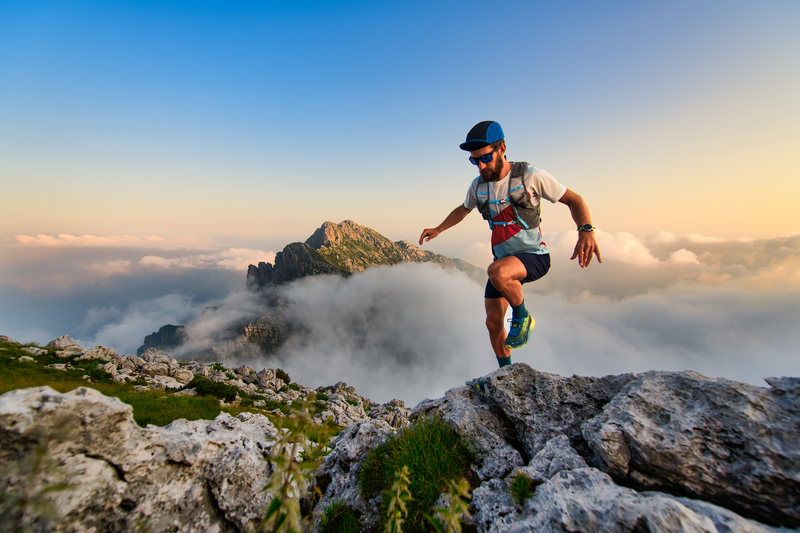
95% of researchers rate our articles as excellent or good
Learn more about the work of our research integrity team to safeguard the quality of each article we publish.
Find out more
ORIGINAL RESEARCH article
Front. Syst. Biol. , 03 December 2021
Sec. Multiscale Mechanistic Modeling
Volume 1 - 2021 | https://doi.org/10.3389/fsysb.2021.764155
In the present work we propose a dynamical mathematical model of the lung cells inflammation process in response to SARS-CoV-2 infection. In this scenario the main protease Nsp5 enhances the inflammatory process, increasing the levels of NF kB, IL-6, Cox2, and PGE2 with respect to a reference state without the virus. In presence of the virus the translation rates of NF kB and IkB arise to a high constant value, and when the translation rate of IL-6 also increases above the threshold value of 7 pg mL−1 s−1 the model predicts a persistent over stimulated immune state with high levels of the cytokine IL-6. Our model shows how such over stimulated immune state becomes autonomous of the signals from other immune cells such as macrophages and lymphocytes, and does not shut down by itself. We also show that in the context of the dynamical model presented here, Dexamethasone or Nimesulide have little effect on such inflammation state of the infected lung cell, and the only form to suppress it is with the inhibition of the activity of the viral protein Nsp5. To that end, our model suggest that drugs like Saquinavir may be useful. In this form, our model suggests that Nsp5 is effectively a central node underlying the severe acute lung inflammation during SARS-CoV-2 infection. The persistent production of IL-6 by lung cells can be one of the causes of the cytokine storm observed in critical patients with COVID19. Nsp5 seems to be the switch to start inflammation, the consequent overproduction of the ACE2 receptor, and an important underlying cause of the most severe cases of COVID19.
Severe Acute Respiratory Syndrome Coronavirus 2 (SARS-CoV-2) virus is an intracellular infectious agent whose replication cycle depends on the host’s cell structures and functions. In particular, it uses the translational apparatus of different types of infected cells to express its proteins (Nakagawa et al., 2016). SARS-CoV-2 causes the Coronavirus Disease 2019 (COVID19) that has infected over 241, 000, 000 persons and killed over 4,900,000 worldwide since the end of 2019. No specific and effective therapeutic drugs to defeat SARS-CoV-2 infection have been developed yet (Díaz, 2020a), although several effective vaccines have been developed and are being applied. Therefore, it is necessary to continue the search for effective therapeutic agents that complement the use of vaccines, to minimize the risk of death from COVID19.
SARS-CoV-2 virion is formed by four proteins: spike (S), envelope (E), membrane (M) and nucleocapside (N) that enclose the virus genome (McBride and Fielding, 2012), which consists of a positive-sense nonsegmented single stranded mRNA [(+)ssRNA] of 30 kb. The open reading frames 1a (orf1a) and 1b (orf1b) are located near the untranslated 5′ region (5′UTR) of the positive single stranded RNA [(+)ssRNA] and they encode for the polyproteins pp1a and pp1ab.
When SARS-CoV-2 virion infects the organism, S protein binds with high affinity to the surface receptor Angiotensin-Converting Enzyme 2 (ACE2), highly abundant in the lung alveolar type II cells and other cell types (Hamming et al., 2004; Gordon et al., 2020), and forms a molecular complex that begins the process of fusion of the virion envelope with the host cell membrane. Finally, viral (+)ssRNA is released into the host cytoplasm (Letko et al., 2020) (Figure 1).
FIGURE 1. Process of infection of lung cells by SARS-CoV-2. Infection begins when the protein S of the virion binds with high affinity to the cells surface receptor ACE2 (Angiotensin-Converting Enzyme 2) that is highly abundant in the lung alveolar type II cells. The formation of the complex S-ACE2 initiates the process of fusion between the virion envelope and the cells membrane leading to the liberation of the nucleocapside with the viral genome into the cytoplasm. SARS-CoV-2 genome consists of a positive-sense nonsegmented single stranded mRNA [(+)ssRNA] of ∼30 kb. The open reading frames 1a (orf1a) and 1b (orf1b) are located near the 5′UTR of the (+)ssRNA and they code for the polyproteins pp1a and pp1ab. Maturation of these polyproteins results in 11 nonstructural proteins (Nsp) from the orf1a segment (Nsp1 to Nsp11) and 5 nonstructural proteins from the orf1b segment (Nsp12 to Nsp16). Nsp proteins form the replication-transcription complex (RTC) a set of nested subgenomic minus-strands of RNA [(−)sgRNA] are synthesized in a process of discontinuous transcription. These (− sgRNAs serve as the templates for the production of subgenomic mRNAs from which the structural proteins E, M, N and S, together with the accessory proteins orf 3a, orf6, orf7a, orf7b, orf8, orf9b, orf9c and orf10 are synthesized. The presence of viral (+)ssRNA initiates the TLR3 and TLR4 mediated immune response, which increases the concentration of free NF κB in cytoplasm. Cytoplasmic NF κB enters the nucleus where promotes the transcription of a series of target genes. Viral protein Nsp5 inhibits the inhibition exerted by HDAC2 on nuclear NF κB transcription factor, enhancing the synthesis of inflammatory cytokines like IL-6 (Figure drawn with Biorender template https://app.biorender.com/).
The process of translation occurs in the cytoplasm and produces a set of viral polyproteins whose maturation results in 11 nonstructural proteins (Nsp) from the orf1a segment (Nsp1 to Nsp11) and 5 nonstructural proteins from the orf1b segment (Nsp12 to Nsp16). Nsp proteins form the replication-transcription complex (RTC) in a double-membrane vesicle where a set of nested subgenomic minus-strands of RNA ((-)sgRNA) are synthesized in a process of discontinuous transcription. These (-) sgRNAs serve as the templates for the production of subgenomic mRNAs from which the structural proteins E, M, N and S, together with the accessory proteins orf 3a, orf6, orf7a, orf7b, orf8, orf9b, orf9c and orf10 are synthesized (Sevajol et al., 2014; Díaz, 2020a; Dongwan et al., 2020). SARS-CoV-2 uses the host translational machinery to redirect it to viral protein synthesis and replication, while host mRNA translation is inhibited (Nakagawa et al., 2016). SARS-CoV and SARS-CoV-2 genomes have ∼79% of homology (Forster et al., 2020), and most of the set of structural and nonstructural proteins are practically the same. However, the virus species differ in the accessory proteins orf8, orf8a, orf 8b, orf9c and orf10 (Bartlam et al., 2005; Gordon et al., 2020).
Viral proteins are inserted into the host molecular machinery to modify and redirect a great number of host cell functions towards the production of additional viral particles (Masters, 2006; Wu et al., 2020). Experimental analysis of the interaction of viral and host proteins, or interactome, by Gordon and collaborators (Gordon et al., 2020) has been a fundamental contribution to understand the form in which SARS-CoV-2 virus takes control of the host molecular network to produce new virions and propagate the infection (Hekman et al., 2020). Gordon and collaborators (2020) cloned, tagged and expressed 26 viral proteins in human cells using affinity-purification mass spectrometry to identify the human proteins physically associated with each other. They found around 332 SARS-CoV-2-human protein-protein interactions that constitute the virus interactome. From these results, the construction of the network representation of the interactome is possible. In the particular case of the Gordon interactome, the analysis of its undirected network model indicates a modular free-scale hierarchical type of structure in which proteins orf8, N and Nsp7 are the main hubs (Díaz, 2020a).
Despite all the molecular knowledge rapidly accumulated on SARS-CoV-2 itself and the infection or inflammation processes in which it is involved in human cells, we still lack dynamical models to understand which are the key nodes underlying severe inflammation processes in some cases. Mathematical and computational dynamic network models are useful tools to integrate data, find experimental holes and also propose or understand the underlying dynamics mechanisms involved in the complex viral/human networks involved during SARS-CoV-2 infection (Breitling, 2010; Díaz, 2020a; Enciso et al., 2020; Weinstein et al., 2020). The number of nodes, the number of connections of each node to its neighbours, and the distribution of these connections in the network determine its complexity, structure and dynamical properties (Kumar et al., 2020).
In this network, the viral main protease Nsp5 is the main protease (Mpro) involved in the assembly of the polyprotein cluster that translates viral RNA, and in the post-translational modification of viral proteins through its ADP ribose phosphatase activity (Nakagawa et al., 2016). SARS-CoV-2 Nsp5 has 96% sequence homology with its SARS-CoV counterpart, and its level of phylogenetic conservation by sequence and structure is high having high homogeneity in its volume and electrostatic profile throughout the Coronaviridae family. Interest in elucidating the functional structure of this protease has resulted in new reports of 3D structures showing that Nsp5 is a complex formed by two equal protomers (A and B) each consisting of 306 residues structured in three domains: I Chymotrypsin-like domain (residues 8–101), Picornavirus 3C protease-like domain II (residues 102–184), and Globular Cluster domain III (residues 201–303). Domains I and II have an antiparallel β-barrel structure and associate with their counterparts to form a dimer, which loop residues 185–200 connect to domain III made up of five α-helixes. The substrate binding site is found in the antiparallel β-barrels located between domains I and II, forming a catalytic pocket composed of four subsites (S1, S2, S3 and S4) that exhibit charged residues that include the nucleophilic sulphur atom of Cys145, which forms a dyad with the imidazole ring of His 41. Therefore, the blocking one of these residues with a small molecule could inhibit the enzymatic activity of Nsp5 (Mukherjee et al., 2011; Pillaiyar et al., 2016; Konwar and Sarma, 2021).
There are several crystallographic structures of Mpro with peptidomimetic inhibitors that show interactions with the enzyme at S3-S4 subsites in SARS-CoV-2, SARS-CoV and MERS. This type of inhibitor incorporates an imidazole ring of cyclic ketoamides with five-components that binds covalently to Cys145 or its equivalent in the different isoforms of the enzyme. Although these types of molecules are not optimal for drug development, due to proteolytic degradation, they have made possible to describe the dynamics of the enzymatic mechanism of this active site for the search and development of molecules with better pharmacodynamic parameters. An example of viable candidates for clinical implementation is boceprevir and telaprevir, whose mechanism of action consists of blocking the catalytic dyad through the covalent binding to the sulphur atom of Cys145 (Pan et al., 2012).
In the SARS-CoV-2 molecular network, Nsp5 has a special role in lung inflammation process through its link with Histone Deacetylase 2 (HDAC2) (Díaz, 2020a; Gordon et al., 2020; Hekman et al., 2020) (Figures 1, 2); suggesting that Nsp5 represses HDAC2 inhibitory action on NF κB (Figure 1), and thus enhances the transcription of the pro-inflammatory genes targeted by the Nuclear Factor κB (NF κB) (Figures 1, 2) (Wagner et al., 2015). Such role distinguishes SARS-CoV and SARS-CoV-2. The SARS-CoV virus uses N protein to promote the sustained transcription of the Cyclooxygenase 2 (Cox2) enzyme by binding directly to the NF-κB transcriptional regulatory elements, and to the CCAAT/enhancer binding proteins of the gene Cox2 (Yan et al., 2006). Hence, SARS-CoV and SARS-CoV-2 produce severe acute respiratory syndrome through different molecular mechanisms.
FIGURE 2. Circuit representation of inflammation. IL-6, NF κB, Cox2, and PGE2 are the basic nodes of the circuit that represents the basic features of inflammation of lung cells during SARS-CoV-2 infection. In this circuit the arrows represent activation and the bars inhibition. In the figure are shown two positive feedback loops between IL-6 and NF κB, and between NF κB and Cox2. IL-6 also induces the expression of the ACE2 receptor in the lung cell membrane. NF κB is inhibited by HDAC2, which in turn is inhibited by the viral protein Nsp5. The circuit has two targets for the anti-inflammatory drugs Nimesulide and Dexamethasone.
The change from a hub (N protein in SARS-CoV) to a poor connected protein (Nsp5 in SARS-CoV-2) as the underlying cause of severe acute respiratory syndrome could be an adaptation that made SARS-CoV-2 more pathogenic (8,098 people worldwide became sick with SARS during the 2003 outbreak against 241, 000, 000 during the 2020–2021 SARS-Cov-2 outbreak). Nsp5 is also the main protease that cleavages the SARS-CoV-2 polyprotein at 11 conserved sites, and its malfunction or deletion could stop the viral replication cycle and lung inflammation (Cohen et al., 2000; Xun et al., 2020). Thus, Nsp5 is a molecular switch that shows a low rate of mutation (Vilar and Isom, 2020), and its malfunctioning can decrease the strength of inflammation and the persistence of ACE2 in the lung epithelial cells giving rise to a less intense inflammatory response (Cohen et al., 2000; Xun et al., 2020).
Activation of the NF κB family of proteins (p65/RelA, p105/p50, p100/p52, RelB, and c-Rel) is mediated by the phosphorylation of the inhibitor of NF κB proteins (IκBs) by IκB kinases (IKKs) and the posterior ubiquitinylation and degradation of the phosphorylated IκBs (Figure 1). The released NF κB proteins enter the nucleus to activate specific genes (Wagner et al., 2015). In the nucleus, HDAC2 blocks the transcriptional activity of NF κB, inhibiting the production and release of the cytokine Interleukin 6 (IL-6) and IκB, and interfering with the functioning of the positive feedback circuit between IL-6 and NF κB (Rahman, and MacNee, 1998) (Figure 3A). As we mentioned before, the sustained inactivation of HDAC2 by Nsp5 produces a sustained increase in the transcriptional activity of NF κB (Figures 1, 2) that leads to a high production of IL-6 in the lung epithelium cells. Release of this excess of IL-6 to the vascular system contributes to the “cytokines storm” observed in critical patients (Leisman et al., 2020; Magro, 2020), although the average serum concentration of IL-6 in critical COVID19 patients are lower with respect to other respiratory syndromes (Leisman et al., 2020). However, the uninterrupted production of IL-6 is closely associated to a persistent presence of the ACE2 receptor in the alveolar type 2 cells due to enhanced ACE2 gene expression mediated by the JAK-STAT and PI3K/Akt pathways (Zegeye et al., 2018; Hennighausen and Lee, 2020; Mokuda et al., 2020) (Figure 2).
FIGURE 3. Feedback loops of the model of inflammation. The mathematical model proposed in this work is based on the dynamical features of three feedback loops: (A) two positive feedback loops between IL-6 and NF κB, and NF κB and IκB; (B) a negative feedback loop between PGE2 and IκB. In Figure A, α (t) is a external signal that represents the amount of IL-6 produced by immune cells like monocytes and lymphocytes, among others.
NF κB also promotes transcription of the Cox2 gene leading to the expression and increased enzymatic activity of Cox2 enzyme (Figures 2, 3B). However, NF κB, Cox2 and IL-6 also form a positive feedback circuit in which NF κB promotes Cox2 enzymatic activity that catalyses the conversion of Arachidonic acid (AA) into Prostaglandin G2 (PGG2), which is in turn modified by the peroxidase moiety of the Cox2 enzyme to produce prostaglandin H2 (PGH2) that is converted to prostaglandin E2 (PGE2) (Alexanian and Sorokin, 2017). PGE2 is then released to the vascular system, binds to its membrane EP2 and EP4 prostanoid receptors and promotes the cleavage of the IκB-NF κB complex increasing the amount of free NF κB, which increases the production of IL-6 (Figure 3B) (Cho et al., 2014; Bouffi et al., 2010). IL-6, in turn, increases NF κB activity (Wang et al., 2003) (Figure 2). These regulatory circuits has three therapeutic targets: IL-6 production (Tocilizumab) (Magro 2020), Cox2 (Nimesulide) (Alexanian and Sorokin, 2017) and NF κB (Dexamethasone) (Newton et al., 1998; Aghai et al., 2006). However, none of these have shown to be really effective for preventing or reverting acute inflammatory cases after SARS-CoV-2 infection.
In this work, we propose a dynamical mathematical model that suggests that the three feedback loops mentioned above constitute a minimal circuit for the inflammatory process in the epithelial lung cells during SARS-CoV-2 infection (Figure 2), and we use an ordinary differential equations (ODEs) continuous model to explore the effect of Nsp5 in the qualitative dynamics of this circuit in which this viral protein acts as an enhancing perturbation in the phase space of this dynamical system (Díaz, 2020b) (Figure 2). For the model of the circuit, we chose as main molecular components the proteins NF κB, IκB, IL-6, Cox2, and PGE2. In this model, Nsp5 prevents the movement of HDAC2 into the nucleus allowing an increase in NF κB transcriptional activity. The input that turns on the circuit in lung cells is a signal from monocytes, lymphocytes, and other immune cells that activates IL-6 production. We propose that this model represents the basic set of interactions that settles on the inflammation reaction to the invasion of the lung cells by SARS-CoV-2, and that it is a first approximation to understand the main dynamical features of this response.
Our hypothesis is that Nsp5 leads to the sustained overproduction of IL-6, Cox2, PGE2, and NF κB, in the epithelial lung cells, which is a necessary but not a sufficient condition for a cytokine storm. In the particular case of epithelial lung cells, we found that the computational and mathematical analysis of the model supports our hypothesis, and that Nsp5 effectively enhances NF κB, IL-6, Cox2 and PGE2 production during the time that remains bounded to HDAC2. Furthermore, the model predicts the existence of an over stimulated immune state (OSIS) in which the amount of IL-6 produced by the infected lung cells is high, and such state becomes autonomous and robust, independent of any stimulus from external sources like monocytes, and lymphocytes. Hence this OSIS cannot be completely shut down by classical anti-inflammatory drugs like Nimesulide and Dexamethasone, and can be possibly beaten only with specific inhibitors of Nsp5 (Xun et al., 2020).
Immune response to infections involves complex spatio-temporal processes involving multiple molecular components, non-linear interactions and cell-cell interactions. In consequence, the immune response to infections is a multidimensional, multiscale and multilevel dynamical process in which innate and acquired immunity mechanisms are involved to protect the organism against pathogens (Eftimie et al., 2016). In this scenario, the number of variables that become both sufficient and necessary for a detailed enough description of the spatio-temporal dynamics of the immune system is tremendously high. Some computational integrative efforts have been made in this direction (Danos et al., 2007), but imply very large systems that cannot be modeled with continuous quantitative models that are, in turn, necessary if we want to understand which are key nodes driving the system towards the OSIS. The lack of quantitative experimental data and knowledge of the values of most of the model parameters are additional challenges to achieve such dynamic models of the immune system in response to specific infectious diseases. Thus, most models of the immune system have been qualitative (Eftimie et al., 2016; Martinez-Sanchez et al., 2018).
A possible approach implies the use of ordinary differential equations (ODEs) qualitative modeling that is oriented to determine all the possible trajectories of a dynamical system along its n-dimensional phase space (with n > 0), when the system is subject to a set of initial conditions and parameters values. High dimensional nonlinear dynamical systems can exhibit a variety of coexisting dynamical behaviors that determine the structure of their phase space (Strogatz, 2015). However, the structure of the phase space of biological nonlinear dynamical systems is highly dependent on the set of parameters values. Variations in the value of one or more parameters can drive a drastic change in phase space generating a different qualitative dynamics of the system. This process is known as bifurcation, and it is important to find the possible bifurcations in the system and to identify which parameter or parameters are the responsible of these changes (Strogatz, 2015) (Supplementary Material S1).
In order to avoid the problem due to the high dimensionality and complexity of the immune response to SARS-CoV-2 infection, and to use the minimum number of parameters in the model that we are proposing in this work, we assume that the interaction between nodes IL-6, NF κB, IκB, Cox2 and PGE2 settles on the main dynamical features of the inflammation process in lung epithelial cells due to the presence of the Nsp5 viral protein. In the proposed circuit shown in Figure 2, IL-6, NF κB, Cox2 and PGE2 define three feedback loops (Figures 3A,B): a positive feedback loop between NF κB and IL-6 (Figure 3A); a positive feedback loop between NF κB and IκB (Figure 3A); and a negative feedback loop between PGE2 and IκB (Figure 3B).
These feedback loops are distributed between three compartments:
1) IL-6 is secreted into the extracellular medium where it acts in paracrine and exocrine form. IL-6 binds to its receptor IL6R at the cell surface. Once the complex IL6-IL6R is formed, it activates IKK in the cytoplasm (Wang et al., 2003). PGE2 is also secreted into this compartment where it binds to EP2 and EP4 receptors at the cell surface, activating Protein Kinase B (Akt) in the cytoplasm (Cho et al., 2014).
2) IKK, free NF κB, and the complex IκB-NF κB are located in the cytoplasm, where IκB is degraded by ubiquitination. Protein Cox2 is located in the endoplasmic reticulum (ER).
3) Free NF κB enters the nucleus where induce the transcription of IL6, Cox2, IKB and NF κB genes. Transcriptional activity of nuclear NF κB is inhibited by HDAC2, and enhanced by Nsp5.
In this form, the model cell consists of three compartments: extracellular medium, cytoplasm and nucleus. We assume that the ratio of the cytoplasmatic volume to external volume (Vcyt/Vout) is one, and that the radio of the cytoplasmatic volume to the nuclear volume (Vcyt/Vnuc) is 2. In each compartment the concentration of the molecules are measured in pg mL−1.
In the circuit of Figure 2, the rate of production of free cytoplasmic NF κB depends on the rate of its release from the IκB-NF κB complex, which is proportional to the product of the concentrations of IκB and IKK (Figure 3A), its own rate of production due to nuclear NF κB transcriptional activity inhibited by HDAC2 (Figures 1, 3A), the rate at which is recaptured by IκB (Figure 3A), and the rate of transport into the nucleus:
where nf is the concentration of free cytoplasmic NF κB,
We assume that the concentration of IKK activated by IL-6 is proportional to the external concentration of the cytokine:
where k1 and k 11 are the new rate constants (Table 1).
The rate at which the complex IκB-NF κB is broken in presence of IKK depends on the rate at which IKK and Akt remove IκB from the complex under the action of IL-6 and PGE2, and the rate at which the complex is formed again:
where iκbnf is the concentration of the complex IκB-NF κB, and k4 and k5 are rate constants (Table 1).
The rate at which NF κB enter the nucleus depends on the rate of transport of the molecule into de nucleus, adjusted for the change in volume between the cytoplasmic and nuclear compartments, and on the rate of inhibition of nuclear NK κB by its inhibitors:
where nf* is the nuclear concentration of NF κB and kdeg is a rate constant.
The probabilities of activation of genes IL6, Cox2, IκB and NF κB (Figures 3A,B) are given by:
Supplementary Material S2 for the mathematical deduction of these equations. For Eqs (5)–(7), the variable
We assume that free cytoplasmic HDAC2 is produced according to the rate equation:
where hd is the amount of free cytoplasmic HDAC2 that is produced at a constant rate r, D2 is the rate of transport of free HDAC2 into the nucleus. In this equation, nsp5 is the amount of Nsp5 in the infected cell that sequester HDAC2 in cytoplasm (El Baba & Herbein, 2020), and η is a constant (Table 1). Free cytoplasmic HDCA2 enters the nucleus according to the equation:
where hd* is the amount of HDAC2 in the nucleus, and kdeg2 is a rate constant (Table 1).
Nsp5 is a protein with only one link in the virus interactome (Gordon et al., 2020; Díaz. 2020a; Hekman et al., 2020), which is an input to the circuit of Figure 2. However, the real kinetic mechanism of production of this viral protein is unknown, thus we assume a plausible simple kinetic mechanism that leads Nsp5 to have a maximum constant concentration of 20 nM. We set the parameters ϕ and k18 to the values shown in Table 1:
where ϕ is the constant rate of production of Nsp5, and k18 is a rate constant (Table 1). The effect of the variation of parameter ϕ on the dynamics of the circuit of Figure 2 is analyzed in Supplementary Material S3.
During the early stage of infection by SARS-CoV-2, the rate of variation of the concentration of IL-6 outside the lung cell depends on the rate of production of IL-6 by monocytes, leucocytes and other cells under stimulation by Tall-like Receptors (TLRs) [denoted by α (t)] (Jafarzadeha et al., 2020; Magro, 2020), on the rate of production by lung cells due to nuclear NF κB transcriptional activity enhanced by Nsp5 (Figure 3A), and on the rate of degradation of IL-6 by different mechanisms. Finally, we assume that the concentration of IKK activated by IL-6 is proportional to the external concentration of the cytokine (Eq. (2)):
where
The rate of variation of the amount of free IκB in the system is the balance between the rate of translation of gene IκB and the rate of formation of new IκB-NF κB complexes:
where
The rate of variation of the amount of the enzyme Cox2 in the system depends on the rate of translation of the Cox2 gene, and its rate of inhibition by different mechanisms:
where cox2 is the amount of the enzyme in the system,
The rate of variation of the amount of PGE2 in the system depends on the amount of Cox2, on the amount of IL-6, and on its rate of degradation:
where k11, k12 and k17 are rate constants (Table 1).
In this section we present in detail and focus on the results that are relevant to understand the role of Nsp5 in the process of acute lung inflammation. The rest of the analyses and results related to the mathematical aspects of the model and its stability analysis are reported in Supplementary Material S3.
We solved the model using the Euler predictor-corrector and Runge-Kutta 4,5 methods with time step of 0.05 s and 720,000 integration steps to simulate a period of 10 h post infection, which is the estimated time for the first bursting of new virions into the extracellular medium (Bar-On et al., 2020; Kumar et al., 2020). We made a Boolean simulation of the circuit to confirm the dynamical behavior predicted by the ODEs model. We used the parameter values shown in Table 1 to generate an arbitrary reference state (ARS) of the system for the initial conditions iκbnf = 5 pg ml−1, and the rest of the variables equal to 0 at time t = 0. In this case, the input α (t) = 10 pg ml−1s−1 and nsp5 = 0 for
Figure 4A shows that the output of the model is the transitory activation of NF κB, Cox2 and PGE2, in contrast with a sustained high activation of IL-6. In order to clarify the source of the steady activation of IL-6, we solved the model with the same initial conditions and α (t) = 0. In this case, the system does not turn on during all the time of simulation (data not shown), indicating that the signal from monocytes and other cells is a necessary condition to start the IL-6 sustained production in lung cells (Figure 4A). Figure 4B shows that when nsp5 ≠ 0, α(t) = 0 and equal gene translation rates, the response of the system is a high sustained level of activation of NF κB, Cox2, and PGE2 with respect to the ARS. In this case, the concentration of IL-6 in the cell was only slightly affected by the virus. Figures from 4A to 4D show the first 5 minutes of simulation during which the circuit of Figure 2 reaches its steady state. This result indicates that the presence of the virus induce a sustained and persistent production of NF κB, Cox2, and PGE2 in the circuit of Figure 2.
FIGURE 4. Effect of Nsp5 on IL-6 production. (A) Arbitrary reference state (ARS) of the circuit of Figure 2. In absence of the viral protein Nsp5, the response to an external input α (t) = 10 pg ml−1s−1 is a transitory activation of NF κB, Cox2, and PGE2. In this case, IL-6 shows a steady high concentration. In this case, all the gene translation rates are set to 2 pg ml−1s−1. (B) In presence of Nsp5 the circuit shows steady higher concentrations of NF κB, Cox2, and PGE2 (red line) with respect to ARS (black line). IL-6 steady concentration (black line) is unaffected by the presence of the viral protein (red line). In this panel, α (t) = 10 pg ml−1s−1, and all the gene translation rates are set to 2 pg ml−1s−1. (C) When NF κB and IκB translation rates are
Translation rates of the set of genes of the model rarely have the same value, even when their activation is promoted by the same transcription factor (NF κB in this case). As shown in Table 1, the results of Figures 4A,B are obtained when the rates of translation are the same for all genes. However, in real cells this is not the case, and Cox2, Il-6, NF κB and IκB are translated at different rates. In Homo sapiens the estimated average rate of translation (
This result indicates that a the difference in translation rates between NF κB and IκB (the intensity of the inhibition of NF κB by IκB, Figure 3A) in absence of the virus with respect to their values in ARS induces a low sustained production of NF κB, Cox2, and PGE2 without affecting IL-6 production. However the presence of Nsp5 induces a high production of NF κB and Cox2, and a moderate increase in PGE2 concentration that decreases IL-6 concentration, indicating that a higher rate of IκB translation with respect to NF κB translation can decrease the concentration of the cytokine IL-6 in presence of the virus probably due to a lower concentration of PGE2in the circuit (Figures 2, 3A).
Figure 4D shows that when α (t) = 10 pg ml−1 s−1, nsp5 = 0,
All these results suggest an important dynamical property of the circuit shown in Figure 2: a high value of the parameter
Figure 5 shows the response of the circuit to a step function defined as:
FIGURE 5. Response of the circuit to a step function. (A) When the external signal α (t) is the step function of Eq. 16 of main text and is applied to the ARS in absence of Nsp5 (black line) the level of cytokine IL-6 initially increases to a maximum value of 20 pg mL−1, and becomes zero when the external signal is switched off. There is not any effect on NF κB, Cox2 and PGE2 concentrations in the circuit. However, in presence of Nsp5 (red line) the levels of NF κB, Cox2, PGE2 and IL-6 are increased in response to α t) and never become zero when the external signal is switched off. (B) When the step function of Eq. 16 is applied to the circuit in absence of Nsp5 (black line) with
The latter simulates an external signal of IL-6 initially generated at a rate of 10 pg ml−1 s−1 during 10 min, and switched off after the 10 min. Figure 5A is the response of the system in ARS to the step signal, in absence and presence of Nsp5. Interestingly, in the absence of Nsp5, the circuit exhibits a low concentration of NF κB, Cox2 and PGE2, and a high concentration of IL-6, all which are zero when the external signal is switched off. In presence of Nsp5 the ARS is perturbed by α (t), and reaches a new steady state with high concentrations of NF κB, Cox2, and PGE2 that remain even when the external signal is switched off. Furthermore, the high IL-6 concentration reached when the circuit is turned on falls to a lower concentration different from zero when α (t) = 0.
In order to reduce the effect of Nsp5 on the circuit of Figure 2, we tested the effect of Nimesulide on NF κB production. Nimesulide, a Cox2 inhibitor, acts inhibiting the synthesis of Cox2 and lipooxigenase enzyme and their products (Suleyman et al., 2008). We modified Eq. 14 to introduce an inhibition term that decreases the rate of production of Cox2 in presence of Nimesulide:
where nim is the amount of Nimesulide at time t, whose rate of variation of its concentration in the cell is:
where r2 = 40 pg ml−1 s−1 and k19 = 3 s−1. Solution of the modified model (Eq. (17)) is presented in Figure 6. In Figure 6A, Nimesulide is applied to the circuit in presence of Nsp5, and with all gene translation rates set to 2 pg ml−1 s−1. In this case, Nimesulide has no effect on NF κB, a slightly effect on IL-6, and a significant decrease in Cox2 and PGE2 concentrations to values near to zero in presence of 14 pg ml−1 of the drug. The same effect is observed when the circuit is in OSIS (Figure 6B). Thus, Nimesulide is not an effective anti-inflammatory agent in the context of the dynamic circuit modeled here, and does not impact the concentration of IL-6 in the presence of Nsp5, even at high concentrations.
FIGURE 6. Effect of Nimesulide on lung cell inflammation. (A) Effect of 14 pg mL−1 of Nimesulide on the levels of NF κB, Cox2, PGE2 and IL-6 when α (t) = 10 pg mL−1s−1, and all gene translation rates are 2 pg mL−1s−1 in presence of Nsp5 (red line). Nimesulide decreases the concentration of Cox2 and PGE2, but has not effect on NF κB and IL-6 (blue line). (B) Effect of 14 pg mL−1 of Nimesulide on the OSIS (red line). Nimesulide significantly decreases the concentration of Cox2 and PGE2, but has not effect on NF κB, The drug has a weak effect on IL-6 concentration (blue line).
Dexamethasone (DX), is a potent synthetic glucocorticoid that inhibits NF κB transcription, and is another anti-inflammatory drug that is being used to ameliorate acute inflammatory states due to SARS-CoV-2 infection. In order to test the effect of DX on the inflammatory process in the circuit of Figure 2, we modified Eq. 8 to introduce an inhibition term that decreases the probability of activation of NF κB:
where DX is the amount of DX at time t, whose rate of variation of its concentration in the cell is:
where r3 = 30 pg ml−1 s−1 and k20 = 3 s−1.
Figure 7 shows the effect of DX on the circuit. Figure 7A shows that when DX is applied to the circuit in presence of Nsp5, and when all genes have the same translation rate of 2 pg ml−1 s−1, the drug eliminates NF κB, Cox2 and PGE2 from the circuit, but has a null effect on the concentration of IL-6. However, when the circuit is in OSIS (Figure 7B), DX has little effect on the immune response in presence of Nsp5. Figure 7C shows that when all translation rates are the same (2 pg ml−1 s−1), DX can shut down the immune response in presence of the virus, when the circuit input α (t) is the function of Eq. 16. However, DX cannot shut down the effects of Nsp5 when the circuit is in OSIS, having a little anti-inflammatory effect (Figure 7D). Hence, our results suggest that DX is an effective anti-inflammatory drug during mild inflammatory states, but is less effective during critical acute lung inflammation due to SARS-CoV-2 (Horby et al., 2021). This dynamical behavior of the circuit of Figure 2 is unaffected by higher doses of DX. When DX and Nimesulide are applied together (with 35 pg ml−1 of DX and 40 pg ml−1 of Nimesulide) to the circuit in OSIS, in which α (t) is the function of Eq. 16, there is also a limited anti-inflammatory effect (Figure 8A).
FIGURE 7. Effect of Dexamethasone on lung cell inflammation. (A) Effect of 14 pg mL−1 of Dexamethasone (DX) on the levels of NF κB, Cox2, PGE2 and IL-6 when a (t) = 10 pg mL−1s−1, and all gene translation rates are 2 pg mL−1s−1 in presence of Nsp5 (red line). DX effectively decreases the concentration of NF κB, Cox2 and PGE2 to cero, but has not effect on IL-6 (black line). (B) Effect of 14 pg mL−1 of DX on the OSIS (red line). DX has a weak effect on the concentration of NF κB, Cox2, PGE2, and IL-6 (red line). (C) When the external signal α (t) is the step function of Eq. 16 of main text and is applied to the circuit in presence of Nsp5 (red line), with all gene translation rates equal to 2 pg mL−1s−1, 14 pg mL−1 of DX decreases the concentration of NF κB, Cox2, PGE2 and IL-6 to zero (black line). (D) When the external signal α (t) is the step function of Eq. 16 of main text and 14 pg mL−1 of DX are applied to the circuit in the OSIS, the drug has a practically null effect on the inflammation process.
FIGURE 8. Effect of the molecular docking of Nsp5 on lung inflammation. (A) When DX and Nimesulide are applied together (with 35 pg mL−1 of DX and 40 pg mL−1 of Nimesulide) to the circuit in OSIS (red line), in which α (t) is the function of Eq. 16, there is also a little anti-inflammatory effect. (B) The molecular docking of Nsp5 completely shuts down the inflammation process when the circuit is in OSIS, with α (t) given by Eq. 16, suggesting that Nsp5 is effectively the cause of the acute lung inflammation during SARS-CoV-2 infection.
In contrast, the predicted limited effect of Nimesulide and DX on reverting the OSIS, the molecular docking of Nsp5 with a hypothetical drug like Saquinavir (Xu et al., 2020), is able to completely eliminate inflammation. We tested this hypothesis modifying Nsp5 production in Eq. (11) with an inhibitory term:
where drug is the amount of the hypothetical drug that can remove Nsp5 from the circuit, whose concentration at time t is given by:
with r4 = 30 pg ml−1 s−1 and k21 = 3 s−1.
Figure 8B shows that when the hypothetical drug is applied to the circuit in OSIS, with α (t) given by Eq. 16, the inflammatory process is completely shut down, suggesting that Nsp5 is effectively the key cause of the acute lung inflammation during SARS-CoV-2 infection, and a fundamental potential target for its treatment.
The complexity of the immune response to infections implies a complex set of different kinds of cells that involve complex regulatory networks and molecules. Together, they underlie specific responses that may lead to resolution or to inflammatory responses whose dynamical properties depend on the networks structure (i.e., the number of nodes, links, and the distribution of links between the nodes) occurring at different scales and levels (Lipniacki et al., 2004; Morel et al., 2006; Eftimie et al., 2016). The structure of such complex and dynamical network is not random due to the specific nature of its nodes and links, so that if the network has a hierarchical structure its hubs determine the overall network dynamics and the structure of its phase space (Cessac, 2010; Eftimie et al., 2016; Díaz. 2020b). In high dimensional phase spaces, steady points of diverse kinds can coexist with isolated closed curves (limit cycles), strange attractors of fractal structure, and other limit sets giving rise to a variety of biological dynamical behaviors like molecular switches, periodic and quasi-periodic oscillations, and bursting (among others). Two central problems in the analysis of this kind of dynamical systems are: 1) the structure of the phase space is strongly dependent of the value of the set of parameters of the dynamical system, and 2) the nonlinearity characteristic of biological systems such as the one treated here (Supplementary Material S1) (Nayak et al., 2018).
In most of the ODEs-based biological models so far studied, there is limited knowledge of the real values of the parameters, and this leads to uncertainty concerning the extent to which the model explains the real process under study. In this case, the validation of the model relied on experimental data. Nonetheless, with the exception of some particular cases (Hodgkin and Huxley, 1952), qualitative models have to be proposed and quantitative validations remain ahead (Eftimie et al., 2016). This challenge increases with the dimensionality of the model. Thus, is necessary to choose some particular properties of the network under study (inflammation network in this case) that incorporate key dynamical features of the whole system under study, and that can also aid to reduce the number of parameters of the model to the minimum possible.
In this sense, we limited the scope of this work to the study of the inflammation process of epithelial lung cells during SARS-CoV-2 infection. The epithelial lung cells form a sub-network that have two highly connected nodes: the internal signal integrator NF κB, and the external signal integrator IL-6 Receptor (IL6R) (Magro, 2020), which is activated by external IL-6 (Figures 2, 3). Both integrators trigger the activation of two important intermediate processes: Cox2 and PGE2 synthesis. Thus, we propose, as a first approximation, that the dynamical behavior of this these four nodes of the circuit of Figure 2 reflect with certain accuracy the dynamical properties of the complex inflammation sub-network of lung cells. Thus, the dynamics of IL-6, NF κB, Cox2 and PGE2 nodes is described by a model that consist of 12 nonlinear coupled ordinary differential equations (Table 1), that we assume represents the central dynamical core involved in the lung cells inflammation process in response to SARS-CoV-2 infection. Such core includes three coupled feedback loops shown in Figure 3. We used this low-dimensionality model to explore the effect of Nsp5 in the qualitative dynamics of the inflammation circuit of Figure 2 (Díaz, 2020b; Kumar et al., 2020).
SARS-CoV-2 main protease Nsp5 has a role as an epigenetic regulator of host DNA expression in lung cells; and thus its physical association with HADC2 (Gordon et al., 2020; Kumar et al., 2020) avoids the movement of the deacetylase from the cytoplasm to the nucleus, increasing the probability of the binding of NF κB to the promoter site of its target genes (Eqs. 5–8) (El Baba and Herbein, 2020). NF κB is a central integrator of the signals that initiate the inflammation process (Figure 1), which include IL-6 secreted by monocytes, leucocytes, macrophages, among other cells, together with signals from TLR4 and TLR7 that suppress the IκB-NF κB complex in a MyD88 independent manner (Vidya et al., 2017). NF κB enhanced transcriptional activity includes the transcription of IL-6, Cox2, IκB and NF κB itself that are key genes in the process of inflammation (Figure 3).
Results obtained from our model suggest that Nsp5 can effectively transform a weak inflammation response (Long et al., 2020) into a persistent sustained one that has a relative high concentration of the cytokine IL-6 (Figure 4B). In the example of Figure 4B, all genes have the same translation rate, a condition that have low probably of occurrence in the real human immune system in which every gene is subject to different processes of post transcriptional regulation (Hausser et al., 2019). When this restriction is removed, and IL-6 has a high translation rate, Nsp5 boosts the concentration of the four main proteins of the circuit with respect to their respective values in ARS, and the inflammation response becomes strongly persistent with a high IL-6 concentration. Furthermore, in this state that we named OSIS, the dynamics of the circuit of Figure 2 becomes independent of any kind of external signal and never turns off, leading to the deregulation of the production of the cytokine IL-6 (Figure 5B). In this form, Nsp5 changes the qualitative dynamical behavior of the system, in which the trajectories that initially span around the ARS stable node now span around the OSIS stable node (Supplementary Material S3) (Díaz, 2020b).
An important biological consequence of this change in the qualitative dynamics of the circuit is that ACE2 receptor synthesis also becomes persistent and autonomous of any external signal to the lung cells (Figure 2). Furthermore, neither Nimesulide nor Dexamethasone or both can completely eliminate the OSIS suggesting that this dynamical behavior could be the form in which the virus assures the persistence of its reproductive cycle without any perturbation from the natural defenses of the body. This could be also a possible clue about the possible adaptive substitution of N protein used in SARS-CoV for the use of the main protease Nsp5 in SARS-CoV-2 as the switch for the inflammation process, which is necessary for ACE2 sustained production, taking into consideration that SARS-CoV N protein targets Cox2 while SARS-CoV-2 Nsp5 protein targets the master integrator NF κB. If this hypothesis is true, this is the probable cause of the increased pathogenicity of SARS-CoV-2 with respect to SARS-CoV.
It is of interest that the persistence of the OSIS is supported by a high translational rate of IL-6, which in the model must have a value of
In this extreme situation (OSIS), the unique treatment possible suggested by our model is an inhibitor of Nsp5 like Saquinavir (Xun et al., 2020). In this case, the OSIS is completely shut down, which indirectly implies the down production of ACE2. Saquinavir is an anti-retroviral drug used against the main protease of HIV-1 (Bensussen et al., 2018; Xun et al., 2020), with some undesirable effects like diarrhea, abdominal pain, and nausea. We suggest that this drug could be a plausible treatment against the effects of SARS-CoV-2 in acute lung inflammation, and as a complement to the new vaccine against this coronavirus.
Interferon (IFN) I and III are key secretory factors in the fight against viral infections in lung cells. During the early response to SARS-CoV-2 infection, interferon pathway is blocked by viral proteins like Nsp14, Nsp16, Nsp15, Orf9b, and Nsp3, among others, that interfere with MAVS and TLRs sensing, STATS 1 and 2 activation and IFN synthesis. Experimental results show that this downregulation of IFN activity correlates with an increased severity of COVID19 symptoms (Kim and Shin, 2021). Therefore, we did not include IFN pathway in our model as a primary cause of lung inflammation. However, our model suggest that the initial downregulation of IFN pathway could be partially counteract by the over activation of NF κB caused by Nsp5. The master regulator NF κB targets IFNs genes (Pfeffer, 2011; Kim and Shin, 2021) probably producing a late sustained production of IFN after the initial viral infection adding a component to the cytokine storm.
In a previous work (Díaz, 2020a) the role of the viral protein Orf8 as a hub of the SARS-CoV-2 infection was suggested. This highly connected protein has as main targets those processes related to the vesicular trafficking required for the ensemble of new virions (Figure 1) (Gordon et al., 2020). Thus, the inhibition of the effects of Orf8 in the host cells could block the reproduction cycle of the virus but not the inflammation process because Nsp5 is not directly linked to Orf8 (Gordon et al., 2020). Rapamycin has been suggested as a drug against the effects of Orf8 but produces severe immunosuppressant effects, which, according to the results of our model, will not be of care in cases of severe lung inflammation because Nsp5 uncouple the circuit of Figure 2 from the process of viral replication (Figure 1 and Figure 5B). In this case, a treatment with Rapamycin and Saquinavir can be an alternative for patients with severe lung inflammation. This suggestion needs experimental verification and clinical evaluation.
In this work we propose a dynamical model that puts forward a novel hypothetical mechanisms underlying lung cells inflammation process in response to SARS-CoV-2. In this scenario the main protease Nsp5 effectively enhances the inflammatory process, increasing the levels of NF κB, IL-6, Cox2, and PGE2 with respect to the reference state. When the translation rates of NF κB and IκB are increased to a high constant value, and the translation rate of IL-6 is increased above the threshold value of 7 pg ml−1s−1 the circuit enters in a persistent over stimulated immune state (OSIS) with high levels of the cytokine IL-6. The OSIS never shuts down by itself, and becomes autonomous of the signals from other immune cells like macrophages and lymphocytes. Our model explains why DX or Nimesulide have little effect on the OSIS, and the only means to suppress such acute and sustained inflammation state is by inhibiting the protein Nsp5 with drugs such as Saquinavir.
In this form, the model suggests, in accordance to our hypothesis, that Nsp5 is effectively a key node underlying severe acute lung inflammation during SARS-CoV-2 infection. The persistent production of IL-6 by lung cells during infection can be one of the causes of the cytokine storm observed in critical patients with COVID19. From an evolutionary point of view, the use of Nsp5 as the switch to start inflammation, and the consequent overproduction of the ACE2 receptor, could have driven the increased pathogenicity of SARS-CoV-2 with respect to SARS-CoV.
In the absence of quantitative data on the kinetics of Nsp5 in the host cell that could give us a better overview of the dynamical behaviour of the protein in the model proposed in this work, we highlight the importance of future in vitro and in vivo experimental research. In order to experimentally validate the predictions of our model is necessary to measure the rate of production of Nsp5 during the infection of the host lung cells, and compare it with the rate of production of other viral proteins with immunomodulatory effects as Orf 7b and Orf 8, to know the real magnitude of its contribution to the inflammatory process. Furthermore, the experimental inhibition of Nsp5 could be of aid to eliminate the inhibition of HDAC2, either catalytic or mimetic, in infected cells to confirm experimentally if the predictions of the model are in concordance to the real mechanisms that contribute to the cytokines storm. The implementation of techniques as plasmid induction or transfection for the unique expression of Nsp5 and its effectors in lung epithelial tissue also promises to be a simple option to validate the proposed mathematical model, and in turn it would be an ideal platform to measure the efficacy of Nsp5 inhibitors proposed by bioinformatics and clinics.
The present theoretical work is part of the project “Dynamics of the SARS-CoV-2 network” and is based on the experimental data reported in the literature at the moment.
The original contributions presented in the study are included in the article/Supplementary Material, further inquiries can be directed to the corresponding authors.
AB made the stability and sensitivity analysis of the model. JD made the model. EA-B and JD conceived the study, discussed the data, and wrote the paper.
JD was supported by the PRODEP funding program of the Universidad Autónoma delEstado deMorelos. AB was supported by a CONACYT Postdoctoral grant Modality 3 of ProNacEs. EA-B was supported by UNAM-PAPIIT IN 211721.
The authors declare that the research was conducted in the absence of any commercial or financial relationships that could be construed as a potential conflict of interest.
All claims expressed in this article are solely those of the authors and do not necessarily represent those of their affiliated organizations, or those of the publisher, the editors and the reviewers. Any product that may be evaluated in this article, or claim that may be made by its manufacturer, is not guaranteed or endorsed by the publisher.
JD thanks Erika Juarez Luna for logistical support. AB, EA-B and JD thanks Dr. Antonio Valcarcel for technical support.
The Supplementary Material for this article can be found online at: https://www.frontiersin.org/articles/10.3389/fsysb.2021.764155/full#supplementary-material
Aghai, Z. H., Kumar, S., Farhath, S., Kumar, M. A., Saslow, J., Nakhla, T., et al. (2006). Dexamethasone Suppresses Expression of Nuclear Factor-kappaB in the Cells of Tracheobronchial Lavage Fluid in Premature Neonates with Respiratory Distress. Pediatr. Res. 59, 811–815. doi:10.1203/01.pdr.0000219120.92049.b3
Alexanian, A., and Sorokin, A. (2017). Cyclooxygenase 2: Protein-Protein Interactions and Posttranslational Modifications. Physiol. Genomics 49, 667–681. doi:10.1152/physiolgenomics.00086.2017
Bai, D., Ueno, L., and Vogt, P. K. (2009). Akt-mediated Regulation of NFκB and the Essentialness of NFκB for the Oncogenicity of PI3K and Akt. Int. J. Cancer 125, 2863–2870. doi:10.1002/ijc.24748
Bar-On, Y. M., Flamholz, A., Phillips, R., and Milo, R. (2020). SARS-CoV-2 (COVID-19) by the Numbers. eLife 9, e57309. doi:10.7554/eLife.57309
Bartlam, M., Yang, H., and Rao, Z. (2005). Structural Insights into SARS Coronavirus Proteins. Curr. Opin. Struct. Biol. 15, 664–672. doi:10.1016/j.sbi.2005.10.004
Bensussen, A., Torres-Sosa, C., Gonzalez, R. A., and Díaz, J. (2018). Dynamics of the Gene Regulatory Network of HIV-1 and the Role of Viral Non-coding RNAs on Latency Reversion. Front. Physiol. 9, 1364. doi:10.3389/fphys.2018.01364
Bouffi, C., Bony, C., Courties, G., Jorgensen, C., and Noël, D. (2010). IL-6-Dependent PGE2 Secretion by Mesenchymal Stem Cells Inhibits Local Inflammation in Experimental Arthritis. PLoS ONE 5, e14247. doi:10.1371/journal.pone.0014247
Cessac, B. (2010). A View of Neural Networks as Dynamical Systems. Int. J. Bifurcation Chaos 20, 1585–1629. doi:10.1142/S0218127410026721
Cho, J.-S., Han, I.-H., Lee, H. R., and Lee, H.-M. (2014). Prostaglandin E2 Induces IL-6 and IL-8 Production by the EP Receptors/Akt/NF-Κb Pathways in Nasal Polyp-Derived FibroblastsAllergy Asthma. Allergy Asthma Immunol. Res. 6, 449–457. doi:10.4168/aair.2014.6.5.449
Cohen, R., Erez, K., ben-Avraham, D., and Havlin, S. (2000). Resilience of the Internet to Random Breakdowns. Phys. Rev. Lett. 85, 4626–4628. doi:10.1103/PhysRevLett.85.4626
Danos, V., Feret, J., Fontana, W., Harmer, R., and Krivine, J. (2007). “Rule-based Modelling of Cellular Signalling,” in CONCUR 2007—concurrency Theory (Berlin: Springer), 17–41. doi:10.1007/978-3-540-74407-8_3
Díaz, J. (2020a). SARS-CoV-2 Molecular Network Structure. Front. Physiol. 11, 870. doi:10.3389/fphys.2020.00870
Eftimie, R., Gillard, J. J., and Cantrell, D. A. (2016). Mathematical Models for Immunology: Current State of the Art and Future Research Directions. Bull. Math. Biol. 78, 2091–2134. doi:10.1007/s11538-016-0214-9
El Baba, R., and Herbein, G. (2020). Management of Epigenomic Networks Entailed in Coronavirus Infections and COVID-19. Clin. Epigenet 12, 118. doi:10.1186/s13148-020-00912-7
Enciso, J., Mendoza, L., Álvarez-Buylla, E. R., and Pelayo, R. (2020). Dynamical Modeling Predicts an Inflammation-Inducible CXCR7+ B Cell Precursor with Potential Implications in Lymphoid Blockage Pathologies. PeerJ 8, e9902. doi:10.7717/peerj.9902
Forster, P., Forster, L., Renfrew, C., and Forster, M. (2020). Phylogenetic Network Analysis of SARS-CoV-2 Genomes. Proc. Natl. Acad. Sci. USA 117, 9241–9243. doi:10.1073/pnas.2004999117
Gordon, D. E., Jang, G. M., Bouhaddou, M., Xu, J., Obernier, K., White, K. M., et al. (2020). A SARS-CoV-2 Protein Interaction Map Reveals Targets for Drug Repurposing. Nature 583, 459–468. doi:10.1038/s41586-020-2286-9
Hamming, I., Timens, W., Bulthuis, M., Lely, A., Navis, G., and van Goor, H. (2004). Tissue Distribution of ACE2 Protein, the Functional Receptor for SARS Coronavirus. A First Step in Understanding SARS Pathogenesis. J. Pathol. 203, 631–637. doi:10.1002/path.1570
Hausser, J., Mayo, A., Keren, L., and Alon, U. (2019). Central Dogma Rates and the Trade-Off between Precision and Economy in Gene Expression. Nat. Commun. 10, 68. doi:10.1038/s41467-018-07391-8
Hekman, R. M., Hume, A. J., Goel, R. K., Abo, K. M., Huang, J., Blum, B. C., et al. (2020). Actionable Cytopathogenic Host Responses of Human Alveolar Type 2 Cells to SARS-CoV-2. Mol. Cel 80, 1104–1122. doi:10.1016/j.molcel.2020.11.028
Hennighausen, L., and Lee, H. K. (2020). Activation of the SARS-CoV-2 Receptor Ace2 through JAK/STAT-Dependent Enhancers during PregnancyAce2 through JAK/STAT-Dependent Enhancers during Pregnancy. Cel Rep. 32, 108199. doi:10.1016/j.celrep.2020.108199
Hodgkin, A. L., and Huxley, A. F. (1952). A Quantitative Description of Membrane Current and its Application to Conduction and Excitation in Nerve. J. Physiol. 117, 500–544. doi:10.1113/jphysiol.1952.sp004764
Horby, P., Lim, W. S., Emberson, J. R., Mafham, M., Bell, J. L., Linsell, L., et al. (2021). Dexamethasone in Hospitalized Patients with Covid-19. N. Engl. J. Med. 384, 693–704. doi:10.1056/NEJMoa2021436
Jafarzadeh, A., Chauhan, P., Saha, B., Jafarzadeh, S., and Nemati, M. (2020). Contribution of Monocytes and Macrophages to the Local Tissue Inflammation and Cytokine Storm in COVID-19: Lessons from SARS and MERS, and Potential Therapeutic Interventions. Life Sci. 257, 118102. doi:10.1016/j.lfs.2020.118102
Kim, D., Lee, J.-Y., Yang, J.-S., Kim, J. W., Kim, V. N., and Chang, H. (2020). The Architecture of SARS-CoV-2 Transcriptome. Cell 181, 914–921. doi:10.1016/j.cell.2020.04.011
Kim, Y.-M., and Shin, E.-C. (2021). Type I and III Interferon Responses in SARS-CoV-2 Infection. Exp. Mol. Med. 53, 750–760. doi:10.1038/s12276-021-00592-0
Konwar, M., and Sarma, D. (2021). Advances in Developing Small Molecule SARS 3CLpro Inhibitors as Potential Remedy for corona Virus Infection. Tetrahedron 77, 131761. doi:10.1016/j.tet.2020.131761
Kumar, N., Mishra, B., Mehmood, A., Mohammad Athar, M., and M Shahid Mukhtar, M. S. (2020). Integrative Network Biology Framework Elucidates Molecular Mechanisms of SARS-CoV-2 Pathogenesis. iScience 23, 101526. doi:10.1016/j.isci.2020.101526
Leisman, D. E., Ronner, L., Pinotti, R., Taylor, M. D., Sinha, P., Calfee, C. S., et al. (2020). Cytokine Elevation in Severe and Critical COVID-19: a Rapid Systematic Review, Meta-Analysis, and Comparison with Other Inflammatory Syndromes. Lancet Respir. Med. 8, 1233–1244. doi:10.1016/s2213-2600(20)30404-5
Letko, M., Marzi, A., and Munster, V. (2020). Functional Assessment of Cell Entry and Receptor Usage for SARS-CoV-2 and Other Lineage B Betacoronaviruses. Nat. Microbiol. 5, 562–569. doi:10.1038/s41564-020-0688-y
Lipniacki, T., Paszek, P., Brasier, A. R., Luxon, B., and Kimmel, M. (2004). Mathematical Model of NF-Κb Regulatory Module. J. Theor. Biol. 228, 195–215. doi:10.1016/j.jtbi.2004.01.001
Long, Q.-X., Tang, X.-J., Shi, Q.-L., Li, Q., Deng, H.-J., Yuan, J., et al. (2020). Clinical and Immunological Assessment of Asymptomatic SARS-CoV-2 Infections. Nat. Med. 26, 1200–1204. doi:10.1038/s41591-020-0965-6
Magro, G. (2020). SARS-CoV-2 and COVID-19: Is Interleukin-6 (IL-6) the 'culprit Lesion' of ARDS Onset? what Is There besides Tocilizumab? SGP130Fc. Cytokine: X 2, 100029. doi:10.1016/j.cytox.2020.100029
Martinez-Sanchez, M. E., Huerta, L., Alvarez-Buylla, E. R., and Villarreal Luján, C. (2018). Role of Cytokine Combinations on CD4+ T Cell Differentiation, Partial Polarization, and Plasticity: Continuous Network Modeling Approach. Front. Physiol. 9, 877. doi:10.3389/fphys.2018.00877
Masters, P. S. (2006). The Molecular Biology of Coronaviruses. Adv. Virus. Res. 66, 193–292. doi:10.1016/S0065-3527(06)66005-3
McBride, R., and Fielding, B. (2012). The Role of Severe Acute Respiratory Syndrome (SARS)-Coronavirus Accessory Proteins in Virus Pathogenesis. Viruses 4, 2902–2923. doi:10.3390/v4112902
Messina, F., Giombini, E., Agrati, C., Vairo, F., Ascoli Bartoli, T., Al Moghazi, S., et al. (2020). COVID-19: Viral-Host Interactome Analyzed by Network Based-Approach Model to Study Pathogenesis of SARS-CoV-2 Infection. J. Transl Med. 18, 233. doi:10.1186/s12967-020-02405-w
Mokuda, S., Tokunaga, T., Masumoto, J., and Sugiyama, E. (2020). Angiotensin-converting Enzyme 2, a SARS-CoV-2 Receptor, Is Upregulated by Interleukin 6 through STAT3 Signaling in Synovial Tissues. J. Rheumatol. 47, 1593–1595. doi:10.3899/jrheum.200547
Morel, P. A., Ta'asan, S., Morel, B. F., Kirschner, D. E., and Flynn, J. L. (2006). New Insights into Mathematical Modeling of the Immune System. Ir 36, 157–166. doi:10.1385/IR:36:1:157
Mukherjee, P., Shah, F., Desai, P., and Avery, M. (2011). Inhibitors of SARS-3CLpro: Virtual Screening, Biological Evaluation, and Molecular Dynamics Simulation Studies. J. Chem. Inf. Model. 51, 1376–1392. doi:10.1021/ci1004916
Nakagawa, K., Lokugamage, K. G., and Makino, S. (2016). Viral and Cellular mRNA Translation in Coronavirus-Infected Cells. Adv. Virus. Res. 96, 165–192. Elsevier Inc. ISSN 0065-3527. doi:10.1016/bs.aivir.2016.08.001
Nayak, S. K., Bit, A., Dey, A., Mohapatra, B., and Pal, K. (2018). A Review on the Nonlinear Dynamical System Analysis of Electrocardiogram Signal. J. Healthc. Eng. 2018, 1–19. Article ID 6920420. doi:10.1155/2018/6920420
Newton, R., Seybold, J., Kuitert, L. M., Bergmann, M., and Barnes, P. J. (1998). Repression of Cyclooxygenase-2 and Prostaglandin E2Release by Dexamethasone Occurs by Transcriptional and Post-transcriptional Mechanisms Involving Loss of Polyadenylated mRNA. J. Biol. Chem. 273, 32312–32321. doi:10.1074/jbc.273.48.32312
Pan, Q., Peppelenbosch, M. P., Janssen, H. L., and de Knegt, R. J. (2012). Telaprevir/boceprevir Era: from Bench to Bed and Back. Wjg 18, 6183–6188. doi:10.3748/wjg.v18.i43.6183
Pfeffer, L. M. (2011). The Role of Nuclear Factor κB in the Interferon Response. J. Interferon Cytokine Res. 31, 553–559. doi:10.1089/jir.2011.0028
Pillaiyar, T., Manickam, M., Namasivayam, V., Hayashi, Y., and Jung, S.-H. (2016). An Overview of Severe Acute Respiratory Syndrome-Coronavirus (SARS-CoV) 3CL Protease Inhibitors: Peptidomimetics and Small Molecule Chemotherapy. J. Med. Chem. 59, 6595–6628. doi:10.1021/acs.jmedchem.5b01461
Rahman, I., and MacNee, W. (1998). Role of Transcription Factors in Inflammatory Lung Diseases. Thorax 53, 601–612. doi:10.1136/thx.53.7.601
Sevajol, M., Subissi, L., Decroly, E., Canard, B., and Imbert, I. (2014). Insights into RNA Synthesis, Capping, and Proofreading Mechanisms of SARS-Coronavirus. Virus. Res. 194, 90–99. doi:10.1016/j.virusres.2014.10.008
Strogatz, S. H. (2015). Chemistry and Engineering. 2nd Edition. United States: CRC Press. ISBN-13 : 978-0813349107.
Suleyman, H., Cadirci, E., Albayrak, A., and Halici, Z. (2008). Nimesulide Is a Selective COX-2 Inhibitory, Atypical Non-steroidal Anti-inflammatory Drug. Cmc 15, 278–283. doi:10.2174/092986708783497247
Vidya, M. K., Kumar, V. G., Sejian, V., Bagath, M., Krishnan, G., and Bhatta, R. (2017). Toll-like Receptors: Significance, Ligands, Signaling Pathways, and Functions in Mammals. Int. Rev. Immunol. 37, 20–36. doi:10.1080/08830185.2017.1380200
Vilar, S., and Isom, D. G. (2020). One Year of SARS-CoV-2: How Much Has the Virus Changed? Biology 10, 91. doi:10.3390/biology10020091
Wang, L., Walia, B., Evans, J., Gewirtz, A. T., Merlin, D., and Sitaraman, S. V. (2003). IL-6 Induces NF-Κb Activation in the Intestinal Epithelia. J. Immunol. 171, 3194–3201. doi:10.4049/jimmunol.171.6.3194
Wagner, T., Kiweler, N., and Wolff, K. (2015). Sumoylation of HDAC2 promotes NF-κB-dependent gene expression. Oncotarget 6, 7123–7135. doi:10.18632/oncotarget.3344
Weinstein, N., Mendoza, L., and Álvarez-Buylla, E. R. (2020). A Computational Model of the Endothelial to Mesenchymal Transition. Front. Genet. 11, 40. doi:10.3389/fgene.2020.00040
Wu, A., Peng, Y., Huang, B., Ding, X., Wang, X., Niu, P., et al. (2020). Genome Composition and Divergence of the Novel Coronavirus (2019-nCoV) Originating in China. Cell Host & Microbe 27, 325–328. doi:10.1016/j.chom.2020.02.001
Xu, C., Ke, Z., Liu, C., Wang, Z., Liu, D., Zhang, L., et al. (2020). Systemic In Silico Screening in Drug Discovery for Coronavirus Disease (COVID-19) with an Online Interactive Web Server. J. Chem. Inf. Model. 60, 5735–5745. doi:10.1021/acs.jcim.0c00821
Yan, X., Hao, Q., Mu, Y., Timani, K. A., Ye, L., Zhu, Y., et al. (2006). Nucleocapsid Protein of SARS-CoV Activates the Expression of Cyclooxygenase-2 by Binding Directly to Regulatory Elements for Nuclear Factor-Kappa B and CCAAT/enhancer Binding Protein. Int. J. Biochem. Cel Biol. 38, 1417–1428. doi:10.1016/j.biocel.2006.02.003
Zegeye, M. M., Lindkvist, M., Fälker, K., Kumawat, A. K., Paramel, G., Grenegård, M., et al. (2018). Activation of the JAK/STAT3 and PI3K/AKT Pathways Are Crucial for IL-6 Trans-signaling-mediated Pro-inflammatory Response in Human Vascular Endothelial Cells. Cell Commun Signal 16, 55. doi:10.1186/s12964-018-0268-4
Keywords: SARS-CoV-2 infection, interleukin 6, NFKB, NSP5, COX2, SARS-CoV-2 interactome, nonlinear dynamics of inflammation
Citation: Bensussen A, Álvarez-Buylla ER and Díaz J (2021) SARS-CoV-2 Nsp5 Protein Causes Acute Lung Inflammation, A Dynamical Mathematical Model. Front. Syst. Biol. 1:764155. doi: 10.3389/fsysb.2021.764155
Received: 25 August 2021; Accepted: 11 November 2021;
Published: 03 December 2021.
Edited by:
Francesco Pappalardo, University of Catania, ItalyReviewed by:
Paola Lecca, Free University of Bozen-Bolzano, ItalyCopyright © 2021 Bensussen, Álvarez-Buylla and Díaz. This is an open-access article distributed under the terms of the Creative Commons Attribution License (CC BY). The use, distribution or reproduction in other forums is permitted, provided the original author(s) and the copyright owner(s) are credited and that the original publication in this journal is cited, in accordance with accepted academic practice. No use, distribution or reproduction is permitted which does not comply with these terms.
*Correspondence: José Díaz, YmlvZmlzaWNhQHlhaG9vLmNvbQ==; Elena R. Álvarez Buylla, ZWxlbmFidXlsbGFAcHJvdG9ubWFpbC5jb20=
Disclaimer: All claims expressed in this article are solely those of the authors and do not necessarily represent those of their affiliated organizations, or those of the publisher, the editors and the reviewers. Any product that may be evaluated in this article or claim that may be made by its manufacturer is not guaranteed or endorsed by the publisher.
Research integrity at Frontiers
Learn more about the work of our research integrity team to safeguard the quality of each article we publish.