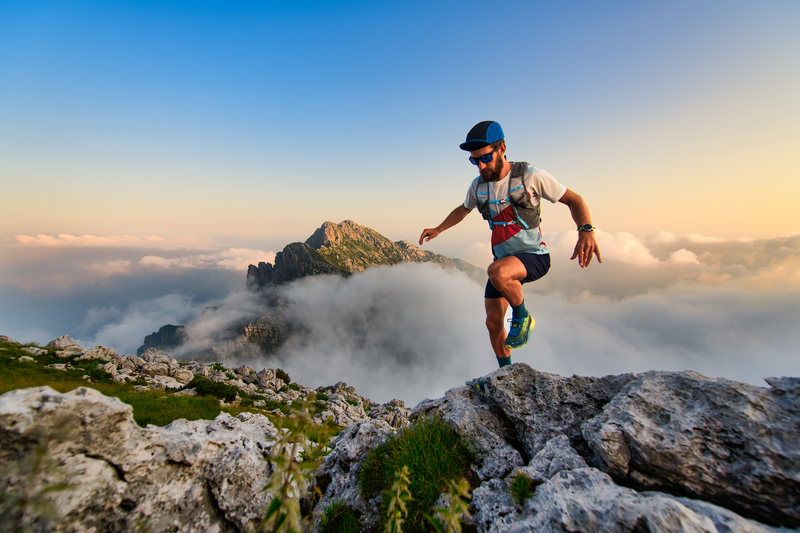
95% of researchers rate our articles as excellent or good
Learn more about the work of our research integrity team to safeguard the quality of each article we publish.
Find out more
BRIEF RESEARCH REPORT article
Front. Sports Act. Living , 04 March 2024
Sec. Sport Psychology
Volume 6 - 2024 | https://doi.org/10.3389/fspor.2024.1338883
This article is part of the Research Topic Remote assessment, measurement, and delivery in sport, physical activity and health View all 5 articles
The objective of the present study was to confirm the convergent validity of information integration theory in the judgment of fatigue in sport, using information integration, subjective, and physiological data. Twenty healthy athletes were confronted with six cycling scenarios in two experimental conditions. In the laboratory condition, the athletes imagined the scenarios and had to cognitively combine the exercise intensity (30%, 50%, and 70% of the maximal intensity) and the exercise duration (15 and 30 min) when judging their expected level of fatigue. In the real sports condition, the athletes enacted each scenario and then rated their subjective fatigue. The heart rate was recorded continuously, so that the physiological training impulse could be calculated. We applied analyses of variance to the data and analyzed correlations between variables. The information integration data from the laboratory condition, the subjective data from the real sports condition, and the objective (physiological) data from the real sports condition were strongly correlated. The information integration patterns concerning fatigue as a function of the exercise duration and intensity obtained respectively from the three data sets were extremely similar.
Over the last few decades, sports trainers have started to consider the athlete as a whole. A variety of physiological and psychological indicators are now used to monitor an individual's level of fatigue and thus optimize his/her physical performance (1). Fatigue occurs when the energy produced by the body is no longer sufficient to respond to an external stimulus, such as physical activity (2). Fatigue leads to a decrease in physical performance (3), and extreme fatigue may lead to injury (4). Hence, fatigue monitoring is a central issue for sports coaches (5).
Training imposes stress on athletes and shifts their physical and psychological well-being along a continuum from acute fatigue to overreaching (6). While overreaching may be incorporated with care into a periodized training plan, progression towards overtraining syndrome is undesirable. Appropriate titration of fatigue is important for both adaptation to training and performance in competition (7). Athletes should be monitored closely to ensure that training elicits the desired effects on their well-being and performance. In order to estimate the level of fatigue induced by training, coaches can rely on objective (physiological) data and/or subjective (psychological) data (8). Coaches can calculate the training load by using various methods based on physiological data (9). For example, the training impulse (TRIMP) is often considered to be a useful means of objectively assessing the training load and the level of fatigue (7). A TRIMP is a unit of physical effort that is calculated from the training duration, the resting heart rate (HR), and the maximal, resting, and mean HRs during the exercise session (10). It presents a multiplicative relationship between the training duration and the HR's variables.
Fatigue can also be considered subjectively (11). Self-reported indicators on judgment scales (such as the Borg rating of perceived exertion scale) are particularly used in endurance sports (e.g., cycling) and appear to be relevant for estimating fatigue during exercise of different intensities (12). However, none of these subjective indicators of fatigue allow us to understand the cognitive process involved in the generation of fatigue. It is therefore essential to develop new approaches that account for the cognitive processes involved in subjective fatigue. We hypothesized that information integration theory [IIT; (13)] can be used to address this shortcoming.
According to IIT, a perception, thought or action depends on the integration of several different types of information. IIT describes the way in which individuals cognitively integrate information to arrive at an overall judgement. Anderson (14) developed the concept of cognitive algebra: the way in which information is combined by an individual will produce additive, multiplicative, and averaging cognitive rules (14). An additive rule is given by a pattern of parallelism. A multiplicative rule, such as a conjunctive rule or a disjunctive rule, is given by a linear fan pattern. When the pattern show a fan open to the left, the cognitive rule is disjunctive, and when the pattern shows a fan open to the left, the cognitive rule is conjunctive. An averaging rule is also given by a parallelism pattern but the concepts of importance weight and prior information are considered (13). In sport, the researchers principally found additive rules and multiplicative rules [for an illustration in sport, see Fruchart (15)].
Graphical methods (based on the response pattern, i.e., the shape of the curves) and statistical methods (mainly repeated-measures analyses of variance) can be used in an IIT analysis. Although IIT has been applied in various domains in sports psychology (e.g., judgments of well-being (15), performance (16), and ethics (17)), its validity in the sports domain is still subject to debate because the experiments do not take place in real-life sporting situations [e.g. (18),]. Indeed, the IIT method is typically based on questionnaires designed by the experimenter and administered in the laboratory. Participants are presented with scenarios based on real sports situations and then have to respond to a question by grading their opinion on a judgment or decision scale.
To the best of our knowledge, only Fruchart et al. (19) have examined the convergent validity of the application of IIT to decision-making patterns for a quick throw-in in handball. The researchers compared the patterns obtained under laboratory conditions (obtained from an IIT-based questionnaire) with the patterns observed in a true sports condition (obtained from a video recording); the two sets of patterns were very similar. This finding demonstrated that the judgment scheme derived in the laboratory study and that inferred from real observations in the sports field were reasonably compatible. However, there are few other studies of this type in the literature. Work on other types of decision or judgment is required to consolidate the validity of an IIT-scenario-based method (13).
IIT scenarios are typically created from the combination of at least two factors that might influence an individuals’ judgment of an indicator (e.g., fatigue). The exercise modalities (e.g., the duration and intensity) are known to determine the onset of fatigue (20, 21). However, the way in which exercise duration and exercise intensity are integrated cognitively during the judgment of fatigue had not previously been investigated.
The primary objective of the present study was to confirm the convergent validity of the IIT method in the judgment of fatigue in sport. To achieve this, we measured the putative associations between information integration data from the laboratory condition, subjective data from the real sports condition, and objective (physiological) data on fatigue from the real sports condition. We also compared the response patterns for cognitive data observed in the laboratory condition with those observed for subjective data in the real sports condition.
Our first hypothesis was that the three types of data (information integration data, subjective data, and physiological data) would be correlated with each other. Our second hypothesis was that exercise duration and exercise intensity would both have a significant effect on the judgment of fatigue. Based on TRIMP, our third hypothesis was individuals would be used a multiplicative integration rule. Lastly, our fourth hypothesis was that the patterns of fatigue as a function of exercise duration and intensity would be similar in the laboratory condition (with information integration data) and the real sports condition (with subjective data).
Twenty healthy, experienced cyclists (16 men and 4 women) agreed to take part in the study. The mean number of years of experience (Myears) was 6.3 (SD = 4.3). The participants’ age ranged from 19 to 33 (Mage = 23; SD = 2.1). The participants did not receive any remuneration for their involvement in the study.
In the laboratory condition, the participants filled out a questionnaire with six scenarios. Each scenario comprised a cycling situation, a question, and a response scale. The six situations were generated by combining the duration factor (two levels: 15 or 30 min) and the intensity factor (three levels: 30%, 50% or 70% of the maximal intensity). For each situation, the participants were asked “How tired will you be at the end of this session?”. Each participant then estimated the degree of fatigue at the end of the session on an 11-point rating scale ranging from “Not at all tired” to “Extremely tired” (see Appendix for an example).
In the real sports condition, we transposed these six scenarios into real sports conditions in a sports hall with appropriate equipment (a Wahoo Kickr home trainer). A tablet computer was used for recording the participant's fatigue rating. The participant was equipped with an HR monitor (H10, Polar) and the HR was recorded throughout the exercise session. Each situation's exercise load was calculated by applying Banister's formula (10): TRIMP = T × ΔHR × k, where T is the exercise duration (in minutes), ΔHR is (HRexercise—HRrest)/(HRmax-HRrest), and k is 0.86 (for a woman) or 0.64 (for a man).
The study protocol was approved by the independent ethics committee at the University of Toulouse (Toulouse, France; reference: 2022-586). The study included a laboratory condition (which took place in a quiet laboratory room) and a real sports condition (in a sports hall). Each participant was first confronted with the laboratory condition and then with the real sports condition. An initial appointment was arranged with the participant so that he/she could be given information about the study's objectives and procedures and could then give his/her written, informed consent to participation in the study. Each participant kept a copy of the consent form, and another copy was archived by the investigators.
In the laboratory condition, the participant had to read the description of the various cycling scenarios and imagine him/herself performing them. For each scenario, the participant had to judge his/her expected level of fatigue. In line with Anderson's methodology (13), a familiarization phase was followed by an experimental phase. The laboratory session lasted for approximately 10 min and thus provided information on integration data.
At the end of the laboratory session, the investigator made an appointment with the participant so that he/she could take part in the real sports condition. According to the 2 × 3 factorial design described above, each participant performed each of the six real cycling scenarios on separate days and had a three-day interval between sessions. For each real sports situation, the participant performed the physical activity and then indicated his/her level of fatigue on the same scale used in the laboratory condition. The HR was recorded throughout the exercise session. We varied the exercise intensity and duration, using the values from the six laboratory scenarios. The intensity levels were based on the maximal aerobic power (22). The participants were not informed of the exercise duration and intensity values that were to be applied to the session. After the participant had completed the six real sports scenarios, the investigator explained the previously hidden aspects of the protocol. The real sports condition provides us with subjective data and objective (physiological) data.
The participant’s fatigue rating data from the laboratory condition and the real sports condition were converted into numerical data by calculating the distance between the point on the response scale and the left anchor (the origin).
The numerical data were analyzed statistically and graphically. For each of the three types of data, parametric tests were conducted after the normality of distribution had been checked using the Shapiro-Wilk test. Four repeated-measures analyses of variance (ANOVAs) were conducted according to the duration×intensity factorial design: the first on information integration data from the laboratory condition, the second on subjective data from the real sports condition, the third on the mean HR data, and the fourth on the TRIMP data. Tukey's post hoc tests were performed on the intensity factor. We calculated Pearson coefficient for the correlations between the variables. All statistical analyses were performed with Statistica software (version 8).
In a graphical analysis of the data, the curves rise from left to right (indicating an effect of intensity) and are separate (indicating an effect of duration) (Figure 1). In the laboratory condition (the bottom panel in Figure 1) and in the real sports condition (the middle panel in Figure 1), the curves form a fan opening to the right [indicating that the participants used the same integration conjunctive (multiplicative) rule in both conditions]. The duration×intensity interaction was statistically significant (p < .05) in the laboratory condition [F(2, 38) = 3.67, p = .035, η2p = .16] and in the real sports condition [F(2, 38) = 7.71, p = .002, η2p = .29] (Tables 1, 2). In both conditions, Tukey's post hoc test revealed significant differences (p < .001) between the three levels of intensity.
Figure 1. Effect of duration and intensity on the fatigue rating in a laboratory condition (top panel) and in a real sports condition (middle panel) and on the TRIMP in a real sports condition (bottom panel). Legend. In the three panels, the three levels of the intensity factor are on the x-axis, and each line corresponds to one level of the duration factor. In the top and middle panels, the fatigue rating is on the y-axis. In the bottom panel, the TRIMP is on the y-axis.
Table 1. Mean and standard deviation values in each scenario for information integration data from the laboratory condition, subjective data, and physiological data (HR mean and TRIMP) from the real motor sports condition.
Table 2. Main results of the ANOVAs of information integration data from the laboratory condition and subjective data and objective (TRIMP) data from the real sports condition.
The ANOVA on mean HR data showed that (i) the duration factor was not significant, F(1, 19) = 1.33, p = .30, η2p = .05; (ii) the intensity factor was significant, F(2, 38) = 3.67, p < .001, η2p = .96; and (iii) the duration×intensity interaction was significant, F(2, 38) = 3.73, p = .03, η2p = .16 (Table 1). Tukey's post hoc test revealed significant differences between the three levels of the intensity factor (p < .001).
With regard to the TRIMP data, the curves rise from left to right (indicates an effect of intensity), are separate (indicating an effect of duration) and form a fan opening to the right indicating an integration conjunctive (multiplicative) rule (Figure 1, bottom panel). The duration×intensity interaction was statistically significant (p < .05): F(2, 38) = 54.50, p < .001, η2p = .74 (Tables 1, 2). Tukey's post hoc test revealed significant differences between the three levels of the intensity factor (p < .001).
The information integration data from the laboratory condition was correlated with subjective data from the real sports condition and with the objective data (mean HR and TRIMP) from the real sports condition (Table 3). The subjective data from the real sports condition was correlated with the set of objective data (mean HR and TRIMP) from the real sports condition. The mean HR and TRIMP data were correlated with each other.
Table 3. Correlations between the information integration data from laboratory condition, the subjective data from the real sports condition, and the objective (mean HR and TRIMP) data from the real sports condition.
The primary objective of the present study was to confirm the convergent validity of the IIT method in the judgment of fatigue in sport, using information integration, subjective and physiological data. To this end, we tested the putative association between information integration data from the laboratory condition, subjective data from the real sports condition, and objective (physiological) data from the real sports condition, as a function of the participants’ level of fatigue in different sports situations. We also compared response patterns for the information integration data observed in the laboratory condition and the subjective data observed in the real sports condition.
Our first hypothesis was that the three data sets would be correlated with each other; this was confirmed by the study's results. The physiological data (mean HR and TRIMP) from the real sports condition were correlated with the information integration data from the laboratory condition and the subjective data in the real sports condition. This finding confirms the existence of close relationships between physiological and psychological indicators of fatigue (2). The information integration data from the laboratory condition were also associated with subjective data in the real sports condition. This result supports the correlation found between data observed in a judgment condition (i.e., an information integration condition) and in a real condition (19).
The second hypothesis was that the exercise duration and intensity would have a significant effect on fatigue judgment. In the laboratory condition and in the real sports condition, both exercise duration and intensity were associated with a higher fatigue rating and a higher TRIMP. These findings were in line with literature data on the exercise duration and intensity as indicators of the physiological level of athletes’ fatigue (21). The two factors are also essential in the judgment of fatigue.
Our third hypothesis was that individuals would be used a multiplicative cognitive rule. This was confirmed by the form of patterns. The use of the IIT method and the observed significant intensity-by-duration interactions for all three fatigue measures strongly supports a multiplicative integration rule. Moreover, while the factorial curves in laboratory condition and the factorial curves for subjective estimates of fatigue in real condition are virtually identical, the observed linear-fan pattern (indicating a multiplicative integration rule) is far more pronounced for physiological fatigue. This suggests (i) that participants’ subjective estimates of fatigue mirrored their subsequent (and prior) physical fatigue (as indicated by the significant intercorrelations between these measures), (ii) that exercise intensity and duration are integrated multiplicatively -and physiologically- to determine fatigue (i.e., the relative impact of intensity on fatigue increases with greater duration), and (iii) that this multiplicative integration is particularly pronounced for physical fatigue.
Our fourth hypothesis was that the response pattern of fatigue (as a function of duration and intensity) would be similar in the laboratory condition and the real sports condition (19). Our results confirmed this hypothesis. When judging their level of fatigue, the athletes combined both factors in the same way via a questionnaire presenting a real situation and when performed that activity. This result confirms the validity of IIT found by Fruchart et al. (19) in decision-making for a quick throw-in in handball.
However, our study went further than that reported by Fruchart et al. (19) in the comparison between the response patterns in the laboratory and a real sports condition. Furthermore, we looked at objective (physiological) measures of fatigue. The results based on physiological (TRIMP) data were very similar to those based on information integration data. Exercise intensity and duration both had a significant effect on fatigue. The curves showing the interaction between intensity and duration had the same conjunctive (multiplicative) integration pattern as seen for the information integration data. Further research is required to determine whether physiological integration rules exists in the same way that information integration rules do.
Our study had some limitations, which might open up opportunities for further research. Firstly, it would have been interesting to record other physiological data (e.g., the blood serotonin level) and compare them with the information integration data on fatigue (2). Secondly, we looked at a single type of judgment (i.e., judgment of fatigue); in order to further confirm the validity of IIT in sports, judgment in other situations should be investigated. For instance, one could evaluate whether athletes’ judgments of performance are similar in the laboratory and in a real sports situation. Thirdly, we did not assess the degree of participants’ exercise during each 3-day rest period; such activity could moderate the observed results. Fourthly, we did not study potential moderating effects of athletic fitness and/or skill on this difference between subjective estimates and physiological integration of exercise intensity and duration (for example, would they posit that greater fitness/skill would be expected to reduce this difference, as athletes gain experience with physiological responses to exertion?).
Our results show that (i) when one is careful to use the same factors and rating scales in the laboratory and in the real sports condition and (ii) when the physiological and the rating responses express the same underlying dependent variable (“fatigue”) and the factors in the imaginary setting successfully operationalize the intended determinants of fatigue manipulated in the real setting, it is then possible to directly assess the agreement between the information integration data from the laboratory condition and the physiological and subjective data from the real sports condition.
In terms of IIT, finding overall similar response patterns with ratings and the physiological response is more fundamental than finding a significant correlation between ratings and TRIMP or mean HR. Under the assumption that the ratings provide a linear scale of “fatigue” (meaning an equal-interval scale for the expression of fatigue), finding a similar pattern with TRIMP would signal it as a proper “psychophysiological” (rather than just physiological) index of fatigue, unlike HR. This allows us to contrast the IIT approach to sports fatigue with other rating methods, such as the Borg rating of perceived exertion where there is no “structural criterion” to establish proper “psychophysiological” measure. Of course, the small number of levels used in the design (e.g., only two levels in one of the factors and three in the other) limits the assessment of response linearity in accordance with IIT methodology, which must be merely assumed, rather than established.
Our results may have implications for sports training. Athletes have to monitor a large number of variables before, during and after training (1). The physiological data collected enables coaches to quantify the training load. Although these indicators are highly relevant, they focus solely on the athlete's somatic responses to training. The emergence of judgment indicators would help to (i) take greater account of the cognitive dimension of adaptations to training, (ii) determine the weight given to various factors included in sports situations, and (iii) monitor athletes more closely and provide them with more precise, individualized training programmes. Indeed, IIT studies in the field of sports psychology have shown that an athlete's judgment position (i.e., the manner in which an athlete cognitively combine various pieces of information when judging a situation) depends on his/her characteristics [e.g., (17,23)]. Our results also suggest that while athletes may generally be able to estimate their physical fatigue, they appear to underestimate this fatigue under high levels of both exercise intensity and duration. Coaches should be vigilant about how athletes estimate their level of fatigue. Combining a cognitive-indicator-based IIT method recorded in the laboratory, and physiological and subjective indicators recorded during sports activity might constitute a novel way of monitoring training.
The raw data supporting the conclusions of this article will be made available by the authors, without undue reservation.
The studies involving humans were approved by Independent ethics committee at the University of Toulouse (Toulouse, France; reference: 2022-586). The studies were conducted in accordance with the local legislation and institutional requirements. The participants provided their written informed consent to participate in this study.
AL: Writing – original draft. AG: Writing – original draft. EF: Writing – original draft.
The author(s) declare that no financial support was received for the research, authorship, and/or publication of this article.
The authors thank National Altitude Training Centre Font-Romeu for contribution.
The authors declare that the research was conducted in the absence of any commercial or financial relationships that could be construed as a potential conflict of interest.
All claims expressed in this article are solely those of the authors and do not necessarily represent those of their affiliated organizations, or those of the publisher, the editors and the reviewers. Any product that may be evaluated in this article, or claim that may be made by its manufacturer, is not guaranteed or endorsed by the publisher.
1. Taylor K-L, Chapman DW, Cronin JB, Newton MJ, Gill N. Fatigue monitoring in high performance sport: a survey of current trends. JASC. (2012) 20:12–23.
2. Tornero-Aguilera JF, Jimenez-Morcillo J, Rubio-Zarapuz A, Clemente-Suárez VJ. Central and peripheral fatigue in physical exercise explained: a narrative review. Int J Environ Res Public Health. (2022) 19:3909. doi: 10.3390/ijerph19073909
3. McKenna MJ, Hargreaves M. Resolving fatigue mechanisms determining exercise performance: integrative physiology at its finest!. J Appli Physiol. (2008) 104:286–7. doi: 10.1152/japplphysiol.01139.2007
4. Wang C. Sports-induced fatigue recovery of competitive aerobics athletes based on health monitoring. Comput Intell Neurosci. (2022) 2022:1–10. doi: 10.1155/2022/9542397
5. Gabbett TJ. Debunking the myths about training load, injury and performance: empirical evidence, hot topics and recommendations for practitioners. Br J Sports Med. (2020) 54:58–66. doi: 10.1136/bjsports-2018-099784
6. Fry RW, Morton AR, Keast D. Overtraining in athletes. An update. Sports Med. (1991) 12:32–65. doi: 10.2165/00007256-199112010-00004
7. Pyne DB, Martin DT. Fatigue - insights from individual and team sports. In: Marino FE, editors. Regulation of Fatigue in Exercise. New York: Nova Publishers (2011). p. 177–86.
8. Lourenço J, Gouveia ÉR, Sarmento H, Ihle A, Ribeiro T, Henriques R, et al. Relationship between objective and subjective fatigue monitoring tests in professional soccer. Int J Environ Res Public Health. (2023) 20:1539. doi: 10.3390/ijerph20021539
9. Halson SL. Monitoring training load to understand fatigue in athletes. Sports Med. (2014) 44:139–47. doi: 10.1007/s40279-014-0253-z
10. Banister EW. Modeling elite athletic performance. In: Mac Dougall JD, Wenger HA, Green HJ, editors. Physiological Testing of Elite Athletes. Champaign, Illinois: Human Kinetics (1991).
11. Ten Haaf T, Van Staveren S, Oudenhoven E, Piacentini MF, Meeusen R, Roelands B, et al. Prediction of functional overreaching from subjective fatigue and readiness to train after only 3 days of cycling. Int J Sports Physiol Perform. (2017) 12:S287–94. doi: 10.1123/ijspp.2016-0404
12. Bok D, Rakovac M, Foster C. An examination and critique of subjective methods to determine exercise intensity: the talk test, feeling scale, and rating of perceived exertion. Sports Med. (2022) 52:2085–109. doi: 10.1007/s40279-022-01690-3
15. Fruchart E. Judgments of happiness during trail running: pleasure, engagement, and meaning. Psychol Sport Exerc. (2021) 55:101938. doi: 10.1016/j.psychsport.2021.101938
16. Fruchart E, Rulence-Pâques P. Predicting sports performance from well-being: a mapping of professional athletes’, amateur athletes’ and non-athletes’ positions. Eur Rev Appl Psychol. (2022) 72:100793. doi: 10.1016/j.erap.2022.100793
17. Fruchart E, Rulence-Paques P. Mapping positions on forgiving an aggressor in sport. Front Psychol. (2021) 12:561031. doi: 10.3389/fpsyg.2021.561031
18. Bordas A, Fruchart E. Pacing strategy in trail running: a cognitive subtractive model of the affective balance between effort and pleasure? Psychol Sport Exerc. (2023) 67:102409. doi: 10.1016/j.psychsport.2023.102409
19. Fruchart E, Rulence-Pâques P, Mullet E. Ecological validity test of laboratory studies of information integration. Teorie and Modelli. (2007) 12:281–8.
20. Amann M. Central and peripheral fatigue: interaction during cycling exercise in humans. Med Sci Sports Exerc. (2011) 43:2039–45. doi: 10.1249/MSS.0b013e31821f59ab
21. Azevedo RA, Cruz R, Couto P, Silva-Cavalcante MD, Boaric D, Okuno D, et al. Effects of prior high-intensity endurance exercise in subsequent 4-km cycling time trial performance and fatigue development. Sciences & Sport. (2022) 37:70e1-11. doi: 10.1016/j.scispo.2020.12.008
22. Walsh RM, Noakes TD, Hawley JA, Dennis SC. Impaired high-intensity cycling performance time at low levels of dehydration. Int J Sports Med. (1994) 15(7):392–8. doi: 10.1055/s-2007-1021076
23. Fruchart E, Rulence-Pâques P. Mapping judgment of well-being in sport. Int J Sport Exerc Psychol. (2021) 19(4):488–503. doi: 10.1080/1612197X.2020.1717577
Keywords: validity, information integration, fatigue, training impulse, interdisciplinary
Citation: Legall A, Gaston A-F and Fruchart E (2024) Validity of information integration based on subjective and physiological data from a real sports condition: application to the judgment of fatigue in sport. Front. Sports Act. Living 6:1338883. doi: 10.3389/fspor.2024.1338883
Received: 15 November 2023; Accepted: 22 February 2024;
Published: 4 March 2024.
Edited by:
Michele Vicovaro, University of Padua, ItalyReviewed by:
Philip Moore, George Washington University, United States© 2024 Legall, Gaston and Fruchart. This is an open-access article distributed under the terms of the Creative Commons Attribution License (CC BY). The use, distribution or reproduction in other forums is permitted, provided the original author(s) and the copyright owner(s) are credited and that the original publication in this journal is cited, in accordance with accepted academic practice. No use, distribution or reproduction is permitted which does not comply with these terms.
*Correspondence: Eric Fruchart ZXJpYy5mcnVjaGFydEB1bml2LXBlcnAuZnI=
Disclaimer: All claims expressed in this article are solely those of the authors and do not necessarily represent those of their affiliated organizations, or those of the publisher, the editors and the reviewers. Any product that may be evaluated in this article or claim that may be made by its manufacturer is not guaranteed or endorsed by the publisher.
Research integrity at Frontiers
Learn more about the work of our research integrity team to safeguard the quality of each article we publish.