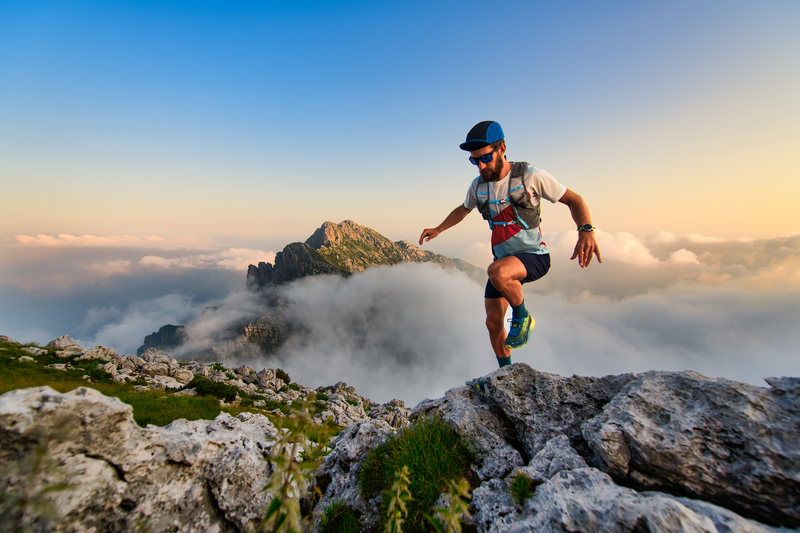
95% of researchers rate our articles as excellent or good
Learn more about the work of our research integrity team to safeguard the quality of each article we publish.
Find out more
ORIGINAL RESEARCH article
Front. Surg. , 19 August 2022
Sec. Surgical Oncology
Volume 9 - 2022 | https://doi.org/10.3389/fsurg.2022.903271
Purpose: Oral squamous cell carcinoma (OSCC) is the most common oral cancer worldwide. Pyroptosis is a type of programmed cell death mediated by caspase, accompanied by an inflammatory response, and plays an important role in cancer progression. The purpose of this study was to explore and identify potential biomarkers and further elucidate the potential role of cell pyroptosis in OSCC.
Methods: We regarded the samples from The Cancer Genome Atlas database as a training dataset, screened differentially expressed genes (DEGs), and further screened out OSCC phenotypic characteristic genes by using weighted gene co-expression network analysis. The analysis of 42 known pyroptosis-related genes showed that Psuch genes were widely expressed, mutated, and methylated in OSCC samples.
Results: Through correlation analysis, we identified our OSCC pyroptosis-related DEGs. To further evaluate the prognostic value of pyroptosis-related regulators, we constructed a seven gene-based prognostic signature using Cox univariate analysis and least absolute shrinkage and selection operator Cox regression analysis. Meanwhile, we found that patients in the low-risk group had higher immune infiltration. Moreover, our results also indicated significant differences in sensitivity to cisplatin and gefitinib between the high-risk and low-risk groups.
Conclusion: Our study successfully constructed the pyroptosis-related prognostic signature, which might play a potential prediction role in OSCC prognosis. Our findings also suggested that pyroptosis-related regulators might be novel biomarkers for tumor diagnosis and treatment in OSCC.
Oral squamous cell carcinoma (OSCC) is a common malignant tumor type of oral cancer and brings huge health burden to society (1). According to the report of the World Health Organization (WHO), the annual incidence rate of OSCC worldwide is more than 300,000 cases globally (2). The prognosis of OSCC patients is poor, with an approximately 50% 5-year survival rate due to the limited understanding of molecular mechanisms (3). In the last few decades, although the technological and biological advances recently have been developed, the prognosis of this disease has not changed (4). Therefore, the identification of novel and efficient prognostic predictors is necessary and may guide personalized clinical treatment.
Pyroptosis is a programmed mode of cell death in the body's immune response, mediated by cysteine aspartate-specific proteases (caspase), accompanied by an inflammatory response, and has been reported to play an important role in cancer development (5, 6). Pyroptosis is thought to be a response to infection and is reported to be usually triggered by inflammasome (7). Increasing evidence revealed that pyroptosis could affect the overall survival of cancer patients by changing the immune infiltration levels in the tumor microenvironment (TME) (8). For example, Liang et al. constructed a prognostic model using pyroptosis-related genes and found that this model might efficiently predict the prognosis of gastric cancer patients (9). Cao et al. identified a series of long non-coding RNAs regulating the progression of pyroptosis and then established a pyroptosis-related lncRNA prognostic model for ovarian cancer (10). In addition, many reports confirmed that the pyroptosis level was significantly correlated to immunotherapy response in diverse types of human cancers such as glioma (11), pancreatic adenocarcinoma (12), and esophageal adenocarcinoma (13). These studies further determined the crucial value of pyroptosis in cancer, as well as the patient prognosis. However, the potential prognostic significance of pyroptosis in OSCC is still lacking and needs to be extended for guiding clinical directed treatment.
In the present study, we identified 278 potential regulators that are differentially expressed in OSCC, which are significantly associated with 42 pyroptosis genes by using the limma and weighted gene co-expression network analysis (WGCNA) function packages in R. The least absolute shrinkage and selection operator (LASSO) Cox regression model is a popular algorithm widely used in medical research for feature selection (14–17). Further, we integrated the LASSO Cox regression model analysis to narrow down the range of candidate genes and finally screened out seven pyroptosis-related regulators to construct a prognostic model. Seven pyroptosis-related regulators determined the efficiency and accuracy of the predictive model in both datasets, including training and validation sets.
Moreover, we also evaluated the immune infiltration levels between two risk groups and found that patients in the low-risk group had a stronger infiltration ratio of the microenvironment. Our results suggested that the prognostic model might guide the use of antineoplastic drugs such as gefitinib and cisplatin clinically.
The RNA FPKM expression profiles and clinical information of the training dataset (316 OSCC samples and 30 normal samples) were downloaded from the HNSCC cohort of The Cancer Genome Atlas (TCGA-HNSC). Meanwhile, similar information about the validation dataset (40 OSCC samples) was generated from the International Cancer Genome Consortium (ICGC). The original data is normalized by the log2(x+1) method, and the standardization was performed based on the robust multi-array method. Subsequently, the differential expression analysis was carried out by the limma function package of R language (18), with |log 2 [fold change (FC)]| > 1 and P ≤ 0.05 as the set.
The “WGCNA” function package of R language was used to perform the WGCNA as previously described (19). In brief, according to the gene expression value of genes, genes with high similarity were divided into the same module by the dynamic clipping tree method, and the module was identified. Next, the module eigengene (ME) value and the correlation coefficient between the ME value and phenotype of interest were calculated. Furthermore, phenotypes referred to disease states, specifically, under the significant correlation coefficient (P < 0.05); the larger the absolute value of ME, the closer the module to the benefit phenotype.
The 42 pyroptosis-related genes were obtained from previous studies and the GO:0070269 pathway (20–24), and they are presented in Supplementary Table S1. For 42 genes, Gene Ontology (GO) analysis (including biological process, molecular function, and cellular component) and Kyoto Encyclopedia of Genes and Genomes (KEGG) pathway enrichment analysis was conducted by the clusterProfiler function package of R language (25), with P < 0.05 as the significant threshold.
To screen out the key genes that associated with OSCC prognosis, univariate Cox regression analysis was applied by using the “survival” function of R language. Then, a prognosis model was constructed with these candidate genes. Risk score = Gene 1 * β1 + Gene 2 * β2 +… Gene n * βn. The β symbol represents the regression coefficient for each gene of interest obtained from the training dataset. Subsequently, we divided patients into high- and low-risk groups based on the median risk score. The prognostic effect was evaluated by using the time-dependent ROC curve using the “timeROC” function. In addition, the validation dataset was used to verify the accuracy of this prognostic signature.
XCELL in TIMER (http://timer.comp-genomics.org/) was used to evaluate the percentage of immune cell types, that is, the infiltration levels of diverse types of immune cells among OSCC samples as previously described (26). Further, the differences in immune cell invasion between two risk groups was assessed using SSGESA according to the previous studies (27).
To investigate whether patients with OSCC could benefit clinically from immunotherapy, chemotherapy combined with immunotherapy has been shown to be more effective than either alone. We investigated the correlation between risk score and clinical response to chemotherapeutic agents. pRRophetic is an R software package designed to assess clinical drug responses (the half-maximal inhibitory concentration, IC50) by integrating baseline gene expression levels and drug sensitivity data in cancer cell lines (1, 28).
R V4.1.0 (http://www.Rproject.org) was used to perform the statistical evaluation. The Wilcoxon test was used to compare the differences between the two groups. P < 0.05 was considered the significant threshold.
First, the differential expression analysis between OSCC samples and normal samples was performed, and we identified 2,956 differentially expressed genes (DEGs) (Figure 1A). To perform the WGCNA analysis, the soft threshold power analysis was used to obtain the scale-free fitting index of network topology (Figure 1B). Here, according to the WGCNA algorithm, the gene expression network was assumed to obey the scale-free distribution, and the gene co-expression network was constructed. Subsequently, we constructed the hierarchical clustering trees by calculating the dissimilarity coefficients of different nodes. In addition, we grouped high-similarity genes into the same module and low-similarity genes into different modules and visualized these modules. For WGCNA analysis of 2,956 DEGs, we set the soft threshold to 7 to construct a scale-free network (Figure 1C). Further, the adjacency matrix and topological overlap matrix were constructed and are shown in Figure 1D. Finally, seven modules were obtained based on average hierarchical clustering and dynamic tree clipping (Figure 1E). The MEs and Pearson correlation coefficients for disease status were calculated for all modules to determine which module was associated with OSCC, and we found that the blue module (R = 0.68, P = 3e-48) was significantly negatively correlated to disease status (Figure 1E). Scatter plot of correlation between the blue module most related to phenotype and genes is shown in Figure 1F. Hence, the 310 genes in the blue module were selected for the subsequent analysis.
Figure 1. Identification of phenotypic-specific differentially expressed genes (DEGs). (A) Differential gene volcano map between Oral squamous cell carcinoma (OSCC) samples and normal samples. (B) Soft threshold power analysis for generating the scale-free fitting index of network topology. (C) Hierarchical cluster analysis to detect co-expression clusters with corresponding color assignments. (D) Heat map depicting the topological overlap matrix between DEGs based on co-representation module (TOM). (E) Heat maps of correlations between modular signature genes and OSCC phenotypes. (F) Scatter plot of the correlation between blue module and genes most correlated to phenotype.
To assess the change of pyroptosis genes in OSCC, we downloaded the 42 pyroptosis-related genes from previous studies and the GO:0070269 pathway. As shown in Figures 2A,B, we found that the expression and methylation modification of 42 pyroptosis-related genes were significantly changed in OSCC samples compared to normal samples. Meanwhile, we observed the extensive mutations of pyroptosis-related genes in OSCC samples compared to that in normal samples (Figure 2C). Then, GO and KEGG enrichment analyses were carried out using these 42 pyroptosis-related genes, and the results revealed that these genes were mainly enriched in pyroptosis, interleukin-1 β production, interleukin-1 production, regulation of interleukin-1 β production, and other biological processes (Figure 2D) and also in the NOD-like receptor signaling pathway, legionellosis, Salmonella infection, pathogenic Escherichia coli infection, and other signaling pathways (Figure 2E).
Figure 2. Evaluation of pyroptosis genes in Oral squamous cell carcinoma. Expression heat map (A), methylation heat map (B), and top 30 mutant waterfall diagrams (C) of 42 pyroptosis-related genes in the training dataset. Gene Ontology analysis (D) and Kyoto Encyclopedia of Genes and Genomes pathway enrichment analysis (E) using 42 pyroptosis-related genes in the training dataset.
To explore which key genes might affect the process of pyroptosis and thus the occurrence and development of OSCC, we conducted the correlation analysis of 310 DEGs in the blue module and 42 pyroptosis-related genes and obtained a total of 278 key genes that might impact the pyroptosis-related genes (Figure 3A), suggesting that these genes might regulate the pyroapoptotic process of OSCC. The expression and methylation modification of 278 pyroptosis-related regulators were significantly changed in OSCS samples compared to those in normal samples (Figures 3B,D). PCA analysis showed that these 278 pyroptosis-related regulators could distinguish OSCC samples from normal samples (Figure 3C). Finally, by performing the Univariate Cox regression analysis combined with clinical information of patients, we screened out 13 key pyroptosis-related key regulators that are closely correlated to OSCC prognosis (Figure 3E). These genes were NFKBIL2, FAM72D, FAM72B, COL27A1, TAF1A, AURKAPS1, DDX12, CKS2, HIST1H3F, MAML3, LOC283314, FST, and MCEE.
Figure 3. Identification of pyroptosis-related key regulators. (A) Correlation network diagram of 278 pyroptosis-related key regulators and 42 pyroptosis-related genes. Expression heat map (B,C) and methylation heat map (D) of 278 pyroptosis-related key regulators in the training dataset. (E) PCA clustering of 278 pyroptosis-related key regulators in the training dataset. (F) Prognostic forest map of 13 pyroptosis-related signatures.
Then, we evaluated the prognostic values of these pyroptosis-related key regulators. Correlation analysis showed that there was an obvious expression correlation among the 13 pyroptosis-related key regulators (Figure 4A). Then, the penalty coefficient of LASSO regression was generated by using the glmnet function of R language, and we found that as lambda increases, the degrees of freedom and residuals decrease (Figure 4B). Genes with penalty factor 0 were reserved as the final screening variables (Figure 4C). Therefore, seven genes (FAM72D, COL27A1, HIST1H3F, MAML3, LOC283314, FST, and MCEE) were retained, and their corresponding penalty coefficients are given in Table 1. Subsequently, risk scores were the sum of the product of the penalty coefficient and expression value. Then, we set the median value of risk scores as the threshold and separated patients in the training dataset into high- and low-score groups; survival analysis revealed that patients in low-score groups had a better prognosis than that in high-score group (Figure 4D). Moreover, a similar method was applied to the validation dataset, and the same trend was observed (Figure 4E). Based on the clinical characteristics of univariate Cox risk regression combined with the training set and validation set, the results demonstrated that the risk score was a relative independent prognostic indicator considering the age, gender, grade, and stage in both the training dataset (Figure 4F) and validation dataset (Figure 4G).
Figure 4. Construction and validation of the prognostic model. (A) Correlation network diagram of 13 pyroptosis-related key regulators. (B) Parameter selection in least absolute shrinkage and selection operator (LASSO) regression. (C) Coefficient distribution in LASSO regression. Survival analysis based on risk scores in the training set (D) and validation dataset (D). (E) Univariate Cox risk regression considering other clinical factors in the training dataset. (F) Univariate Cox risk regression considering other clinical factors in the validation dataset.
Subsequently, we evaluated the difference in immune infiltration between high- and low-risk score groups and found that the levels of immune infiltration in the high-risk group were obviously lower than those in the low-risk group (Figure 5A). The correlation scatter plot also showed that the infiltration ratio of immune cells and stromal cells in the tumor microenvironment was negatively correlated to the prognostic of OSCC patients determined by our prognostic model. The higher the risk score, the lower the infiltration ratio of the microenvironment (Figures 5B–D). In addition, patients in the low-risk group showed a significant survival advantage due to the abundant infiltration of innate immune cells, including B cells, iDCs, TIL, and activated CD8 T cells (Figure 5E). Meanwhile, check-point, cytolytic_activity, MHC_class_I, T_cell_co-inhibition, and other immune functions exhibited higher levels in the low-risk group (Figure 5F).
Figure 5. Analysis of immune infiltration levels. (A) Comparison of immune microenvironments between high and low-risk groups. (B–D) Scatter plot of the correlation between total score, stromal cell score, immune cell score, and risk score in the microenvironment. (E) Difference between groups at high or low risk of Oral squamous cell carcinoma under different immune cell types. (F) Differences between groups at high and low risk of OSCC under different activated immune pathways.
Next, we analyzed the DEGs between high- and low-risk score groups= and identified 2,830 DEGs (Figure 6A). GO analysis revealed that these DEGs were closely enriched in mitotic nuclear division, centromeric region, ATP-dependent activity, acting on DNA biological processes (Figure 6B), and also enriched in DNA replication, ECM−receptor interaction, and mismatch repair signaling pathways (Figure 6C). To investigate whether patients with OSCC could benefit from immunotherapy clinically, we evaluated the risk model and chemotherapy response (cisplatin and gefitinib) using the pRRophetic function of R language and found that there were significant differences in sensitivity to cisplatin and gefitinib between high- and low-risk groups (Figure 6D). Finally, we conducted gene set enrichment analysis (GSEA) based on the DEGs between high- and low-risk groups to explore the drug resistance signaling pathway, and the results showed that the DEGs could affect the drug resistance of gefitinib, cisplatin, adriamycin, and other drugs (Figure 6E). These findings suggested that our model might guide the use of antineoplastic drugs clinically.
Figure 6. Evaluation of the association between the risk model and chemotherapy response. (A) Volcano map of DEGs in high- and low-risk groups in the training dataset. Gene Ontology analysis (B) and Kyoto Encyclopedia of Genes and Genomes pathway enrichment analysis (C) of DEGs in two risk groups in the training dataset. (D) Predicted differences in drug sensitivity between high- and low-risk groups. (E) GSEA analysis of differential genes in drug resistance pathways between high- and low-risk groups.
Oral cancer is becoming a global health problem due to its relatively high incidence and mortality. Also, due to the poor prognosis of OSCC after surgery combined with chemoradiotherapy (29, 30), increasing research studies have paid more attention to the identification of efficient prognostic predictors in OSCC such as microRNA levels (31), long non-coding RNAs (32), and apoptosis-related genes (33). Although the role of pyroptosis in human cancers have been studied, their prognostic values in many other malignancies have not been well characterized. Pyroptosis occurs in pathogen-infected cells as a manifestation of programmed cell death, which induces an inflammatory response in the body (34) and has diverse roles. Pyroptosis has tumor growth inhibitory effects on colorectal and skin cancers (35, 36); hence, we hypothesized that pyroptosis might also play a potential role in OSCC. In this study, our results systematically analyzed the expression of pyroptosis-related genes in OSCC samples and further identified a series of potential genes that regulated these genes. Then, a prognosis model was constructed based on seven optimized pyroptosis-related regulators, and our data determined that the prognostic model might be useful for predicting the prognosis of OSCC patients.
In the present study, seven pyroptosis-related regulatory genes (FAM72D, COL27A1, HIST1H3F, MAML3, LOC283314, FST, and MCEE) were finally selected for the construction of a prognostic model, and our data confirmed that the seven gene-based prognostic model could accurately predict the outcome of OSCC patients. The prognostic values of these seven key genes in OSCC or other human diseases have been investigated. For example, a previous study established a signature using nine hepatitis C virus-induced genes including FAM72D, which could better predict the prognosis of hepatic cancer and also provide a novel way to investigate the potential mechanism of HCVIGs in this disease (37). Hu et al. found the downregulation of COL27A1 in poor segmental congenital scoliosis (38), and Laura et al. revealed a steel syndrome patient due to the compound heterozygous COL27A1 mutations in the eye (39). Garciaz et al. found that the downregulation of the HIST1H3F level could predict a better prognosis in patients with acute myeloid leukemia (40). A recent study performed an integrated analysis and revealed that miR-20°c-3p and miR-52°c-3p could intermediate core-regulatory genes including MAML3 to affect stemness and metastasis in gastric cancer (41). Liu et al. demonstrated that the downregulated level of FST was significantly correlated to the poor survival of patients with triple-negative breast cancer (42). Tang et al. analyzed the genetic polymorphisms that affected pancreatic cancer survival and found that MCEE level was closely related to triple-negative breast cancer prognosis (43). Although the prognostic values of these seven pyroptosis-related regulatory genes in human cancers have been partially explored, their significance in OSCC remains unclear. Here, we investigated the prognostic values of the seven genes and revealed that this seven-gene-based prognostic model could be potentially used for clinical prediction of overall survival. Our findings might provide a new way for personalized treatment.
Gefitinib is a tyrosine kinase inhibitor with low molecular weight (44), and cisplatin is a cytotoxic, DNA-damaging alkylated chemotherapy drug (45). Increasing clinical applications have reported that these two drugs were widely used for the treatment of different types of solid tumors including OSCC (46). However, the efficiency of two drugs in tumors often depends on the tumor size, stage, and other clinical factors. Hence, a well understanding of chemotherapy response against different tumor types is more urgent, which might contribute to applying efficient chemotherapy drugs. In this study, there were significant differences in sensitivity to cisplatin and gefitinib between high- and low-risk groups. These findings might provide clinical medicine for OSCC.
In conclusion, we constructed a seven-gene-based prognostic signature based on seven pyroptosis-related regulators and even investigated the correlation between the risk model and chemotherapy response. The results might guide the use of antineoplastic drugs clinically. However, more patient data were needed to be collected for verifying the accuracy of our prognostic model.
Publicly available datasets were analyzed in this study. This data can be found here: The datasets used and/or analyzed during the current study available from the corresponding author on reasonable request.
DZ and XW did the literature research; DZ, XW, and SZ conducted data analysis. This work was performed by DZ and AZ. The manuscript was written by DZ and edited by QH and LC. All authors read and approved the final submission. All authors contributed to the article and approved the submitted version.
This work was jointly supported by the National Natural Science Foundation of China (31971273), the Natural Science Foundation of Shanghai Science and Technology Commission (21ZR1437100), and the Shanghai Rising-Star Program (21QA1405400).
The authors thank the financial support from the National Natural Science Foundation of China (31971273), the Natural Science Foundation of Shanghai Science and Technology Commission (21ZR1437100), and the Shanghai Rising-Star Program (21QA1405400). The authors gratefully acknowledge the Innovative Research Team of High-level Local Universities in Shanghai, Oral and Maxillofacial Regeneration and Functional Restoration.
The authors declare that the research was conducted in the absence of any commercial or financial relationships that could be construed as a potential conflict of interest.
All claims expressed in this article are solely those of the authors and do not necessarily represent those of their affiliated organizations, or those of the publisher, the editors and the reviewers. Any product that may be evaluated in this article, or claim that may be made by its manufacturer, is not guaranteed or endorsed by the publisher.
The Supplementary Material for this article can be found online at: https://www.frontiersin.org/articles/10.3389/fsurg.2022.903271/full#supplementary-material.
1. Geeleher P, Cox NJ, Huang RS. Clinical drug response can be predicted using baseline gene expression levels and in vitro drug sensitivity in cell lines. Genome Biol. (2014) 15:R47. doi: 10.1186/gb-2014-15-3-r47
2. Wang Q, Gao P, Wang X, Duan Y. Investigation and identification of potential biomarkers in human saliva for the early diagnosis of oral squamous cell carcinoma. Clin Chim Acta. (2014) 427:79–85. doi: 10.1016/j.cca.2013.10.004
3. Malik UU, Zarina S, Pennington SR. Oral squamous cell carcinoma: key clinical questions, biomarker discovery, and the role of proteomics. Arch Oral Biol. (2016) 63:53–65. doi: 10.1016/j.archoralbio.2015.11.017
4. Garajei A, Parvin M, Mohammadi H, Allameh A. Evaluation of the expression of miR-486-3p, miR-548-3p, miR-561-5p and miR-509-5p in tumor biopsies of patients with oral squamous cell carcinoma. Pathogens. (2022) 11:211. doi: 10.3390/pathogens11020211
5. Yang F, Bettadapura SN, Smeltzer MS, Zhu H, Wang S. Pyroptosis and pyroptosis-inducing cancer drugs. Acta Pharmacol Sin. (2022). doi: 10.1038/s41401-022-00887-6. [Epub ahead of print]
6. Wang S, Zhang MJ, Wu ZZ, Zhu SW, Wan SC, Zhang BX, et al. GSDME is related to prognosis and response to chemotherapy in oral cancer. J Dent Res. (2022) 101(7):848–58. doi: 10.1177/00220345211073072
7. Liu T, Hou M, Li M, Qiu C, Cheng L, Zhu T, et al. Pyroptosis: a developing foreland of ovarian cancer treatment. Front Oncol. (2022) 12:828303. doi: 10.3389/fonc.2022.828303
8. Niu X, Chen L, Li Y, Hu Z, He F. Ferroptosis, necroptosis, and pyroptosis in the tumor microenvironment: perspectives for immunotherapy of SCLC. Semin Cancer Biol. (2022) S1044-579X(22)00065-7. doi: 10.1016/j.semcancer.2022.03.009
9. Liang C, Fan J, Liang C, Guo J. Identification and validation of a pyroptosis-related prognostic model for gastric cancer. Front Genet. (2021) 12:699503. doi: 10.3389/fgene.2021.699503
10. Cao X, Zhang Q, Zhu Y, Huo X, Bao J, Su M. Derivation, comprehensive analysis, and assay validation of a pyroptosis-related lncRNA prognostic signature in patients with ovarian cancer. Front Oncol. (2022) 12:780950. doi: 10.3389/fonc.2022.780950
11. Fan T, Wan Y, Niu D, Wang B, Zhang B, Zhang Z, et al. Comprehensive analysis of pyroptosis regulation patterns and their influence on tumor immune microenvironment and patient prognosis in glioma. Discover. Oncology. (2022) 13:1–18. doi: 10.1007/s12672-022-00474-5
12. Wang J, Zhang H. Bioinformatic analysis of prognostic value of pyroptosis-related genes and its effect on immune cell infiltration in pancreatic adenocarcinoma. Int J Gen Med. (2022) 15:2311–9. doi: 10.2147/ijgm.s350959
13. Li GS, He RQ, Liu J, He J, Fu ZW, Yang LJ, et al. Prognostic signature of esophageal adenocarcinoma based on pyroptosis-related genes. BMC Med Genomics. (2022) 15:1–14. doi: 10.1186/s12920-022-01196-x
14. Cao R, Yuan L, Ma B, Wang G, Qiu W, Tian Y. An EMT-related gene signature for the prognosis of human bladder cancer. J Cell Mol Med. (2020) 24:605–17. doi: 10.1111/jcmm.14767
15. Zhang Y, Li H, Zhang W, Che Y, Bai W, Huang G. LASSO-based cox-PH model identifies an 11-lncRNA signature for prognosis prediction in gastric cancer. Mol Med Rep. (2018) 18:5579–93. doi: 10.3892/mmr.2018.9567
16. Liu Z, Xu H, Weng S, Ren Y, Han X. Stemness refines the classification of colorectal cancer with stratified prognosis, multi-omics landscape, potential mechanisms, and treatment options. Front Immunol. (2022) 13:828330. doi: 10.3389/fimmu.2022.828330
17. Liu Z, Lu T, Li J, Wang L, Xu K, Dang Q, et al. Development and clinical validation of a novel six-gene signature for accurately predicting the recurrence risk of patients with stage II/III colorectal cancer. Cancer Cell Int. (2021) 21:359. doi: 10.1186/s12935-021-02070-z
18. Ritchie ME, Phipson B, Wu D, Hu Y, Law CW, Shi W, et al. Limma powers differential expression analyses for RNA-sequencing and microarray studies. Nucleic Acids Res. (2015) 43:e47. doi: 10.1093/nar/gkv007
19. Langfelder P, Horvath S. WGCNA: an R package for weighted correlation network analysis. BMC Bioinformatics. (2008) 9:559. doi: 10.1186/1471-2105-9-559
20. Karki R, Kanneganti TD. Diverging inflammasome signals in tumorigenesis and potential targeting. Nat Rev Cancer. (2019) 19:197–214. doi: 10.1038/s41568-019-0123-y
21. Xia X, Wang X, Cheng Z, Qin W, Lei L, Jiang J, et al. The role of pyroptosis in cancer: pro-cancer or pro-“host”? Cell Death Dis. (2019) 10:1–13. doi: 10.1038/s41419-019-1883-8
22. Wang B, Yin Q. AIM2 Inflammasome activation and regulation: a structural perspective. J Struct Biol. (2017) 200:279–82. doi: 10.1016/j.jsb.2017.08.001
23. Latz E, Xiao TS, Stutz A. Activation and regulation of the inflammasomes. Nat Rev Immunol. (2013) 13:397–411. doi: 10.1038/nri3452
24. Shao W, Yang Z, Fu Y, Zheng L, Liu F, Chai L, et al. The pyroptosis-related signature predicts prognosis and indicates immune microenvironment infiltration in gastric cancer. Front Cell Dev Biol. (2021) 9:676485. doi: 10.3389/fcell.2021.676485
25. Yu G, Wang LG, Han Y, He QY. Clusterprofiler: an R package for comparing biological themes among gene clusters. OMICS. (2012) 16:284–7. doi: 10.1089/omi.2011.0118
26. Yao F, Zhan Y, Pu Z, Lu Y, Chen J, Deng J, et al. LncRNAs target ferroptosis-related genes and impair activation of CD4(+) T cell in gastric cancer. Front Cell Dev Biol. (2021) 9:797339. doi: 10.3389/fcell.2021.797339
27. Wang XF, Lei W, Liu CM, Yang J, Zhu YH. BOLA3 Is a prognostic-related biomarker and correlated with immune infiltrates in lung adenocarcinoma. Int Immunopharmacol. (2022) 107:108652. doi: 10.1016/j.intimp.2022.108652
28. Zhang T, Yu S, Zhao S. Development and verification of a microsatellite instability-related risk signature for predicting survival and therapy effectiveness in gastric cancer. J Gastrointest Oncol. (2022) 13:84–101. doi: 10.21037/jgo-21-808
29. Kiss F, Kormos V. Functional transient receptor potential ankyrin 1 and vanilloid 1 ion channels are overexpressed in human oral squamous cell carcinoma. Int J Mol Sci. (2022) 23:1921. doi: 10.3390/ijms23031921
30. Liu L, Guo B, Han Y, Xu S, Liu S. MARCH1 Silencing suppresses growth of oral squamous cell carcinoma through regulation of PHLPP2. Clin Transl Oncol. (2022) 24(7):1311–21. doi: 10.1007/s12094-021-02769-5
31. Scholtz B, Horváth J, Tar I, Kiss C. Salivary miR-31-5p, miR-345-3p, and miR-424-3p are reliable biomarkers in patients with oral squamous cell carcinoma. Pathogens. (2022) 11:229. doi: 10.3390/pathogens11020229
32. Xu Z, Li X, Pan L, Tan R, Ji P, Tang H. Development of a lncRNA-based prognostic signature for oral squamous cell carcinoma. J Oral Pathol Med. (2022) 51(4):358–68. doi: 10.1111/jop.13281
33. He S, Chakraborty R, Ranganathan S. Proliferation and apoptosis pathways and factors in oral squamous cell carcinoma. Int J Mol Sci. (2022) 23:1562. doi: 10.3390/ijms23031562
34. Bedoui S, Herold MJ, Strasser A. Emerging connectivity of programmed cell death pathways and its physiological implications. Nat Rev Mol Cell Biol. (2020) 21:678–95. doi: 10.1038/s41580-020-0270-8
35. Zaki MH, Vogel P, Body-Malapel M, Lamkanfi M, Kanneganti TD. IL-18 production downstream of the Nlrp3 inflammasome confers protection against colorectal tumor formation. J Immunol. (2010) 185:4912–20. doi: 10.4049/jimmunol.1002046
36. Ma X, Guo P, Qiu Y, Mu K, Zhu L, Zhao W, et al. Loss of AIM2 expression promotes hepatocarcinoma progression through activation of mTOR-S6K1 pathway. Oncotarget. (2016) 7:36185–97. doi: 10.18632/oncotarget.9154
37. Wei J, Wang B, Gao X, Sun D. Prognostic value of a novel signature with nine hepatitis C virus-induced genes in hepatic cancer by mining GEO and TCGA databases. Front Cell Dev Biol. (2021) 9:648279. doi: 10.3389/fcell.2021.648279
38. Hu Z, Xu Y. Bioinformatics analysis and experimental verification identify downregulation of COL27A1 in poor segmental congenital scoliosis. Comput Math Methods Med. (2022) 2022:2616827. doi: 10.1155/2022/2616827
39. Pölsler L, Schatz UA, Simma B, Zschocke J, Rudnik-Schöneborn S. A Syrian patient with steel syndrome due to compound heterozygous COL27A1 mutations with colobomata of the eye. Am J Med Genet A. (2020) 182:730–4. doi: 10.1002/ajmg.a.61478
40. Garciaz S, N’Guyen Dasi L, Finetti P, Chevalier C, Vernerey J, Poplineau M, et al. Epigenetic down-regulation of the HIST1 locus predicts better prognosis in acute myeloid leukemia with NPM1 mutation. Clin Epigenetics. (2019) 11:1–14. doi: 10.1186/s13148-019-0738-6
41. Azimi M, Totonchi M, Rahimi M, Firouzi J, Sahranavard P, Emami Razavi A, et al. An integrated analysis to predict micro-RNAs targeting both stemness and metastasis in human gastric cancer. J Gastroenterol Hepatol. (2021) 36:436–45. doi: 10.1111/jgh.15176
42. Liu S, Liu B, Zhao Q, Shi J, Gu Y, Guo Y, et al. Down-regulated FST expression is involved in the poor prognosis of triple-negative breast cancer. Cancer Cell Int. (2021) 21:1–12. doi: 10.1186/s12935-021-01977-x
43. Tang H, Wei P, Chang P, Li Y, Yan D, Liu C, et al. Genetic polymorphisms associated with pancreatic cancer survival: a genome-wide association study. Int J Cancer. (2017) 141:678–86. doi: 10.1002/ijc.30762
44. Jackman DM, Cioffredi LA, Jacobs L, Sharmeen F, Morse LK, Lucca J, et al. A phase I trial of high dose gefitinib for patients with leptomeningeal metastases from non-small cell lung cancer. Oncotarget. (2015) 6:4527–36. doi: 10.18632/oncotarget.2886
45. Khalil A, Jameson MJ. The EGFR inhibitor gefitinib enhanced the response of human oral squamous cell carcinoma to cisplatin in vitro. Drugs R D. (2017) 17:545–55. doi: 10.1007/s40268-017-0204-x
Keywords: oral squamous cell carcinoma, pyroptosis, immune infiltration, overall survival, prognostic signature
Citation: Zeng D, Wang X, Zhang S, Zheng A, Huang Q and Cao L (2022) Pyroptosis-related gene-based prognostic signature for predicting the overall survival of oral squamous cell carcinoma patients. Front. Surg. 9:903271. doi: 10.3389/fsurg.2022.903271
Received: 24 March 2022; Accepted: 7 July 2022;
Published: 19 August 2022.
Edited by:
Qi Xue, Chinese Academy of Medical Sciences and Peking Union Medical College, China© 2022 Zeng, Wang, Zhang, Zheng, Huang and Cao. This is an open-access article distributed under the terms of the Creative Commons Attribution License (CC BY). The use, distribution or reproduction in other forums is permitted, provided the original author(s) and the copyright owner(s) are credited and that the original publication in this journal is cited, in accordance with accepted academic practice. No use, distribution or reproduction is permitted which does not comply with these terms.
*Correspondence: Lingyan Cao bGluZ3lhbmNhb2hvc0AxNjMuY29t Qingfeng Huang aHcxNjg1QDE2My5jb20=
Specialty Section: This article was submitted to Surgical Oncology, a section of the journal Frontiers in Surgery
Disclaimer: All claims expressed in this article are solely those of the authors and do not necessarily represent those of their affiliated organizations, or those of the publisher, the editors and the reviewers. Any product that may be evaluated in this article or claim that may be made by its manufacturer is not guaranteed or endorsed by the publisher.
Research integrity at Frontiers
Learn more about the work of our research integrity team to safeguard the quality of each article we publish.