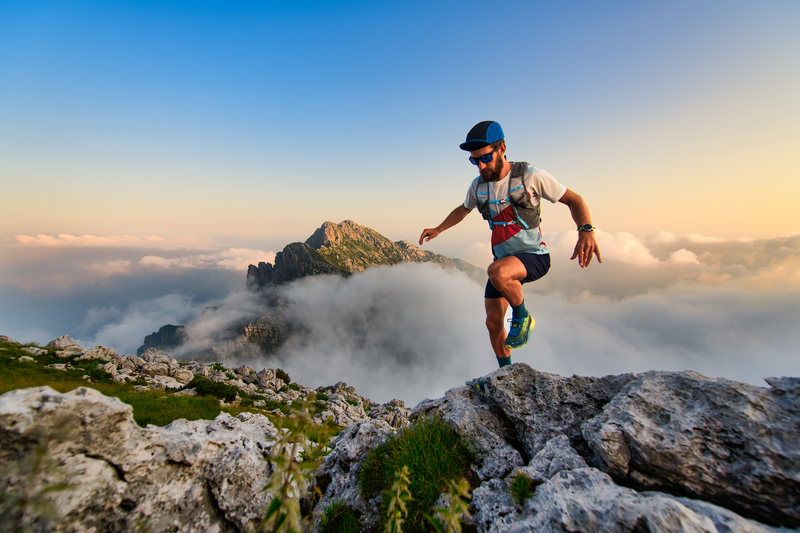
95% of researchers rate our articles as excellent or good
Learn more about the work of our research integrity team to safeguard the quality of each article we publish.
Find out more
SYSTEMATIC REVIEW article
Front. Surg. , 06 January 2023
Sec. Surgical Oncology
Volume 9 - 2022 | https://doi.org/10.3389/fsurg.2022.1045295
Background: To assess the predictive value of radiomics for preoperative lymph node metastasis (LMN) in patients with biliary tract cancers (BTCs).
Methods: PubMed, Embase, Web of Science, Cochrane Library databases, and four Chinese databases [VIP, CNKI, Wanfang, and China Biomedical Literature Database (CBM)] were searched to identify relevant studies published up to February 10, 2022. Two authors independently screened all publications for eligibility. We included studies that used histopathology as a gold standard and radiomics to evaluate the diagnostic efficacy of LNM in BTCs patients. The quality of the literature was evaluated using the Radiomics Quality Score (RQS) and the Quality Assessment of Diagnostic Accuracy Studies 2 (QUADAS-2). The diagnostic odds ratio (DOR), sensitivity, specificity, positive likelihood ratio (PLR), negative likelihood ratio (NLR), and area under the receiver operating characteristic curve (AUC) were calculated to assess the predictive validity of radiomics for lymph node status in patients with BTCs. Spearman correlation coefficients were calculated, and Meta-regression and subgroup analyses were performed to assess the causes of heterogeneity.
Results: Seven studies were included, with 977 patients. The pooled sensitivity, specificity and AUC were 83% [95% confidence interval (CI): 77%, 88%], 78% (95% CI: 71, 84) and 0.88 (95% CI: 0.85, 0.90), respectively. The substantive heterogeneity was observed among the included studies (I2 = 80%, 95%CI: 58,100). There was no threshold effect seen. Meta-regression showed that tumor site contributed to the heterogeneity of specificity analysis (P < 0.05). Imaging methods, number of patients, combined clinical factors, tumor site, model, population, and published year all played a role in the heterogeneity of the sensitivity analysis (P < 0.05). Subgroup analysis revealed that magnetic resonance imaging (MRI) based radiomics had a higher pooled sensitivity than contrast-computed tomography (CT), whereas the result for pooled specificity was the opposite.
Conclusion: Our meta-analysis showed that radiomics provided a high level of prognostic value for preoperative LMN in BTCs patients.
Biliary tract cancers (BTCs) are malignant tumors derived from biliary epithelial cells, including intrahepatic and extrahepatic cholangiocarcinoma and gallbladder cancer (1–3). The global incidence is increasing (4). Radical resection is the only option to prolong survival in patients with BTCs (5). Unfortunately, less than 35% of patients are suitable for early surgery (6, 7). Recurrence after curative resection remains high, with intrahepatic cholangiocarcinoma (ICC) reaching 50%–70% (8). According to previous studies, lymph node metastasis (LMN) is the most relevant adverse prognostic factor after BTCs surgery (9). In addition, LMN is a relative contraindication for liver transplantation (10). Therefore, it is crucial to evaluate the accurate status of lymph nodes by non-introductive means, which is key for BTCs to guide treatment and determine prognosis.
Medical imaging methods play an important role in assessing the status of lymph nodes in BTCs. Conventional imaging examinations include ultrasonography, computer tomography (CT), positron emission tomography/computed tomography (PET-CT), and magnetic resonance imaging (MRI) (11, 12). Nowadays, preoperative assessment of lymph node status remains difficult. Razumilava et al. Studies have shown that CT has a sensitivity of 30%–50% in diagnosing lymph node status (7). There is no consensus on the evaluation of the preoperative lymph node status of BTCs by current detection methods (13, 14). A label non-invasive detection method called radiomics has recently been utilized to predict chemotherapy response, lymph node metastasis, and tumor classification (15). it extracts high-dimensional radiomics information from medical images and combines machine learning algorithms for clinical decision-making (16, 17). A systematic review and meta-analysis by Huang et al. found that radiomics might be an effective tool for assessing preoperative microvascular invasion in hepatocellular carcinoma (17). Several published studies have employed a radiomics model to predict LNM in BTCs (18–20). Due to differences in imaging modality, study methodology, sample size imaging modalities, research methods, sample size and so on, the reported diagnostic efficiency ranged from 68% to 98% in the above studies. Therefore, the performance of radiomics for preoperative LMN identification in clinical practice remains uncertain.
The purpose of this meta-analysis was to determine the diagnostic efficacy of radiomics for preoperative LMN prediction in patients with BTCs.
This meta-analysis was conducted following the Preferred Reporting Items for Systematic Reviews and Meta-Analyses (PRISMA) statement recommended by the Cochrane Collaboration (21). This study was prospectively registered in PROSPERO (CRD42022333874).
Two authors (YM and YH) independently searched PubMed, Embase, Web of Science, Cochrane Library, and four Chinese Databases [VIP, CNKI, Wanfang, and Chinese BioMedical Literature Databases (CBM)] to determine the studies published as of February 10, 2022. The search formula was as follows: [(lymph node metastasis) OR (lymph node) OR(LMN)] and [(Biliary Tract Cancer) OR (intrahepatic cholangiocarcinoma) OR (extrahepatic cholangiocarcinoma) OR (ICC) OR (ECC)] and [(radiomics) OR (machine learning) OR (deep learning) OR (artificial intelligence) OR (texture)]. After eliminating duplicate articles, the titles and abstracts of all remaining articles were reviewed. When it was ambiguous whether the article was included merely by title and abstract, the entire publication was downloaded and reviewed. All studies were independently screened by two authors (YM and YH). Discuss the inclusion issues if there were any discrepancies. In order to find other relevant publications, we also carefully went through the reference lists for each important study that we had already identified as well as earlier systematic reviews.
Inclusion criteria were as follows: (1) diagnosis of BTCs by pathologic criteria; (2) determination of LMN by pathologic diagnosis; (3) CT, MRI, PET-CT, or ultrasonography were performed before surgical resection, liver transplantation, or other treatments; (4) imaging analysis based on radiomics.
Exclusion criteria are as follows: (1) having received any treatment (radiotherapy, chemotherapy, or immunotherapy) before the examination; (2) Patients who received palliative surgery without lymph node resection; (3) Reviews, editorials, letters, and animal articles are excluded.
The Radiomics Quality Score (RQS) and Quality Assessment of Diagnostic Accuracy Studies 2 (QUADAS-2) were used by the two authors (YM and YH) to evaluate the methodological quality and risk of bias of the chosen studies independently, respectively (22, 23).
The data extraction and quality evaluation of the retrieved research are independently completed by the two authors. We extracted data about patient characteristics, imaging methods, and research characteristics from each selected study. Patient characteristics included the total number of subjects, the number of subjects with LMN and no-LMN, sensitivity, and specificity. The number of true positive (TP), true negative (TN), false positive (FP), and false negative (FN) was calculated according to the number of LMN, non-LMN, sensitivity, and specificity reported in each included study. The reference formula was as follows: sensitivity = TP/(TP+FN), specificity = TN/(FP+TN). The studies that provide a two-by-two contingency table or sufficient data to reconstruct such a table are eligible for analysis. The best model presented in the study was included in our meta-analysis when there were two or more prediction models based on the same cohort of patients in one study.
Statistical analysis was performed using Stata software (version 16.0) and Review Manager software (version 5.3). The sensitivity, specificity, positive likelihood ratio (PLR), negative likelihood ratio (NLR), and diagnostic odds ratio (DOR) with their corresponding 95% confidence intervals (CI) were calculated. A summary receiver operating characteristic (sROC) curve was plotted and the area under the curve (AUC) was calculated to demonstrate the diagnostic value of the joint studies (24). AUC was 0.5–0.7, 0.7–0.9, and > 0.9, indicating low, medium, and high diagnostic power, respectively.
We drew forest plots to show the variation among studies and to detect heterogeneity for the pooled sensitivity and specificity. The threshold effect resulting in heterogeneity was assessed using the spearman correlation coefficient. If P > 0.05, there was no threshold effect. The heterogeneity caused by the non-threshold effect was measured by Cochrane's Q-test and inconsistency index I2. When P < 0.05, the difference was considered significant, and I2 ≥ 50% was considered moderate to high heterogeneity among studies (25). Meta-regression and subgroup analysis were used to study the potential sources of heterogeneity. We conducted a univariate meta-regression analysis of some related covariates, including the tumor site (ICC or no-ICC), combined clinical factors (yes or no), imaging methods (MRI or CT), number of patients (≥ 150 or < 150), QUADAS-2 applicability risk (no or high risk), model (logistic regression or machine learning), population (single or multicenter), number of radiomics features (≥ 300 or < 300), and published year (before 2020 or after 2020). Additionally, a sensitivity analysis was carried out by removing one study at a time to assess the effect of a single study on the overall estimation. Deeks' funnel plot was used to check publication bias (26).
A Fagan plot was calculated to assess clinical utility by indicating the post-test probability (P-post) of LNM when pretest probabilities (P-pre, suspicion of LNM) were provided (27).
The literature search and study selection was shown in Figure 1. The included studies were published between 2018 and 2021 (four contract-CT based on radiomics studies (18, 28, 29) and three MRI based on radiomics studies (19, 30–32) were included in the meta-analysis). A total of 977 BTCs patients were included. Of those, 554 patients (56.8%) had a pathological diagnosis of no-LMN, while 423 patients (43.2%) had a pathological diagnosis of LMN. The baseline characteristics of the included studies was showed in Table 1.
A detailed report of the RQS project scores was shown in Table 2. The RQS included in the study ranged from 11 to 20 points. The publication with the highest percentage of RQS was 56.0%. Indicating excellent reproducibility across readers, the interclass correlation coefficient (ICC) among separate readers rating publications was 0.972 (95% CI: 0.854–0.997, P < 0.001). The RQS scores assessed by both readers were presented in Supplementary materials.
The methodological quality of the studies according to the QUADAS-2 assessment was illustrated in Figure 2. Two studies obtained an unclear risk of bias in the index testing (28, 30). Uncertain risk of bias was found in both the flow and time domains in two studies (19, 28). There were relatively few concerns about the applicability of the three domains (patient selection, index test, and reference criteria). No significant publication bias risk was detected by Deeks' funnel plot analysis (Figure 3; P = 0.77).
Figure 2. Methodological quality assessment of the included studies based on the QUADAS-2 scale. (A) Individual studies, (B) summary.
The pooled sensitivity and specificity were 83% (77%, 88%) and 78% (71%, 84%), respectively, based on radiomics assessment of lymph node status in each patient. AUC, DOR, PLR and NLR were 0.88 (0.85, 0.90), 17.82 (11.42, 27.80), 3.80 (2.88, 5.00) and 0.21 (0.16, 0.29), respectively. Figure 4 shows the forest plots for sensitivity and specificity, while Figure 5 shows the sROC curve.
Figure 4. Forest plot of sensitivity and specificity based on radiomics for preoperative prediction of LMN in BTCs.
Figure 5. Summary receiver operating characteristic curves (sROC) based on radiomics for preoperative prediction of LMN in BTCs.
The Spearman correlation coefficient for the threshold effect was found to be −0.67 and P = 0.45, indicating that there was no threshold effect. There was considerable heterogeneity among the studies (overall I² = 80%; 95%CI: 58.00,100.00; P = 0.003). The forest plots indicated high heterogeneity with I2 values > 50% for sensitivity (I² = 65.90%; 95% CI: 38.41, 93.39; P = 0.01) and specificity (I² = 68.00%; 95% CI: 42.55, 93.46; P < 0.01).
A univariate meta-regression analysis was used to determine the sources of heterogeneity. The outcomes of subgroup analysis and univariate meta-regression were displayed in Table 3. The results showed that the sources of heterogeneity in the sensitivity analysis, in addition to QUADAS, included number of radiomics features, tumor site, imaging methods, number of patients, combined clinical factors, model, population, and publication year (P < 0.05). Additionally, tumor site contributed to the heterogeneity in the specificity analysis (P < 0.05).
In terms of tumor site, the sensitivity (84%; 95% CI: 76, 93 vs. 83%; 95% CI: 76, 90) and specificity (78%; 95% CI: 68, 87 vs. 78%; 95% CI: 70, 85) were basically equivalent among studies with ICC studies (n = 3) and no-ICC studies (n = 4). In comparison to radiomics combined with clinical risk factors, radiomics alone had better sensitivity (86%; 95% CI: 77, 94 vs. 82%; 95% CI: 76, 89) and specificity (81%; 95% CI: 69, 94 vs. 77%; 95%; CI: 70, 85). Moreover, the pooled sensitivity (86%; 95% CI: 81, 92 vs. 80%; 95% CI: 72, 87) and specificity (80%; 95% CI: 70, 89 vs. 77%; 95% CI: 69, 85) of studies published after 2020 (n = 3) was relatively high than that of earlier studies (n = 4). Regardless of the QUADAS risk, the specificity (78%;95% CI: 63, 93 vs. 78%; 95% CI: 71, 85) was roughly same for both. Furthermore, QUADAS high-risk studies (n = 3; 88%; 95% CI: 78, 98) had a marginally greater sensitivity than no high-risk studies (n = 4; 82%; 95% CI: 77, 88).
Among different imaging methods, MRI (n = 3) had higher sensitivity (87%; 95% CI: 81, 93 vs. 80%; 95% CI: 73, 87), but the specificity of contract-CT (81%; 95% CI: 75, 86) better than MRI (71%; 95% CI: 60, 82). In addition, multicenter center studies (n = 1) exhibited greater sensitivity (88%; 95% CI: 78, 98 vs. 82%; 95% CI: 77, 88) than s single-center studies (n = 6). The pooled sensitivity for more radiomics features (n = 3) was higher (88%; 95% CI: 81, 94 vs. 80%; 95% CI: 74, 87), whereas the trend for pooled specificity was the opposite (75%; 95% CI: 65, 85 vs. 80%; 95% CI: 73, 88). Similarly, studies with 150 patients or fewer (n = 4) had a greater pooled sensitivity (84%; 95% CI: 77, 91) but a lower specificity (77%; 95% CI: 67, 86) than studies with more than 150 patients (n = 3; sensitivity, 82%; 95% CI: 74, 91; specificity, 79%; 95% CI: 71, 87). For modeling methods, machine learning (n = 3) approach had exhibited better sensitivity (87%; 95% CI: 81, 93)] than logistic regression (n = 4; 79%; 95% CI: 73, 86) and lower specificity [72%; 95% CI: 60, 85) than logistic regression (79%; 95% CI: 71, 87).
No significant changes were observed when each included study was eliminated from the analysis one by one. The results of sensitivity analyses for each study are shown in Table 4.
Using radiomics studies would increase the posttest probability to 49 from 20% with a PLR of 4 when the pretest was positive and would reduce the posttest probability to 5% with an NLR of 0.21 when the pretest was negative (Figure 6).
The seven studies were included in the meta-analysis. The diagnostic efficacy of radiomics for preoperative LMN in BTCs patients was judged by combining diagnostic effect size and fitting the sROC curve. Analyze the heterogeneity of the studies and their sources, and identify factors that may affect the results. Finally, through sensitivity analysis, publication bias and clinical application value were detected to evaluate the credibility of this meta-analysis.
Our meta-analysis showed high sensitivity (83%; 95% CI: 77%, 88%), specificity (78%; 95% CI: 71, 84) and AUC (0.88; 95% CI: 0.85, 0.90). In addition, the likelihood ratio and post-test probability indicate that the post-test probability increases from 20% to 49% when the current test is positive and the PLR is 4; when the current measurement is negative and the NLR is 0.21, the post-test probability is reduced to 5%. This further indicates that radiomics is helpful to improve the accuracy of predicting LMN in BTCs patients. All things considered, radiomics can assist us in possible resolution treatment protocols for BTCs LMN patients before surgery, increase the survival rate of BTCs patients, and decrease the probability of recurrence.
Currently, using visual observation and interpretation of medical images to evaluate lymph node metastasis in BTCs is still challenging (33). Radiomics, as a personalized assessment tool, has proved to be a promising non-invasive tumor lymph node evaluation to overcome the limitations of visual assessment of lymph node images by imaging physicians (34). Our findings demonstrate that radiomics can increase to 83% the sensitivity of preoperative BTCs lymph node metastatic evaluation. The radiomics method, which offers crucial supplementary information on imaging phenotypes and may contain a wealth of data, maybe the cause. This includes texture features that reflect the pattern or spatial distribution of voxel intensity in the region of interest (ROI), which are connected to tumor heterogeneity (35, 36). Wavelet features can also offer multi-frequency information to measure tumor heterogeneity and raise diagnostic accuracy (35, 37, 38). Therefore, we have reason to believe that radiomics, which is used by professional radiologists for ROI segmentation and high-throughput feature extraction for disease classification and prognosis, may be more suitable for the preoperative evaluation of LMN in patients with BTCs.
Although there was no discernible threshold effect, there was overall significant heterogeneity between studies (I² = 80%; 95%CI: 58, 100; P = 0.003). We conducted meta-regression to detect the sources of heterogeneity. Due to the limited number of included studies, only univariable meta-regression analysis was performed. The results revealed that factors such as the tumor site, imaging methods, number of patients, combined clinical factors, number of radiomics features, model, population, and published year all contributed to the heterogeneity of sensitivity analysis. In addition, the methodologies utilized in each of the included studies varied, contributing to the heterogeneity in a way that made it impossible to identify all of its sources.
We used key factors for subgroup analysis. In the study design subgroup analysis, the imaging methods results showed that the pooled sensitivity of MRI imaging is better than that of contract-CT, which is similar to the previous research results (28, 39, 40). From the number of features and modeling methods of the included studies, the extraction of more radiomics features could improve the pooled sensitivity, and the possible reason texture features could improve the accuracy of the model. However, numerous features had an impact on feature selection and model robustness, so its specificity was lower. Machine learning still needs to be investigated in terms of feature selection and model generalization potential because it can increase sensitivity but decrease specificity.
Studies have demonstrated that in patients with BTCs, the CA199 and CT lymph node status are independent predictors of LMN (18, 36). Our meta-analysis showed that combined clinical factors did not improve the diagnostic ability of radiomics. Therefore, combining clinical features in radiomics to improve the diagnostic accuracy of LNM needs further exploration. The diagnostic sensitivity of ICC was slightly higher than no-ICC, but the overall diagnostic efficacy had no significant difference. It is reasonable to think that more multicenter studies in the future will increase the predictive performance of MLN in patients with BTCs because its pooled sensitivity was greater than that of single-center studies. There is only one high-risk trial, however, there is no obvious heterogeneity in the sensitivity or specificity of the QUADAS-2 score. The ability to identify LMN in the future study will be improved by controlling study quality to lessen bias.
Despite radiomics' strong capacity to predict, the quality of the included research as a whole range (RQS range from 11 points to 20 points). There is no cost-benefit analysis or prospective design. One study only received external validation. The QUADAS-2 data quality assessment revealed some additional issues. For example, index testing results from two studies showed uncertain bias risks.
Our meta-analysis that used radiomics to predict the lymph node status of preoperative BTCs offered two advantages. First of all, this study, which was the first meta-analysis to assess the diagnostic efficacy of preoperative prediction of lymph node status in BTCs patients by radiomics evaluation method, involved seven studies and 977 BTCs patients. Second, we used subgroup analysis to evaluate the effects of different factors on the heterogeneity of the studies, providing a guide for upcoming radiomics research and clinical evaluation.
There are some limitations to our study. First, there are few qualified radiomics studies, and different medical centers use various inspection equipment. As a result, research methods vary from study to study, and imaging methods, ROI, feature extraction, and modeling methods provide many options. Second, different imaging methods, number of patients, and tumor sites may lead to heterogeneity. Therefore, we use regression analysis to identify the sources of heterogeneity. Finally, while there are some uncertainties associated with the QUADAS-2 evaluation, the risks of uncertainty may not significantly affect the results and therefore the overall quality of the study can be analyzed.
In conclusion, radiomics is a useful tool for predicting LMN in patients with BTCs. Radiomics study on LMN prediction, however, is still in its early phases. Further study on the quality of radiomics is required in the segmentation of ROI, method repeatability, model building, and overfitting solutions. To demonstrate the clinical value of radiomics, further high-quality, multicenter, large-scale prospective trials are required.
The original contributions presented in the study are included in the article/Supplementary Material, further inquiries can be directed to the corresponding author/s.
Study design: YM, YL, JL, YP, YZ, and WM. Literature search and study selection: YM, YH, and WM. Data extraction and quality assessment: YH, YL, QS, and BZ. Statistical analysis: YH, HL, and JL. Study supervision: YZ, WM, and XL. WM and YL obtained the research fund. Editing and review of the manuscript: all authors. All authors contributed to the article and approved the submitted version.
This work was supported by the National Natural Science Foundation of China (Grant No. 82060551); Health industry scientific research project of Gansu Province (Grant No. GSWSKY2020-11 sCLU); and Lanzhou Chengguan District Science and technology planning project (Grant No. 2019RCCX0038).
The authors declare that the research was conducted in the absence of any commercial or financial relationships that could be construed as a potential conflict of interest.
All claims expressed in this article are solely those of the authors and do not necessarily represent those of their affiliated organizations, or those of the publisher, the editors and the reviewers. Any product that may be evaluated in this article, or claim that may be made by its manufacturer, is not guaranteed or endorsed by the publisher.
The Supplementary Material for this article can be found online at: https://www.frontiersin.org/articles/10.3389/fsurg.2022.1045295/full#supplementary-material.
BTCs: biliary tract cancers; LMN: lymph node metastasis; CBM: China Biomedical Literature Database; RQS: Radiomics Quality Score; QUADAS-2: Quality Assessment of Diagnostic Accuracy Studies 2; DOR: diagnostic odds ratio; PLR: positive likelihood ratio; NLR: negative likelihood ratio; AUC: area under the receiver operating characteristic curve; CI: confidence interval; MRI: magnetic resonance imaging; CT: computed tomography; ICC: intrahepatic cholangiocarcinoma; PET-CT: positron emission tomography/computed tomography; PRISMA: Preferred Reporting Items for Systematic Reviews and Meta-Analyses; TP: true positive; TN: true negative; FP: false positive; FN: and false negative; sROC: summary receiver operating characteristic; ROI: region of interest; ML: logistic regression; LR: machine learning
1. Mavros MN, Economopoulos KP, Alexiou VG, Pawlik TM. Treatment and prognosis for patients with intrahepatic cholangiocarcinoma: systematic review and meta-analysis. JAMA Surg. (2014) 149:565–74. doi: 10.1001/jamasurg.2013.5137
2. Hundal R, Shaffer EA. Gallbladder cancer: epidemiology and outcome. Clin Epidemiol. (2014) 6:99–109. doi: 10.2147/CLEP.S37357
3. Khan SA, Clements O, Jin UK, Joseph E. Reply to: “letter regarding [risk factors for intrahepatic and extrahepatic cholangiocarcinoma: a systematic review and meta-analysis]”. J Hepatol. (2020) 72:95–103. doi: 10.1016/S0168-8278(20)30719-4
4. DeSantis CE, Kramer JL, Jemal A. The burden of rare cancers in the United States. CA: a Cancer J Clin. (2017) 67:261–72. doi: 10.3322/caac.21400
5. Horgan AM, Amir E, Walter T, Knox JJ. Adjuvant therapy in the treatment of biliary tract cancer: a systematic review and meta-analysis. J Clin Oncol. (2012) 30:1934–40. doi: 10.1200/JCO.2011.40.5381
6. Chan E, Berlin J. Biliary tract cancers: understudied and poorly understood. J Clin Oncol. (2015) 33:1845–8. doi: 10.1200/JCO.2014.59.7591
7. Razumilava N, Gores GJ. Cholangiocarcinoma. Lancet (London, England). (2014) 383:2168–79. doi: 10.1016/S0140-6736(13)61903-0
8. Zhang XF, Beal EW, Bagante F, Chakedis J, Weiss M, Popescu I, et al. Early versus late recurrence of intrahepatic cholangiocarcinoma after resection with curative intent. Br J Surg. (2018) 105:848–56. doi: 10.1002/bjs.10676
9. Mao ZY, Guo XC, Su D, Wang LJ, Zhang TT, Bai L. Prognostic factors of cholangiocarcinoma after surgical resection: a retrospective study of 293 patients. Med Sci Moni. (2015) 21:2375–81. doi: 10.12659/MSM.893586
10. Rizvi S, Khan SA, Hallemeier CL, Kelley RK, Gores GJ. Cholangiocarcinoma - evolving concepts and therapeutic strategies. Nat Rev Clin Oncol. (2018) 15:95–111. doi: 10.1038/nrclinonc.2017.157
11. Wang Y, Zhou CW, Zhu GQ, Li N, Qian XL, Chong HH, et al. A multidimensional nomogram combining imaging features and clinical factors to predict the invasiveness and metastasis of combined hepatocellular cholangiocarcinoma. Ann Transl Med. (2021) 9:1518. doi: 10.21037/atm-21-2500
12. Zhou Y, Zhou G, Gao X, Xu C, Wang X, Xu P. Apparent diffusion coefficient value of mass-forming intrahepatic cholangiocarcinoma: a potential imaging biomarker for prediction of lymph node metastasis. Abdom Radiol (New York). (2020) 45:3109–18. doi: 10.1007/s00261-020-02458-x
13. Saleh M, Virarkar M, Bura V, Valenzuela R, Javadi S, Szklaruk J, et al. Intrahepatic cholangiocarcinoma: pathogenesis, current staging, and radiological findings. Abdom Radiol (New York). (2020) 45:3662–80. doi: 10.1007/s00261-020-02559-7
14. Blechacz B, Komuta M, Roskams T, Gores GJ. Clinical diagnosis and staging of cholangiocarcinoma. Nature Reviews Gastroenterology & Hepatol. (2011) 8:512–22. doi: 10.1038/nrgastro.2011.131
15. Huang YQ, Liang CH, He L, Tian J, Liang CS, Chen X, et al. Development and validation of a radiomics nomogram for preoperative prediction of lymph node metastasis in colorectal cancer. J Clin Oncol. (2016) 34:2157–64. doi: 10.1200/JCO.2015.65.9128
16. Liang W, Xu L, Yang P, Zhang L, Wan D, Huang Q, et al. Novel nomogram for preoperative prediction of early recurrence in intrahepatic cholangiocarcinoma. Front Oncol. (2018) 8:360. doi: 10.3389/fonc.2018.00360
17. Tang Y, Zhang T, Zhou X, Zhao Y, Xu H, Liu Y, et al. The preoperative prognostic value of the radiomics nomogram based on CT combined with machine learning in patients with intrahepatic cholangiocarcinoma. World J Surg Oncol. (2021) 19:45. doi: 10.1186/s12957-021-02162-0
18. Ji GW, Zhu FP, Zhang YD, Liu XS, Wu FY, Wang K, et al. A radiomics approach to predict lymph node metastasis and clinical outcome of intrahepatic cholangiocarcinoma. Eur Radiol. (2019) 29:3725–35. doi: 10.1007/s00330-019-06142-7
19. Yang C, Huang M, Li S, Chen J, Yang Y, Qin N, et al. Radiomics model of magnetic resonance imaging for predicting pathological grading and lymph node metastases of extrahepatic cholangiocarcinoma. Cancer Lett. (2020) 470:1–7. doi: 10.1016/j.canlet.2019.11.036
20. Tang Y, Yang CM, Su S, Wang WJ, Fan LP, Shu J. Machine learning-based radiomics analysis for differentiation degree and lymphatic node metastasis of extrahepatic cholangiocarcinoma. BMC cancer. (2021) 21:1268. doi: 10.1186/s12885-021-08947-6
21. McInnes MDF, Moher D, Thombs BD, McGrath TA, Bossuyt PM, Clifford T, et al. Preferred reporting items for a systematic review and meta-analysis of diagnostic test accuracy studies: the PRISMA-DTA statement. Jama. (2018) 319:388–96. doi: 10.1001/jama.2017.19163
22. Huang J, Tian W, Zhang L, Huang Q, Lin S, Ding Y, et al. Preoperative prediction power of imaging methods for microvascular invasion in hepatocellular carcinoma: a systemic review and meta-analysis. Front Oncol. (2020) 10:887. doi: 10.3389/fonc.2020.00887
23. Lambin P, Leijenaar RTH, Deist TM, Peerlings J, de Jong EEC, van Timmeren J, et al. Radiomics: the bridge between medical imaging and personalized medicine. Nat Rev Clin Oncol. (2017) 14:749–62. doi: 10.1038/nrclinonc.2017.141
24. Moses LE, Shapiro D, Littenberg B. Combining independent studies of a diagnostic test into a summary ROC curve: data-analytic approaches and some additional considerations. Stat Med. (1993) 12:1293–316. doi: 10.1002/sim.4780121403
25. Higgins JP, Thompson SG, Deeks JJ, Altman DG. Measuring inconsistency in meta-analyses. BMJ (Clin Res ed). (2003) 327:557–60. doi: 10.1136/bmj.327.7414.557
26. Deeks JJ, Macaskill P, Irwig L. The performance of tests of publication bias and other sample size effects in systematic reviews of diagnostic test accuracy was assessed. J Clin Epidemiol. (2005) 58:882–93. doi: 10.1016/j.jclinepi.2005.01.016
27. Hellmich M, Lehmacher W. A ruler for interpreting diagnostic test results. Methods Inf Med. (2005) 44:124–6. doi: 10.1055/s-0038-1633930
28. Ji GW, Zhang YD, Zhang H, Zhu FP, Wang K, Xia YX, et al. Biliary tract cancer at CT: a radiomics-based model to predict lymph node metastasis and survival outcomes. Radiol. (2019) 290:90–8. doi: 10.1148/radiol.2018181408
29. Liu X, Liang X, Ruan L, Yan S. A clinical-radiomics nomogram for preoperative prediction of lymph node metastasis in gallbladder cancer. Front Oncol. (2021) 11:633852. doi: 10.3389/fonc.2021.633852
30. Xu L, Yang P, Liang W, Liu W, Wang W, Luo C, et al. A radiomics approach based on support vector machine using MR images for preoperative lymph node status evaluation in intrahepatic cholangiocarcinoma. Theranostics. (2019) 9:5374–85. doi: 10.7150/thno.34149
31. Yao X, Huang X, Yang C, Hu A, Zhou G, Lei J, et al. A novel approach to assessing differentiation degree and lymph node metastasis of extrahepatic cholangiocarcinoma: prediction using a radiomics-based particle swarm optimization and support vector machine model. JMIR Med Inform. (2020) 8:e23578. doi: 10.2196/23578
32. Huang C. Development and validation of a preoperative radiomics nomogram for prediction of lymph node metastasis of intrahepatice cholangiocarcinoma.: Shanghai, China: Naval Medical University; 2019.
33. Bridgewater J, Galle PR, Khan SA, Llovet JM, Park JW, Patel T, et al. Guidelines for the diagnosis and management of intrahepatic cholangiocarcinoma. J Hepatol. (2014) 60:1268–89. doi: 10.1016/j.jhep.2014.01.021
34. Yu Y, He Z, Ouyang J, Tan Y, Chen Y, Gu Y, et al. Magnetic resonance imaging radiomics predicts preoperative axillary lymph node metastasis to support surgical decisions and is associated with tumor microenvironment in invasive breast cancer: a machine learning, multicenter study. EBioMedicine. (2021) 69:103460. doi: 10.1016/j.ebiom.2021.103460
35. Mayerhoefer ME, Materka A, Langs G, Häggström I, Szczypiński P, Gibbs P, et al. Introduction to radiomics. J Nuc Med. (2020) 61:488–95. doi: 10.2967/jnumed.118.222893
36. Wang Y, Shao J, Wang P, Chen L, Ying M, Chai S, et al. Deep learning radiomics to predict regional lymph node staging for hilar cholangiocarcinoma. Front Oncol. (2021) 11:721460. doi: 10.3389/fonc.2021.721460
37. Rizzo S, Botta F, Raimondi S, Origgi D, Fanciullo C, Morganti AG, et al. Radiomics: the facts and the challenges of image analysis. Euro Radiol Exp. (2018) 2:36. doi: 10.1186/s41747-018-0068-z
38. Lafata KJ, Wang Y, Konkel B, Yin FF, Bashir MR. Radiomics: a primer on high-throughput image phenotyping. Abdominal Radiol (New York). (2022) 47:2986–3002. doi: 10.1007/s00261-021-03254-x.
39. Hwang J, Kim YK, Park MJ, Lee MH, Kim SH, Lee WJ, et al. Differentiating combined hepatocellular and cholangiocarcinoma from mass-forming intrahepatic cholangiocarcinoma using gadoxetic acid-enhanced MRI. J Magn Res Imag: JMRI. (2012) 36:881–9. doi: 10.1002/jmri.23728
Keywords: biliary tract cancers, lymph node metastasis, radiomics, diagnosis, meta-analysis
Citation: Ma Y, Lin Y, Lu J, He Y, Shi Q, Liu H, Li J, Zhang B, Zhang J, Zhang Y, Yue P, Meng W and Li X (2023) A meta-analysis of based radiomics for predicting lymph node metastasis in patients with biliary tract cancers. Front. Surg. 9:1045295. doi: 10.3389/fsurg.2022.1045295
Received: 15 September 2022; Accepted: 31 October 2022;
Published: 6 January 2023.
Edited by:
Ulrich Ronellenfitsch, University Hospital Halle (Saale), GermanyReviewed by:
Yawei Qian, Wuhan University, China© 2023 Ma, Lin, Lu, He, Shi, Liu, Li, Zhang, Zhang, Zhang, Yue, Meng and Li. This is an open-access article distributed under the terms of the Creative Commons Attribution License (CC BY). The use, distribution or reproduction in other forums is permitted, provided the original author(s) and the copyright owner(s) are credited and that the original publication in this journal is cited, in accordance with accepted academic practice. No use, distribution or reproduction is permitted which does not comply with these terms.
*Correspondence: Wenbo Meng bWVuZ3diQGx6dS5lZHUuY24= Ping Yue ZHJ5dWVwaW5nQHNpbmEuIENvbQ==
†These authors have contributed equally to this work
Specialty Section: This article was submitted to Surgical Oncology, a section of the journal Frontiers in Surgery
Disclaimer: All claims expressed in this article are solely those of the authors and do not necessarily represent those of their affiliated organizations, or those of the publisher, the editors and the reviewers. Any product that may be evaluated in this article or claim that may be made by its manufacturer is not guaranteed or endorsed by the publisher.
Research integrity at Frontiers
Learn more about the work of our research integrity team to safeguard the quality of each article we publish.