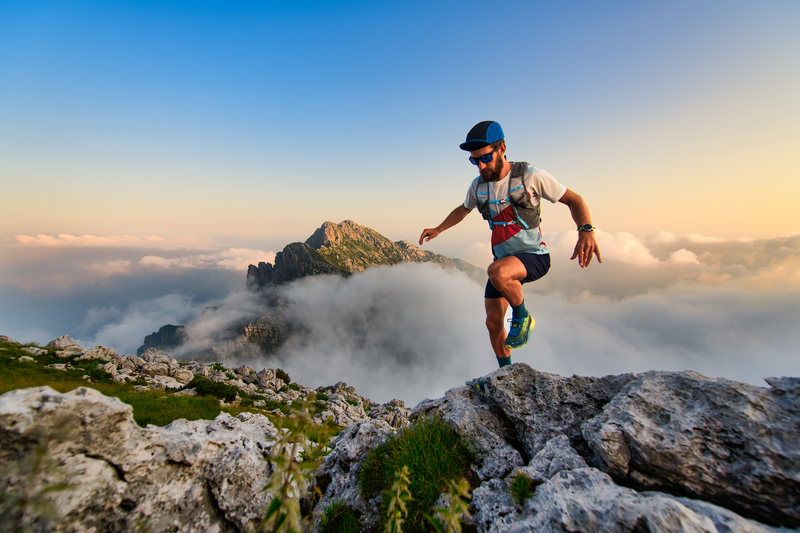
94% of researchers rate our articles as excellent or good
Learn more about the work of our research integrity team to safeguard the quality of each article we publish.
Find out more
CORRECTION article
Front. Sports Act. Living
Sec. Sports Science, Technology and Engineering
Volume 7 - 2025 | doi: 10.3389/fspor.2025.1570591
This article is part of the Research Topic Harnessing Artificial Intelligence in Sports Science: Enhancing Performance, Health, and Education View all articles
This article is a correction to:
Addressing Grading Bias in Rock Climbing: Machine and Deep Learning Approaches
The final, formatted version of the article will be published soon.
You have multiple emails registered with Frontiers:
Please enter your email address:
If you already have an account, please login
You don't have a Frontiers account ? You can register here
1 IntroductionRock climbing’s popularity as a recreational sport is growing dramatically. It is a unique activity for both the body and the mind. In a puzzle-solving manner, climbers strategically scale vertical natural or artificial rock routes–a series of rock features–using their hands and feet. People are drawn to rock climbing because it is an activity in which one can improve physical fitness, problem-solving skills, and self-confidence (1, 2). It is estimated that the rock climbing gym market size was valued at 3 billion USD in 2023 (1), and this projected to double by 2032 (1). In the last five years, the establishment of rock climbing gyms in the US has grown by 6.46% per year (3). Within this time frame, three of the disciplines of rock climbing: sport climbing, bouldering, and speed climbing made their debut appearance at the 2020 Tokyo Olympics. More recently, it reached global audiences again at the 2024 Paris Olympics. Of the three disciplines, bouldering has the largest share of the rock climbing gym market (1, 2). This is because bouldering is the most accessible discipline of climbing, as it requires little equipment and technical knowledge. Recent trends in gym establishment highlight the increasing accessibility to bouldering. In the past decade, 50% of the gyms established in the United States and Canada are only bouldering gyms (3). To capitalize on this popularity, accessibility is crucial for climbing gym success.Climbing accessibility is highly dependent on route setters. Route setters produce climbing routes, the central service of a climbing gym. They are responsible for producing routes that are varied yet consistent in difficulty. Gyms vary their route difficulties to capture the largest audience possible [Market Research Future], catering to a range of climber experience levels from novice to advanced. However, the grading scales used to rate climbing route difficulty are often subjective according to the region, the gym, and the setter of the route (4). General factors considered when determining route difficulty are rock hold types, the number of rock holds on a route, the distance between the rock holds, and the angle of ascent (5). Therefore, it seems that the positioning and sequencing of holds are critical to route difficulty. But holds may be positioned and sequenced in an almost infinite number of ways. Setting a route is like composing a song (6, 7); there are constraints that govern its composition, but the liberty to operate within those constraints is quite large. When operating within these constraints, a route can be developed in a multitude of ways. This wide variance of route generation is a challenge for generalizing route difficulty. without a large sample size, route setters introduce their own biases when determining route difficulty, which then inadvertently affects the climber (i.e., the customer).2 MotivationRoute setters are in an awkward position. The act of setting routes is inherently subjective, but the success of a climb depends on the ability of the setter to objectively set routes. This is the Grading Bias Problem: the setter of a route introduces their biases when declaring a route’s difficulty.Reporting the objective grade of a climbing route is critical in the climbing community and can be aided by machine and deep learning technology (5). Increasingly, machine learning and deep learning techniques are being used to objectively classify the route difficulty. The objectives of this review article are to (1) understand how today’s route setters maintain objectivity in their setting, (2) to review the state-of-the-art approaches in determining climbing route difficulty with machine learning and deep learning, and (3) to suggest new areas for research. Together, these objectives are intended to address how climbing gyms can integrate machine and deep learning systems to streamline route setting and eliminate route difficulty bias for greater consistency and accessibility.3 Document layoutThe Grading Bias Problem will be thoroughly explored in subsequent sections of this paper. Section 4 provides context on rock climbing grade scales and how route setters currently set routes with the goals of objectivity and accessibility. Section 5 details the survey methodology and inclusion criteria. Section 6 identifies the approaches and methods of various deep and machine learning techniques to determine the climbing route difficulty and their success rates. Section 7 discusses the trends, performance, and shortcomings of current machine learning and deep learning techniques. In addition, it is argued that a route-centric approach with a natural language-like model is most optimal. Section 7 continues by proposing future areas for research, in which some proposals are based on works auxiliary to the survey.4 Background: route grading systems and settingClimbing route difficulty can be graded on a variety of scales (Figure 1). The grade scale depends greatly on the discipline, subdisciplines, and climbing systems. In free climbing, the climber ascends a route without any artificial aid. The climber ascends a route by only the natural or artificial features of the rock. But a free climber can still use safety equipment (e.g., rope) in the event that they fall. In the subdisciplines of traditional (trad), sport, and ice climbing, of which the climber ascends a vertical face that is typically greater than 4 meters, the climber’s main tool for protection is a belay system consisting of rope, harness, and either temporary or permanent anchor points. The grading scales of these three “roped” disciplines account for risk to the climber in addition to the technical difficulty of climbing movement. Risk to the climber is most apparent in trad and ice climbing. In both trad and ice climbing, the climber sets and removes protection in rock crevices as they ascend. This protective gear is more prone to fail because they are not intended to be permanent fixtures in the rock or ice face. For ice climbing, it is particularly critical to gauge risk to the climber because the conditions of ice is greatly dependent of weather factors such as temperature, humidity, and precipitation. Although risk to the climber is still a concern in sport climbing, it is greatly reduced because
Keywords: Rock climbing, Bouldering, route grade difficulty, deep learning, machine learning
Received: 03 Feb 2025; Accepted: 10 Feb 2025.
Copyright: © 2025 O'Mara and Mahmud. This is an open-access article distributed under the terms of the Creative Commons Attribution License (CC BY). The use, distribution or reproduction in other forums is permitted, provided the original author(s) or licensor are credited and that the original publication in this journal is cited, in accordance with accepted academic practice. No use, distribution or reproduction is permitted which does not comply with these terms.
* Correspondence:
Muhhamad Shaad Mahmud, University of New Hampshire, Durham, United States
Disclaimer: All claims expressed in this article are solely those of the authors and do not necessarily represent those of their affiliated organizations, or those of the publisher, the editors and the reviewers. Any product that may be evaluated in this article or claim that may be made by its manufacturer is not guaranteed or endorsed by the publisher.
Research integrity at Frontiers
Learn more about the work of our research integrity team to safeguard the quality of each article we publish.