown soil microbes: A new frontier for natural products. Chem. Biol. 5 (10), R245–R249. doi:10.1016/s1074-5521(98)90108-9
Hårdeman, F., and Sjöling, S. (2007). Metagenomic approach for the isolation of a novel low-temperature-active lipase from uncultured bacteria of marine sediment. FEMS Microbiol. Ecol. 59 (2), 524–534. doi:10.1111/j.1574-6941.2006.00206.x
Healy, F. G., Ray, R. M., Aldrich, H. C., Wilkie, A. C., Ingram, L. O., and Shanmugam, K. T. (1995). Direct isolation of functional genes encoding cellulases from the microbial consortia in a thermophilic, anaerobic digester maintained on lignocellulose. Appl. Microbiol. Biotechnol. 43 (4), 667–674. doi:10.1007/BF00164771
Ilmberger, N., Meske, D., Juergensen, J., Schulte, M., Barthen, P., and Rabausch, U. (2012). Metagenomic cellulases highly tolerant towards the presence of ionic liquids—Linking thermostability and halotolerance. Appl. Microbiol. Biotechnol. 95 (1), 135–146. doi:10.1007/s00253-011-3732-2
Jia, B., Xuan, L., Cai, K., Hu, Z., Ma, L., and Wei, C. (2013). NeSSM: A next-generation sequencing simulator for metagenomics. PLoS One 8 (10), e75448. doi:10.1371/journal.pone.0075448
Kaur, G., Singh, A., Sharma, R., Sharma, V., Verma, S., and Sharma, P. K. (2016). Cloning, expression, purification and characterization of lipase from Bacillus licheniformis, isolated from hot spring of Himachal Pradesh, India. 3 Biotech. 6, no. 1 : 1-10. doi:10.1007/s13205-016-0369-y
Kim, S. J., Lee, C. M., Kim, M. Y., Yeo, Y. S., Yoon, S. H., and Kang, H. C. (2007). Screening and characterization of an enzyme with beta-glucosidase activity from environmental DNA. J. Microbiol. Biotechnol. 17 (6), 905–912.
Kumar, S., Krishnani, K. K., Bhushan, B., and Brahmane, M. P. (2015). Metagenomics: Retrospect and prospects in high throughput age. Canovas M, editor. Biotechnol. Res. Int. 2015: 1-13. doi:10.1155/2015/121735
Williamson, L. L., Borlee, B. R., Schloss, P. D., Guan, C., and Allen, H. K. (2005). Intracellular screen to identify metagenomic clones that induce or inhibit a quorum-sensing biosensor. Appl. Environ. Microbiol. 71 (10), 6335–6344. doi:10.1128/AEM.71.10.6335-6344.2005
Lappalainen, T., Sammeth, M., Friedländer, M. R., ‘t Hoen, P. A. C., Monlong, J., and Rivas, M. A. (2013). Transcriptome and genome sequencing uncovers functional variation in humans. Nature 501 (7468), 506–511. doi:10.1038/nature12531
Lear, G., Dickie, I., Banks, J. C., Boyer, S., Buckley, H. L., and Buckley, T. R. (2018). Methods for the extraction, storage, amplification and sequencing of DNA from environmental samples. N. Z. J. Ecol. 42 (1), 10–50A. doi:10.20417/nzjecol.42.9
López-López, O., E. Cerdan, M., and I. Gonzalez Siso, M. (2014). New extremophilic lipases and esterases from metagenomics. Curr. Protein Pept. Sci. 15 (5), 445–455. doi:10.2174/1389203715666140228153801
Lucena-Aguilar, G., Sánchez-López, A. M., Barberán-Aceituno, C., Carrillo-Ávila, J. A., López-Guerrero, J. A., and Aguilar-Quesada, R. (2016). DNA source selection for downstream applications based on DNA quality indicators analysis. Biopreserv. Biobank. 14 (4), 264–270. doi:10.1089/bio.2015.0064
Madhavan, A., Sindhu, R., Parameswaran, B., Sukumaran, R. K., and Pandey, A. (2017). Metagenome analysis: A powerful tool for enzyme bioprospecting. Appl. Biochem. Biotechnol. 183, 636–651. doi:10.1007/s12010-017-2568-3
Mehmood, M. A., Sehar, U., and Ahmad, N. (2014). Use of bioinformatics tools in different spheres of life sciences. J. Data Min. Genomics Proteomics 5, 1–13. doi:10.4172/2153-0602.1000158
Mircea, P., Marion, W., Don, H., Karsten, Z., and Garcia, J. A. (2007). Targeted access to the genomes of low-abundance organisms in complex microbial communities. Appl. Environ. Microbiol. 73(10):3205–3214. doi:10.1128/AEM.02985-06
Muyzer, G., de Waal, E. C., and Uitterlinden, A. G. (1993). Profiling of complex microbial populations by denaturing gradient gel electrophoresis analysis of polymerase chain reaction-amplified genes coding for 16S rRNA. Appl. Environ. Microbiol. 59 (3), 695–700. doi:10.1128/AEM.59.3.695-700.1993
Nazir, A., and Harinarayanan, R. (2016). (p)ppGpp and the bacterial cell cycle. J. Biosci. 2, 277–282. doi:10.1007/s12038-016-9611-3
Paula, C. C., Vidaurre Montoya, Q., Meirelles, L., Farinas, C., Rodrigues, A., and Seleghim, M. (2019). High cellulolytic activities in filamentous fungi isolated from an extreme oligotrophic subterranean environment (Catão cave) in Brazil. An. Acad. Bras. Cienc. 91, e20180583. doi:10.1590/0001-3765201920180583
Pessoa, T. B. A., Rezende, R. P., Marques, E. de L. S., Pirovani, C. P., dos Santos, T. F., and dos Santos Gonçalves, A. C. (2017). Metagenomic alkaline protease from mangrove sediment. J. Basic Microbiol. 57 (11), 962–973. doi:10.1002/jobm.201700159
Pottkämper, J., Barthen, P., Ilmberger, N., Schwaneberg, U., Schenk, A., and Schulte, M. (2009). Applying metagenomics for the identification of bacterial cellulases that are stable in ionic liquids. Green Chem. 11 (7), 957–965. doi:10.1039/B820157A
Prayogo, F. A., Budiharjo, A., Kusumaningrum, H. P., Wijanarka, W., Suprihadi, A., and Nurhayati, N. (2020). Metagenomic applications in exploration and development of novel enzymes from nature: A review. J. Genet. Eng. Biotechnol. 18 (1), 39. doi:10.1186/s43141-020-00043-9
Pucker, B., Schilbert, H. M., and Schumacher, S. F. (2019). Integrating molecular biology and bioinformatics education. J. Integr. Bioinform. 16 (3), 20190005. doi:10.1515/jib-2019-0005
Rashid, M., and Stingl, U. (2015). Contemporary molecular tools in microbial ecology and their application to advancing biotechnology. Biotechnol. Adv. 33 (8), 1755–1773. doi:10.1016/j.biotechadv.2015.09.005
Razzaq, A., Shamsi, S., Ali, A., Ali, Q., Sajjad, M., and Malik, A. (2019). Microbial proteases applications. Front. Bioeng. Biotechnol. 7, 110. doi:10.3389/fbioe.2019.00110
Rondon, R. M., August, P. R., Bettermann, A. D., Brady, S. F., Grossman, H. T., and Liles, M. R. (2000). Cloning the soil metagenome: A strategy for accessing the genetic and functional diversity of uncultured microorganisms. Appl. Environ. Microbiol. 66(6):2541–2547. doi:10.1128/AEM.66.6.2541-2547.2000
Schmidt, T., Jung, C., and Metzlaff, M. (1991). Distribution and evolution of two satellite DNAs in the genus Beta. Theor. Appl. Genet. 82 (6), 793–799. doi:10.1007/BF00227327
Schmidt, T. M., DeLong, E. F., and Pace, N. R. (1991). Analysis of a marine picoplankton community by 16S rRNA gene cloning and sequencing. J. Bacteriol. 173 (14), 4371–4378. doi:10.1128/jb.173.14.4371-4378.1991
Schröder, C., Elleuche, S., Blank, S., and Antranikian, G. (2014). Characterization of a heat-active archaeal β-glucosidase from a hydrothermal spring metagenome. Enzyme Microb. Technol. 57, 48–54. doi:10.1016/j.enzmictec.2014.01.010
Sims, D., Sudbery, I., Ilott, N. E., Heger, A., and Ponting, C. P. (2014). Sequencing depth and coverage: Key considerations in genomic analyses. Nat. Rev. Genet. 15 (2), 121–132. doi:10.1038/nrg3642
Staley, J. T., and Konopka, A. (1985). Measurement of in situ activities of nonphotosynthetic microorganisms in aquatic and Terrestrial habitats. Annu. Rev. Microbiol. 39 (1), 321–346. doi:10.1146/annurev.mi.39.100185.001541
Taku, U., and Kentaro, M. (2010). Product-induced gene expression, a product-responsive reporter assay used to screen metagenomic libraries for enzyme-encoding genes. Appl. Environ. Microbiol. 76(21):7029–7035. doi:10.1128/AEM.00464-10
Tang, L., Xia, Y., Wu, X., Chen, X., Zhang, X., and Li, H. (2017). Screening and characterization of a novel thermostable lipase with detergent-additive potential from the metagenomic library of a mangrove soil. Gene 625, 64–71. doi:10.1016/j.gene.2017.04.046
Tavano, O. L., Berenguer-Murcia, A., Secundo, F., and Fernandez-Lafuente, R. (2018). Biotechnological applications of proteases in food technology. Compr. Rev. Food Sci. Food Saf. 17 (2), 412–436. doi:10.1111/1541-4337.12326
Tiwari, R., Nain, L., Labrou, N. E., and Shukla, P. (2018). Bioprospecting of functional cellulases from metagenome for second generation biofuel production: A review. Crit. Rev. Microbiol. 44 (2), 244–257. doi:10.1080/1040841X.2017.1337713
Torsvik, V., ØvreåsTorsvik, L. V., and Ovreas, L. (2002). Microbial diversity and function in soil: From genes to ecosystems. Curr. Opin. Microbiol. 5, 240–245. doi:10.1016/s1369-5274(02)00324-7
Torsvik, V., Goksøyr, J., and Daae, F. (1990). High diversity in DNA of soil bacteria. Appl. Environ. Microbiol. 56, 782–787. doi:10.1128/AEM.56.3.782-787.1990
Tourlousse, D. M., Kurisu, F., Tobino, T., and Furumai, H. (2013). Sensitive and substrate-specific detection of metabolically active microorganisms in natural microbial consortia using community isotope arrays. FEMS Microbiol. Lett. 342 (1), 70–75. doi:10.1111/1574-6968.12112
Tourlousse, D. M., Kurisu, F., Tobino, T., and Furumai, H. (2013). Sensitive and substrate-specific detection of metabolically active microorganisms in natural microbial consortia using community isotope arrays. FEMS Microbiol. Lett. 342(1):70–75. doi:10.1111/1574-6968.12112
Tyson, G. W., Chapman, J., Hugenholtz, P., Allen, E. E., Ram, R. J., Richardson, P. M., et al. (2004). Community structure and metabolism through reconstruction of microbial genomes from the environment. Nature 428 (6978), 37–43. doi:10.1038/nature02340
Uchiyama, T., Abe, T., Ikemura, T., and Watanabe, K. (2005). Substrate-induced gene-expression screening of environmental metagenome libraries for isolation of catabolic genes. Nat. Biotechnol. 23, 88–93. doi:10.1038/nbt1048
Venter, J. C., Remington, K., Heidelberg, J. F., Halpern, A. L., Rusch, D., and Eisen, J. A. (2004). Environmental genome shotgun sequencing of the Sargasso Sea. Science 304 (5667), 66–74. doi:10.1126/science.1093857
Woese, C. R., Stackebrandt, E., Macke, T. J., and Fox, G. E. (1985). A phylogenetic definition of the major eubacterial taxa. Syst. Appl. Microbiol. 6, 143–151. doi:10.1016/s0723-2020(85)80047-3
Yan, W., Li, F., Wang, L., Zhu, Y., Dong, Z., and Bai, L. (2017). Discovery and characterizaton of a novel lipase with transesterification activity from hot spring metagenomic library. Biotechnol. Rep. 14, 27–33. doi:10.1016/j.btre.2016.12.007
Zarafeta, D., Kissas, D., Sayer, C., Gudbergsdottir, S. R., Ladoukakis, E., and Isupov, M. N. (2016). Discovery and characterization of a thermostable and highly halotolerant GH5 cellulase from an Icelandic hot spring isolate. PLoS One 11 (1), e0146454. doi:10.1371/journal.pone.0146454
Keywords: metagenomics, NGS, enzymes, bio-catalyst, microbiome
Citation: Patel T, Chaudhari HG, Prajapati V, Patel S, Mehta V and Soni N (2022) A brief account on enzyme mining using metagenomic approach. Front. Syst. Biol. 2:1046230. doi: 10.3389/fsysb.2022.1046230
Received: 16 September 2022; Accepted: 28 November 2022;
Published: 14 December 2022.
Edited by:
Nikolas Dovrolis, Democritus University of Thrace, GreeceReviewed by:
Ranjith Kumavath, Pondicherry University, Puducherry, IndiaCopyright © 2022 Patel, Chaudhari, Prajapati, Patel, Mehta and Soni. This is an open-access article distributed under the terms of the Creative Commons Attribution License (CC BY). The use, distribution or reproduction in other forums is permitted, provided the original author(s) and the copyright owner(s) are credited and that the original publication in this journal is cited, in accordance with accepted academic practice. No use, distribution or reproduction is permitted which does not comply with these terms.
*Correspondence: Vimalkumar Prajapati, dmltYWxwcmFqYXBhdGlAbmF1Lmlu
†These authors have contributed equally to this work
‡ORCID: Vimalkumar Prajapati, orcid.org/0000-0003-4257-1728