- 1General Surgery Department, Hospital of Xinjiang Production and Construction Corps, Urumchi, China
- 2General Surgery Department, Peking Union Medical College Hospital, China Academy of Medical Science & Peking Union Medical College, Beijing, China
Background: Colorectal cancer (CRC) is one of the most common malignant tumors with recurrence and metastasis after surgical resection. This study aimed to identify the physiological changes after surgery and explore metabolites and metabolic pathways with potential prognostic value for CRC.
Methods: An ultra-high-performance liquid chromatography Q-exactive mass spectrometry was used to profile serum metabolites from 67 CRC patients and 50 healthy volunteers. Principal component analysis (PCA) and orthogonal projections to latent structures-discriminant analysis were used to distinguish the internal characteristics of data in different groups. Multivariate statistics were compiled to screen the significant metabolites and metabolic pathways.
Result: A total of 180 metabolites were detected. Under the conditions of variable importance in projection >1 and p-value <0.05, 46 differentially expressed metabolites were screened for further pathway enrichment analysis. Based on the Kyoto Encyclopedia of Genes and Genomes database and Small Molecule Pathway Database, three metabolic pathways—arginine and proline metabolism, ascorbate and aldarate metabolism, and phenylalanine metabolism—were significantly altered after surgical resection and identified as associated with the removal of CRC. Notably, gamma-linolenic acid was upregulated in the CRC preoperative patients compared with those in healthy volunteers but returned to healthy levels after surgery.
Conclusion: Through serum-based metabolomics, our study demonstrated the differential metabolic characteristics in CRC patients after surgery compared with those before surgery. Our results suggested that metabonomic analysis may be a powerful method for exploring physiological alterations in CRC patients after surgery as well as a useful tool for identifying candidate biomarkers and monitoring disease recurrence.
Introduction
Colorectal cancer (CRC) ranks third among malignant tumors and second among the leading causes of cancer-related deaths worldwide (1). Despite the great advances in early detection and multimodality therapy for CRC (2), the outcome of patients with advanced tumors is still not satisfactory. Distant metastasis and recurrence are the major causes of death in patients with CRC (3). Therefore, there is an urgent need to better understand the underlying mechanisms of CRC occurrence and progression and to explore more effective interventions to prevent CRC recurrence.
Previous studies have shown that metabolic disorders contribute to the formation of a protumorigenic environment and are associated with the risk of CRC (4). Metabolomics, as a branch of systems biology following genomics, transcriptomics, and proteomics, was established to detect and analyze the profiling of metabolites in biofluids, cells, and tissues (5). Metabolomics is a technology used for identifying endogenous compounds as biomarkers in diseases and for unveiling potential mechanisms associated with disease processes (6). A variety of analytical platforms, including high-performance liquid chromatography/mass spectrometry (HPLC/MS), UPLC time-of-flight MS (UPLC-TOF-MS), and ultra-performance LC–MS (LC–MS), have been used to profile metabolites in various tumors by using tissue, urine, and serum samples (7, 8). A variety of metabolites, including tyrosine and glutamine–leucine, have been identified as biomarkers for the early diagnosis of CRC (9, 10). Our previous study also showed the important predictive role of hexadecanedioic acid, 4-dodecylbenzenesulfonic acid, 2-pyrocatechuic acid, and formylanthranilic acid in CRC patients by comparing serum differential metabolites between CRC patients and healthy controls (11).
Currently, surgical resection, including sufficient adjacent large intestine, mesentery, nearby lymph nodes, and blood vessels, is still the mainstay treatment for CRC patients (12). However, there are few studies exploring the changes in the serum metabolite profiles before and after surgery for patients with CRC. The metabolic impact of radical tumor resection on CRC patients remains unknown. In addition, there is still a lack of effective biomarkers for monitoring tumor recurrence after surgery. In our study, metabolomic technology based on ultra-high-performance liquid chromatography (UHPLC) was used to analyze the metabolic differences in the serum of CRC patients before and after radical surgical resection. The identification of affected metabolites and metabolic pathways may reveal the metabolic alterations associated with radical resection of colorectal tumors, providing insights into the comprehensive physiological changes associated with radical resection for CRC and improving guidelines for postoperative care, which may offer new methods for predicting the prognosis of surgical resection in CRC patients.
Materials and methods
Participants
The inclusion criteria for the healthy control group were healthy adults aged 18–80 years without any forms of cancer. Patients were included in the CRC group of this research if they met the following inclusion criteria: those who were 18–80 years old, had a definite diagnosis of carcinoma by biopsy pathology through colonoscopy or proctoscopy, successfully underwent radical resection of CRC, and had provided informed consent to participate in the study.
Patients who met the following conditions were excluded: those who had any other malignancy; had chronic renal failure; had human immunodeficiency virus infection or metabolic diseases, such as diabetes, fatty liver and obesity; or declined to participate.
A total of 50 healthy volunteers and 67 CRC patients were recruited for this study at the Peking Union Medical College Hospital between 2019 and 2021. All 67 CRC patients underwent radical resection of CRC by the same experienced surgical team. Information on the clinical characteristics of the patients, namely, sex, age, body mass index (BMI), level of serum carcinoma embryonic antigen (CEA), and histopathology classification, was collected and analyzed retrospectively. The research conformed to the Declaration of Helsinki and the guidelines of ethical standards. The research was reviewed and approved by the Ethics Committee of Peking Union Medical College Hospital. Signed informed consent was provided by all participants in this research.
Collection and preparation of samples
All blood samples were collected in the morning after fasting for 12 h, centrifuged at 3,000 rpm for 15 min at 4°C to separate the serum within 2 h, and preserved at −80°C for subsequent analysis. Blood samples of CRC patients were collected just before surgery and 1 week after the operation.
All serum samples were thawed at 4°C for approximately 1 h. Then, a 150 µl of serum was added to a 100 µl of freshly prepared internal standard solution and a 450 µl of precooled formaldehyde. The thoroughly mixed samples in a 2.0-ml Eppendorf tube were then centrifuged at 14,000 × g for 15 min at room temperature. The resulting supernatants were collected and dried in a centrifugal vacuum evaporator for 18 h.
Metabolomic analysis
The identification and quantitation of metabolites were performed by LC–MS/MS analyses using UHPLC on a Thermo Scientific UltiMate Dionex 3000 RSLC HPF system (Thermo Fisher Scientific, U.S.) with a UPLC BEH Amide column (2.1 mm × 100 mm, 1.7 µm) coupled to Q-Exactive Orbitrap (Thermo Fisher Scientific, USA). The instrument operated at a 60,000 resolution with a full mass scan ranging from 67 to 1,000 m/z. The mobile phase consisted of 25 mmol/L ammonium acetate plus an aqueous solution of 25 ammonia hydroxide (pH = 9.75) (A), and acetonitrile (B) was used to conduct chromatographic separation at 3 µl/min at 4°C.
Under the control of acquisition software (Xcalibur, Thermo Fisher Scientific), a QE HFX mass spectrometer was used to acquire MS/MS spectra in information-dependent acquisition (IDA) mode. The parameters are detailed as follows: sheath gas flow rate=30 Arb, aux gas flow rate=25 Arb, capillary temperature=350°C, full MS resolution=60,000, MS/MS resolution=7,500, and collision energy=10/30/60 in NCE mode. All the serum samples were complementarily analyzed in positive ion mode (+3.6 kV) and negative ion mode (−3.2 kV) in metabolic profiling studies. The metabolites were identified on the basis of a detected chromatographic peak with an associated retention time and a unique accurate m/z for UHPLC. MetaboScape 3.0 software was used for UHPLC data analyses including peak extraction, denoising, and normalization.
Statistical analysis
SIMCA software (V16.0.2, Sartorius Stedim Data Analytics AB, Umea, Sweden) was employed to perform logarithmic (LOG) and centralization (CTR) conversions on the data. Then, principal component analysis (PCA) and orthogonal projections to latent structures-discriminant analysis (OPLS-DA) were conducted to estimate the classification and validate grouping trends in different groups. A 200-iter permutation test for the OPLS-DA model was implemented to prevent overfitting. The intercept of R2 on the Y-axis was less than 0.4 and that of Q2 on the Y-axis was less than 0, indicating that the model was not overfitting with good robustness.
A paired t-test was conducted to compare the differences in metabolites between the pre- and postoperation groups. Differences in metabolites between CRC patients and healthy volunteers were compared with the unpaired Student's t-test. The VIP value represents the overall contribution and importance of each X-variable. A VIP value of <1 was considered significant. A p-value of <0.05 was considered statistically significant. MetaboAnalyst 4.0 (http://www.metaboanalyst.ca/MetaboAnalyst/) was used for pathway analysis and enrichment analysis based on the Kyoto Encyclopedia of Genes and Genomes (KEGG, http://www.genome.jp/kegg/) database and Small Molecule Pathway Database (SMPDB, http://smpdb.ca/) to further explore the metabolic pathways involved in the change in metabolites. iPath 3.0 (http://pathways.embl.de/) was used for the visualization of the crucial metabolites and significant metabolic pathways.
Results
Characteristics of participants
The population demographics and clinical characteristics of our study subjects are presented in Table 1, and these are sex, age, BMI value, level of serum CEA, and cancer location. The mean age was 60.79 years for patients with CRC and 61.52 years for controls (p = 0.648). There was also no difference in BMI or sex between CRC patients and healthy controls (p = 0.214, 0.88). In all 67 patients, the diagnosis of colon carcinoma was confirmed by a histopathological examination in 32 patients, and rectal carcinoma was confirmed in 35 patients. The mean value of CEA was 5.91 mmol/L for CRC patients.
Metabolic profiling of colorectal cancer patients
A total of 180 metabolites were detected by UHPLC. PCA and OPLS-DA were used to perform discriminative pattern recognition analysis for the metabolite data. PCA is an unsupervised projection method that converts a set of observed potentially correlated variables into linearly unrelated variables by orthogonal transformation. In our research, PCA revealed that the metabolomic characteristics between the pre- and postoperation groups were significantly different (Figure 1A). OPLS-DA score plots also displayed a well-distinguished and clustered pattern between pre- and postoperative patients (Figure 1B). The permutation test verified the validity of the OPLS-DA model with all permuted Q2 and R2 values lower than the original values, and the Q2 (cum) intercepted the Y-axis at −1.23 (Figure 1C).
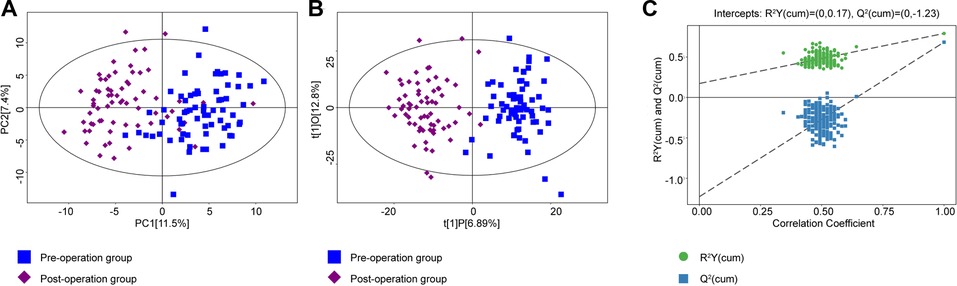
Figure 1. (A) PCA was conducted between the preoperation group (blue dots) and postoperation group (purple dots). (B) The OPLS-DA model was constructed using data from 67 CRC patients' serum metabonomics before (blue) and after (purple) surgery. (C) The permutation test plot of OPLS-DA (green, the value of R2Y; blue, the value of Q2; permutation test with 200 times, a p value of CV-ANOVA < 0.01). PCA, principal component analysis; OPLS-DA, orthogonal projections to latent structures-discriminant analysis; CRC, colorectal cancer.
Identification of different metabolites
Based on the variable importance of the projection (VIP) threshold and p values in the paired-samples t-test for metabolites identified through the OPLS-DA model that we established, 46 differentially expressed metabolites were screened between pre- and postoperation groups with the criteria of VIP > 1 and p values <0.05. The log fold change values represent the difference between the two groups. Thirty-two metabolites with log fold change values <0 were downregulated, while 14 metabolites with log fold change values >0 were upregulated in postoperative patients compared with those in preoperative patients. The expression levels of these metabolites are presented in the heat map (Figure 2A). In addition, the correlations of these differentiated metabolites are declared by chord analysis in Figure 2B.
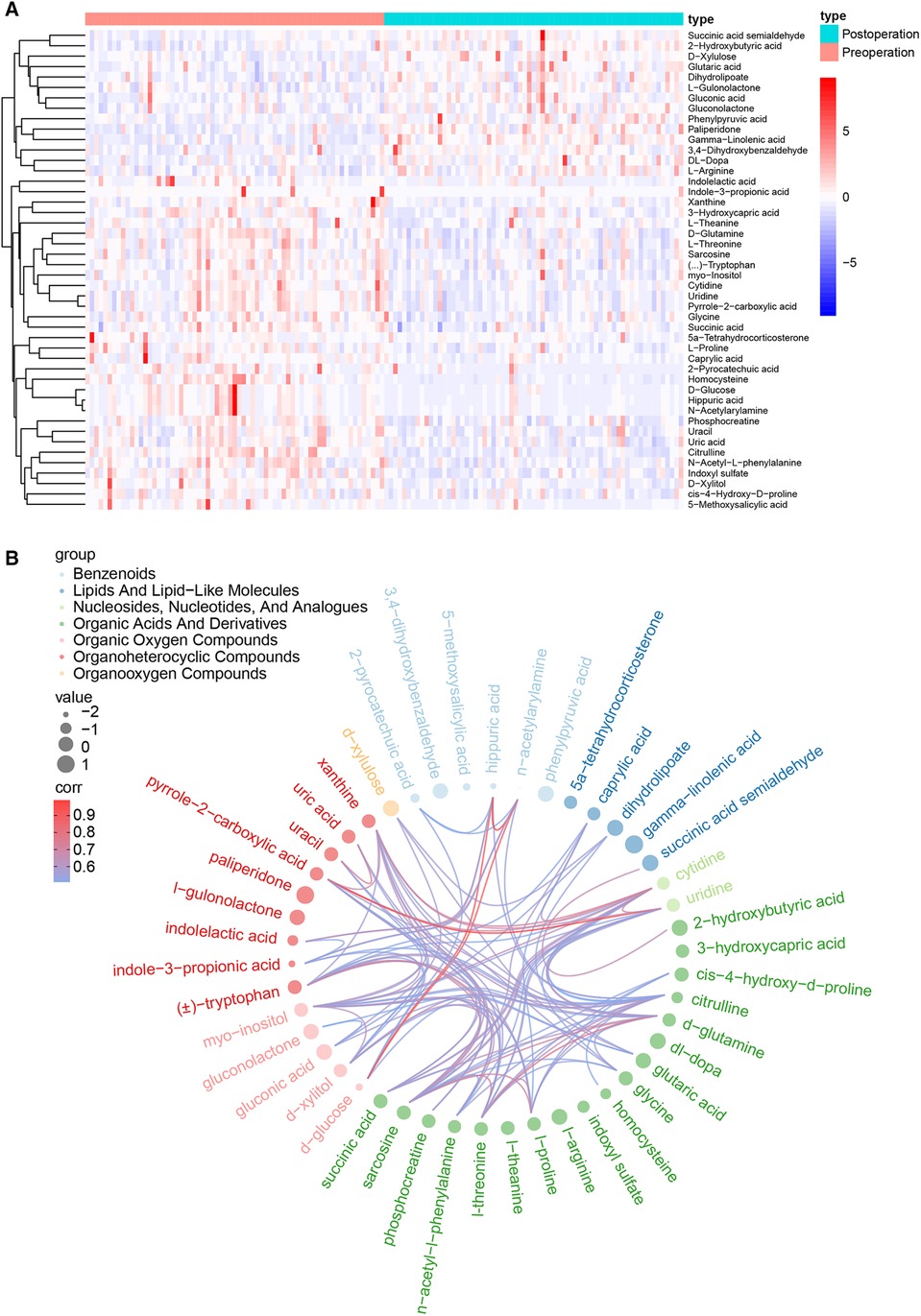
Figure 2. (A) The heat map for the 46 critical metabolites between the pre- and postoperation groups, including 32 downregulated metabolites and 14 upregulated metabolites. (B) The correlation network of the 46 differentially expressed metabolites.
Metabolic pathway analysis
Next, pathway analysis was conducted on the basis of the KEGG database for the differentially expressed metabolites. The metabolic pathways potentially associated with these 46 differential metabolites were screened and are presented in Table 2 and Figure 3A, including arginine and proline metabolism, ascorbate and aldarate metabolism, and phenylalanine metabolism (p = 0.0149, 0.0165, and 0.0256). In addition, the enrichment analysis module of MetaboAnalyst 4.0 based on the metabolite sets from SMPDB was used to strengthen the understanding of the altered metabolic pathways and validate the correlation of arginine and proline metabolism with radical resection of CRC (Figure 3B, Supplementary Table S1, p = 0.0272). The metabolic network of the differential metabolites and altered metabolic pathways in the KEGG general metabolic pathway map is shown in Figure 3C.
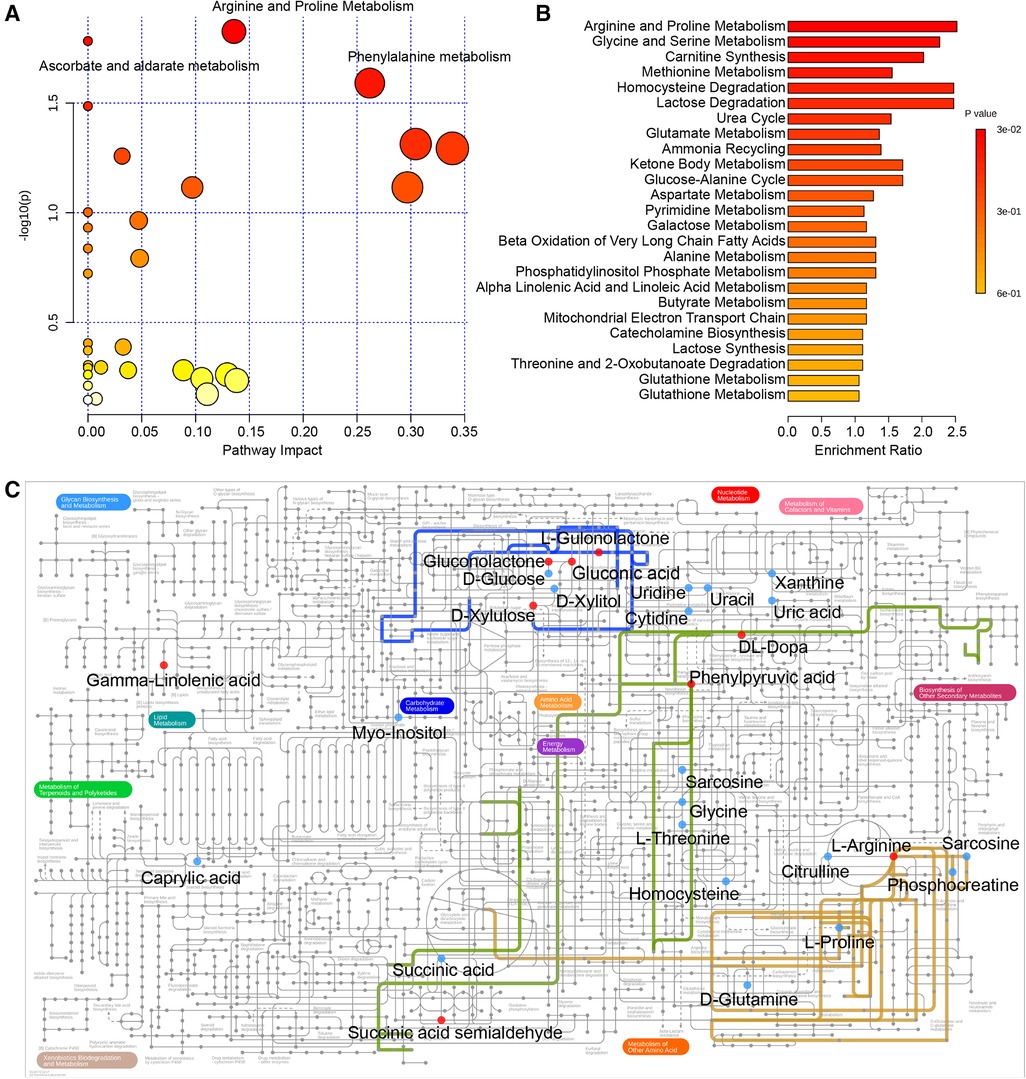
Figure 3. (A) Pathway analysis based on the KEGG database. (B) Enrichment analysis based on SMPDB. (C) Metabolic network of the crucial metabolites and significant metabolic pathways in the KEGG general metabolic pathway map. The following colored dots and lines denote the following: red dots, increased metabolites after surgery; blue dots, decreased metabolites after surgery; blue line, ascorbate and aldarate metabolism; yellow line, arginine and proline metabolism; green line, phenylalanine metabolism. KEGG, Kyoto Encyclopedia of Genes and Genomes; SMPDB, Small Molecule Pathway Database.
Identification of the significant metabolites in colorectal cancer
By combining the serum metabolites in the control group, we identified significantly altered metabolites for both the preoperative group vs. the postoperative group and the preoperative group vs. the control group. Interestingly, there was one metabolite, gamma-linolenic acid (GLA), which was evidently decreased in the preoperative CRC patients compared with that in healthy volunteers, and then returned to the healthy group level after the operation (Figure 4). Ultimately, we selectively compared the expression levels of metabolites involved in the metabolic pathways associated with radical resection of CRC. In the process of arginine and proline metabolism, l-arginine in the postoperation group was increased, while L-proline, cis-4-hydroxy-d-proline, and phosphocreatine in the postoperation group were decreased compared with those in the healthy control group. The serum levels of cis-4-hydroxy-d-proline and phosphocreatine in CRC patients before hydroxy were significantly lower than those in healthy volunteers. In the metabolic pathways of ascorbate and aldarate, the preoperative myo-orthosito was significantly lower than that of healthy volunteers and higher than that of postoperative patients, while the postoperative l-gulono-1,4-lactone was significantly higher than that of healthy volunteers without any difference between the preoperative and healthy groups. Among the phenylalanine metabolism pathways, the postoperative phenylpyruvate was increased, while postoperative hippurate was prominently decreased compared with that of healthy volunteers.
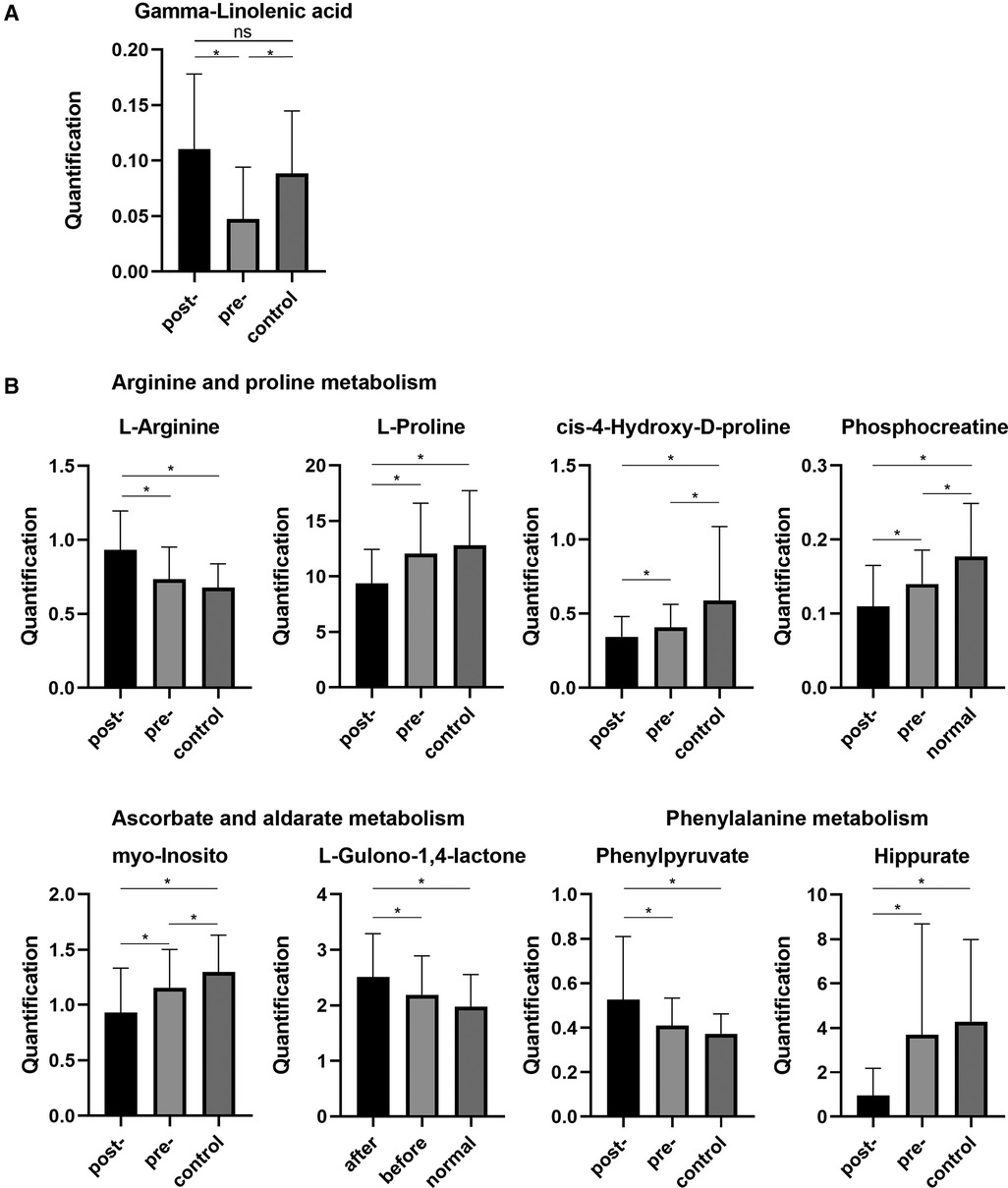
Figure 4. (A) Serum levels of GLA in healthy samples and preoperative and postoperative samples. (B) The expression levels of metabolites involved in the metabolic pathways associated with radical resection of CRC. GLA, gamma-linolenic acid. CRC, colorectal cancer. *p < 0.05. ns, not significant.
Discussion
In this study, we used UHPLC to conduct serum untargeted metabolomic analysis for CRC patients and revealed the characteristic differences in the metabolomic profiles in CRC patients before and after surgery. Furthermore, the significant metabolic pathways related to CRC were also identified.
Our results showed that the metabolic profiles of arginine and proline were significantly altered in the serum of patients before and after surgery, among which, l-proline, cis-4-hydroxy-d-proline, and cis-4-hydroxy-d-proline were significantly downregulated after surgery. However, l-arginine was upregulated after surgery. As a nonessential amino acid, proline is the only proteinogenic secondary amino acid with an α amino group within the pyrrolidine ring (13). Proline is an important product of glutamine, which can be converted to proline via pyrroline-5-carboxylate/glutamate-γ-semialdehyde (P5C/GSA) (14). Proline and P5C are interconvertibly catalyzed by proline dehydrogenase/proline oxidase (PRODH/POX) and PYCRs, which play a role in maintaining redox homeostasis between the cytosol and the mitochondria, and contribute to the recycling of cellular NAD(P)H to NAD(P). Recently, studies have focused on the conducive role of proline for metabolic reprogramming of cancer and its clinical significance (14–16). Two well-recognized oncogenes, namely, c-MYC and phosphoinositide 3-kinase (PI3K), robustly upregulate the proline biosynthetic pathway by increasing its enzymes (P5CS, PYCR1/2/L) (13). Meanwhile, proline catabolism involving PRODH/POX, which is regulated by P53, peroxisome proliferator-activated receptor γ (PPARγ), and AMP-activated protein kinase (AMPK), can also initiate ROS-mediated senescence, apoptosis, and autophagy in tumors. The process of proline synthesis has been demonstrated to be necessary for tumor cell growth, and an increased level of proline synthesis has been observed in breast metastatic cancer cells, melanoma cells, and ovarian cancer stem cells (14). In addition, in lung cancer cell lines expressing high levels of endogenous MYC and proline synthetic enzymes, a knockdown of proline enzymes or MYC could significantly inhibit tumor growth. Supplementation with proline or P5C partly rescued the decrease in proliferation induced by a knockdown of P5CS or PYCR1/2. A high expression of PYCR1 was also significantly associated with poor prognostic molecular subtypes of breast cancer, which is consistent with our results of downregulated proline levels post operation compared with that of the preoperative period. However, its underlying mechanisms remain unclear. Here, it deserves mention that, not necessarily due to the disruption of protein synthesis, the proline metabolic pathway also functions to regulate redox-dependent signaling through parametabolic mechanisms. In tumors, proline for protein synthesis is lower than that in adjacent normal tissues. On the other hand, proline is also indispensable for tumors to help manage stress during tumorigenic growth (13). This might explain why there were no differences in proline levels in our results between samples from preoperative patients and healthy people.
It is generally accepted that ascorbate has a potential therapeutic effect on tumors by generating sustainable ascorbate radical and H2O2 in extracellular fluid (17). However, the underlying mechanisms for the selective cytotoxicity to cancer cells but not to normal ascorbate cells are not well understood. Recent research showed that high-dose intravenous ascorbate as an adjuvant could improve chemosensitivity and relieve the toxicity of chemotherapy for ovarian cancer, which was related to an activation of the ATM/AMPK pathway and inhibition of mTOR signaling due to DNA damage and ATP depletion triggered by ascorbate-generated ROS (18). In addition, another study reported that some genes involved in ascorbate and aldarate metabolism were overexpressed in adenocarcinoma compared with those in normal tissues (19). It has also been reported that ascorbate and aldarate metabolism are significantly disordered in renal cell carcinoma (20). In CRC, intravenous ascorbate is associated with the inhibition of tumor glycolysis, thus advancing tumor regression and improving the tolerance and side effects of chemotherapy (21, 22). However, the impact of surgical resection of CRC tumors on ascorbate and aldarate metabolism has not been studied. We found that the levels of compounds involved in ascorbate and aldarate metabolism increased following surgery, which may play a potential role in inhibiting tumor recurrence and may be a potential factor that improves the prognosis of CRC.
We also noticed that phenylalanine metabolism changed after the operation. Previous studies have shown the significant differences in serum phenylalanine levels between gastroesophageal cancer patients and healthy volunteers, which may be accounted for by the dysfunction of phenylalanine hydroxylase activity in inflammatory or malignant disease states (23–25). In some tumors such as melanoma, hepatocarcinoma, leukemia, and breast cancer, lowering the serum levels of tyrosine and phenylalanine could inhibit the growth and metastases of tumors and improve the response to chemotherapy. A low tyrosine and phenylalanine diet might be correlated with the stabilization and regression of cancer (26). However, the underlying mechanism remains unclear. In our research, instead of serum levels of phenylalanine or tyrosine, significant changes in phenethylamine and hippurate levels were observed compared with those before surgery. Even so, we think the elevated phenylpyruvate levels can also indicate the reduced activity of phenylalanine hydroxylase in patients with CRC, which may suggest that it is necessary to control and monitor serum phenylalanine levels after surgery.
GLA, as a polyunsaturated fatty acid (PUFA), is an intermediate product in the process of linoleic acid (all cis-6,9-octadecadienoic acid) metabolism (27). Recently, GLA has gained importance due to its antifibrotic, anti-inflammatory, and tumoricidal properties with little or no side effects. Previous studies have reported that low GLA levels are associated with the progression of inflammatory breast cancer (28). In mammary gland carcinoma induced by 7,12-dimethylbenz (a) anthracene (DMBA), GLA has been proven to activate the mitochondrial-mediated death apoptosis pathway, regulate hypoxia-induced cell signaling, and decrease the synthesis of de novo fatty acids fatty acid to execute its antitumor effect by mediating the cholinergic anti-inflammatory pathway (29). In addition, GLA has been reported to decrease the expression of the metastasis-associated protein osteonectin (or SPARC) and increase the expression of metastasis suppressor genes to inhibit tumor metastasis. GLA could also decrease the β-catenin expression of Wnt/b-catenin signaling pathway to inhibit gastric cancer cell growth and EMT induced by hypoxia (30). Additionally, polyunsaturated fatty acids, including GLA, could induce an apoptosis of colon cancer cells (31). However, further research is needed to determine the impact of GLA on CRC and its underlying mechanism. In our results, the preoperative serum GLA levels of CRC patients were significantly lower than those of the normal control group, which is consistent with the tendency described in previous studies. Interestingly, we observed that postoperative serum GLA levels in CRC patients were significantly elevated and approached the preoperative level, highlighting the potential role of GLA as an effective biomarker for CRC to help predict prognosis following surgery and detect postoperative recurrence.
Although we found that CRC surgery resection could bring about changes in some metabolic pathways, including arginine and proline metabolism, phenylalanine metabolism, and ascorbate and aldarate metabolism, there is no evidence in serum metabolomics that surgery can reverse the metabolic reprogramming caused by CRC. In addition, limited by the sample size, we could not group tumors according to their clinical stage, which might have effects on serum metabolites. In future studies, larger sample sizes and longer follow-up periods are necessary to explore the metabolomic profile associated with CRC and confirm its prognostic value. Furthermore, metabolomic information from CRC patients with adjuvant chemotherapy is needed to analyze differences in the sensitivity of different metabolomic characteristics to chemotherapy and the target metabolites for adjuvant treatments.
Conclusion
In summary, our study established a serum metabolomic signature associated with CRC surgery resection and explored the physiological alterations in CRC patients after surgery. The results suggested that removal of the tumor may affect the metabolic profiling of organisms caused by CRC, which provides insights into the scheme for postoperative nutritional support and postoperative adjuvant therapy. In addition, we identified the diagnostic and prognostic value of GLA as a novel CRC effective biomarker that can monitor treatment efficacy and tumor recurrence.
Data availability statement
The raw data supporting the conclusions of this article will be made available by the authors without undue reservation.
Ethics statement
The studies involving human participants were reviewed and approved by the Ethics Committee of Peking Union Medical College Hospital. The patients/participants provided their written informed consent to participate in this study. Written informed consent was obtained from the individuals for the publication of any potentially identifiable images or data included in this article.
Author contributions
FZ and XB contributed to the design of this study and data analysis, drafted the article, and revised the article. All authors contributed to the acquisition of the data and to the draft and revised versions of the article. All authors agreed on the journal to which the article will be submitted and gave final approval of the version to be published. All authors contributed to the article and approved the submitted version.
Funding
This study was funded by the Technology Cooperation Programme of Xinjiang Production and Construction Corps (2020BC010).
Acknowledgments
We gratefully thank all the subjects who participated in this study and acknowledge the support of Biotree Biomedical Technology Co.
Conflict of interest
The authors declare that the research was conducted in the absence of any commercial or financial relationships that could be construed as a potential conflict of interest.
Publisher's note
All claims expressed in this article are solely those of the authors and do not necessarily represent those of their affiliated organizations, or those of the publisher, the editors and the reviewers. Any product that may be evaluated in this article, or claim that may be made by its manufacturer, is not guaranteed or endorsed by the publisher.
Supplementary material
The Supplementary Material for this article can be found online at: https://www.frontiersin.org/articles/10.3389/fsurg.2022.913967/full#supplementary-material.
References
1. Sung H, Ferlay J, Siegel RL, Laversanne M, Soerjomataram I, Jemal A, et al. Global cancer statistics 2020: GLOBOCAN estimates of incidence and mortality worldwide for 36 cancers in 185 countries. CA Cancer J Clin. (2021) 71(3):209–49. doi: 10.3322/caac.21660
2. Punt CJ, Koopman M, Vermeulen L. From tumour heterogeneity to advances in precision treatment of colorectal cancer. Nat Rev Clin Oncol. (2017) 14(4):235–46. doi: 10.1038/nrclinonc.2016.171
3. Choi JH, Jang TY, Jeon SE, Kim JH, Lee CJ, Yun HJ, et al. The small-molecule Wnt inhibitor ICG-001 efficiently inhibits colorectal cancer stemness and metastasis by suppressing MEIS1 expression. Int J Mol Sci. (2021) 22(24):13413. doi: 10.3390/ijms222413413
4. Esposito K, Chiodini P, Colao A, Lenzi A, Giugliano D. Metabolic syndrome and risk of cancer: a systematic review and meta-analysis. Diabetes Care. (2012) 35(11):2402–11. doi: 10.2337/dc12-0336
5. Johnson CH, Ivanisevic J, Siuzdak G. Metabolomics: beyond biomarkers and towards mechanisms. Nat Rev Mol Cell Biol. (2016) 17(7):451–9. doi: 10.1038/nrm.2016.25
6. Rinschen MM, Ivanisevic J, Giera M, Siuzdak G. Identification of bioactive metabolites using activity metabolomics. Nat Rev Mol Cell Biol. (2019) 20(6):353–67. doi: 10.1038/s41580-019-0108-4
7. Yang QJ, Zhao JR, Hao J, Li B, Huo Y, Han YL, et al. Serum and urine metabolomics study reveals a distinct diagnostic model for cancer cachexia. J Cachexia Sarcopenia Muscle. (2018) 9(1):71–85. doi: 10.1002/jcsm.12246
8. Jang C, Chen L, Rabinowitz JD. Metabolomics and isotope tracing. Cell. (2018) 173(4):822–37. doi: 10.1016/j.cell.2018.03.055
9. Li J, Li J, Wang H, Qi LW, Zhu Y, Lai M. Tyrosine and glutamine–leucine are metabolic markers of early-stage colorectal cancers. Gastroenterology. (2019) 157(1):257–259.e5. doi: 10.1053/j.gastro.2019.03.020
10. Zhang A, Sun H, Yan G, Wang P, Han Y, Wang X. Metabolomics in diagnosis and biomarker discovery of colorectal cancer. Cancer Lett. (2014) 345(1):17–20. doi: 10.1016/j.canlet.2013.11.011
11. Zhang C, Zhou S, Chang H, Zhuang F, Shi Y, Chang L, et al. Metabolomic profiling identified serum metabolite biomarkers and related metabolic pathways of colorectal cancer. Dis Markers. (2021) 2021:6858809. doi: 10.1155/2021
12. Hashiguchi Y, Muro K, Saito Y, Ito Y, Ajioka Y, Hamaguchi T, et al. Japanese society for Cancer of the Colon and rectum. Japanese Society for Cancer of the Colon and Rectum (JSCCR) guidelines 2019 for the treatment of colorectal cancer. Int J Clin Oncol. (2020) 25(1):1–42. doi: 10.1007/s10147-019-01485-z
13. Phang JM. Proline metabolism in cell regulation and cancer biology: recent advances and hypotheses. Antioxid Redox Signal. (2019) 30(4):635–49. doi: 10.1089/ars.2017.7350
14. Liu W, Hancock CN, Fischer JW, Harman M, Phang JM. Proline biosynthesis augments tumor cell growth and aerobic glycolysis: involvement of pyridine nucleotides. Sci Rep. (2015) 5:17206. doi: 10.1038/srep17206
15. Elia I, Broekaert D, Christen S, Boon R, Radaelli E, Orth MF, et al. Proline metabolism supports metastasis formation and could be inhibited to selectively target metastasizing cancer cells. Nat Commun. (2017) 8:15267. doi: 10.1038/ncomms15267
16. Sahu N, Dela Cruz D, Gao M, Sandoval W, Haverty PM, Liu J, et al. Proline starvation induces unresolved ER stress and hinders mTORC1-dependent tumorigenesis. Cell Metab. (2016) 24(5):753–61. doi: 10.1016/j.cmet.2016.08.008
17. Kouakanou L, Peters C, Brown CE, Kabelitz D, Wang LD. Vitamin C, from supplement to treatment: a re-emerging adjunct for cancer immunotherapy? Front Immunol. (2021) 12:765906. doi: 10.3389/fimmu.2021.765906
18. Ma Y, Chapman J, Levine M, Polireddy K, Drisko J, Chen Q. High-dose parenteral ascorbate enhanced chemosensitivity of ovarian cancer and reduced toxicity of chemotherapy. Sci Transl Med. (2014) 6(222):222ra18. doi: 10.1126/scitranslmed.3007154
19. Liu CC, Chen WS, Lin CC, Liu HC, Chen HY, Yang PC, et al. Topology-based cancer classification and related pathway mining using microarray data. Nucleic Acids Res. (2006) 34(14):4069–80. doi: 10.1093/nar/gkl583
20. Atrih A, Mudaliar MA, Zakikhani P, Lamont DJ, Huang JT, Bray SE, et al. Quantitative proteomics in resected renal cancer tissue for biomarker discovery and profiling. Br J Cancer. (2014) 110(6):1622–33. doi: 10.1038/bjc.2014.24
21. Tian W, Wang Z, Tang NN, Li JT, Liu Y, Chu WF, et al. Ascorbic acid sensitizes colorectal carcinoma to the cytotoxicity of arsenic trioxide via promoting reactive oxygen species-dependent apoptosis and pyroptosis. Front Pharmacol. (2020) 11:123. doi: 10.3389/fphar.2020.00123
22. El Halabi I, Bejjany R, Nasr R, Mukherji D, Temraz S, Nassar FJ, et al. Ascorbic acid in colon cancer: from the basic to the clinical applications. Int J Mol Sci. (2018) 19(9):2752. doi: 10.3390/ijms19092752
23. Neurauter G, Grahmann AV, Klieber M, Zeimet A, Ledochowski M, Sperner-Unterweger B, et al. Serum phenylalanine concentrations in patients with ovarian carcinoma correlate with concentrations of immune activation markers and of isoprostane-8. Cancer Lett. (2008) 272(1):141–7. doi: 10.1016/j.canlet.2008.07.002
24. Ploder M, Neurauter G, Spittler A, Schroecksnadel K, Roth E, Fuchs D. Serum phenylalanine in patients post trauma and with sepsis correlate to neopterin concentrations. Amino Acids. (2008) 35(2):303–7. doi: 10.1007/s00726-007-0625-x
25. Wiggins T, Kumar S, Markar SR, Antonowicz S, Hanna GB. Tyrosine, phenylalanine, and tryptophan in gastroesophageal malignancy: a systematic review. Cancer Epidemiol Biomarkers Prev. (2015) 24(1):32–8. doi: 10.1158/1055-9965.EPI-14-0980
26. Harvie MN, Campbell IT, Howell A, Thatcher N. Acceptability and tolerance of a low tyrosine and phenylalanine diet in patients with advanced cancer—a pilot study. J Hum Nutr Diet. (2002) 15(3):193–202. doi: 10.1046/j.1365-277X.2002.00365.x
27. Kapoor R, Huang YS. Gamma linolenic acid: an antiinflammatory omega-6 fatty acid. Curr Pharm Biotechnol. (2006) 7(6):531–4. doi: 10.2174/138920106779116874
28. Chas M, Goupille C, Arbion F, Bougnoux P, Pinault M, Jourdan ML, et al. Low eicosapentaenoic acid and gamma-linolenic acid levels in breast adipose tissue are associated with inflammatory breast cancer. Breast. (2019) 45:113–7. doi: 10.1016/j.breast.2019.04.001
29. Roy S, Singh M, Rawat A, Devi U, Gautam S, Yadav RK, et al. GLA supplementation regulates PHD2 mediated hypoxia and mitochondrial apoptosis in DMBA induced mammary gland carcinoma. Int J Biochem Cell Biol. (2018) 96:51–62. doi: 10.1016/j.biocel.2018.01.011
30. Wang Y, Shi J, Gong L. Gamma linolenic acid suppresses hypoxia-induced gastric cancer cell growth and epithelial-mesenchymal transition by inhibiting the Wnt/b-catenin signaling pathway. Folia Histochem Cytobiol. (2020) 58(2):117–26. doi: 10.5603/FHC.a2020.0012
Keywords: colorectal cancer, metabolomic profiling, tumorectomy, gamma-linolenic acid (GLA), proline, ascorbate, phenylalanine
Citation: Zhuang F, Bai X, Shi Y, Chang L, Ai W, Du J, Liu W, Liu H, Zhou X, Wang Z and Hong T (2022) Metabolomic profiling identifies biomarkers and metabolic impacts of surgery for colorectal cancer. Front. Surg. 9:913967. doi: 10.3389/fsurg.2022.913967
Received: 6 April 2022; Accepted: 18 July 2022;
Published: 25 August 2022.
Edited by:
Kee Yuan Ngiam, National University Health System (Singapore), SingaporeReviewed by:
Anurag Srivastava, National Institute of Cancer Prevention and Research (ICMR), IndiaJun Cao, Affiliated Hospital of Yangzhou University, China
Mingwei Gao, Second Affiliated Hospital of Dalian Medical University, China
© 2022 Zhuang, Bai, Shi, Chang, Ai, Du, Liu, Liu, Zhou, Wang and Hong. This is an open-access article distributed under the terms of the Creative Commons Attribution License (CC BY). The use, distribution or reproduction in other forums is permitted, provided the original author(s) and the copyright owner(s) are credited and that the original publication in this journal is cited, in accordance with accepted academic practice. No use, distribution or reproduction is permitted which does not comply with these terms.
*Correspondence: Tao Hong aHQ3MTA4MTJAc29odS5jb20=
†These authors have contributed equally to this work and share first authorship
Specialty Section: This article was submitted to Surgical Oncology, a section of the journal Frontiers in Surgery
Abbreviations CRC, colorectal cancer; UHPLC, ultra-high-performance liquid chromatography; CEA, carcinoma embryonic antigen; BMI, body mass index; PCA, principal component analysis; OPLS-DA, orthogonal projections to latent structures-discriminant analysis; KEGG, Kyoto Encyclopedia of Genes and Genomes; SMPDB, Small Molecule Pathway Database; VIP, variable importance in projection; GLA, gamma-linolenic acid; P5C/GSA, pyrroline-5-carboxylate/glutamate-γ-semialdehyde; PYCRs, P5C reductases; PRODH/POX, proline dehydrogenase/proline oxidase; AMPK, AMP-activated protein kinase.