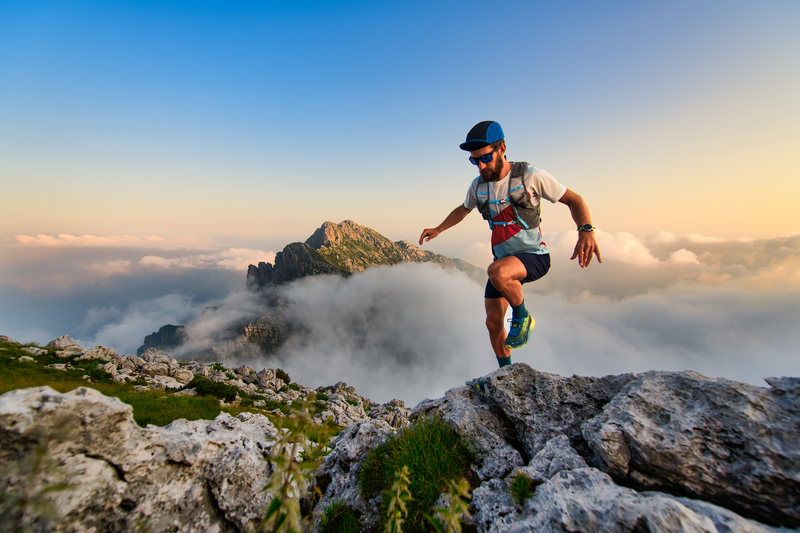
95% of researchers rate our articles as excellent or good
Learn more about the work of our research integrity team to safeguard the quality of each article we publish.
Find out more
REVIEW article
Front. Sports Act. Living , 05 August 2024
Sec. Sports Science, Technology and Engineering
Volume 6 - 2024 | https://doi.org/10.3389/fspor.2024.1375513
Player movement is a fundamental component of evaluating performance in most team sports. Movement can be evaluated across multiple scales, referring to the function of anatomical structures through various planes of motion or an individual regulating their field position based on the movement of opposition players. Developments in commercially available tracking systems have afforded end users the ability to investigate the spatiotemporal features of movement in fine detail. These advancements, in conjunction with overlaid contextual information, have provided insights into the strategies adopted by players in relation to their movement. Understanding movement beyond its semantic value allows practitioners to make informed decisions surrounding performance evaluation and training design. This investigation proposes a framework to guide the analysis of player movement within team sports environments. The framework describes how operational standards for assessing movement can be designed in reference to theory and a set training philosophy. Such practice allows for the spatial and temporal complexities within team sports to be described and could potentially lead to better-applied outcomes through greater interdisciplinary collaboration and an improved holistic understanding of movement. To inform its development, this study evaluates the current research and identifies several open questions to guide future investigations.
Understanding the features of player movement within team sports is a key component in athlete monitoring, performance evaluation, and training design processes (1). Determining what movement is and how to measure it effectively are fundamental requirements for developing strategies to improve sporting performance. Player movement is often defined and measured at varying levels of complexity across team sports and high-performance environments (2, 3). Developing a framework that acknowledges this complexity would allow for greater collaboration between disciplines by ensuring a shared language, potentially leading to better-applied outcomes.
Player movement can be measured across multiple scales, for example, the kinematic analysis of an anatomical structure's function through various planes of motion or positional descriptions of an individual in response to team tactical behaviour (4). In team-based invasion sports, movement is increasingly viewed through a spatiotemporal lens. Spatiotemporal data typically include the location of individuals or events with reference to space and time (5). Spatiotemporal features of movement can be described using one-dimensional derivatives of displacement, such as velocity, acceleration, and their angular properties; two-dimensional representations of manoeuvrability, such as tortuosity (5, 6); or three-dimensional depictions of limb kinematics (7), which are increasingly being used for in situ technique analysis, as well as various adjudication applications such as semi-automated offside assessment in football (8). As such, identifying a technology solution capable of measuring the intended movement feature with appropriate validity and reliability is paramount to successful evaluation.
Tracking systems such as Global Navigation Satellite Systems (GNSS), Local Positioning Systems (LPS), and Optical Tracking Systems (OTS) have provided the means to capture spatiotemporal information within indoor and outdoor team sports competitions. Such solutions provide positional data (longitude and latitude coordinates) and aggregated descriptors of movement, such as total running volume and maximal velocity. These systems often contain inertial measurement units (IMUs), which include a triaxial accelerometer, a triaxial gyroscope, and a triaxial magnetometer. IMUs have been used to assess kinematic movements such as stride length, flight time, and ground contact time, as well as acceleration metrics and the automatic detection of match phases (9, 10). A “player load” or “dynamic load” metric is an example of triaxial resultant information derived from an IMU being operationalised by a technology provider (11). Developments to these operating systems have seen capturing rates evolve from 1 to 100–300 Hz (9, 12, 13). In addition, computer vision research has brought about optical tracking algorithms that adopt deep learning models to produce ball or player-tracking solutions (14). Adoption of optical tracking solutions in sports varies due to unique sport-specific requirements. For example, greater revenue and salary cap confinements in the National Basketball Association (NBA), combined with controlled playing conditions (indoor environment, fixed camera operating systems, and fewer players to track), have granted relative access to the sport (15). Contrastingly, in Australian football, varying field sizes across the competition require significant camera infrastructure and therefore limit the viability of such a system (14).
This review aims to provide a framework for analysing players’ physical movement within team sports. To inform its development, this study evaluates the current research and identifies several open questions to guide future investigations.
To understand player movement beyond its semantic value, research has assessed the influence of various contexts. The constraint-led approach (CLA) provides an appropriate convention to categorise contexts previously used to measure sporting performance (2, 16). Constraints refer to the boundaries by which movement strategies emerge (2) and are categorised as individual, task, or environmental (17). Individual constraints refer to contextual factors that describe physical and technical attributes, historical descriptions of experience, and states of cognition (2). Task constraints describe rules and instructions and are related to the task goal (2, 18). Environmental constraints are external to the organism and refer to the physical or sociocultural conditions surrounding performance (2, 18). Table 1 provides a selection of constraints used to provide context to player movement in team sports. For example, understanding individual movement behaviour during offensive and defensive periods of play allows performance staff to make informed decisions regarding training load prescription, while understanding the influence of numerical imbalances may assist coaches in making more informed decisions when their team is influenced by injuries or player exclusions.
Table 1. Summary of various contexts reported in the literature for the analysis of player movement in team sports.
Research will likely continue to build on the body of work mentioned above, largely driven by rapid technology enhancements. While this alone will probably lead to incremental improvements in the understanding of player movement in team sports, access to more data also has the potential to lead to misuse and/or misinterpretation. As a result, additional attention being paid to the areas below will likely have an accelerative and perhaps more fundamental impact comparatively.
Technological improvements to commercially available tracking systems have afforded the end user the ability to answer related questions in greater detail. For example, near real-time access to limb tracking enables precise rule adjudication and technique analysis. Prior to these recent improvements, the utilisation of tracking data to date has often been limited to the use of discrete representations of movement. For example, speed is captured at 10 Hz via GNSS but is often reported as an average speed over the duration of a drill. This is likely partly due to the lack of computational power to deliver outputs in a timely manner. These representations include describing the movement as a volume of running within predetermined arbitrary velocity thresholds, counts of efforts above set thresholds, and measures of intensity derived by volume and duration metrics (107, 138, 139). Such representations of movement do not consider the emergent patterns within team sports and reduce human behaviour to isolated numerical values (140, 141). While value models and rule adjudication techniques in sports routinely use continuous movement data, their benefits are yet to be fully realised in routine practice. Discrete representations of movement are easily understood and acted upon, whereas continuous data require greater computation capacity but allow for the complexity within the sport to be accounted for. For example, previous examinations of maximal periods of movement have provided a guide to infer training intensity, whereas the automation of skeletal tracking data from competition would support the development of three-dimensional representations of movement during different components of the game (e.g., fast break, one-on-one situations, etc.). This actualises the potential for real-time coaching, omnipresent monitoring, more precise training prescription, and enhanced broadcast fan engagement features.
Another potential accelerator in this area relates to the implementation of theoretical frameworks to underpin player movement analysis. The adoption of an overarching, organisation-wide theoretical framework has been suggested as a method of unifying action across disciplines (16). Such a framework would offer an approach to combining operational methodologies and techniques alongside theory and a set training philosophy (2, 142, 143). It could also serve to coordinate and unify activity, redirect resource allocations, stimulate coherent interdisciplinary communication, and promote a more holistic understanding of sports performance (5, 144). The collaboration of subdiscipline specialists allows for analysis to be communicated through consistent language, which could influence the transfer of knowledge and ultimately improve performance (41). Within sports science, the integration of a framework allows scientific methodologies to reflect the broader training philosophy of organisations. Within the strength and conditioning discipline, it underpins exercise selection and progression. Within the coaching discipline, it underpins training design and performance evaluation, while in the medical discipline, it underpins rehabilitation design and physical screening.
Historically rooted in the physics literature, complex systems theory has recently been adopted by sports practitioners to underpin the analysis of movement (62, 121, 145, 146). This theory offers a framework through which a system’s stability, variability, and transition between fluid states can be described (147). Its adoption would be advantageous to sports performance, as this process-oriented philosophy embraces the complex constructs that operate within the team-sporting performance (147). Although all sports performance staff may benefit from its adoption, analysts and coaches are examples of two beneficiaries of complex systems research. The analysis of collective movement behaviour has enabled analysts to describe opposition ball movement methods and train accordingly, while the documentation of constraints on performance has permitted coaches to manipulate constraints in training to promote learning outcomes (148, 149). Complex systems theory describes athletes and teams as a complex adaptive system (CAS) whose behavioural properties continuously evolve in response to changing environments (12) and are unable to be understood outside of its context (150). In team-based sports, the theory recognises that individuals and teams regulate tactical behaviour in response to changing match constraints. It considers that control of tactical behaviour is distributed among different players and that individuals have the capacity to learn (4, 41, 151). Previous investigations have utilised a CAS approach to conceptualise team synergy in various sporting environments (13, 62, 121). Recent efforts to enhance interpretability have manifested in updated definitions and visual depictions of the 16 defining features of CAS theory (152). Complex systems theory provides a framework in which data analysis techniques can reference theory in the analysis of movement features. Table 2 provides an outline of the CAS features, their definitions, an analytical method that aligns with each feature, examples from the literature, and their proposed influence on practice.
Table 2. Matrix describing the 16 defining features of a complex systems theoretical approach and the analytical method through which they can influence practice.
More detailed data require not only greater computational but also analytical capacity. This permits moving beyond viewing sporting contexts in isolation and provides the ability to establish causal relationships between constraint groups or movement features (153). Such methodology offers a one-dimensional representation of movement while emphasising outcome behaviour and neglecting motives and causes (153). Recent investigations have adopted multivariate or time series approaches that address the temporal features and relatedness within constraints (18, 154–156). This allows the analytical process to be aligned to a feature within a CAS.
The proposed framework below (Table 3) can assist practitioners and researchers in extracting the most from player movement data while developing operational standards that align with a set training philosophy. An example using a complex systems theory to evaluate the change in running intensity throughout a match has been included as a case study.
Assuming progress in each of the above-mentioned areas, both practice and research are well-positioned to find solutions to further pervasive and impactful questions relating to player movement in team sports. A summary of some of these questions, many of which have already been posed in the literature, aimed at stimulating future investigations is provided as follows:
• OPEN QUESTION 1: Existing models for evaluating the difficulty of skilled actions have been made assessing the two-dimensional distance between ball carrier and receiver/target. Can a model be developed that accounts for three-dimensional space, the effect of ball spin and the role movement has on skill execution? (12) Such information would provide practitioners with a method of evaluating the complexities of skilled behaviour that could influence the training design and analysis processes.
• OPEN QUESTION 2: Investigations into player density have adopted commitment models to evaluate options afforded to players (20). How does physical capacity intersect with whether players should commit to a contest or not? Understanding the role physical capacity has on commitment would assist the development of density models that are reflective of human capacity. This would assist practitioners in making informed decisions around performance analysis and training design.
• OPEN QUESTION 3: Understanding an individual's intention is a key component to skill acquisition (172, 173). Individual intent is a constraint that shapes skill execution, and its identification allows coaches tactically to guide intention through instruction or drill design (16). Can spatiotemporal data provide a means to identify and evaluate a player's intentions and motivations? Whilst spatiotemporal data cannot identify underlying decision-making processes, it has the ability to identify relative risk tolerances, player passing option preferences and the quality of their decisions relative to other options. Understanding intent allows the quality of decision-making to be evaluated independently of skill execution and to guide the education process.
• OPEN QUESTION 4: High-performers are often referred to as having strong anticipatory skills (174, 175). How does the temporal component of decision-making influence outcomes and can spatiotemporal data provide insight into the temporal elements of decision-making risk and reward? Such information would infer the assessment of decision-making qualities and guide education and training processes.
• OPEN QUESTION 5: Spatiotemporal data actualises the potential for real-time omnipresent monitoring during training and match play. Can spatiotemporal data be used to identify meaningful fatigue before it occurs within open match play? Such information would allow coaches to optimise within-match rotation policies and potentially lead to better match outcomes.
• OPEN QUESTION 6: The definition of, and subsequent methods of measuring momentum in team sports is a largely unrefined research area. Providing a clear definition and a method of measuring such phenomenon allows end users to attempt to control its influence on match outcome. Can motion models infer momentum control and the techniques used to generate or retain it? Such information would help infer strategy and training design.
• OPEN QUESTION 7: Artificial intelligence (AI) models have the ability to consider information independently of human input and offer an alternative insight to a coach (176, 177). Understanding the differences in human–machine judgements within the coaching discipline is a fundamental component to building a symbiotic relationship between AI and sport moving forward. Can a model built on spatiotemporal data identify individual instantaneous critical fatigue, collective momentum, player form and opposition strategy, to inform which changes to player formation and strategy are required to improve the probability of match outcomes in real time? What are the associated risks involved? And how do these judgements differ from a coach's subjective model? Such models would operate as a decision-support system to enhance match outcomes, whilst concurrently informing the human–machine relationship within the coaching discipline.
• OPEN QUESTION 8: Within team-sport open-play scenarios, movement can be considered respective of the dynamic regulation of positioning in response to generating offensive or preserving defensive stability (107, 178). As such, training may foster environments that encourage desirable individual and collective spatial behaviour. How can spatiotemporal data infer individual or collective learning outcomes within open-play scenarios? Such information would assist with the evaluation of training outcomes and assessment of player development.
• OPEN QUESTION 9: As sporting organisations continually refine resourcing related to data architecture and processes, it is important to prioritise data sources that influence decision-making and performance. If we consider that physiological data could be used to compliment player tracking data, how would we establish the weight of each variable on performance? Generating a system to evaluate the influence of new data would allow organisations to prioritise resources, increase player performance, and enhance further innovation within the performance domain.
As long as technology continues to develop, the collection and analysis of spatiotemporal data will remain a common practice in high-performance sporting environments. Given this persistent nature, it is imperative that the analysis and description of player movement reflect not only the underlying physical quality but also the broader department philosophy rather than being reactive to resource availability. The merger of a set training philosophy with appropriate data analytical tools allows for the assessment of the complex features of movement in team sports. The stated open questions illustrate nascent areas of sports practice in which benefits could be actualised through the unification of theory and analytics. Such practice describes a methodology where data analysts operate within the confinements of a broader department-shared philosophy when analysing human physical movement. Ultimately, this promotion of interdisciplinary collaboration has the potential to achieve better-applied outcomes for players and organisations.
SP: Conceptualization, Writing – original draft, Writing – review & editing. GD: Conceptualization, Writing – review & editing. SR: Conceptualization, Writing – review & editing.
The authors declare that no financial support was received for the research, authorship, and/or publication of this article.
The authors declare that the research was conducted in the absence of any commercial or financial relationships that could be construed as a potential conflict of interest.
All claims expressed in this article are solely those of the authors and do not necessarily represent those of their affiliated organizations, or those of the publisher, the editors and the reviewers. Any product that may be evaluated in this article, or claim that may be made by its manufacturer, is not guaranteed or endorsed by the publisher.
1. Gregory S, Robertson S, Aughey R, Duthie G. The influence of tactical and match context on player movement in football. J Sports Sci. (2022) 40(9):1063–77. doi: 10.1080/02640414.2022.2046938
2. Browne P, Sweeting AJ, Woods CT, Robertson S. Methodological considerations for furthering the understanding of constraints in applied sports. Sports Med Open. (2021) 7(1):22. doi: 10.1186/s40798-021-00313-x
3. Rothwell M, Davids K, Stone J, O’Sullivan M, Vaughan J, Newcombe D, et al. A department of methodology can coordinate transdisciplinary sport science support. J Expert. (2020) 3(1):55–65.
4. Pol R, Balague N, Ric A, Torrents C, Kiely J, Hristovski R. Training or synergizing? Complex systems principles change the understanding of sport processes. Sports Med Open. (2020) 6(1):28. doi: 10.1186/s40798-020-00256-9
5. Rein R, Memmert D. Big data and tactical analysis in elite soccer: future challenges and opportunities for sports science. Springerplus. (2016) 5(1):1410. doi: 10.1186/s40064-016-3108-2
6. Duthie GM, Robertson S, Thornton HR. A GNSS-based method to define athlete manoeuvrability in field-based team sports. PLoS One. (2021) 16(11):e0260363. doi: 10.1371/journal.pone.0260363
7. Soltani P, Morice AH. A multi-scale analysis of basketball throw in virtual reality for tracking perceptual-motor expertise. Scand J Med Sci Sports. (2023) 33(2):178–88. doi: 10.1111/sms.14250
8. Association, FIFA. Semi-Automated Offside Technology. (2023). Available online at: https://www.fifa.com/technical/football-technology/football-technologies-and-innovations-at-the-fifa-world-cup-2022/semi-automated-offside-technology (Accessed November 22, 2023).
9. Norris M, Anderson R, Kenny IC. Method analysis of accelerometers and gyroscopes in running gait: a systematic review. Proc Inst Mech Eng Pt P J Sports Eng Tech. (2014) 228(1):3–15. doi: 10.1177/1754337113502472
10. Lefèvre T, Guignard B, Karcher C, Reche X, Font R, Komar J. A deep dive into the use of local positioning system in professional handball: automatic detection of players’ orientation, position and game phases to analyse specific physical demands. PLoS One. (2023) 18(8):e0289752. doi: 10.1371/journal.pone.0289752
11. Bredt SdGT, Chagas MH, Peixoto GH, Menzel HJ, de Andrade AGP. Understanding player load: meanings and limitations. J Hum Kinet. (2020) 71:5. doi: 10.2478/hukin-2019-0072
12. Gudmundsson J, Horton M. Spatio-temporal analysis of team sports. ACM Comput Surv (CSUR). (2017) 50(2):1–34. doi: 10.1145/3054132
13. Sheehan WB, Tribolet R, Novak AR, Fransen J, Watsford ML. A holistic analysis of collective behaviour and team performance in Australian football via structural equation modelling. Sci Med Footb. (2023) 7(1):64–73. doi: 10.1080/24733938.2022.2046286
14. Torres-Ronda L, Beanland E, Whitehead S, Sweeting A, Clubb J. Tracking systems in team sports: a narrative review of applications of the data and sport specific analysis. Sports Med Open. (2022) 8(1):15. doi: 10.1186/s40798-022-00408-z
15. Sangüesa AA, Moeslund TB, Bahnsen CH, Iglesias RB. Identifying basketball plays from sensor data; towards a low-cost automatic extraction of advanced statistics. Paper Presented at the 2017 IEEE International Conference on Data Mining Workshops (ICDMW); New Orleans, LA, USA (2017).
16. Glazier PS. Towards a grand unified theory of sports performance. Hum Mov Sci. (2017) 56(Pt A):139–56. doi: 10.1016/j.humov.2015.08.001
17. Woods CT, Rudd J, Robertson S, Davids K. Wayfinding: how ecological perspectives of navigating dynamic environments can enrich our understanding of the learner and the learning process in sport. Sports Med Open. (2020) 6(1):51. doi: 10.1186/s40798-020-00280-9
18. Balague N, Pol R, Torrents C, Ric A, Hristovski R. On the relatedness and nestedness of constraints. Sports Med Open. (2019) 5(1):6. doi: 10.1186/s40798-019-0178-z
19. Fernandez J, Bornn L. Wide open spaces: a statistical technique for measuring space creation in professional soccer. Paper Presented at the Sloan Sports Analytics Conference; Boston USA (2018).
20. Spencer B, Jackson K, Robertson S. Fitting motion models to contextual player behavior. Proceedings of the 12th International Symposium on Computer Science in Sport (Iacss 2019), 1028 (2020). p. 11–8. doi: 10.1007/978-3-030-35048-2_2
21. Cervone D, D’Amour A, Bornn L, Goldsberry K. Pointwise: predicting points and valuing decisions in real time with nba optical tracking data. Paper Presented at the Proceedings of the 8th MIT Sloan Sports Analytics Conference; Boston, MA, USA (2014).
22. Spencer B, Bedin T, Farrow D, Jackson K. A method for evaluating player decision-making in the Australian football league. Paper Presented at the Proceedings of 14th Australasian Conference on Mathematics and Computers in Sport, (Sippy Downs, QLD: University of the Sunshine Coast). Sippy Downs, QLD: University of the Sunshine Coast (2018).
23. Spencer B, Jackson K, Bedin T, Robertson S. Modeling the quality of player passing decisions in Australian rules football relative to risk, reward, and commitment. Front Psychol. (2019) 10:1777. doi: 10.3389/fpsyg.2019.01777
24. Power P, Ruiz H, Wei X, Lucey P. Not all passes are created equal: objectively measuring the risk and reward of passes in soccer from tracking data. Paper Presented at the Proceedings of the 23rd ACM SIGKDD International Conference on Knowledge Discovery and Data Mining; New York, NY, United States. Sippy Downs, QLD: University of the Sunshine Coast (2017).
25. Vilar L, Araújo D, Davids K, Correia V, Esteves PT. Spatial-temporal constraints on decision-making during shooting performance in the team sport of futsal. J Sports Sci. (2013) 31(8):840–6. doi: 10.1080/02640414.2012.753155
26. Alexander JP, Spencer B, Sweeting AJ, Mara JK, Robertson S. The influence of match phase and field position on collective team behaviour in Australian rules football. J Sports Sci. (2019) 37(15):1699–707. doi: 10.1080/02640414.2019.1586077
27. Vella A, Clarke AC, Kempton T, Ryan S, Coutts AJ. Assessment of physical, technical, and tactical analysis in the Australian football league: a systematic review. Sports Med Open. (2022) 8(1):124. doi: 10.1186/s40798-022-00518-8
28. Vella A, Clarke AC, Kempton T, Ryan S, Holden J, Coutts AJ. Possession chain factors influence movement demands in elite Australian football match-play. Sci Med Footb. (2021) 5(1):72–8. doi: 10.1080/24733938.2020.1795235
29. Gronow D, Dawson B, Heasman J, Rogalski B, Peeling P. Team movement patterns with and without ball possession in Australian football league players. Int J Perform Anal Sport. (2014) 14(3):635–51. doi: 10.1080/24748668.2014.11868749
30. Sheehan W, Tribolet R, Novak AR, Fransen J, Watsford ML. An assessment of physical and spatiotemporal behaviour during different phases of match play in professional Australian football. J Sports Sci. (2021) 39(19):2232–41. doi: 10.1080/02640414.2021.1928408
31. Rennie MJ, Kelly SJ, Bush S, Spurrs RW, Austin DJ, Watsford ML. Phases of match-play in professional Australian football: distribution of physical and technical performance. J Sports Sci. (2020) 38(14):1682–9. doi: 10.1080/02640414.2020.1754726
32. Gregory S. Ready Player Run: Off-Ball Run Identification and Classification. Barça Sport Analytics Summit (2019).
33. Gabbett TJ, Polley C, Dwyer DB, Kearney S, Corvo A. Influence of field position and phase of play on the physical demands of match-play in professional rugby league forwards. J Sci Med Sport. (2014) 17(5):556–61. doi: 10.1016/j.jsams.2013.08.002
34. Read DB, Jones B, Williams S, Phibbs PJ, Darrall-Jones JD, Roe GA, et al. The physical characteristics of specific phases of play during rugby union match play. Int J Sports Physiol Perform. (2018) 13(10):1331–6. doi: 10.1123/ijspp.2017-0625
35. Ferioli D, Rampinini E, Martin M, Rucco D, La Torre A, Petway A, et al. Influence of ball possession and playing position on the physical demands encountered during professional basketball games. Biol Sport. (2020) 37(3):269–76. doi: 10.5114/biolsport.2020.95638
36. Sheehan WB, Tribolet R, Watsford ML, Novak AR, Rennie M, Fransen J. Tactical analysis of individual and team behaviour in professional Australian football: original investigation. Sci Med Footb. (2022) 6(2):172–80. doi: 10.1080/24733938.2021.1923792
37. Ribeiro J, Lopes R, Silva P, Araujo D, Barreira D, Davids K, et al. A multilevel hypernetworks approach to capture meso-level synchronisation processes in football. J Sports Sci. (2020) 38(5):494–502. doi: 10.1080/02640414.2019.1707399
38. Silva P, Chung D, Carvalho T, Cardoso T, Davids K, Araujo D, et al. Practice effects on intra-team synergies in football teams. Hum Mov Sci. (2016) 46:39–51. doi: 10.1016/j.humov.2015.11.017
39. Clemente MF, Couceiro SM, Martins FM, Mendes R, Figueiredo AJ. Measuring collective behaviour in football teams: inspecting the impact of each half of the match on ball possession. Int J Perform Anal Sport. (2013) 13(3):678–89. doi: 10.1080/24748668.2013.11868680
40. Bourbousson J, Sève C, McGarry T. Space–time coordination dynamics in basketball: part 2. The interaction between the two teams. J Sports Sci. (2010b) 28(3):349–58. doi: 10.1080/02640410903503640
41. Vella A, Clarke AC, Kempton T, Ryan S, Holden J, Coutts AJ. Technical involvements and pressure applied influence movement demands in elite Australian football match-play. Sci Med Footb. (2022) 6(2):228–33. doi: 10.1080/24733938.2021.1942537
42. Wing C, Hart NH, Ma'ayah F, Nosaka K. Physical and technical demands of offence, defence, and contested phases of play in Australian football. BMC Sports Sci Med Rehabil. (2022b) 14(1):33. doi: 10.1186/s13102-022-00425-1
43. Llana S, Burriel B, Madrero P, Fernández J. Is it worth the effort? Understanding and contextualizing physical metrics in soccer. arXiv preprint arXiv. 2204.02313. (2022).
44. Travassos B, Monteiro R, Coutinho D, Yousefian F, Goncalves B. How spatial constraints afford successful and unsuccessful penetrative passes in elite association football. Sci Med Footb. (2023) 7(2):157–64. doi: 10.1080/24733938.2022.2060519
45. Wing C, Hart NH, Ma'ayah F, Nosaka K. Physical and technical demands of Australian football: an analysis of maximum ball in play periods. BMC Sports Sci Med Rehabil. (2022) 14(1):15. doi: 10.1186/s13102-022-00405-5
46. Esmaeili A, Clifton P, Aughey RJ. A league-wide evaluation of factors influencing match activity profile in elite Australian football. Front Sports Act Living. (2020) 2:579264. doi: 10.3389/fspor.2020.579264
47. Corbett DM, Sweeting AJ, Robertson S. Weak relationships between stint duration, physical and skilled match performance in Australian football. Front Physiol. (2017) 8:820. doi: 10.3389/fphys.2017.00820
48. Dillon PA, Kempton T, Ryan S, Hocking J, Coutts AJ. Interchange rotation factors and player characteristics influence physical and technical performance in professional Australian rules football. J Sci Med Sport. (2018) 21(3):317–21. doi: 10.1016/j.jsams.2017.06.008
49. Montgomery PG, Wisbey B. The effect of interchange rotation period and number on Australian football running performance. J Strength Cond Res. (2016) 30(7):1890–7. doi: 10.1519/Jsc.0000000000000597
50. Bradley PS, Noakes TD. Match running performance fluctuations in elite soccer: indicative of fatigue, pacing or situational influences? J Sports Sci. (2013) 31(15):1627–38. doi: 10.1080/02640414.2013.796062
51. Moreira A, Saldanha Aoki M, Carling C, Alan Rodrigues Lopes R, Felipe Schultz de Arruda A, Lima M, et al. Temporal changes in technical and physical performances during a small-sided game in elite youth soccer players. Asian J Sports Med. (2016) 7(4):e35411. doi: 10.5812/asjsm.35411
52. García F, Vázquez-Guerrero J, Castellano J, Casals M, Schelling X. Differences in physical demands between game quarters and playing positions on professional basketball players during official competition. J Sports Sci Med. (2020) 19(2):256.
53. Dalton-Barron N, Palczewska A, Weaving D, Rennie G, Beggs C, Roe G, et al. Clustering of match running and performance indicators to assess between-and within-playing position similarity in professional rugby league. J Sports Sci. (2022) 40(15):1712–21. doi: 10.1080/02640414.2022.2100781
54. Ade JD, Drust B, Morgan OJ, Bradley PS. Physiological characteristics and acute fatigue associated with position-specific speed endurance soccer drills: production vs maintenance training. Sci Med Footb. (2021) 5(1):6–17. doi: 10.1080/24733938.2020.1789202
55. Ward PA, Ramsden S, Coutts AJ, Hulton AT, Drust B. Positional differences in running and nonrunning activities during elite American football training. J Strength Cond Res. (2018) 32(7):2072–84. doi: 10.1519/JSC.0000000000002294
56. Lignell E, Fransson D, Krustrup P, Mohr M. Analysis of high-intensity skating in top-class ice hockey match-play in relation to training status and muscle damage. J Strength Cond Res. (2018) 32(5):1303–10. doi: 10.1519/JSC.0000000000001999
57. Alexander JP, Spencer B, Mara JK, Robertson S. Collective team behaviour of Australian rules football during phases of match play. J Sports Sci. (2019) 37(3):237–43. doi: 10.1080/02640414.2018.1491113
58. Spencer B, Morgan S, Zeleznikow J, Robertson S. Measuring player density in Australian rules football using Gaussian mixture models. Paper Presented at the Proceedings of the Complex Systems in Sport, International Congress Linking Theory and Practice; Barcelona (2017).
59. Alexander JP, Jackson KB, Bedin T, Gloster MA, Robertson S. Quantifying congestion with player tracking data in Australian football. PLoS One. (2022) 17(8):e0272657. doi: 10.1371/journal.pone.0272657
60. Duarte R, Araújo D, Folgado H, Esteves P, Marques P, Davids K. Capturing complex, non-linear team behaviours during competitive football performance. J Syst Sci Complex. (2013) 26(1):62–72. doi: 10.1007/s11424-013-2290-3
61. Maneiro R, Blanco-Villaseñor Á, Amatria M. Analysis of the variability of the game space in high performance football: implementation of the generalizability theory. Front Psychol. (2020) 11:534. doi: 10.3389/fpsyg.2020.00534
62. Ric A, Torrents C, Gonçalves B, Torres-Ronda L, Sampaio J, Hristovski R. Dynamics of tactical behaviour in association football when manipulating players’ space of interaction. PLoS One. (2017) 12(7):e0180773.28708868
63. Veale JP, Pearce AJ, Carlson JS. Player movement patterns in an elite junior Australian rules football team: an exploratory study. J Sports Sci Med. (2007) 6(2):254–60. Available online at: https://www.ncbi.nlm.nih.gov/pubmed/2414933724149337
64. Black GM, Gabbett TJ, Naughton GA, McLean BD. The effect of intense exercise periods on physical and technical performance during elite Australian football match-play: a comparison of experienced and less experienced players. J Sci Med Sport. (2016) 19(7):596–602. doi: 10.1016/j.jsams.2015.07.007
65. Brewer C, Dawson B, Heasman J, Stewart G, Cormack S. Movement pattern comparisons in elite (AFL) and sub-elite (WAFL) Australian football games using GPS. J Sci Med Sport. (2010) 13(6):618–23. doi: 10.1016/j.jsams.2010.01.005
66. Aughey RJ. Widening margin in activity profile between elite and sub-elite Australian football: a case study. J Sci Med Sport. (2013) 16(4):382–6. doi: 10.1016/j.jsams.2012.10.003
67. Bradley PS, Di Mascio M, Peart D, Olsen P, Sheldon B. High-intensity activity profiles of elite soccer players at different performance levels. J Strength Cond Res. (2010) 24(9):2343–51. doi: 10.1519/JSC.0b013e3181aeb1b3
68. Bradley PS, Carling C, Gomez Diaz A, Hood P, Barnes C, Ade J, et al. Match performance and physical capacity of players in the top three competitive standards of English professional soccer. Hum Mov Sci. (2013) 32(4):808–21. doi: 10.1016/j.humov.2013.06.002
69. Bradley PS, Archer DT, Hogg B, Schuth G, Bush M, Carling C, et al. Tier-specific evolution of match performance characteristics in the English premier league: it’s getting tougher at the top. J Sports Sci. (2016) 34(10):980–7. doi: 10.1080/02640414.2015.1082614
70. Cormack SJ, Smith RL, Mooney MM, Young WB, O’Brien BJ. Accelerometer load as a measure of activity profile in different standards of netball match play. Int J Sports Physiol Perform. (2014) 9(2):283–91. doi: 10.1123/ijspp.2012-0216
71. Johnston RD, Thornton HR, Wade JA, Devlin P, Duthie GM. The distribution of match activities relative to the maximal mean intensities in professional rugby league and Australian football. J Strength Cond Res. (2022) 36(5):1360–6. doi: 10.1519/JSC.0000000000003613
72. Johnston RD, Murray NB, Austin DJ, Duthie G. Peak movement and technical demands of professional Australian football competition. J Strength Cond Res. (2021) 35(10):2818–23. doi: 10.1519/JSC.0000000000003241
73. Delaney JA, Thornton HR, Burgess DJ, Dascombe BJ, Duthie GM. Duration-specific running intensities of Australian football match-play. J Sci Med Sport. (2017) 20(7):689–94. doi: 10.1016/j.jsams.2016.11.009
74. Sanders GJ, Roll B, Peacock CA, Kollock RO. Maximum movement workloads and high-intensity workload demands by position in NCAA division I collegiate football. J Strength Cond Res. (2020) 34(7):1974–81. doi: 10.1519/JSC.0000000000002611
75. Delves RI, Bahnisch J, Ball K, Duthie GM. Quantifying mean peak running intensities in elite field hockey. J Strength Cond Res. (2021) 35(9):2604–10. doi: 10.1519/JSC.0000000000003162
76. McLean BD, Strack D, Russell J, Coutts AJ. Quantifying physical demands in the national basketball association—challenges around developing best-practice models for athlete care and performance. Int J Sports Physiol Perform. (2019) 14(4):414–20. doi: 10.1123/ijspp.2018-0384
77. Roell M, Helwig J, Gollhofer A, Roecker K. Duration-specific peak acceleration demands during professional female basketball matches. Front Sports Act Living. (2020) 2:33. doi: 10.3389/fspor.2020.00033
78. Graham S, Zois J, Aughey R, Duthie G. The peak player load™ of state-level netball matches. J Sci Med Sport. (2020) 23(2):189–93. doi: 10.1016/j.jsams.2019.09.014
79. Calder AR, Duthie GM, Johnston RD, Engel HD. Physical demands of female collegiate lacrosse competition: whole-match and peak periods analysis. Sport Sci Health. (2021) 17:103–9. doi: 10.1007/s11332-020-00659-x
80. Ryan S, Coutts AJ, Hocking J, Dillon PA, Whitty A, Kempton T. Physical preparation factors that influence technical and physical match performance in professional Australian football. Int J Sports Physiol Perform. (2018) 13(8):1021–7. doi: 10.1123/ijspp.2017-0640
81. Mooney M, Cormack S, O'Brien B, Coutts AJ. Do physical capacity and interchange rest periods influence match exercise-intensity profile in Australian football? Int J Sports Physiol Perform. (2013) 8(2):165–72. doi: 10.1123/ijspp.8.2.165
82. Duthie GM, Thornton HR, Delaney JA, McMahon JT, Benton DT. Relationship between physical performance testing results and peak running intensity during professional rugby league match play. J Strength Cond Res. (2020) 34(12):3506–13. doi: 10.1519/JSC.0000000000002273
83. Gabbett TJ, Stein JG, Kemp JG, Lorenzen C. Relationship between tests of physical qualities and physical match performance in elite rugby league players. J Strength Cond Res. (2013) 27(6):1539–45. doi: 10.1519/JSC.0b013e318274f236
84. Stares J, Dawson B, Heasman J, Rogalski B. Relationship between pre-season strength and power measures and performance in elite Australian football. Int J Perform Anal Sport. (2015) 15(3):777–93. doi: 10.1080/24748668.2015.11868830
85. Baker DG, Newton RU. Comparison of lower body strength, power, acceleration, speed, agility, and sprint momentum to describe and compare playing rank among professional rugby league players. J Strength Cond Res. (2008) 22(1):153–8. doi: 10.1519/JSC.0b013e31815f9519
86. Peterson BJ, Fitzgerald JS, Dietz CC, Ziegler KS, Baker SE, Snyder EM. Off-ice anaerobic power does not predict on-ice repeated shift performance in hockey. J Strength Cond Res. (2016) 30(9):2375–81. doi: 10.1519/JSC.0000000000001341
87. Van de Casteele F, Deprez D, Van Haaren J, Derave W, Lievens E. In professional football the decline in high-intensity running activities from first to second half is more pronounced in players with a fast muscle typology. Scand J Med Sci Sports. (2023) 34:1.
88. Bradley PS, Vescovi JD. Velocity thresholds for women’s soccer matches: sex specificity dictates high-speed running and sprinting thresholds—female athletes in motion (FAiM). Int J Sports Physiol Perform. (2015) 10(1):112–6. doi: 10.1123/ijspp.2014-0212
89. Bradley PS, Dellal A, Mohr M, Castellano J, Wilkie A. Gender differences in match performance characteristics of soccer players competing in the UEFA champions league. Hum Mov Sci. (2014) 33:159–71. doi: 10.1016/j.humov.2013.07.024
90. Hopwood HJ, Bellinger PM, Compton HR, Bourne MN, Derave W, Lievens E, et al. Match running performance in Australian football is related to muscle fiber typology. Int J Sports Physiol Perform. (2023) 18(12):1442–8. doi: 10.1123/ijspp.2023-0014
91. Johnston RD, Murray NB, Austin DJ. The influence of pre-season training loads on in-season match activities in professional Australian football players. Sci Med Footb. (2019) 3(2):143–9. doi: 10.1080/24733938.2018.1501160
92. Crang ZL, Hewitt A, Scott TJ, Kelly VG, Johnston RD. Relationship between preseason training load, match performance, and match activities in professional rugby league. J Strength Cond Res. (2022) 36(9):2581–8. doi: 10.1519/JSC.0000000000003891
93. Booth M, Cobley S, Orr R. The effect of training loads on performance measures and injury characteristics in rugby league players: a systematic review. Int J Sports Physiol Perform. (2018) 13(10):1259–72. doi: 10.1123/ijspp.2017-0329
94. Bradley PS, Lago-Penas C, Rey E. Evaluation of the match performances of substitution players in elite soccer. Int J Sports Physiol Perform. (2014) 9(3):415–24. doi: 10.1123/ijspp.2013-0304
95. Schuth G, Carr G, Barnes C, Carling C, Bradley PS. Positional interchanges influence the physical and technical match performance variables of elite soccer players. J Sports Sci. (2016) 34(6):501–8. doi: 10.1080/02640414.2015.1127402
96. Delaney JA, Thornton HR, Duthie GM, Dascombe BJ. Factors that influence running intensity in interchange players in professional rugby league. Int J Sports Physiol Perform. (2016) 11(8):1047–52. doi: 10.1123/ijspp.2015-0559
97. Thornton HR, Duthie GM, Pitchford NW, Delaney JA, Benton DT, Dascombe BJ. Effects of a 2-week high-intensity training camp on sleep activity of professional rugby league athletes. Int J Sports Physiol Perform. (2017) 12(7):928–33. doi: 10.1123/ijspp.2016-0414
98. Bellinger PM, Ferguson C, Newans T, Minahan CL. No influence of prematch subjective wellness ratings on external load during elite Australian football match play. Int J Sports Physiol Perform. (2020) 15(6):801–7. doi: 10.1123/ijspp.2019-0395
99. McGuinness A, McMahon G, Malone S, Kenna D, Passmore D, Collins K. Monitoring wellness, training load, and running performance during a major international female field hockey tournament. J Strength Cond Res. (2020) 34(8):2312–20. doi: 10.1519/JSC.0000000000002835
100. Wellman AD, Coad SC, Flynn PJ, Climstein M, McLellan CP. Movement demands and perceived wellness associated with preseason training camp in NCAA division I college football players. J Strength Cond Res. (2017) 31(10):2704–18. doi: 10.1519/JSC.0000000000002106
101. Corbett DM, Sweeting AJ, Robertson S. A change point approach to analysing the match activity profiles of team-sport athletes. J Sports Sci. (2019) 37(14):1600–8. doi: 10.1080/02640414.2019.1577941
102. Teune B, Woods C, Sweeting A, Inness M, Robertson S. A method to inform team sport training activity duration with change point analysis. PLoS One. (2022) 17(3):e0265848. doi: 10.1371/journal.pone.0265848
103. Cormack SJ, Mooney MG, Morgan W, McGuigan MR. Influence of neuromuscular fatigue on accelerometer load in elite Australian football players. Int J Sports Physiol Perform. (2013) 8(4):373–8. doi: 10.1123/ijspp.8.4.373
104. Mooney MG, Cormack S, O'Brien BJ, Morgan WM, McGuigan M. Impact of neuromuscular fatigue on match exercise intensity and performance in elite Australian football. J Strength Cond Res. (2013) 27(1):166–73. doi: 10.1519/JSC.0b013e3182514683
105. Rowell AE, Aughey RJ, Hopkins WG, Esmaeili A, Lazarus BH, Cormack SJ. Effects of training and competition load on neuromuscular recovery, testosterone, cortisol, and match performance during a season of professional football. Front Physiol. (2018) 9:668. doi: 10.3389/fphys.2018.00668
106. Silva N, Travassos B, Gonçalves B, Brito J, Nakamura F, Abade E. Effects of warm-up duration on acute physical performance in highly trained male futsal players. Int J Sports Sci Coach. (2023) 36:17479541231208009.
107. Alexander JP, Bedin T, Jackson KB, Robertson S. Team numerical advantage in Australian rules football: a missing piece of the scoring puzzle? PLoS One. (2021) 16(7):e0254591. doi: 10.1371/journal.pone.0254591
108. Carling C, Bloomfield J. The effect of an early dismissal on player work-rate in a professional soccer match. J Sci Med Sport. (2010) 13(1):126–8. doi: 10.1016/j.jsams.2008.09.004
109. Lago C. The influence of match location, quality of opposition, and match status on possession strategies in professional association football. J Sports Sci. (2009) 27(13):1463–9. doi: 10.1080/02640410903131681
110. Gabbett TJ. Influence of the opposing team on the physical demands of elite rugby league match play. J Strength Cond Res. (2013) 27(6):1629–35. doi: 10.1519/JSC.0b013e318274f30e
111. Kempton T, Sirotic AC, Coutts AJ. A comparison of physical and technical performance profiles between successful and less-successful professional rugby league teams. Int J Sports Physiol Perform. (2017) 12(4):520–6. doi: 10.1123/ijspp.2016-0003
112. Aughey RJ. Increased high-intensity activity in elite Australian football finals matches. Int J Sports Physiol Perform. (2011) 6(3):367–79. doi: 10.1123/ijspp.6.3.367
113. Hulin BT, Gabbett TJ, Kearney S, Corvo A. Physical demands of match play in successful and less-successful elite rugby league teams. Int J Sports Physiol Perform. (2015) 10(6):703–10. doi: 10.1123/ijspp.2014-0080
114. Wellman AD, Coad SC, Flynn PJ, Siam TK, McLellan CP. Comparison of preseason and in-season practice and game loads in national collegiate athletic association division I football players. J Strength Cond Res. (2019) 33(4):1020–7. doi: 10.1519/JSC.0000000000002173
115. Fox A, Spittle M, Otago L, Saunders N. Activity profiles of the Australian female netball team players during international competition: implications for training practice. J Sports Sci. (2013) 31(14):1588–95. doi: 10.1080/02640414.2013.792943
116. Sullivan C, Bilsborough JC, Cianciosi M, Hocking J, Cordy J, Coutts AJ. Match score affects activity profile and skill performance in professional Australian football players. J Sci Med Sport. (2014) 17(3):326–31. doi: 10.1016/j.jsams.2013.05.001
117. Chmura P, Konefał M, Chmura J, Kowalczuk E, Zając T, Rokita A, et al. Match outcome and running performance in different intensity ranges among elite soccer players. Biol Sport. (2018) 35(2):197–203. doi: 10.5114/biolsport.2018.74196
118. Lorenzo-Martínez M, de Dios-Álvarez VM, Padrón-Cabo A, Costa PB, Rey E. Effects of score-line on internal and external load in soccer small-sided games. Int J Perform Anal Sport. (2020) 20(2):231–9. doi: 10.1080/24748668.2020.1736938
119. Douglas A, Johnston K, Baker J, Rotondi MA, Jamnik VK, Macpherson AK. On-ice measures of external load in relation to match outcome in elite female ice hockey. Sports. (2019) 7(7):173. doi: 10.3390/sports7070173
120. Bourbousson J, Sève C, McGarry T. Space–time coordination dynamics in basketball: part 1. Intra-and inter-couplings among player dyads. J Sports Sci. (2010a) 28(3):339–47. doi: 10.1080/02640410903503632
121. Ramos J, Lopes RJ, Araújo D. What’s next in complex networks? Capturing the concept of attacking play in invasive team sports. Sports Med. (2018) 48:17–28. doi: 10.1007/s40279-017-0786-z
122. Sheehan WB, Tribolet R, Watsford ML, Novak AR, Rennie MJ, Fransen J. Using cooperative networks to analyse behaviour in professional Australian football. J Sci Med Sport. (2020) 23(3):291–6. doi: 10.1016/j.jsams.2019.09.012
123. Vilar L, Araujo D, Davids K, Travassos B. Constraints on competitive performance of attacker–defender dyads in team sports. J Sports Sci. (2012) 30(5):459–69. doi: 10.1080/02640414.2011.627942
124. Bradley PS, Carling C, Archer D, Roberts J, Dodds A, Di Mascio M, et al. The effect of playing formation on high-intensity running and technical profiles in English FA premier league soccer matches. J Sports Sci. (2011) 29(8):821–30. doi: 10.1080/02640414.2011.561868
125. Bradley PS, Lago-Penas C, Rey E, Gomez Diaz A. The effect of high and low percentage ball possession on physical and technical profiles in English FA premier league soccer matches. J Sports Sci. (2013) 31(12):1261–70. doi: 10.1080/02640414.2013.786185
126. Ötting M, Karlis D. Football tracking data: a copula-based hidden Markov model for classification of tactics in football. Ann Oper Res. (2023) 325(1):167–83. doi: 10.1007/s10479-022-04660-0
127. Yu D, Boucher C, Bornn L, Javan M. Playing Fast Not Loose: Evaluating team-level pace of play in ice hockey using spatio-temporal possession data. arXiv preprint arXiv:1902.02020. (2019).
128. Ribeiro JN, Yousefian F, Illa J, Couceiro M, Sampaio J, Travassos B. The effects of players’ rotations on high-intensity activities in professional futsal players. J Hum Kinet. (2024) 90(90):215–26.38380292
129. Aughey RJ, Goodman CA, McKenna MJ. Greater chance of high core temperatures with modified pacing strategy during team sport in the heat. J Sci Med Sport. (2014) 17(1):113–8. doi: 10.1016/j.jsams.2013.02.013
130. Mohr M, Krustrup P, Nybo L, Nielsen JJ, Bangsbo J. Muscle temperature and sprint performance during soccer matches–beneficial effect of re-warm-up at half-time. Scand J Med Sci Sports. (2004) 14(3):156–62. doi: 10.1111/j.1600-0838.2004.00349.x
131. Hiscock D, Dawson B, Heasman J, Peeling P. Game movements and player performance in the Australian football league. Int J Perform Anal Sport. (2012) 12(3):531–45. doi: 10.1080/24748668.2012.11868617
132. Lago C, Casais L, Dominguez E, Sampaio J. The effects of situational variables on distance covered at various speeds in elite soccer. Eur J Sport Sci. (2010) 10(2):103–9. doi: 10.1080/17461390903273994
133. Murray NB, Gabbett TJ, Chamari K. Effect of different between-match recovery times on the activity profiles and injury rates of national rugby league players. J Strength Cond Res. (2014) 28(12):3476–83. doi: 10.1519/JSC.0000000000000603
134. Dupont G, Nedelec M, McCall A, McCormack D, Berthoin S, Wisløff U. Effect of 2 soccer matches in a week on physical performance and injury rate. Am J Sports Med. (2010) 38(9):1752–8. doi: 10.1177/0363546510361236
135. Quinn M, Sinclair J, Atkins S. Differences in the high speed match-play characteristics of rugby league players before, during and after a period of tramseridian transition. Int J Perform Anal Sport. (2015) 15(3):1065–76. doi: 10.1080/24748668.2015.11868851
136. Stone KJ, Hughes MG, Stembridge MR, Meyers RW, Newcombe DJ, Oliver JL. The influence of playing surface on physiological and performance responses during and after soccer simulation. Eur J Sport Sci. (2016) 16(1):42–9. doi: 10.1080/17461391.2014.984768
137. Modric T, Esco M, Perkovic S, Basic Z, Versic S, Morgans R, et al. Artificial turf increases the physical demand of soccer by heightening match running performance compared with natural grass. J Strength Cond Res. (2023) 37(11):2222–8. doi: 10.1519/JSC.0000000000004539
138. San Martin A. Contextual constraints in terminological definitions. Front Commun. (2022) 7:885283. doi: 10.3389/fcomm.2022.885283
139. Renshaw I, Chow JY, Davids K, Hammond J. A constraints-led perspective to understanding skill acquisition and game play: a basis for integration of motor learning theory and physical education praxis? Phys Educ Sport Pedagogy. (2010) 15(2):117–37. doi: 10.1080/17408980902791586
140. Colomer CME, Pyne DB, Mooney M, McKune A, Serpell BG. Performance analysis in rugby union: a critical systematic review. Sports Med Open. (2020) 6(1):4. doi: 10.1186/s40798-019-0232-x
141. Araújo D, Davids K, Bennett SJ, Button C, Chapman G. Emergence of sport skills under constraints. In: Skill Acquisition in Sport: Research, Theory and Practice. Oxfordshire: Routledge (2004). p. 409.
142. Balague N, Torrents C, Hristovski R, Kelso JA. Sport science integration: an evolutionary synthesis. Eur J Sport Sci. (2017) 17(1):51–62. doi: 10.1080/17461391.2016.1198422
143. Burwitz L, Moore PM, Wilkinson DM. Future directions for performance-related sports science research: an interdisciplinary approach. J Sports Sci. (1994) 12(1):93–109. doi: 10.1080/02640419408732159
144. Teune B, Woods C, Sweeting A, Inness M, Robertson S. The influence of environmental and task constraint interaction on skilled behaviour in Australian football. Eur J Sport Sci. (2022) 22(8):1268–75. doi: 10.1080/17461391.2021.1958011
145. Hristovski R, Araújo D, Balagué Serre N, Button C, Passos P. Complex Systems in Sport. London: Routledge (2014).
146. Newell KM. Constraints on the development of coordination. In: Wade MG, Whiting HTA, editors. Motor Development in Children: Aspects of Coordination and Control. The Netherlands, Dordrecht: Martinus Nijhoff (1986). p. 341–60. doi: 10.1007/978-94-009-4460-2_19
147. Glazier PS. Game, set and match? Substantive issues and future directions in performance analysis. Sports Med. (2010) 40:625–34. doi: 10.2165/11534970-000000000-00000
148. Vilar L, Araújo D, Davids K, Button C. The role of ecological dynamics in analysing performance in team sports. Sports Med. (2012) 42:1–10. doi: 10.2165/11596520-000000000-00000
149. Travassos B, Davids K, Araújo D, Esteves TP. Performance analysis in team sports: advances from an ecological dynamics approach. Int J Perform Anal Sport. (2013) 13(1):83–95. doi: 10.1080/24748668.2013.11868633
150. Johnston RJ, Watsford ML, Austin D, Pine MJ, Spurrs RW. Player acceleration and deceleration profiles in professional Australian football. J Sports Med Phys Fitness. (2015) 55(9):931–9. Available online at: https://www.ncbi.nlm.nih.gov/pubmed/2647063626470636
151. Bittencourt NFN, Meeuwisse WH, Mendonca LD, Nettel-Aguirre A, Ocarino JM, Fonseca ST. Complex systems approach for sports injuries: moving from risk factor identification to injury pattern recognition-narrative review and new concept. Br J Sports Med. (2016) 50(21):1309–14. doi: 10.1136/bjsports-2015-095850
152. Boehnert J. The visual representation of complexity: Sixteen key characteristics of complex systems (2018).
153. Paul D. Factors affecting match running performance of elite soccer players: shedding some light on the complexity. Int J Sports Physiol Perform. (2015) 10(4):516–9. doi: 10.1123/ijspp.2015-0029
154. Weaving D, Jones B, Marshall P, Till K, Abt G. Multiple measures are needed to quantify training loads in professional rugby league. Int J Sports Med. (2017) 38(10):735–40. doi: 10.1055/s-0043-114007
155. Weaving D, Jones B, Till K, Abt G, Beggs C. The case for adopting a multivariate approach to optimize training load quantification in team sports. Front Physiol. (2017) 8:1024. doi: 10.3389/fphys.2017.01024
156. Weaving D, Jones B, Ireton M, Whitehead S, Till K, Beggs CB. Overcoming the problem of multicollinearity in sports performance data: a novel application of partial least squares correlation analysis. PLoS One. (2019) 14(2):e0211776. doi: 10.1371/journal.pone.0211776
157. Javed A, Malik KM, Irtaza A, Malik H. A decision tree framework for shot classification of field sports videos. J Supercomput. (2020) 76:7242–67. doi: 10.1007/s11227-020-03155-8
158. Santamaría-Bonfil G, Gershenson C, Fernández N. A package for measuring emergence, self-organization, and complexity based on shannon entropy. Front Robot AI. (2017) 4:10. doi: 10.3389/frobt.2017.00010
159. Wright WA, Smith RE, Danek M, Greenway P. A generalisable measure of self-organisation and emergence. Paper Presented at the International Conference on Artificial Neural Networks (2001).
160. Moschoyiannis S, Elia N, Penn AS, Lloyd DJ, Knight C. A web-based tool for identifying strategic intervention points in complex systems. arXiv preprint arXiv:1608.00655. (2016).
161. Chib S. Markov chain Monte Carlo methods: computation and inference. Handb Econom. (2001) 5:3569–649. doi: 10.1016/S1573-4412(01)05010-3
162. Perl J. Artificial neural networks in sports: new concepts and approaches. Int J Perform Anal Sport. (2001) 1(1):106–21. doi: 10.1080/24748668.2001.11868253
163. Silva P, Duarte R, Esteves P, Travassos B, Vilar L. Application of entropy measures to analysis of performance in team sports. Int J Perform Anal Sport. (2016) 16(2):753–68. doi: 10.1080/24748668.2016.11868921
164. Toussaint M, von Seelen W. Complex adaptation and system structure. BioSystems. (2007) 90(3):769–82. doi: 10.1016/j.biosystems.2007.03.004
165. Djelic M-L, Quack S. Overcoming path dependency: path generation in open systems. Theory Soc. (2007) 36:161–86. doi: 10.1007/s11186-007-9026-0
166. Zhu Y, Imamura M, Nikovski D, Keogh E. Introducing time series chains: a new primitive for time series data mining. Knowl Inf Syst. (2019) 60:1135–61. doi: 10.1007/s10115-018-1224-8
167. Chen S, Guo W. Auto-Encoders in deep learning—a review with new perspectives. Mathematics. (2023) 11(8):1777.
168. Duan B, Zhao Y, Liu H. Maximum entropy principle and topological optimization of truss structures. In: Cheng FY, editor. Computational Mechanics in Structural Engineering. Vol. 1999. 6000 Broken Sound Parkway, NW Suite 300, Boca Raton, Florida 33487, US: Elsevier (1999). p. 179–92
169. Jamro M., Rzonca D. (2014). Measuring, monitoring, and analysis of communication transactions performance in distributed control system. Paper Presented at the Computer Networks: 21st International Conference, CN 2014, Brunów, Poland, June 23–27, 2014. Proceedings 21.
170. Sheehan RB, Herring MP, Campbell MJ. Associations between motivation and mental health in sport: a test of the hierarchical model of intrinsic and extrinsic motivation. Front Psychol. (2018) 9:707. doi: 10.3389/fpsyg.2018.00707
171. Gou M. Modeling for athletic sports strategies based on fuzzy system. Paper Presented at the Fuzzy Information and Engineering Volume 2 (2009).
172. Myszka S, Yearby T, Davids K. (Re) conceptualizing movement behavior in sport as a problem-solving activity. Front Sports Act Living. (2023) 5:1130131. doi: 10.3389/fspor.2023.1130131
173. Pinder RA, Davids K, Renshaw I, Araújo D. Representative learning design and functionality of research and practice in sport. J Sport Exerc Psychol. (2011) 33(1):146–55. doi: 10.1123/jsep.33.1.146
174. Nuri L, Shadmehr A, Ghotbi N, Attarbashi Moghadam B. Reaction time and anticipatory skill of athletes in open and closed skill-dominated sport. Eur J Sport Sci. (2013) 13(5):431–6. doi: 10.1080/17461391.2012.738712
175. Araujo D, Davids K, Chow J, Passos P. The development of decision making skill in sport: an ecological dynamics perspective. Perspect Cogn Act Sport. (2009) 7(8):157–69. doi: 10.1016/j.psychsport.2006.07.002
176. Najafabadi MM, Villanustre F, Khoshgoftaar TM, Seliya N, Wald R, Muharemagic E. Deep learning applications and challenges in big data analytics. J Big Data. (2015) 2(1):1–21. doi: 10.1186/s40537-014-0007-7
177. Sweeting AJ, Aughey RJ, Cormack SJ, Morgan S. Discovering frequently recurring movement sequences in team-sport athlete spatiotemporal data. J Sports Sci. (2017) 35(24):2439–45. doi: 10.1080/02640414.2016.1273536
Keywords: spatiotemporal analysis, physical movement, complex systems, analytics, open questions, working framework
Citation: Parker S, Duthie G and Robertson S (2024) A framework for player movement analysis in team sports. Front. Sports Act. Living 6:1375513. doi: 10.3389/fspor.2024.1375513
Received: 23 January 2024; Accepted: 25 June 2024;
Published: 5 August 2024.
Edited by:
Valentina Camomilla, Università degli Studi di Roma Foro Italico, ItalyReviewed by:
Brice Guignard, Université Claude Bernard Lyon 1, France© 2024 Parker, Duthie and Robertson. This is an open-access article distributed under the terms of the Creative Commons Attribution License (CC BY). The use, distribution or reproduction in other forums is permitted, provided the original author(s) and the copyright owner(s) are credited and that the original publication in this journal is cited, in accordance with accepted academic practice. No use, distribution or reproduction is permitted which does not comply with these terms.
*Correspondence: Stan Parker, c3RhbmxleS5wYXJrZXIxQGxpdmUudnUuZWR1LmF1
†ORCID:
Stan Parker
orcid.org/0000-0002-2085-3495
Grant Duthie
orcid.org/0000-0001-5920-0363
Sam Robertson
orcid.org/0000-0002-8330-0011
Disclaimer: All claims expressed in this article are solely those of the authors and do not necessarily represent those of their affiliated organizations, or those of the publisher, the editors and the reviewers. Any product that may be evaluated in this article or claim that may be made by its manufacturer is not guaranteed or endorsed by the publisher.
Research integrity at Frontiers
Learn more about the work of our research integrity team to safeguard the quality of each article we publish.