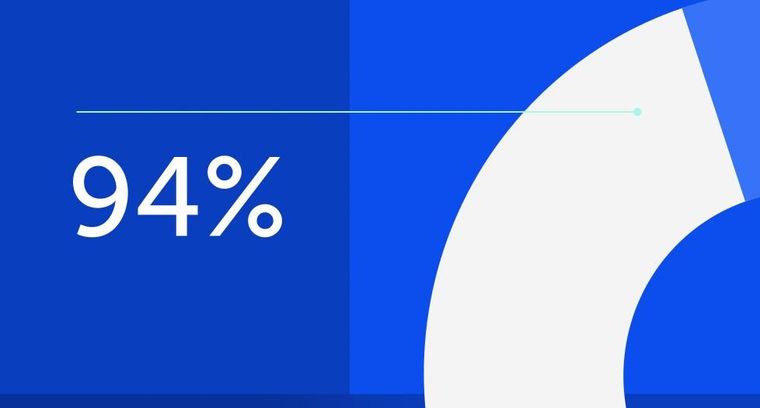
94% of researchers rate our articles as excellent or good
Learn more about the work of our research integrity team to safeguard the quality of each article we publish.
Find out more
ORIGINAL RESEARCH article
Front. Surg., 16 June 2022
Sec. Surgical Oncology
Volume 9 - 2022 | https://doi.org/10.3389/fsurg.2022.920589
This article is part of the Research TopicAdvances in Surgical Treatment of Hepatobiliary TumorsView all 13 articles
Objective: Hepatocellular carcinoma (HCC) is the second leading cause of cancer-related deaths worldwide. This study aims to construct a novel practical nomogram and risk stratification system to predict cancer-specific survival (CSS) in HCC patients with severe liver fibrosis.
Methods: Data on 1,878 HCC patients with severe liver fibrosis in the period 1975 to 2017 were extracted from the Surveillance, Epidemiology, and End Results database (SEER). Patients were block-randomized (1,316 training cohort, 562 validation cohort) by setting random seed. Univariate and multivariate COX regression analyses were employed to select variables for the nomogram. The consistency index (C-index), the area under time-dependent receiver operating characteristic curve (time-dependent AUC), and calibration curves were used to evaluate the performance of the nomogram. Decision curve analysis (DCA), the C-index, the net reclassification index (NRI), and integrated discrimination improvement (IDI) were used to compare the nomogram with the AJCC tumor staging system. We also compared the risk stratification of the nomogram with the American Joint Committee on Cancer (AJCC) staging system.
Results: Seven variables were selected to establish the nomogram. The C-index (training cohort: 0.781, 95%CI: 0.767–0.793; validation cohort: 0.793, 95%CI = 95%CI: 0.779–0.798) and the time-dependent AUCs (the training cohort: the values of 1-, 3-, and 5 years were 0.845, 0.835, and 0.842, respectively; the validation cohort: the values of 1-, 3-, and 5 years were 0.861, 0.870, and 0.876, respectively) showed satisfactory discrimination. The calibration plots also revealed that the nomogram was consistent with the actual observations. NRI (training cohort: 1-, 2-, and 3-year CSS: 0.42, 0.61, and 0.67; validation cohort: 1-, 2-, and 3-year CSS: 0.26, 0.52, and 0.72) and IDI (training cohort: 1-, 3-, and 5-year CSS:0.16, 0.20, and 0.22; validation cohort: 1-, 3-, and 5-year CSS: 0.17, 0.26, and 0.30) indicated that the established nomogram significantly outperformed the AJCC staging system (P < 0.001). Moreover, DCA also showed that the nomogram was more practical and had better recognition.
Conclusion: A nomogram for predicting CSS for HCC patients with severe liver fibrosis was established and validated, which provided a new system of risk stratification as a practical tool for individualized treatment and management.
HCC is the second leading cause of cancer-related mortality globally and the fifth most severe malignancy (1, 2). The five-year survival rate for patients with HCC is low due to therapeutic restriction (3). The risk factors vary depending on the distribution of the region. For example, chronic hepatitis B virus infection is the primary factor in Asia, while chronic hepatitis C virus infection, alcoholic liver disease, and nonalcoholic fatty liver disease are the prominent risk factors in Europe and America (4–7). A total of 80%–90% HCC patients have biopsy evidence of liver fibrosis (8). Liver fibrosis, a chronic liver injury repair process, is characterized by the activation of hepatic stellate cells into myofibroblasts and the production of large amounts of extracellular matrix, leading to a gradual destruction of the normal structure and physiological function of liver tissue, with scar tissue replacing liver parenchyma and ultimately death, which may eventually lead to cirrhosis, liver failure, or liver cancer (9). Severe liver fibrosis is an irreversible biological process for which no drugs have been proven to be effective (10, 11). Moreover, the management of HCC patients with severe liver fibrosis is extremely controversial in nature (12).
The American Joint Committee on Cancer (AJCC) tumor-node-metastasis (TNM) system is the most commonly used method to evaluate the prognosis of patients with HCC (13). However, the TNM system has some limitations such as low accuracy, ignoring of other factors (age, sex, etc.), and poor performance in predicting individual survival risk (14). As a result, a new and personalized prediction model is needed to evaluate the prognosis of HCC patients.
Recently, clinical models related to nomograms have been widely applied for the survival prediction of tumor patients through a comprehensive analysis of neoplasm-related risk factors (15, 16). Moreover, nomograms can effectively predict tumor prognosis and promote personalized medicine. However, there are no prognostic models for HCC patients with severe fibrosis (Ishak 5–6; Advanced/severe fibrosis; METAVIR F4; Batt-Ludwig 4; Cirrhosis). Therefore, it is necessary to establish a practical, reliable, and specific prediction model to predict CSS for HCC patients with severe liver fibrosis.
Clinically relevant data were extracted from the SEER database between 1975 and 2017 via SEER*Stat 8.3.9.2 software. The SEER database was made publicly accessible and private data for all patients were removed from the database, which indicated that institutional review board approval and informed consent were not required.
The inclusion criteria were as follows: (a) HCC patients with severe liver fibrosis (Ishak 5–6; Advanced/severe fibrosis; METAVIR F4; Batt-Ludwig 4; Cirrhosis); (b) complete treatment information. The exclusion criteria were as follows: (a) unknown liver fibrosis score or mild liver fibrosis; (b) metastatic liver cancer; (c) imperfect treatment information; (d) unknown tumor stage; (e) unknown tumor pathological grade; (f) unknown household income; (g) other tumor death and unknown cause of death. Finally, eleven variables were included from the SEER database: age (at diagnosis), ethnicity, gender, tumor number, pathological grade, tumor size, extension, tumor stage (AJCC stage), type of surgery, AFP, and insurance. In addition, the seventh edition of the AJCC-TNM staging was used for the analysis.
All patients were randomly divided into a training cohort and a validation cohort at a ratio of 7:3. The training cohort was used to create a nomogram, while the validation cohort was used for validation. Univariate and multivariate COX regression analyses were employed to obtain significant factors that significantly affected CSS and further construct the nomogram (Supplementary Table).
C-index and ROC curves reflected the predictive capability of the nomogram. The value was above 0.5, indicating predictive performance, which could be divided into low precision (0.5–0.7), medium precision (0.71–0.9), and high precision (>0.9). 1-, 3-, and 5- year calibration curves were plotted to evaluate calibrating ability, and the 45-degree line was used as the actual outcome of the primary model.
Based on the nomogram, a novel risk stratification system was developed, which could divide patients into low-, middle-, and high-risk groups (the best cut-off value for the total score was selected by using X-tile). The net reclassification index (NRI), C-index, IDI, and decision curve analysis (DCA) were adopted to compare the risk stratification of the nomogram model with that of the AJCC stage system. The NRI, C-index, and integrated discrimination improvement (IDI) were applied to assess the improvement in risk prediction and determine the effectiveness of the new model. DCA was performed to evaluate the net benefit of various models. Kaplan–Meier curves were used to compare the risk stratification of the nomogram with that of AJCC staging criteria.
Univariate and multivariate COX regression analyses, C-index, calibration curves, ROC curves, and DCA curves were generated using R version 4.1.2 and related software packages. The optimal cut-off point for risk stratification was selected utilizing X-tile (version 3.6.1). Statistical differences of distribution between the training and the validation cohorts were analyzed by the Chi-square test. All p-values resulted from two-side statistical testing, while a p-value less than 0.05 was considered statistically significant.
A total of 1,878 HCC patients with severe liver fibrosis were included in our study, with 1,316 (70%) in the training cohort and 562 (30%) in the validation cohort. The flow diagram is shown in Figure 1. Of all patients eligible for the study, there were 1,465 (77.82%) male patients and 413 female patients. There were 1,331 white and 243 black patients, which accounted for 71.14% and 12.99%, respectively. Of all the patients, 805 were treated conservatively and 300 were treated locally. A total of 394 patients underwent resection of liver masses and 379 underwent liver transplantation. A total of 1,539 patients were well-differentiated, while 339 were poorly differentiated. The baseline information related to the training and validation groups is provided in Table 1. There was no statistically significant difference between the two cohorts.
Figure 1. Flow diagram of the hepatocellular carcinoma patients with severe liver fibrosis with training and validation cohorts.
Table 1. Demographics and clinical characteristics of hepatocellular carcinoma patients with severe liver fibrosis at diagnosis.
Univariate COX regression analysis showed that age, race, pathological grade, AJCC stages, tumor size, AFP, surgery, tumor size, and income were all statistically significant on prognosis (P < 0.05) (Table 2). Multivariate analysis suggested that age, pathological grade, AJCC stages, AFP, surgery, and tumor size were independent prognostic factors affecting the CSS of HCC patients with severe liver fibrosis, which were, therefore, included in the nomogram model.
Table 2. The results of univariate and multivariate Cox regression analyses on variables for the prediction of CSS.
Eventually, 7 variables (age, pathological grade, AJCC stages, tumor size, AFP, surgery, and income) were selected to construct the nomogram to predict the probability of CSS in HCC patients with severe liver fibrosis (Figure 2). First, risk scores for each variable were derived based on the information of patients. The total risk score of the patient is obtained by adding the scores of all variables, and the corresponding position of the risk score of the patient can be found in the line of total scores. Finally, the probability of 1-, 3-, and 5-year CSS for HCC patients with severe liver fibrosis could be referred by drawing a straight line on the last 3 rows. The C-indexes for the training and validation cohorts were 0.781 (95% CI: 0.767–0.793) and 0.793 (95% CI: 0.779–0.798) (P < 0.05), respectively. The calibration curve, ROC curve, and DCA curve are shown in Figures 3–5. The 1-, 3-, and 5-year time-dependent AUCs for the training cohort were 0.845, 0.835, and 0.842, respectively, while those for the validation cohort were 0.861, 0.870, and 0.876, respectively, manifesting that the model had excellent predictive performance. In addition, the nomogram-related DCA curves at 1, 3, and 5 years in the training and validation cohorts also exhibited promising potential for clinical application and better positive net benefits. The calibration curves revealed good consistency in the probability of 1-, 3-, and 5-year CSS between the nomogram prediction and the observed results in both cohorts.
Figure 3. ROC of the nomogram for 1-, 3-, and 5-year prediction. (A) Training cohorts based on the nomogram; (B) Validation cohorts based on the nomogram.
Figure 4. Calibration plots of 1-year, 3-year, and 5-year CSS for hepatocellular carcinoma patients with severe liver fibrosis. (A,C,E) Calibration plot of 1-year, 3-year, and 5-year CSS in the training cohort; (B,C,F) Calibration plot of 1-year, 3-year, and 5-year CSS in the training cohort; CSS, cancer-specific survival.
Figure 5. Decision curve analysis. (A,C,E) DCA curve of 1-year, 3-year, and 5-year CSS in the training cohort; (B,D,F) DCA curve of 1-year, 3-year, and 5-year CSS in the validation cohort. DCA, decision curve analysis; CSS, cancer-specific survival.
In the training cohort, the C-index of the nomogram was higher than that of the AJCC stage system (Figure 6). The NRIs for the 1-year, 3-year, and 5-year CSS were 0.42 (95% CI = 0.27–0.56), 0.61 (95% CI = 0.50–0.77) and 0.67 (95% CI = 0.49–0.80), respectively, in the training cohort, and 0.26 (95% CI = 0.17–0.46), 0.52 (95% CI = 0.22–0.76), and 0.72 (95% CI = 0.44–0.92), respectively, in the validation cohort. IDI (training cohort: 1-, 3-, and 5-year CSS: 0.16, 0.20, and 0.22; validation cohort: 1-, 3-, and 5-year CSS: 0.17, 0.26, and 0.30) indicated that the established nomogram significantly outperformed the AJCC staging system (P < 0.05) (Table 3). These results indicated that the nomogram was more accurate than predictions based on AJCC staging criteria. In addition, we compared the net benefit of the nomogram with the AJCC staging criteria. DCA curves in both the training and the validation cohorts showed that the nomogram better predicted 1-, 3-, and 5-year CSS because it added more net benefit compared with the AJCC staging criteria as well as the treat-all-patients scheme and the treat-none scheme.
Figure 6. C-index analysis. (A) The nomogram related C-index; (B) AJCC staging criteria related C-index.
Table 3. NRI and IDI of the nomogram and AJCC staging criteria alone in CSS prediction for hepatocellular carcinoma patients with severe liver fibrosis.
Along with the generation of the nomogram, a risk stratification system, which was distinguished according to the calculation of the total score, was developed. All patients were classified into three risk groups: low risk (total score <446), middle risk (446 ≤ total score <504), and high risk (total score ≥504) (Figure 7). Kaplan–Meier curves presented clearly marked survival differences among patients in different risk groups. In contrast, the AJCC staging criteria model had limited ability to identify I–II and III–IV in both the training and the validation cohorts (Figure 8).
Figure 8. Kaplan–Meier CSS curves of hepatocellular carcinoma patients with severe liver fibrosis based on different criteria. (A,B) Kaplan–Meier CSS curves of training and validation cohorts based on the new risk stratification system; (C,D) Kaplan–Meier CSS curves of training and validation cohorts based on AJCC staging criteria.
HCC is the sixth most common malignant cancer in incidence worldwide (17, 18), with 80%–90% of patients suffering from liver damage, chronic inflammation, and fibrous repair (19). Findings have shown that fibroblasts secreting cytokines and growth hormones can implicitly or explicitly accelerate the value-added and invasion of HCC, while a proportion of tumor-associated fibroblasts is a part of the malignant microenvironment (20, 21). HCC, combined with severe liver fibrosis, has made clinicians face substantial challenges in therapy. Meanwhile, clinical evidence for the prognosis of HCC patients with serious liver fibrosis is scarce, and there is still a shortage of risk models. Consequently, this research developed and validated a nomogram to predict the prognostic value by analyzing the demographic and clinical characteristics of HCC patients with serious liver fibrosis in the SEER database. Multiple validation results indicated that the nomogram had favorable discriminatory ability. Based on the nomogram, we developed a novel risk stratification system where patients were classified into low-risk, middle-risk, and high-risk groups. Compared with the AJCC criteria, this risk stratification system not only accurately predicted the prognosis of patients, but also provided individualized management and treatment for HCC patients with serious liver fibrosis.
Age was an independent predictor for CSS in HCC patients with serious liver fibrosis, which indicated that older age was associated with poor prognosis. Multiple studies have shown that AJCC TNM stage is an independent influencing factor for HCC, which is generally consistent with our findings (22). Patients with a higher pathological grading have a longer CSS than those with a lower pathological grading, implying that pathological grading reflects the prognosis of HCC. AFP is one of the most relevant physiological markers for screening, clinical diagnosis, effectiveness evaluation, and post-treatment monitoring in high-risk populations of liver cancer. According to current studies (23), prediction models including serum AFP can enhance the predicting recurrence of tumor after liver transplantation. Consequently, some surgeons will choose AFP models to select HCC patients who may not match Milan transplantation criteria (24). In addition, researchers are working on constructing a predictive model including AFP for HCC that involves Child B liver function. CSS is noticeably shorter in AFP-positive patients than in AFP-negative patients, which reveals that AFP exhibits a substantial predictive value in predicting long-term CSS in HCC patients with severe liver fibrosis (25, 26).
Currently, HCC is treated with chemotherapy, local therapy, mass resection, and liver transplantation. Nevertheless, there is no universally accepted treatment for HCC with severe liver fibrosis. HCC patients with severe hepatic fibrosis and those with slight liver fibrosis had diverse prognoses in previous decades (27, 28). Furthermore, it is widely considered that surgery may worsen the prognosis of HCC patients with severe liver fibrosis. Several guidelines have recommended chemotherapy, combined with local therapy, as the first-line treatment for HCC patients with severe liver fibrosis (29, 30). However, with the implementation and advancement of minimally invasive techniques in clinical practice, the rates of postoperative liver failure, mortality, and infection have decreased significantly (31, 32). Thus, surgery may provide better long-term benefits than local therapy based on acceptable short-term postoperative mortality and infection rates. According to existing studies, the five-year postoperative survival rate for HCC patients with severe liver fibrosis is up to 35% (32–34). Several of the most influential hepatobiliary institutions have argued that HCC in the presence of significant liver fibrosis is not an absolute contraindication to surgery and that patients with grade B or even C liver cancer can benefit from surgery (35, 36).
Despite the promising application of the nomogram in predicting CSS in HCC with severe liver fibrosis, this study had several limitations. First, the data lacked information on patient etiology, for instance, hepatitis B or C virus infection and alcoholic liver disease, which might affect tumor characteristics. Moreover, data on hematological indicators and surgical margins were not recorded. Finally, our model lacked a multicenter clinical sample for further validation to provide more convincing evidence.
In conclusion, a practical and reliable nomogram for predicting CSS for HCC patients with severe liver fibrosis was constructed based on the significant risk factors identified in the analysis, which could effectively solve the survival paradox caused by the AJCC staging system and might help physicians make appropriate clinical decisions.
The original contributions presented in the study are included in the article/Supplementary Material, and further inquiries can be directed to the corresponding author/s.
The SEER database was made publicly available and the private data of all patients were eliminated from the database. Therefore, informed consent and institutional review board approval were not required.
Conceptualization was done by DY, YS, and FZ, data curation was performed by CC and KZ, formal analysis was conducted by DY, XX, and YS, writing of the original draft was done by DY, YS, and FZ, and the work of writing the review and editing was taken up by YD. All authors contributed to the article and approved the submitted version.
The Supplementary Material for this article can be found online at: https://www.frontiersin.org/articles/10.3389/fsurg.2022.920589/full#supplementary-material.
The authors declare that the research was conducted in the absence of any commercial or financial relationships that could be construed as a potential conflict of interest.
All claims expressed in this article are solely those of the authors and do not necessarily represent those of their affiliated organizations, or those of the publisher, the editors and the reviewers. Any product that may be evaluated in this article, or claim that may be made by its manufacturer, is not guaranteed or endorsed by the publisher.
1. Santucci C, Carioli G, Bertuccio P, Malvezzi M, Pastorino U, Boffetta P, et al. Progress in cancer mortality, incidence, and survival: a global overview. Eur J Cancer Prev. (2020) 29(5):367–81. doi: 10.1097/CEJ.0000000000000594
2. Torre LA, Siegel RL, Ward EM, Jemal A. Global cancer incidence and mortality rates and trends–an update. Cancer Epidemiol Biomarkers Prev. (2016) 25(1):16–27. doi: 10.1158/1055-9965.EPI-15-0578
3. Arora S, Harmath C, Catania R, Mandler A, Fowler KJ, Borhani AA. Hepatocellular carcinoma: metastatic pathways and extra-hepatic findings. Abdom Radiol (NY). (2021) 46(8):3698–707. doi: 10.1007/s00261-021-03151-3
4. Gassmann P, Spieker T, Haier J, Schmidt F, Mardin WA, Senninger N. Prognostic impact of underlying liver fibrosis and cirrhosis after curative resection of hepatocellular carcinoma. World J Surg. (2010) 34(10):2442–51. doi: 10.1007/s00268-010-0655-5
5. Roberts SK, Majeed A, Kemp W. Controversies in the management of hepatitis B: hepatocellular carcinoma. Clin Liver Dis. (2021) 25(4):785–803. doi: 10.1016/j.cld.2021.06.006
6. Marrero JA. Hepatocellular carcinoma. Curr Opin Gastroenterol. (2006) 22(3):248–53. doi: 10.1097/01.mog.0000218961.86182.8c
7. Neuschwander-Tetri BA. Non-alcoholic fatty liver disease. BMC Med. (2017) 15(1):45. doi: 10.1186/s12916-017-0806-8
8. Kubo N, Araki K, Kuwano H, Shirabe K. Cancer-associated fibroblasts in hepatocellular carcinoma. World J Gastroenterol. (2016) 22(30):6841–50. doi: 10.3748/wjg.v22.i30.6841
9. Baglieri J, Brenner DA, Kisseleva T. The role of fibrosis and liver-associated fibroblasts in the pathogenesis of hepatocellular carcinoma. Int J Mol Sci. (2019) 20(7):1723–41. doi: 10.3390/ijms20071723
10. Schuppan D, Ashfaq-Khan M, Yang AT, Kim YO. Liver fibrosis: direct antifibrotic agents and targeted therapies. Matrix Biol. (2018) 68-69:435–51. doi: 10.1016/j.matbio.2018.04.006
11. Tan Z, Sun H, Xue T, Gan C, Liu H, Xie Y, et al. Liver fibrosis: therapeutic targets and advances in drug therapy. Front Cell Dev Biol. (2021) 9:730176. doi: 10.3389/fcell.2021.730176
12. Mendoza YP, Becchetti C, Watt KD, Berzigotti A. Risks and rewards of bariatric surgery in advanced chronic liver diseases. Semin Liver Dis. (2021) 41(4):448–60. doi: 10.1055/s-0041-1731705
13. Chan AC, Fan ST, Poon RT, Cheung TT, Chok KS, Chan SC, et al. Evaluation of the seventh edition of the American Joint Committee on Cancer tumour-node-metastasis (TNM) staging system for patients undergoing curative resection of hepatocellular carcinoma: implications for the development of a refined staging system. HPB (Oxford). (2013) 15(6):439–48. doi: 10.1111/j.1477-2574.2012.00617.x
14. Minagawa M, Ikai I, Matsuyama Y, Yamaoka Y, Makuuchi M. Staging of hepatocellular carcinoma: assessment of the Japanese TNM and AJCC/UICC TNM systems in a cohort of 13,772 patients in Japan. Ann Surg. (2007) 245(6):909–22. doi: 10.1097/01.sla.0000254368.65878.da
15. Dhir M, Samson KK, Yepuri N, Yanala UR, Smith LM, Are C. Preoperative nomogram to predict posthepatectomy liver failure. J Surg Oncol. (2021) 123(8):1750–6. doi: 10.1002/jso.26463
16. Pan M, Yang Y, Teng T, Lu F, Chen Y, Huang H. Development and validation of a simple-to-use nomogram to predict liver metastasis in patients with pancreatic neuroendocrine neoplasms: a large cohort study. BMC Gastroenterol. (2021) 21(1):101. doi: 10.1186/s12876-021-01685-w
17. Fujiwara N, Friedman SL, Goossens N, Hoshida Y. Risk factors and prevention of hepatocellular carcinoma in the era of precision medicine. J Hepatol. (2018) 68(3):526–49. doi: 10.1016/j.jhep.2017.09.016
18. Moon AM, Singal AG, Tapper EB. Contemporary epidemiology of chronic liver disease and cirrhosis. Clin Gastroenterol Hepatol. (2020) 18(12):2650–66. doi: 10.1016/j.cgh.2019.07.060
19. Keenan BP, Fong L, Kelley RK. Immunotherapy in hepatocellular carcinoma: the complex interface between inflammation, fibrosis, and the immune response. J Immunother Cancer. (2019) 7(1):267. doi: 10.1186/s40425-019-0749-z
20. Barry AE, Baldeosingh R, Lamm R, Patel K, Zhang K, Dominguez DA, et al. Hepatic stellate cells and hepatocarcinogenesis. Front Cell Dev Biol. (2020) 8(709):709–25. doi: 10.3389/fcell.2020.00709
21. Matsuda M, Seki E. Hepatic stellate cell-macrophage crosstalk in liver fibrosis and carcinogenesis. Semin Liver Dis. (2020) 40(3):307–20. doi: 10.1055/s-0040-1708876
22. European Association for the Study of the Liver. EASL clinical practice guidelines: management of hepatocellular carcinoma. J Hepatol. (2018) 69(1):182–236. doi: 10.1016/j.jhep.2018.03.019
23. Berardi G, Morise Z, Sposito C, Igarashi K, Panetta V, Simonelli I, et al. Development of a nomogram to predict outcome after liver resection for hepatocellular carcinoma in Child-Pugh B cirrhosis. J Hepatol. (2020) 72(1):75–84. doi: 10.1016/j.jhep.2019.08.032
24. Tsilimigras DI, Hyer JM, Diaz A, Bagante F, Ratti F, Marques HP, et al. Synergistic impact of alpha-fetoprotein and tumor burden on long-term outcomes following curative-intent resection of hepatocellular carcinoma. Cancers (Basel). (2021) 13(4):747–59. doi: 10.3390/cancers13040747
25. Akoad ME, Pomfret EA. Surgical resection and liver transplantation for hepatocellular carcinoma. Clin Liver Dis. (2015) 19(2):381–99. doi: 10.1016/j.cld.2015.01.007
26. Asrani SK, Devarbhavi H, Eaton J, Kamath PS. Burden of liver diseases in the world. J Hepatol. (2019) 70(1):151–71. doi: 10.1016/j.jhep.2018.09.014
27. Cauchy F, Fuks D, Belghiti J. HCC: current surgical treatment concepts. Langenbecks Arch Surg. (2012) 397(5):681–95. doi: 10.1007/s00423-012-0911-2
28. Vivarelli M, Montalti R, Risaliti A. Multimodal treatment of hepatocellular carcinoma on cirrhosis: an update. World J Gastroenterol. (2013) 19(42):7316–26. doi: 10.3748/wjg.v19.i42.7316
29. Kanwal F, Singal AG. Surveillance for hepatocellular carcinoma: current best practice and future direction. Gastroenterology. (2019) 157(1):54–64. doi: 10.1053/j.gastro.2019.02.049
30. Xie DY, Ren ZG, Zhou J, Fan J, Gao Q. 2019 Chinese clinical guidelines for the management of hepatocellular carcinoma: updates and insights. Hepatobiliary Surg Nutr. (2020) 9(4):452–63. doi: 10.21037/hbsn-20-480
31. Pan Y, Xia S, Cai J, Chen K, Cai X. Efficacy of laparoscopic hepatectomy versus open surgery for hepatocellular carcinoma with cirrhosis: a meta-analysis of case-matched studies. Front Oncol. (2021) 11:652272. doi: 10.3389/fonc.2021.652272
32. Vega EA, Kutlu OC, Joechle K, De La Cruz N, Ko D, Conrad C. Preoperative prognosticators of safe laparoscopic hepatocellular carcinoma resection in advanced cirrhosis: a propensity score matching population-based analysis of 1799 western patients. J Gastrointest Surg. (2019) 23(6):1157–65. doi: 10.1007/s11605-019-04139-7
33. Di Sandro S, Bagnardi V, Najjar M, Buscemi V, Lauterio A, De Carlis R, et al. Minor laparoscopic liver resection for hepatocellular carcinoma is safer than minor open resection, especially for less compensated cirrhotic patients: propensity score analysis. Surg Oncol. (2018) 27(4):722–9. doi: 10.1016/j.suronc.2018.10.001
34. Goh EL, Chidambaram S, Ma S. Laparoscopic vs open hepatectomy for hepatocellular carcinoma in patients with cirrhosis: a meta-analysis of the long-term survival outcomes. Int J Surg. (2018) 50:35–42. doi: 10.1016/j.ijsu.2017.12.021
35. Beumer BR, de Wilde RF, Metselaar HJ, de Man RA, Polak WG, Ijzermans JNM. The treatment effect of liver transplantation versus liver resection for HCC: a review and future perspectives. Cancers (Basel). (2021) 13(15). doi: 10.3390/cancers13153730
Keywords: severe liver fibrosis, nomogram, cancer-specific survival, risk stratification system, hepatocellular carcinoma
Citation: Yang D, Su Y, Zhao F, Chen C, Zhao K, Xiong X and Ding Y (2022) A Practical Nomogram and Risk Stratification System Predicting Cancer-Specific Survival for Hepatocellular Carcinoma Patients With Severe Liver Fibrosis. Front. Surg. 9:920589. doi: 10.3389/fsurg.2022.920589
Received: 14 April 2022; Accepted: 25 May 2022;
Published: 16 June 2022.
Edited by:
Georgios C. Sotiropoulos, National and Kapodistrian University of Athens, GreeceReviewed by:
Nikolaos Machairas, National and Kapodistrian University of Athens, GreeceCopyright © 2022 Yang, Su, Zhao, Chen, Zhao, Xiong and Ding. This is an open-access article distributed under the terms of the Creative Commons Attribution License (CC BY). The use, distribution or reproduction in other forums is permitted, provided the original author(s) and the copyright owner(s) are credited and that the original publication in this journal is cited, in accordance with accepted academic practice. No use, distribution or reproduction is permitted which does not comply with these terms.
*Correspondence: Youming Ding ZGluZ3ltNjJAMTYzLmNvbQ== Chen Chen YXBwcmVjaWF0aW9uQHdodS5lZHUuY24=
†These authors have contributed equally to this work
Specialty section: This article was submitted to Surgical Oncology, a section of the journal Frontiers in Surgery
Disclaimer: All claims expressed in this article are solely those of the authors and do not necessarily represent those of their affiliated organizations, or those of the publisher, the editors and the reviewers. Any product that may be evaluated in this article or claim that may be made by its manufacturer is not guaranteed or endorsed by the publisher.
Research integrity at Frontiers
Learn more about the work of our research integrity team to safeguard the quality of each article we publish.