- Department of Orthopedics and Traumatology, Adana Turgut Noyan Research and Training Centre, Başkent University, Adana, Türkiye
Background: This bibliometric study aimed to identify and analyze the top 100 articles related to artificial intelligence in the field of orthopedics.
Methods: The articles were assessed based on their number of citations, publication years, countries, journals, authors, affiliations, and funding agencies. Additionally, they were analyzed in terms of their themes and objectives. Keyword co-occurrence, co-citation of authors, and co-citation of references analyses were conducted using VOSviewer (version 1.6.19).
Results: The number of citations of these articles ranged from 32 to 272, with six papers having more than 200 citations The years of 2019 (n: 37) and 2020 (n: 19) together constituted 56% of the list. The USA was the leading contributor country to this field (n: 61). The most frequently used keywords were “machine learning” (n: 26), “classification” (n: 18), “deep learning” (n: 16), “artificial intelligence” (n: 14), respectively. The most common themes were decision support (n: 25), fracture detection (n: 24), and osteoarthrtitis staging (n: 21). The majority of the studies were diagnostic in nature (n: 85), with only two articles focused on treatment.
Conclusions: This study provides valuable insights and presents the historical perspective of scientific development on artificial intelligence in the field of orthopedics. The literature in this field is expanding rapidly. Currently, research is generally done for diagnostic purposes and predominantly focused on decision support systems, fracture detection, and osteoarthritis classification.
Introduction
The concept of artificial intelligence (AI) was initially proposed by McCarthy, a cognitive and computer scientist, in 1956 (1). Since then, as an innovative technology, the application of artificial intelligence in various fields, including medicine and orthopedics, has garnered significant attention. In the near future, the impact of artificial intelligence applications could lead to a significant transformation in the practice of orthopedics (2). Artificial intelligence in orthopedics can yield benefits in areas such as preoperative diagnosis, patient selection, outcome and complication prediction, data tracking optimization, and the intraoperative surgical performance (2, 3).
Robot-assisted surgeries, navigated or computer-assisted surgeries in orthopedics are being applied in many places and their areas of use are expanding (4, 5). In addition to this, semi-automated or fully automated preoperative planning and templating systems are also available (6). It is difficult not to foresee an increase in AI usage in orthopedics over time, similar to its expansion in other fields. The importance of data has increased during this period, and the data obtained preoperatively, intraoperatively, and postoperatively has grown. Evaluating such a large amount of data has become difficult for humans, and the presence of AI-based systems is necessary in a much shorter timeframe for processing this data which is called big data. These systems save time for orthopedists and increase the accuracy rate in diagnosis and treatment, thereby enhancing the surgeon's chances of success. However, in order for orthopedists to be able to delegate this to AI-enhanced systems, they must first have basic knowledge on this subject. In this rapidly evolving field, mastering knowledge has also become more challenging. Therefore, gaining access to the most impactful and essential articles in this field has become crucial.
The increasing number of publications in the field of artificial intelligence-related orthopedics has made it challenging to grasp the subject and the literature comprehensively. Consequently, students, orthopedic residents, expert physicians, and engineers engaged in this field feel the need to delve into the most significant works primarily. Citation analysis is one of the most critical parameters illustrating a study's significance and influence within the academic community. Citation analyses are brought to light through bibliometric studies. Bibliometric analysis involves the computer-aided investigation of literature published in a specific topic or field using an appropriate methodology. Through these analyses, aspects such as the impact, content, citations received, authors, and finer details of articles are meticulously examined. The number of citations an article receives is a crucial measure of its influence.
Similar bibliometric studies have been conducted in orthopedics and other medical fields (7–9). However, as far as we know, this is the first bibliometric analysis focusing on the highest-impact articles within the field of orthopedics, those related to artificial intelligence. In this study, our objective was to identify and conduct a bibliometric analysis of the top 100 articles with the highest number of citations in the field of orthopedics, specifically those related to artificial intelligence. Our hypothesis was that due to the accelerated growth of studies related to artificial intelligence, newer articles might find a more prominent place in the list.
Materials and methods
Ethical committee approval was not sought for this study, as it was a bibliometric study and involving no human subjects. The data were collected using the Thomson ISI Web of Science Core Collection (WOSCC). The reason for using Web of Science in this article to obtain the data is its greater consistency and reliability than other databases, making it the primary choice for similar bibliometric studies in the literature (9, 10). All relevant articles were searched through the search engine. The search scope was set to “all fields” to minimize the risk of overlooking potential articles. The search query consisted of two parts. In the first row (#1), the queried keywords were “artificial intelligence” OR “neural network” OR “deep learning” OR “machine learning” OR “data mining” OR “big data” (All fields search). In the second row (#2), the queried keywords were “orthopaedics” OR “orthopaedic” OR “orthopedics” OR “orthopedic” OR “sports medicine” OR “fracture” OR “trauma” OR “osteoarthritis” OR “bone cancer” OR “arthroplasty” OR “joint replacement” (All fields search). The final dataset was #1 AND #2. Articles indexed in the Science Citation Index Expanded (SCI-Expanded) or Emerging Sources Citation Index (ESCI) (Web of Science Index) were included. No language restriction was applied. The search was conducted on August 17, 2023, within a single day, as a precaution against potential updates of the literature. No restrictions were applied regarding the publication date of the articles, resulting in a total of 5,561 records. The articles were ranked based on the number of citations from the most to the least (Figure 1). For sensitivity in the research, two independent researchers (NT, SD) evaluated the titles, abstracts and full texts of the articles to be included. The articles were screened to ensure their relevance to the field of orthopedics. Upon evaluation of the articles obtained from the search, if an article was deemed to be an artificial intelligence study unrelated to the field of orthopedics, it was excluded from the study. The top 100 most cited articles were identified and exported in plain text format for further analysis. Result analyses were performed in Web of Science. These articles were assessed in terms of authors, citations, publication year, country/region, area of orthopedics, institutions, journals, the annual number of published articles and funding agencies. The impact factor values of the journals were obtained from the 2022 Journal Citation Reports (JCR). The country of the first author was considered as the country of origin for each respective article. VOSviewer (version 1.6.19) was used for the advanced bibliometric analysis.
Data analysis
VOSviewer is a bibliometric tool that facilitates quantitative analysis of the literature. This tool enables the generation of co-occurrence, co-citation, and co-authorship maps for the defined dataset. Keywords were assessed using co-occurrence analysis. Keyword co-occurrence analysis examines how many times a pair of keywords are mentioned within the same article. For citation analysis, references, cited authors, and journals were selected. If a third article references two other articles in its reference list, then these two referenced articles have a co-citation relationship with each other. Authors and institutions were evaluated through cooperative network analysis.
Results
In this study, we compiled the top 100 most cited articles in the field of orthopedics related to artificial intelligence (AI). The articles were presented in descending order based on their citation number (Table 1). It was observed that the number of citations of these articles ranged from 32 to 272, with six papers having more than 200 citations. The article titled “Deep-learning-assisted diagnosis for knee magnetic resonance imaging: Development and retrospective validation of MRNet” by Bien et al. was the most cited and published in PLOS Medicine journal, with an annual citation rate of 55.4 (11). The average citation count for the articles in the Top 100 list was determined to be 72.35.
The articles within the Top 100 list were published between 1995 and 2022, spanning over a 27-year period (Figure 2). When examined by decades, there were 3 articles from the 1990s, 2 from the 2000s, 73 from the 2010s, and 22 from the 2020s. The most highly cited articles were predominantly published in 2019 (n: 37) and 2020 (n: 19), comprising 56% of the Top 100 list. Even though they have been recently published, two articles from 2021 and one from 2022 were able to be included in this list. The oldest paper was published in 1995 with a title of “Injury severity and probability of survival assessment in trauma patients using a predictive hierarchical network model derived from ICD-9 codes” in Journal of Trauma-Injury Infection and Critical Care (12). Robert Rutledge used neural networks for computer-assisted decision-making in this study.
The articles originated from 28 different countries, with the USA (n: 61) contributing significantly to the literature in terms of publication volume (Table 2). England (n: 12) was the second, followed by others including Germany (n: 7), Netherlands (n: 6), Canada (n: 5), and France (n: 5).
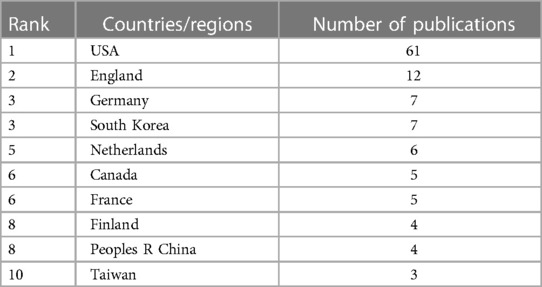
Table 2. Ranking of the countries based on the number of publications (the countries with ≥ 3 publications.
These articles were published in 55 different journals. “The Journal of Arthroplasty”, which had an impact factor of 3.5 in 2022, was the most published journal title with ten articles, while “Radiology Artificial Intelligence” had six articles, “Radiology” had five, and “Clinical Orthopaedics And Related Research,” “PLOS ONE,” and “Scientific Reports” had four each. The journals with two or more articles and their respective impact factors are presented in Table 3. The journal with the biggest impact factor was “Radiology”.
A total of 608 authors contributed to the Top 100 list. Among the high-yield authors with the most cited articles, four individuals led the list with contributions to six articles each: Haeberle HS, Majumdar S, Pedoia V, and Ramkumar PN (Table 4). All four authors were affiliated with institutions in the USA. Both Haeberle Hs and Ramkumar PN have received a total of 422 citations. A total of 250 different institutions contributed to the Top 100 list. The institutions with highest number of publications were University of California System (n: 13), followed by University of California San Francisco (n: 10), Harvard University (n: 9), Stanford University (n: 9), Baylor College of Medicine (n: 8), and Cleveland Clinic Foundation (n: 7).
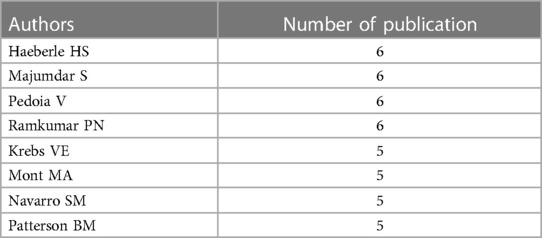
Table 4. Ranking of the authors based on the number of publications (the authors with ≥5 publications).
The majority of articles, 96%, had three or more authors. Two articles were single-authored (Bini, Stefano A.; Rutledge, R), and two were co-authored by two individuals. Funding agencies were acknowledged in 55 articles. The most prominent contributors included the United States Department Of Health Human Services (n: 18), National Institutes Of Health NIH USA (n: 17), GlaxoSmithKline (n: 7), Merck Company (n: 7), Novartis (n: 7), and Pfizer (n: 7). Sixty articles were categorized as “all open access.”
Among the articles, 94 were found in SCI-expanded journals (n: 94), while 6 were in ESCI journals. Of the total, 97 articles were categorized as original studies, with only 3 articles identified as reviews. Only five of the studies were classified as clinical studies which involved people. The ninety-seven original articles were categorized according to the themes of the researches (Table 5). Decision support systems were used in a quarter of the list (n: 25). Fracture detection (n: 24) was the second most researched subject. They were followed by osteoarthritis and its staging (n: 21). The other themes were intraarticular joint evaluation (n: 8), osteoporosis (n: 6), gait analysis (n: 3), biomaterials (2), implant identification (n: 2), determination of bone age (n: 1), surgery which was vertebroplasty (n: 1), detection of spinal disorders and deformities (n: 1), trauma scoring systems (n: 1), education (n: 1), remote monitoring after arthroplasty (n: 1).
The studies were predominantly conducted for diagnostic purposes (n: 85), with only one study conducted for therapeutic purposes (Table 6). Fracture diagnosis studies involved the use of CT in only two articles, with the remaining 23 articles relying on x-ray images. All eight articles, which investigate intraarticular structure pathologies, used MRI scanning. In four of the osteoarthritis diagnosis and staging studies, MRI imaging was used, while x-ray images were employed in the remaining 17 research studies.
Regarding the Top 100 list, it was observed that only 38 articles were published in the WOS orthopedics category, while the majority were published in journals from other fields. The most closely related fields on this topic were radiology, nuclear medicine, surgery, and computer science.
Bibliometric co-occurrence analysis
An analysis was conducted on the 497 keywords obtained from the studies in this list. The minimum number of occurrences of a keyword was set at two. 102 keywords met the criteria. The keywords were analyzed and visualized by VOSviewer software (Figure 3). Each keyword is represented by a circle in the figure. The keywords that co-occur more frequently are displayed with larger circles. The most frequently used keywords were “machine learning” (n: 26), “classification” (n: 18), “deep learning” (n: 16), “artificial intelligence” (n: 14), respectively. The other keywords with the highest occurrences comprising the top ten were “osteoarthritis”, “risk factors”, “prediction”, “arthroplasty”, “artificial-intelligence”, and “convolutional neural network”, respectively (Table 7).
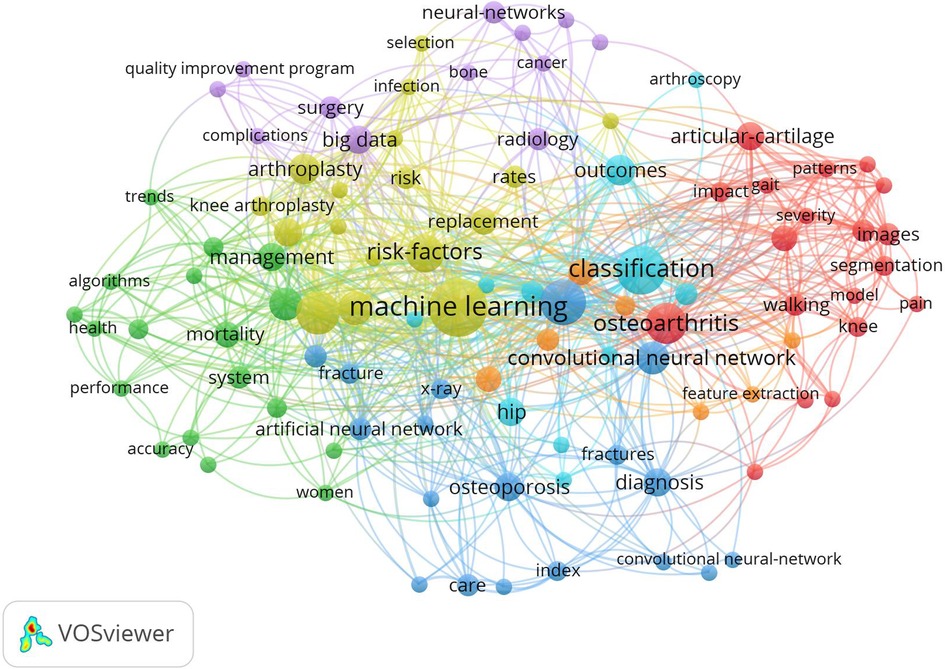
Figure 3. Mapping on co-occurrence of keywords related to orthopedics and traumatology with artificial intelligence. Each point represented in different colors and sizes represents a keyword. An increase in the size of a point signifies a higher frequency of usage for that keyword. The line connecting two points indicates that both keywords appeared in the same article. The figure was created using VOSviewer (version 1.6.19) software.
Bibliometric co-citation analysis
Co-citations of authors were analyzed. The mapping is shown in Figure 4. When the link between two authors is stronger, they appear closer to each other, and the line between them is thicker. If there is a line between two authors, it signifies that they are referenced in the same article. The minimum number of citations of an author was set at three, and 222 authors met the threshold of the 2,309 authors. Tiulpin, A had the most citations of 20 and total link strength of 350.
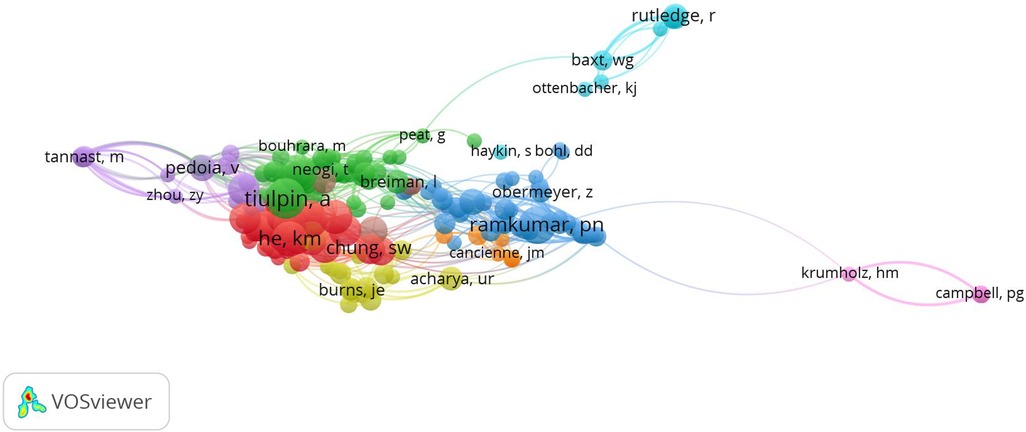
Figure 4. Mapping on co-cited authors of publications related to orthopedics and traumatology with artificial intelligence. The varying colors represent different authors who were cited. An increase in the size of a point signifies a higher citation frequency of the author. The line connecting two points indicates that both authors had been cited in the same article. The length of a line indicates the proximity between two authors; the closer the link, the shorter the line. The figure was created using VOSviewer (version 1.6.19) software.
Co-citations of references are beneficial for assessing the presence of similarity by determining the number of articles in which these references are collectively cited. The minimum number of citations of a cited article was set at three, and 112 met the threshold of the 2,874 cited references. The article “Radiological assessment of osteo-arthrosis” by JH Kellgren and JS Lawrence had the most citations of 15 (13). The visual analysis is shown in Figure 5.
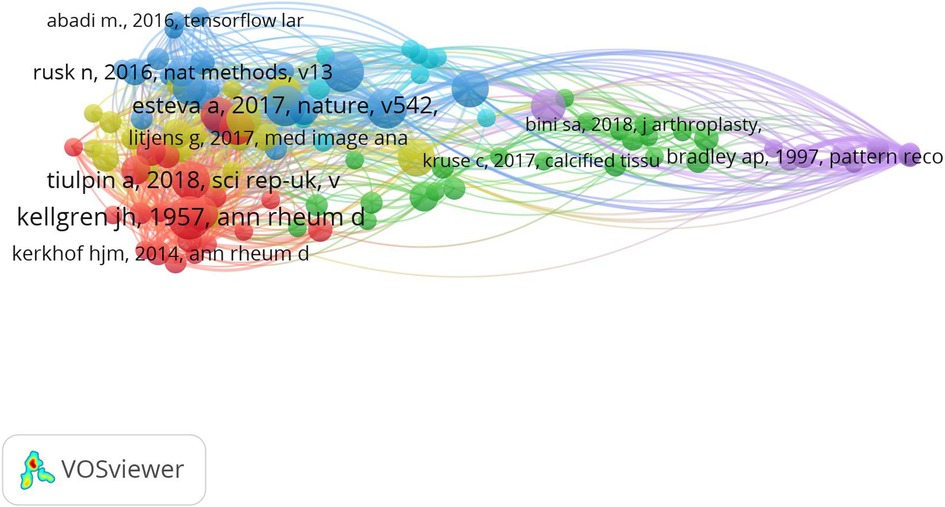
Figure 5. Mapping on co-cited references of publications related to orthopedics and traumatology with artificial intelligence. The varying colors represent different articles which were cited and categorized the articles into distinct clusters. An increase in the size of a point signifies a higher citation frequency of the articles. The line connecting two points indicates that both references had been cited in the same article. The length of a line indicates the proximity between two articles; the closer the link, the shorter the line. The figure was created using VOSviewer (version 1.6.19) software.
Discussion
This bibliometric analysis provides valuable insights into the literature related to the utilization of artificial intelligence in orthopedics up until the present day. The authors of the most cited articles, journal names, countries, and other key characteristics were highlighted. The results of this article indicate that research on artificial intelligence applications in orthopedics will continue to accelerate. The rapid increase in the number of publications in recent years, along with the fact that the most cited articles are closely aligned with the year 2019, serves as indicators of this trend. Interestingly, when considering only the years 2019 and 2020 together, we observed that 56 articles from the list were published during these two years. This observation confirms our initial hypothesis. As far as we know, this is the first study to examine the top 100 most cited articles related to artificial intelligence in orthopedic research. The findings from this article will provide valuable insights for future studies and guide researchers interested in this subject.
When examining the countries where the research was conducted, it is evident that developed countries, especially the USA, have significantly contributed to the literature. We observed that most of the top 100 articles (61 of them) originated solely from the USA. Countries’ support and funding for artificial intelligence research play a crucial role in this distribution. In the realm of artificial intelligence applications in other medical fields as well, the USA leads in academic output (14, 15). The nearest contributor, the England, has contributed less than 20% of the USA's publications. The level of support for science is directly proportional to a country's level of development. As global prosperity increases, other countries will also contribute more to publications related to the use of artificial intelligence in orthopedics, accelerating the integration of artificial intelligence applications into clinical practice.
Keyword analysis reveals that the prominent keywords are “machine learning,” “deep learning,” “artificial intelligence,” and “classification.” Co-occurrence keyword analysis indicates that “machine learning” is the most frequently used keyword. Machine learning, a subset of artificial intelligence, revolves around the principle of enabling virtual machines to learn from data without explicit programming. Machine learning has advanced the field by allowing preoperative risk analysis, outcome prediction, and mortality rate determination.
Fracture recognition systems can lead to a dramatic reduction in misdiagnosis rates and treatment deficiencies. Fracture recognition systems are among the most studied areas of machine learning, focusing particularly on hip and wrist fractures (16, 17). The increasing incidence of osteoporosis due to an aging population has led to a rise in these fracture types, which are the most common and have significant morbidity and mortality. Fracture detection using artificial neural networks minimizes human error. Similarly, arthrosis staging systems can lead to more accurate treatments in orthopedic and rheumatology fields through early diagnosis and precise staging. One of the fundamental issues in classification systems is the challenges encountered in interobserver and intraobserver reliability tests (18). The primary goal of classification systems is to guide treatment. Thus, even though these studies appear to be focused on classification, their true objective is to pave the way for more successful treatments by developing control mechanisms for high-cost surgeries. While the current artificial intelligence studies in the field are primarily diagnostic and preoperative aids for surgeons, we anticipate that they will evolve to be more treatment-oriented and have a greater impact on intraoperative surgical management in the future. Risk classification systems will enable more personalized discussions with patients and their families based on clearer information, shedding light on potential complications and outcomes.
Direct radiographs have been in the foreground and predominantly used in both fracture recognition system and osteoarthritis classification system studies. The fact that direct radiography is the focus of studies can be explained by the fact that it is easier to work with a single two-dimensional cross-sectional image than MRI or CT.
When categorizing the listed studies for diagnostic and therapeutic purposes, we observed that most focused on diagnostic applications, particularly involving decision support systems. These studies predominantly pertain to radiology, which is consistent with the existing literature. In a conducted bibliometric analysis, the top 100 most cited articles in the healthcare field were compiled, and approximately one-fifth of these articles were found to be related to radiology (19). This can be attributed to the fact that radiology benefits from a multitude of artificial intelligence techniques. While fracture diagnosis constitutes the most common application within the field of orthopedics, other frequently employed aspects involve identifying intra-articular structures through MRI and evaluating structures through MRI, as well as the evaluation of structures such as the anterior cruciate ligament, meniscus, and cartilage. By using AI methods, AI models can be trained on large datasets, making pattern recognition and learning easier.
The boundaries of artificial intelligence extend beyond the scope of this study. Clinical intelligence and patient management, which clinicians develop over years in diagnosis and treatment, encompass human aspects that artificial intelligence cannot replicate. While the use of artificial intelligence, especially in robotic surgeries, is currently prominent in orthopedic practice, its impact is bound to increase significantly in everyday practice with technological advancement.
The rate of increase in articles related to artificial intelligence does not progress in direct proportion to its practical implications. In order for orthopedists and other medical professionals in various fields to integrate artificial intelligence applications into their daily practices, reliable clinical studies with high-level evidence, including trustworthy randomized controlled trials and meta-analyses, are required. To foster the adoption of artificial intelligence applications, substantial collaboration between computer science and medical experts is necessary, which in turn elongates the process. With an augmentation in such collaboration, faster progress can be achieved.
There are certain limitations to this article. Firstly, while Web of Science is one of the top-quality search engines available, it does not encompass every journal in the literature, leading to the exclusion of some articles. The search strategy was designed considering the most frequently used potential keywords related to artificial intelligence, but articles using keywords not included in the search strategy may have been excluded. Another limitation is related to the determination of journals’ impact factors. The latest published 2022 Journal Citation Report has been used to determine journals' impact factors; however, it should be taken into account that this list is updated annually.
Conclusion
This bibliometric study reveals that orthopedic research related to artificial intelligence predominantly focuses on fracture diagnosis and osteoarthritis classification. It can be predicted that research related to bone fractures and joint degeneration in different body regions will rise in the near future considering the rate of increase in the number of studies. The fact that these studies are funded by the countries producing the technology can be accepted as an indicator of the reflection on the number of studies. Currently, studies are predominantly focused on diagnosis and staging; however, over time, it is expected that the focus will shift towards treatment.
Data availability statement
The original contributions presented in the study are included in the article/Supplementary Material, further inquiries can be directed to the corresponding author.
Author contributions
NT: Conceptualization, Data curation, Formal Analysis, Investigation, Methodology, Project administration, Resources, Supervision, Validation, Visualization, Writing – original draft, Writing – review & editing. SB: Conceptualization, Methodology, Project administration, Supervision, Writing – original draft, Writing – review & editing.
Funding
The author(s) declare financial support was received for the research, authorship, and/or publication of this article.
This study was funded by Başkent University Research Foundation.
Conflict of interest
The authors declare that the research was conducted in the absence of any commercial or financial relationships that could be construed as a potential conflict of interest.
Publisher's note
All claims expressed in this article are solely those of the authors and do not necessarily represent those of their affiliated organizations, or those of the publisher, the editors and the reviewers. Any product that may be evaluated in this article, or claim that may be made by its manufacturer, is not guaranteed or endorsed by the publisher.
References
1. McCarthy J, Minsky ML, Rochester N, Shannon CE. A proposal for the dartmouth summer research project on artificial intelligence, August 31, 1955. AI Mag. (2006) 27(4):12. doi: 10.1609/aimag.v27i4.1904
2. Beyaz S. A brief history of artificial intelligence and robotic surgery in orthopedics & traumatology and future expectations. Jt Dis Relat Surg. (2020) 31(3):653. doi: 10.5606/ehc.2020.75300
3. Lee LS, Chan PK, Wen C, Fung WC, Cheung A, Chan VWK, et al. Artificial intelligence in diagnosis of knee osteoarthritis and prediction of arthroplasty outcomes: a review. Arthroplasty. (2022) 4(1):16. doi: 10.1186/s42836-022-00118-7
4. Londhe SB, Shetty S, Shetty V, Desouza C, Banka P, Antao N. Comparison of time taken in conventional versus active robotic-assisted total knee arthroplasty. Clin Orthop Surg. (2024) 16(2):259–64. doi: 10.4055/cios23062
5. Hestehave RA, Gundtoft PH, Nielsen CL, Brink O, Rölfing JD. Poor usability of computer-assisted navigation for hip fracture surgery. Arch Orthop Trauma Surg. (2024) 144(1):251–7. doi: 10.1007/s00402-023-05096-2
6. Anwar A, Zhang Y, Zhang Z, Li J. Artificial intelligence technology improves the accuracy of preoperative planning in primary total hip arthroplasty. Asian J Surg. (2024) 10:S1015-9584(24)00186-6. doi: 10.1016/j.asjsur.2024.01.133
7. Zhang Z, Zhu Y, Wang Q, Chang T, Liu C, Zhu Y, et al. Global trends and research hotspots of exercise for intervening diabetes: a bibliometric analysis. Front Public Heal. (2022) 10:902825. doi: 10.3389/fpubh.2022.902825
8. Cheng K, Guo Q, Yang W, Wang Y, Sun Z, Wu H. Mapping knowledge landscapes and emerging trends of the links between bone metabolism and diabetes mellitus: a bibliometric analysis from 2000 to 2021. Front Public Heal. (2022) 10:918483. doi: 10.3389/fpubh.2022.918483
9. Li Y, Xu G, Long X, Ho Y-S. A bibliometric analysis of classic publications in web of science category of orthopedics. J Orthop Surg Res. (2019) 14:1–11. doi: 10.1186/s13018-018-1031-7
10. Li C, Wang L, Perka C, Trampuz A. Clinical application of robotic orthopedic surgery: a bibliometric study. BMC Musculoskelet Disord. (2021) 22:1–14. doi: 10.1186/s12891-020-03840-y
11. Bien N, Rajpurkar P, Ball RL, Irvin J, Park A, Jones E, et al. Deep-learning-assisted diagnosis for knee magnetic resonance imaging: development and retrospective validation of MRNet. PLoS Med. (2018) 15(11):e1002699. doi: 10.1371/journal.pmed.1002699
12. Rutledge R. Injury severity and probability of survival assessment in trauma patients using a predictive hierarchical network model derived from ICD-9 codes. J Trauma Acute Care Surg. (1995) 38(4):590–7; discussion 597-601. doi: 10.1097/00005373-199504000-00022
13. Kellgren JH, Lawrence J. Radiological assessment of osteo-arthrosis. Ann Rheum Dis. (1957) 16(4):494. doi: 10.1136/ard.16.4.494
14. Tran BX, McIntyre RS, Latkin CA, Phan HT, Vu GT, Nguyen HLT, et al. The current research landscape on the artificial intelligence application in the management of depressive disorders: a bibliometric analysis. Int J Environ Res Public Health. (2019) 16(12):2150. doi: 10.3390/ijerph16122150
15. Wang H, Zhou X, Du W, Huang L. The application of artificial intelligence to ophthalmology: a bibliometric study (2000–2021). Arch Clin Med Microbiol. (2022) 1(1):41–50. doi: 10.20944/preprints202111.0080.v1
16. Lin C-C, Ou Y-K, Chen S-H, Liu Y-C, Lin J. Comparison of artificial neural network and logistic regression models for predicting mortality in elderly patients with hip fracture. Injury. (2010) 41(8):869–73. doi: 10.1016/j.injury.2010.04.023
17. Kim DH, MacKinnon T. Artificial intelligence in fracture detection: transfer learning from deep convolutional neural networks. Clin Radiol. (2018) 73(5):439–45. doi: 10.1016/j.crad.2017.11.015
18. Audigé L, Bhandari M, Kellam J. How reliable are reliability studies of fracture classifications? A systematic review of their methodologies. Acta Orthop Scand. (2004) 75(2):184–94. doi: 10.1080/00016470412331294445
Keywords: machine learning, deep learning, decision support, artificial intelligence, classification
Citation: Turgut N and Beyaz S (2024) The 100 most cited articles in artificial intelligence related to orthopedics. Front. Surg. 11:1370335. doi: 10.3389/fsurg.2024.1370335
Received: 14 January 2024; Accepted: 4 April 2024;
Published: 17 April 2024.
Edited by:
Deepti Rana, University of Twente, NetherlandsReviewed by:
António Proença Caetano, NOVA University of Lisbon, PortugalŁukasz A. Poniatowski, Medical University of Warsaw, Poland
© 2024 Turgut and Beyaz. This is an open-access artificial distributed under the terms of the Creative Commons Attribution License (CC BY). The use, distribution or reproduction in other forums is permitted, provided the original author(s) and the copyright owner(s) are credited and that the original publication in this journal is cited, in accordance with accepted academic practice. No use, distribution or reproduction is permitted which does not comply with these terms.
*Correspondence: Necmettin Turgut ZHJuZWNtZXR0aW50dXJndXRAaG90bWFpbC5jb20=