- 1CIFD, Sports Research, and Training Center, Jean Piaget University of Angola, Luanda, Angola
- 2Department of Sport Sciences, CIDESD, Research Center in Sports Sciences, Health Sciences, and Human Development, University of Beira Interior, Covilhã, Portugal
- 3KinesioLab – Research Unit in Human Movement, Institute of Piaget, Almada, Portugal
- 4Department of Sport and Well Being, Polytechnic Institute of Castelo Branco, Castelo Branco, Portugal
- 5Sport, Health and Exercise Research Unit—SHERU, Polytechnic Institute of Castelo Branco, Castelo Branco, Portugal
- 6Research Unit for Sport and Physical Activity, Faculty of Sport Sciences and Physical Education, University of Coimbra, Coimbra, Portugal
- 7CIDEFES, Centre for Research in Sport, Physical Education, Exercise and Health, Lusófona University, Lisbon, Portugal
- 8COD, Center of Sports Optimization, Sporting Clube de Portugal, Lisbon, Portugal
- 9Portugal Football Scholl, Portugal Football Federation, Lisbon, Portugal
Background: Tracking devices, such as global (GPS) and local (LPS) positioning systems, combined with physiological measurements, have become reliable tools to characterize movement patterns, assessing the external load (EL), internal load (IL), fatigue, and performance of athletes in team sports. This scoping review aims to provide a comprehensive understanding of the applicability of tracking systems in physical performance analysis within team sports and the wellbeing of athletes based on research strategies and combined variables.
Methods: A systematic search was conducted in PubMed, Web of Knowledge, and Scopus databases according to PRISMA guidelines. The 79 studies that were reviewed met the following criteria: (1) contained relevant data regarding elite athletes′ performance; (2) athletes' EL and IL; (3) were written in the English language; (4) were related only to team sports.
Results: The findings indicate that tracking technology has been engaged in several research areas, including performance analysis, training vs. match load management, injuries, and nutrition, through characterization and correlational studies. Metrics, primarily focused on kinematic and mechanical EL aspects, have been employed in combination with IL data to analyze the performance of athletes. However, the lack of an integrative model for the analysis and integration of EL and IL metrics within each team sport suggests an interesting direction for further research.
Conclusion: There is a need for coherence between the methods and the research goals on performance analysis. The development of a framework that guides experimental studies is highly recommended, particularly on manipulating metrics analyzed between training and match sessions, injury prevention, and nutrition. This will lead to the development of the most applied sports science research to improve the preparation and decision-making of athletes based on reliable data.
Systematic Review Registration: https://inplasy.com/?s=2022120039, identifier 2022120039.
1. Introduction
It is commonly recognized that team sports necessitate the development of various intense activities based on the space and time relationship between teammates and opponents, implying that movement production requires a perception–action relationship, in which short- and long-duration efforts are needed to achieve the most functional individual tactical actions (1). To achieve this, players must persistently adapt their field placement and enhance their preparedness to undertake actions with or without the ball by producing physical patterns such as accelerations, decelerations, changes in direction, short-term movements, and high impacts (1). Therefore, a precise and appropriate assessment of both internal load (IL) and external load (EL) considering the purpose of analysis is required to improve the understanding of the physical efforts and performance of players (2).
Regardless of some reservations about the quality, reliability, and validity of global positioning system (GPS) and local positioning system (LPS) measures (3), tracking athletes' time motion, combined with physiological measures such as heart rate, has become a common method for characterizing athletes' EL and IL (4, 5). An analysis of EL refers to quantifying players' movement demands regarding volume and intensity (6). According to the literature (7), EL can be divided into different categories such as (1) kinematics, which calculates overall movement during exercise; (2) mechanical, which describes the player's overall load during exercise; and (3) metabolic, which measures overall movement energy expenditure during exercise. In addition to the information provided by EL, athletes may experience varied physiological (IL) reactions (8). In this setting, IL is the individual athlete’s response induced by the EL stimulus, which is commonly determined by heart rate (HR) parameters (9). Thus, an investigation of the relationship between EL and IL units might be considered one of the most critical elements to monitor the dynamics of players' fatigue over the microcycle and their preparedness for a competition (10).
A lot of research has been developed in team sports over the last few years in order to characterize game demands and identify the most demanding passages of play, to understand the influence of different training manipulations during training sessions, or even to better understand injury mechanisms (11, 12). Also, some systematic reviews have been published in the last 2 years (4, 13, 14) with specific goals to understand the relationship between IL and EL or to characterize methods to collect and interpret EL (15). According to the literature, combining both information (IL and EL) allows one to perceive how workload promotes physiological impacts in players or comparing match/training sessions (5). As a result, the distinction of EL and IL units may reveal an athlete's level of exhaustion or enable the identification of the training plan. For example, it was already reported that the efficiency index (Effindex), which combines IL and EL, is a powerful tool for the evaluation of fitness status in team sports (14, 16). In addition, it has been emphasized that selecting appropriate metrics is crucial for accurately describing, planning, monitoring, and evaluating the training and competition aspects of each sport (16, 17). This information may aid in the management of training loads, thus lowering the risk of injury (4, 18) and improving athletic performance (4, 19). A systematization of prior research is essential to better understand the variables/forms of analysis that better explain the damage mechanism or performance improvement. However, to the best of our knowledge, no research has elucidated the relationship between various metrics, the intended purpose of the analysis, and its applicability for coaching and scientific purposes. An understanding of how the information from tracking technology devices has been used in previous research and what are the main issues that have been studied are required, to improve further research and practice. Thus, this scoping review aims to understand the applicability of tracking systems for physical performance analysis in team sports in the last decade by allowing an understanding of how research strategies and variables have been used and combined according to research goals. Based on this systematization, a comprehensive framework of physical performance analysis in team sports and athlete wellbeing is proposed.
2. Methods
Preferred Reporting Items for Systematic and Integrative Reviews and Meta-analysis Statements (PRISMA) were used to conduct a scoping review (20).
2.1. Search strategy: database and inclusion criteria
According to the PRISMA statement recommendations, a systematic search was undertaken on the electronic databases of PubMed, Web of Knowledge (all databases), and Scopus (20). A search of relevant articles published between January 2011 and July 2023 was conducted using the keywords [“global position system” OR GPS OR “local position system” OR LPS) AND (“team sports*,” Indoor team sports**”) AND (performance* OR “external load” OR “internal load”)]. The publications that were retrieved had to meet the following requirements: (1) should provide relevant statistics and research strategies about elite athletes' performance; (2) athletes' EL and IL; (3) published in English; and (4) should be only about team sports. Exclusion criteria applied if they (1) specifically pertained to the reliability, validity, or precision of global positioning system equipment; (2) pertained to systematic reviews, (3) were published before 2011.
To improve research accuracy, two reviewers (AF and BT) independently screened titles and abstracts to identify articles that would potentially combine the inclusion criteria after noting the features of each study, such as the authors' names, sample, procedure, and results or main outcomes. In the event of disagreement about the article's eligibility, a third reviewer (HS) was brought in to make a final judgment. Following a review of all papers, the study categories were divided into multiple sports depending on the primary research topic.
2.2. Quality of studies and data extraction
The quality of the studies was assessed in accordance with what is indicated by Faber et al. (21) and using the criteria for critical review forms recommended by Law et al. (22) (16 items) with the goal of finding studies in which low-quality scores could interfere with the results. Both researchers (AF and BT) separately assessed the quality of each eligible article. The interrater agreement was moderate (k = 0.43) (23). The possible criteria for each item were 1 (meets criteria), 0 (does not meet the criteria), or NA (not applicable). According to the methodology assessment, articles were evaluated with regard to their purpose (item 1), relevance of background literature (item 2), appropriateness of the study design (item 3), sample included (items 4 and 5), informed consent procedure (item 6), outcome measures (item 7), validity of measures (item 8), significance of results (item 9), details of intervention (item 10), analysis (item 11), clinical importance (item 12), description of drop-outs (item 13), conclusion (item 14), practical implications (item 15), and limitation (item 16). According to the guidelines of Faber et al. (21), a final score was determined, allowing the publications to be classified as (1) low methodological quality (50%), (2) good methodology quality (50%–75%), or (3) exceptional methodology quality (>75%). A data extraction sheet (based on the Cochrane Consumers and Communication Review Group's data extraction template) (24) was adjusted to meet the research inclusion criteria for this analysis and then evaluated on 10 randomly chosen studies (pilot test). The data were extracted by one author and validated by another. Disagreements were settled by discussions between these two authors (first and last). To organize the findings, the studies were divided into categories based on the major study issues.
3. Results
3.1. Search, selection, and inclusion of studies
The first search generated 772 titles. These data were then exported to Mendeley (Elsevier, San Francisco, CA, USA), a reference manager software. Duplicates (n = 430) were either automatically or manually removed. The remaining 342 titles were then evaluated for relevance using information from their title and abstract, with 164 being eliminated. The remaining 178 papers were examined further, and 99 were excluded based on the following criteria: (1) articles published in languages other than English (n = 5); (2) articles unrelated to team sports (n = 4); (3) articles unrelated to elite athletes (n = 10); (4) systematic reviews (n = 14); (5) articles unrelated to the analysis of athletes' performance by technology (n = 22); and (6) articles concerning the reliability and validation of tracking technology (n = 44). The review comprised 79 studies in total (Figure 1).
To understand the scope of the development of research methodologies in physical performance evaluation in team sports during the last decade, the publication target years were restricted from January 2011 to July 2023. The articles used for in-depth reading revealed that there had been an increase in GPS and LPS research. In this review, 84.8% of the studies were published in the last 5 years (from 2017 to 2023) and 15.2% between 2011 and 2016. On average, 6.3 studies were published per year.
3.2. Quality of the studies
With regard to the quality of the studies, following the proposal of Faber et al. (21), the average score of the 79 selected studies was 89.2%. Fourteen studies achieved 100%; 52 achieved an average rating of ≥75%; 12 achieved a score between 50% and 75%.
3.3. General description of the studies
Studies were categorized by the sport in which the studies were carried out: (1) football (FTB) (41.8%), (2) futsal (FTS) (15.2%), (3) rugby (RG) (11.4%), (4) Australian football (AUF) (7.6%), (5) field hockey (FH) (6.3%), (6) Gaelic football (GF) (6.3%), (7) basketball (BK) (3.8%), (8) American football (AMF) (2.5%), (9) ice hockey (IH) (2.5%), and (10) rink hockey (RH) (2.5%).
3.4. Variables and goals of the studies
The studies were classified based on the criteria used in the study. Most of the team sports research employed tracking technology: (1) combined kinematic and mechanical variables (35.4%); (2) only considered kinematics (21.0%); and (3) included kinematic, mechanical, and metabolic variables (16.5%). On the other hand, the analysis of IL or the relationship between mechanical variables and IL variables was less explored (Figure 2).
Studies were also grouped according to the type of analysis of the studies. The results revealed that the studies were distributed by using the following analyses: (1) characterization and comparison of drills, training, and matches sessions (62.0%), (2) correlation of variables to understand their relationship in training and competition (26.6%), (3) predicting performance, fatigue, and injuries (8.7%), and finally, (4) experimental studies to determine greater training and team management approach (2.7%) (Figure 3).
With regard to the main research topics from the studies in analyses, the following was observed: (1) performance analysis in match demands, (2) performance analysis of training vs. match demands, (3) injuries, and (4) nutrition (Figure 4).
The studies reviewed provide information on the use of tracking systems in various domains of sports sciences, such as the importance of technology in better understanding the physical requirements for coadaptations between match demand information in which players establish interactions with teammates and opponents in order to manipulate training sessions and, as a result, to improve athletes' awareness and physical performance (25, 26). In addition, studies were used to determine whether specialized nutritional intake could improve players' responses to the demands of an indoor team sport (27, 28). Also, in recent years, multiple sports have used various methods to track injuries by understanding the external information that better characterizes sporting activities (7, 29, 30).
With regard to the understanding of training and match demands in the performance and analysis of team sports, several studies have been carried out in order to compare and understand positional (31), tactical, and technical differences (32), intragame information (33, 34), comparisons of the levels of competitions (35), predictors of intensity, fatigue, and match performance (36–39), and more recently, to understand the games’ most high-intensity activities (HIAs) and periods (MIPs) (36, 40) (Figure 4).
3.5. Applicability of the tracking system related to performance analysis in match demands
With regard to the performance analysis of match demands, there are several studies involving the characterization and understanding of EL metrics in various team sports (32, 41–46) as well as its impact on athletes’ IL (36, 47–51). Some studies describe a moderate to high correlation observed between EL and IL (38, 48). In addition, there are studies using tracking systems in which the main goal is to find correlations between metrics; (1) kinematic vs. mechanical (36, 43, 52); (2) kinematic vs. mechanical and metabolic (37, 41).
A study with 118 elite male ruby league players shows significant increases in the offensive and defensive tackles between senior and junior players and between forwards and backs (53). In the same study, independent of the playing level, running demands (HSR, ACC, DEC) were significantly greater in backs than in forwards (53). There is a high prevalence of studies in which EL metrics are classified based on player level and player position; positioning is often related to an event or to the magnitude of EL metrics (33, 46, 53–56). However, positioning associations are not always found in team sports. In a study of nine elite RH players, there were no significant differences throughout all observed metrics during a competition between players' positions (57). Furthermore, variable load distribution (total PL) based on the axis of movement was not verified based on playing position (58). However, total PL appears suitable for monitoring locomotor demands or accelerometer-derived load in FTB (58).
Finally, and more recently, studies seem to aim for the prediction and proposal of models of fatigue (59) and match performance according to match demand information (44, 60–63) (Table 1).
3.6. Applicability of the tracking system related to the performance analysis of training vs. match demands
Based on an analysis of the selected studies, at the same level, studies suggest that it is possible to predict IL such as RPE from GPS training and match data (59), showing that the variation in workload efficiency throughout matches may be explained by the training load variables from the prior days and training cycles (60). In addition, an understanding of load dynamics across training and competition is presented as an important aspect related to performance enhancement in team sports (8, 47, 60, 61), showing, for example, how adjusting specific drills such as large,- medium- and small-sided games may help replicate game demands (86, 87). Correlations between EL and IL in different season periods were applied in order to understand the fluctuation of some metrics such as players’ strength (88), players’ recovery (89), or ACWR, between players’ positions, starters, and non-starters (82, 84, 90, 91). Also, Pizarro et al. (26) demonstrated how different occupational areas may promote different physical variables. The authors state how the manipulation of “floaters” in SSGs according to specific purposes in FTS promotes higher distances and speed variables, accelerations, and decelerations. The same was described by De Jong et al. (81), in which manipulation of the game momentum (with the outfield goalkeeper) promotes changes in team total distances covered (81). Silva et al. (92) demonstrated that teams have the ability to coadapt to performance constraints by manipulating SSGs. In addition, add-ons to SSGs, such as the number of players by drill, player positioning, or touch constraints, are being referenced as potential ways to increase or decrease EL (81, 93) (Table 2).
3.7. Applicability of the tracking system related to injuries
With regard to the analysis related to injury prediction, some kinematic variables such as distance covered and mechanical ones such as accelerations/decelerations were considered. For example, in elite female FH players, the authors verified that the risk for in-game knee injury decreased with the increasing distance covered while running at low speed (30). In the same path, Caparrós et al. (29), in a total of 2,613 observations and 246 games from 33 different players of a professional male BK team, described that athletes with less or equal than three decelerations per game and those running less or equal than 1.3 miles per game had a higher risk of injury. Predictive injury models are also proposed by the use of tracking systems (7). In this study with 26 Italian professional male players, the authors propose a multidimensional approach to injury forecasting in professional FTB based on GPS measurements (7). In addition, three studies from contact team sports (AUF and AMF) presented associations between specific levels of kinematic and mechanical variables with the occurrence of injuries (11, 99). Finally, some studies reported that lower workloads during preseason and higher workloads during competition training weeks increased the risk of non-contact injuries (100; Nobari et al., n.d.) (Table 3).
3.8. Applicability of the tracking system related to nutrition
Two studies that analyzed the variation in physical enhancement through nutritional intake were identified. Before and after the players received 140 mL of beetroot juice (BJ) or placebo (PLA) on two separate days, a sample of 10 competitive male BK players was monitored (27). To determine physical improvements, a specific neuromuscular battery of tests followed by a 40 min-simulated BK match was used to measure the players’ running profile, ACC, and DEC; however, acute moderate doses of BJ (12.8 mmol of NO3-) were not effective in improving neuromuscular performance (27). In the other study, the same author verified that the ingestion of moderate doses of caffeine enhanced several physical variables such as jump height, running profile, number of body impacts, ACC, and DEC in FTS professional players (28) (Table 4).
4. Discussion
The purpose of this scoping review was to understand the applicability of tracking systems in physical performance analysis in team sports over the last decade in order to gain an understanding of how manipulating different variables and research goals in this field may be beneficial for enriching the use of this technology and, as a result, for integrating the revised information in a comprehensive framework that could help clarify the physical performance area for team sports and athlete wellbeing. The main findings were that tracking technology has been applied in team sports as a valuable tool in the following research areas: (1) performance analysis of match demands; (2) performance analysis of training vs. match information; (3) injuries, and (4) nutrition. In addition, the groups of metrics mainly used are (1) kinematic and mechanical; (2) kinematic; (3) kinematic, mechanical, and metabolic. To seek an understanding of athletes’ performance, several studies establish correlational analysis between specific EL metrics and IL information. However, the described EL metrics are manipulated individually rather than in an integrative model, which should include, for example, the principal component analysis (PCA) of each team sport. This indicates that it is still promising to go further into the understanding of how tracking technology can be used to improve training and performance by combining IL and EL data. Finally, studies appear to focus primarily on (1) characterization and comparison of drills, training, and match sessions, followed by (2) correlational analysis between metrics. In line with this, it was possible to consider such relationships for the development of a conceptual framework that supports both applied sport-sciences research and practitioners’ monitoring and intervention (Figure 5). Indeed, the creation of high-performance departments that combine slow-thinking (applied sport-science research) and fast-moving intervention (applied monitoring and intervention) (103) supported by a coherent framework certainly promotes best preparation assessments and decision-making based on reliable data (103).
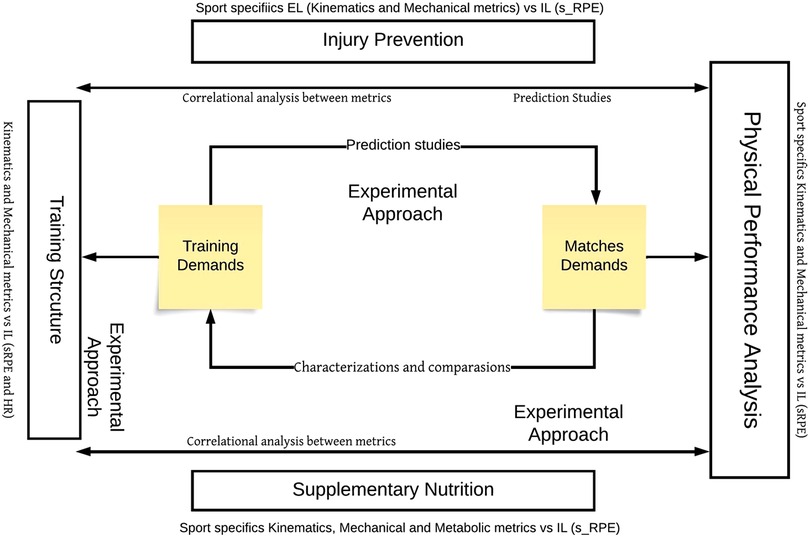
Figure 5. Flow chart of the contextual research applicability of tracking systems based on the screened domains.
4.1. Tracking technology and physical performance analysis of match demands and its comparison with training demands
In team sports, coaches and performance personnel invest a lot of time and resources to follow up on their athletes. Physical training can be described in terms of EL (work) and acute responses and long-term adaptations (IL) (104). Through the development of wearable technology for athletes over the past few years, team sports stakeholders have embraced the practice of monitoring their athletes' EL in both training and competition (54). Consequently, technology is used by coaches and exercise physiologists with the intent of enhancing training data and achieving competitive advantage (54). The focus on athlete performance improvement has been driving the need to understand training and match demands. Several researchers have been characterizing and understanding EL metrics (mainly kinematic and mechanical) during specific training or competitive contexts (32, 59). In addition, and as an attempt to enhance knowledge for training improvement, links have been established between kinematic, mechanical, and metabolic variables, and more recently with IL (86, 87). However, and assuming the physiological adaptations of different team sports exigencies, we suggest that stronger steps must be taken based on experimental approaches seeking to better understand the relationship between EL and IL and athletes’ recovery cycles (50, 53). In this regard, we suggest that studying Large-, Medium-, and Small-sided games (LSGs, MSGs, and SSGs) may be helpful in determining which format is most suited to load specific kinematic or mechanical loads in line with a desired IL (86, 87). Positioning, intragames, level of competition, and tactical comparisons have also been pointed out as references for the understanding of how performance may be improved by better understanding EL metrics, particularly in terms of frequency, duration, and intensity. In addition to the difficulties of collecting data at a top-elite level, in order to create a training program that accurately reflects the physiological demands of the game, we suggest that more elite team sports game-specific data are required. In indoor high-intermittent team sports and based on the metrics selected by Ohmuro et al. (75), in order to understand more accurately the demands of games, we suggest a monitoring of EL by effective playing time (in-game). Despite the fact that an agreement between coaches and athletes on overall RPE and sRPE, as well as RPE and sRPE, in moderate and hard categories, has already been recorded (13), there is a lack of information about how demanding is the playing time, by players’ interchange rotation, particularly in indoor intermittent team sports, and how can that information benefit the training structure, and consequently, team performance.
Team sports performance is regarded as highly complex and multivariate. Researchers integrate players' technique with EL and IL, while there are studies in which EL and IL are categorized by players’ position, exploring the differences between training and competition (73, 75, 98). This correlational approach seems to translate differences that are assessed for a better individual and collective preparation, as reported by Florian et al. (105),. When a selected EL fits with sports specification, we suggest that this type of method is reliable for better interpretation of competitive microcycles. As proposed by Ispyrlidis et al. (98), in FTB, and verified by Fernández et al. (47), in RH, this information may combine the interchanges of matches with competitive profile training and improve all parameters of players’ physical output. At the same level, research indicates that an understanding of load dynamics during training and competition, such as players' intra- and interweek fluctuations, exercises a substantial influence on improving elite athletes’ performance by properly regulating workload and recovery (82). In this review, it is found that several researchers in their studies have developed a longitudinal understanding of load dynamic (combined kinematic, mechanical, and metabolic metrics) across training and competition, proposing that performance enhancement may be a reflection of a proper adjustment of specific metrics (46, 67).
More recently, performance analysis research is stepping forward on the understanding of HIAs and on predictive studies for fatigue and match performance. The literature suggests that during different phases of a training cycle, such as the baseline or competition phase, training loads must be modulated to either enhance or reduce fatigue levels (89). In the light of this, it is important to understand the match HIA in order to manage fatigue properly, matching both variables to adaptation to training and for achieving competitive performance (10, 40). On the other hand, understanding HIAs and their impacts on IL also improves the knowledge of individual players’ recovery strategies (89). The importance of understanding effort categories (moderate and hard) has already been established in a systematic review, in which agreements between coaches and athletes about total RPE and s_RPE were reported (13). Therefore, in order to effectively adjust the volume, frequency, and intensity of the metrics that are more closely associated with game pick periods, this information can assist in developing novel approaches on tapering training strategies. In this sense, kinematic and mechanical variables are statistically manipulated with indirect IL such as RPE, suggesting that it is possible to predict fatigue from GPS/LPS training and match data. In addition, a dissociation between EL and IL units may reveal the state of fatigue of an athlete; therefore, detecting changes with statistical approaches may provide the necessary confidence for implementing changes (10). Nevertheless, in contact team sports such as AUF, AMF, RG, and GF, the use of mechanical metrics seems to be more significant to understanding players’ fatigue and muscle damage (32, 39, 43, 71).
Strength and conditioning coaches may daily obtain reports with more than 100 variables (106). Thus, it is crucial to choose and identify the key performance indicators according to sports specifications (63). In this review, we identified research focused on proposing models for match performance mainly based on kinematic, mechanical, and metabolic variables (44, 60–62, 99). These studies demonstrate how crucial it is to adopt statistical techniques like PCA in team sports to accurately use significant performance variables by excluding the least significant ones.
4.2. Tracking technology and injuries
Athletes compete fiercely to become the greatest in their respective sports, particularly at the highest level. In team sports, the individual or collective success of this process is often compromised by the occurrence of injuries (30). Therefore, in this review, we observed that the development of injury prevention programs is being supported by the association of tracking positioning systems such as the use of a GPS. Recently, studies tried to understand which (EL metrics) and how (volume, intensity, and frequency) training and game demands have a higher association with the occurrence of specific injuries such as the risk for in-game knee injury (30). In specific intermittent team sports such as FH and BK, low kinematics (low-intensity running) (30) and mechanical (DEC) are associated with a higher risk of injury (29). However these studies do not explain whether this lower EL results from acute or chronic adaptations, such as the one by Li et al. (11), which applied the use of technology to understand the relationship between soft tissue injury and training workload in AMF. For this author, the variation of training stimulus may induce a higher risk of injuries. Once studies have shown that the ACWR is a more accurate predictor of injury than the total workload (18, 101) it is highly advised to use tracking technology to determine an athlete's chronic workload. According to the screened manuscript (7, 11), we suggest that the assessment of an athlete's physical activity based on the intensity, duration, and number of actions of kinematic, mechanical, and metabolic metrics may also predict injuries with acceptable precision. Recently, a higher preseason workload, such as a running performance, was related to a lower risk of injuries (100). On the other hand, higher levels of weekly chronic workload (ACWR) indicate greater chances of sustaining non-contact injuries (84, 91). We suggest that these dichotomic reports may support the need for better longitudinal use of tracking systems in different team sports, in order to establish causal correlations between specific parameters, and consequently, to improve injury prevention measures. The sparse research on the use of tracking technology related to injury forecast is mainly noticed in outdoor team sports; thus, it is recommended that future studies extend to high-intermittent indoor team sports such as FTS, handball (HB), RH, and BK. Finally, there is no injury forecast method that links the most demanding scenarios (MDS) or HIAs in team sports according to specific EL variables and IL information. We suggest that characterizing the dynamic of training workload related to teams' sports MDS may contribute to more reliable specific injury predictions.
4.3. Tracking technology and nutrition
The use of nutritional supplements and ergogenic aids to improve performance is becoming more popular among intermittent sports (107), but few have demonstrated benefits (108) such as creatine, caffeine, sodium bicarbonate, and beta-alanine. Indoor team sports (BK, FTS, HB, RH, or netball) are becoming more intense, with explosive demands (37, 47) stronger athletes, and repeated combinations of short and long intermittent efforts (97). This review noticed almost no research on the use of tracking technology to measure nutritional impact in kinematic, mechanical, or metabolic metrics. In this review, only two studies were screened. With regard to the experimental approach developed to understand whether the nutritional supplement of beetroot juice had some impact on the monitored EL variables (27), no significant results were found. Furthermore, the effects of supplementation on physical performance and match running load or true exposure during a competition (total distance covered, speed achieved, number of accelerations and decelerations) in team sports are either sparse or unknown (27). In their recent work, López-Samanes et al. (28) demonstrated how the application of this technology could advance the experimental approach to athletes' nutritional development. The authors showed that 3 mg/kg of caffeine enhanced the physical variables associated with FTS, such as jump and sprint performance, and also improved kinematics (high-speed running) and mechanics (ACC/DEC) during a simulated FTS match. This finding can be interpreted as an opportunity for researchers not only to provide experimental proof of performance enhancement, but also to better understand the role of supplementary nutrition in an athlete's recovery process. To the best of our knowledge, there are no experimental studies aimed to understand athletes' recovery cycles with the influence of supplementary nutrition and monitored by tracking technology.
4.4. Limitations
The fact that this review only included papers published in English in the electronic databases of PubMed, Web of Knowledge, and Scopus may have led to the exclusion of relevant publications in other languages. Furthermore, the exclusion of all systematic reviews can be seen as a constraint because some categories related to the aims of this scoping review may not have been identified because of this exclusion.
4.5. Actual trends and future directions
Currently, research on the use of tracking systems is mainly focused on the physical performance analysis on matches, training vs. matches, followed by injuries, and nutrition. Both kinematic and mechanical variables combined, and then only kinematics, appear to be the primary observed metrics. In addition, we verified that in some studies, IL information is combined solely with kinematic or mechanical metrics. Few studies combined both metrics. These manipulated strategies used for integrating IL information with EL may have been established according to team sports specifications. Still, we suggest that tracking and studying kinematic, mechanical, and IL parameters together in training and matches would provide more accurate data for the purpose of enhancing our understanding of athletes’ performance and, consequently, to better manipulate the structure of drills, for example, the manipulation of LSGs, MLGs, and SSGs, according to specific physiological responses.
Based on the main results screened in this review, further research and practice should consider how frequency, volume, and intensity may be adjusted according to the optimal (1) training structure; (2) to promote injury prevention; (3) to consider nutritional supplements; and (4) to promote physical performance analysis. The integration of these four dimensions has not yet been proposed. Consequently, how can performance analysis lead to the process of enhancing athletes' performance? This integrative vision proposal illustrates the significance of the domains explored in this review and how they may be oriented in accordance with specified research methodologies for both outdoor and indoor team sports (Figure 5). Despite the existing studies, we consider that the combined information of training and match demands remains the main source of the physical performance analyses. However, the integrative and longitudinal contribution of the presented domains related to the proposed researched strategies may help produce more reliable and accurate information, leading to an enhancement of the training structure. The development of more correlational analyses between metrics or prediction studies will improve the understanding of athletes’ performance enhancement. Also, experimental and prediction studies, particularly in the domain of injury prevention and nutrition, as well as in the manipulation of the metrics measured and compared between training and match sessions, are highly recommended. Therefore, because of its impact on high levels of muscle damage, as well as neuromuscular and perceptual fatigue, longitudinal tracking of EL variables, including kinematic, mechanical, and metabolic metrics, when combined with IL, can provide insights into injury prediction and prevention measures. In addition, the application of tracking technology to nutrition in team sports is relatively unexplored, with limited research investigating the impact of nutritional supplements on such metrics. This emphasizes the current trends in research, the need for more integrative models combining EL and IL data, and the importance of experimental and prediction studies to advance the understanding of team sports' physical performance, injury prevention, and nutritional impact. Ultimately, the integration of these domains into a coherent framework can guide both applied sports science research and practical interventions, leading to an improvement in athlete preparation and decision-making based on reliable data, which may promote the implementation of new drills, training, or competitive designs.
Author contributions
AF: Conceptualization, Data curation, Formal Analysis, Investigation, Methodology, Writing – original draft. PD-M: Supervision, Validation, Writing – review and editing. HS: Conceptualization, Data curation, Methodology, Validation, Writing – review and editing. JV-D-S: Supervision, Writing – review and editing. BT: Conceptualization, Formal analysis, Methodology, Supervision, Validation, Writing – review and editing.
Funding
The author(s) declare that financial support was received for the research, authorship, and/or publication of this article.
This work was supported by the National Funding through the Portuguese Foundation for Sciences and Technology, under the project UI/BD/151484/2021 and UI/BD04045/2020.
Conflict of interest
The authors declare that the research was conducted in the absence of any commercial or financial relationships that could be construed as a potential conflict of interest.
The author(s) declared that they were an editorial board member of Frontiers at the time of submission. This had no impact on the peer review process and the final decision.
Publisher's note
All claims expressed in this article are solely those of the authors and do not necessarily represent those of their affiliated organizations, or those of the publisher, the editors and the reviewers. Any product that may be evaluated in this article, or claim that may be made by its manufacturer, is not guaranteed or endorsed by the publisher.
References
1. Taylor JB, Wright AA, Dischiavi SL, Townsend MA, Marmon AR. Activity demands during multi-directional team sports: a systematic activity demands during multi-directional team sports: a systematic review. Sports Med. (2017) 47(12):2533–51. doi: 10.1007/s40279-017-0772-5
2. Ryan S, Coutts AJ, Hocking J, Kempton T. Factors affecting match running performance in professional Australian football. Int J Sports Physiol Perform. (2017) 12(9):1199–204. doi: 10.1123/ijspp.2016-0586
3. Hausler J, Halaki M, Orr R. Application of global positioning system and microsensor technology in competitive rugby league match-play: a systematic review and meta-analysis. Sports Med. (2016) 46(4):559–88. doi: 10.1007/s40279-015-0440-6
4. Helwig J, Diels J, Röll M, Mahler H, Gollhofer A, Roecker K, et al. Relationships between external, wearable sensor-based, and internal parameters: a systematic review. Sensors. (2023) 23:2. doi: 10.3390/s23020827
5. Lovell TWJ, Sirotic AC, Impellizzeri FM, Coutts AJ. Factors affecting perception of effort (session rating of perceived exertion) during rugby league training. Int J Sports Physiol Perform. (2013) 8(1):62–9. doi: 10.1123/ijspp.8.1.62
6. Wallace LK, Slattery KM, Coutts AJ. The ecological validity and application of the session-RPE method for quantifying training loads in swimming. J Strength Cond Res. (2009) 23(1):33–8. doi: 10.1519/JSC.0b013e3181874512
7. Rossi A, Pappalardo L, Cintia P, Iaia FM, Fernàndez J, Medina D. Effective injury forecasting in soccer with GPS training data and machine learning. PLoS One. (2018) 13(7):1–15. doi: 10.1371/journal.pone.0201264
8. Rago V, Muschinsky A, Deylami K, Mohr M, Vigh-Larsen JF. Weekly training load in elite male ice hockey: practice versus competition demands. Int J Sports Physiol Perform. (2021) 17(2):270–7. doi: 10.1123/ijspp.2021-0188
9. Achten J, Jeukendrup AE. Heart rate monitoring: applications and limitations. Sports Med. (2003) 33(7):517–38. doi: 10.2165/00007256-200333070-00004
10. Halson SL. Monitoring training load to understand fatigue in athletes. Sports Med. (2014) 44:139–47. doi: 10.1007/s40279-014-0253-z
11. Li RT, Salata MJ, Rambhia S, Sheehan J, Voos JE. Does overexertion correlate with increased injury? The relationship between player workload and soft tissue injury in professional American football players using wearable technology. Sports Health. (2020) 12(1):66–73. doi: 10.1177/1941738119868477
12. Sánchez-Migallón V, López-Samanes Á, Del Coso J, Navandar A, Aagaard P, Moreno-Pérez V. Effects of consecutive days of matchplay on maximal hip abductor and adductor strength in female field hockey players. BMC Sports Sci Med Rehabil. (2022) 14(1):3. doi: 10.1186/s13102-021-00394-x. PMID: 34980243; PMCID: PMC8725242.
13. Inoue A, dos Santos Bunn P, do Carmo EC, Lattari E, da Silva EB. Internal training load perceived by athletes and planned by coaches: a systematic review and meta-analysis. Sports Med Open. (2022) 8(1):8–35. doi: 10.1186/s40798-022-00420-3
14. Lima-Alves A, Claudino JG, Boullosa D, Couto CR, Teixeira-Coelho F, Pimenta EM. The relationship between internal and external loads as a tool to monitor physical fitness status of team sport athletes: a systematic review. Biol Sport. (2022) 39(3):629–38. doi: 10.5114/BIOLSPORT.2022.107021
15. Rago V, Brito J, Figueiredo P, Costa J, Barreira D, Krustrup P, et al. Methods to collect and interpret external training load using microtechnology incorporating GPS in professional football: a systematic review. Res Sports Med. (2020) 28(3):437–58. doi: 10.1080/15438627.2019.1686703
16. Russell JL, McLean BD, Impellizzeri FM, Strack DS, Coutts AJ. Measuring physical demands in basketball: an explorative systematic review of practices. Sports Med. (2021) 51:81–112. doi: 10.1007/s40279-020-01375-9
17. Torres-Ronda L, Beanland E, Whitehead S, Sweeting A, Clubb J. Tracking systems in team sports: a narrative review of applications of the data and sport specific analysis. Sports Med Open. (2022) 8:1. doi: 10.1186/s40798-022-00408-z
18. Hulin BT, Gabbett TJ, Lawson DW, Caputi P, Sampson JA. The acute: chronic workload ratio predicts injury: high chronic workload may decrease injury risk in elite rugby league players. Br J Sports Med. (2016) 50(4):231–6. doi: 10.1136/bjsports-2015-094817
19. Gabbett TJ, Whyte DG, Hartwig TB, Wescombe H, Naughton GA. The relationship between workloads, physical performance, injury and illness in adolescent male football players. Sports Med. (2014) 44(7):989–1003. doi: 10.1007/s40279-014-0179-5
20. Page MJ, McKenzie JE, Bossuyt PM, Boutron I, Hoffmann TC, Mulrow CD, et al. The PRISMA 2020 statement: an updated guideline for reporting systematic reviews. BMJ. (2021) 372:n71. doi: 10.1136/bmj.n71
21. Faber IR, Bustin PMJ, Oosterveld FGJ, Elferink-Gemser MT, Nijhuis-Van der Sanden MWG. Assessing personal talent determinants in young racquet sport players: a systematic review. J Sports Sci. (2016) 34(5):395–410. doi: 10.1080/02640414.2015.1061201
22. Law M, Stewart D, Pollock N, Letts L, Bosch J, Westmorland M. Guidelines for critical review of qualitative studies based on guidelines for critical review form-qualitative studies by. Design. (2000) 91(4):357–62.
23. Landis JR, Koch GG. The measurement of observer agreement for categorical data. Biometrics. (1977) 33(1):159–74. doi: 10.2307/2529310
24. Ryan R, Hill S. Supporting implementation of cochrane methods in complex communication reviews: resources developed and lessons learned for editorial practice and policy. Health Res Policy Syst. (2019) 17(1):1–11. doi: 10.1186/s12961-019-0435-0
25. Corbett DM, Sweeting AJ, Robertson S. Weak relationships between stint duration, physical and skilled match performance in Australian football. Front Physiol. (2017) 8:1–8. doi: 10.3389/fphys.2017.00820
26. Pizarro D, Práxedes A, Travassos B, Gonçalves B, Moreno Domínguez A. Floaters as coach’s joker? Effects of the floaters positioning in 3vs3 small-sided games in futsal. Int J Perform Anal Sport. (2021) 21(2):197–214. doi: 10.1080/24748668.2020.1866861
27. López-Samanes Á, Parra AG, Moreno-Pérez V, Courel-Ibáñez J. Does acute beetroot juice supplementation improve neuromuscular performance and match activity in young basketball players? A randomized, placebo-controlled study. Nutrients. (2020) 12:1. doi: 10.3390/nu12010188
28. López-Samanes Á, Moreno-Pérez V, Travassos B, Del Coso J. Effects of acute caffeine ingestion on futsal performance in sub-elite players. Eur J Nutr. (2021) 60(8):4531–40. doi: 10.1007/s00394-021-02617-w
29. Caparrós T, Casals M, Solana Á, Peña J. Low external workloads are related to higher injury risk in professional male basketball games. J Sports Sci Med. (2018) 17(2):289–97. PMID: 29769830; PMCID: PMC5950746.
30. Kim T, Cha JH, Park JC. Association between in-game performance parameters recorded via global positioning system and sports injuries to the lower extremities in elite female field hockey players. Cluster Comput. (2016) 21(1):1069–78. doi: 10.1007/s10586-016-0690-6
31. Sweeting AJ, Aughey RJ, Cormack SJ, Morgan S. Discovering frequently recurring movement sequences in team-sport athlete spatiotemporal data. J Sports Sci. (2017) 35(24):2439–45. doi: 10.1080/02640414.2016.1273536
32. Mangan S, Ryan M, Devenney S, Shovlin A, McGahan J, Malone S, et al. The relationship between technical performance indicators and running performance in elite Gaelic football. Int J Perform Anal Sport. (2017) 17(5):706–20. doi: 10.1080/24748668.2017.1387409
33. Pino-Ortega J, Rojas-Valverde D, Gómez-Carmona CD, Bastida-Castillo A, Hernández-Belmonte A, García-Rubio J, et al. Impact of contextual factors on external load during a congested-fixture tournament in elite U’18 basketball players. Front Psychol. (2019) 10:1–11. doi: 10.3389/fpsyg.2019.01100
34. Conte D, Guerriero A, Lupo C, Felipe A, Arruda S. Influence of congested match schedules, pre-match well-being and level of opponents on match loads during world rugby women’s sevens series. Int J Environ Res Public Health. (2021) 18(22):12132. doi: 10.3390/ijerph182212132
35. Beard A, Chambers R, Millet GP, Brocherie F. Comparison of game movement positional profiles between professional club and senior international rugby union players. Int J Sports Med. (2019) 40(6):385–9. doi: 10.1055/a-0858-9973
36. Barron DJ, Collins D, Carling C. Match performance in a reference futsal team during an international tournament—implications for talent development in soccer christopher yiannaki. Biol Sport. (2020) 37(2):147–56. doi: 10.5114/BIOLSPORT.2020.93040
37. Ribeiro JN, Gonçalves B, Coutinho D, Brito J, Sampaio J, Travassos B. Activity profile and physical performance of match play in elite futsal players. Front Psychol. (2020) 11:1709. doi: 10.3389/fpsyg.2020.01709
38. Wiig H, Andersen TE, Luteberget LS, Spencer M. Individual response to external training load in elite football players. Int J Sports Physiol Perform. (2020) 15(5):696–704. doi: 10.1123/ijspp.2019-0453
39. Ryan S, Crowcroft S, Kempton T, Coutts AJ. Associations between refined athlete monitoring measures and individual match performance in professional Australian football. Sci Med Footb. (2021) 5(3):216–24. doi: 10.1080/24733938.2020.1837924
40. Ribeiro JN, Gonçalves B, Illa J, Couceiro M, Sampaio J, Travassos B. Exploring the effects of interchange rotations on high-intensity activities of elite futsal players. Int J Sports Sci Coach. (2022):6–12. doi: 10.1177/17479541221119659
41. Fernández J, Medina D, Gómez A, Arias M, Gavaldà R. Does training affect match performance? A study using data mining and tracking devices. CEUR Workshop Proc. (2016):1842.
42. Malone JJ, Di Michele R, Morgans R, Burgess D, Morton JP, Drust B. Seasonal training-load quantification in elite English premier league soccer players. Int J Sports Physiol Perform. (2015) 10(4):489–97. doi: 10.1123/ijspp.2014-0352
43. Owen SM, Venter RE, Du Toit S, Kraak WJ. Acceleratory match-play demands of a super rugby team over a competitive season. J Sports Sci. (2015) 33(19):2061–9. doi: 10.1080/02640414.2015.1028086
44. Pettersen SA, Johansen HD, Baptista IAM, Halvorsen P, Johansen D. Quantified soccer using positional data: a case study. Front Physiol. (2018) 9:1–6. doi: 10.3389/fphys.2018.00866
45. Polglaze T, Dawson B, Hiscock DJ, Peeling P. A comparative analysis of accelerometer and time—motion data in elite men’s hockey training and competition. Int J Sports Physiol Perform. (2015) 10(4):446–51. doi: 10.1123/ijspp.2014-0233
46. Tierney PJ, Young A, Clarke ND, Duncan MJ. Match play demands of 11 versus 11 professional football using global positioning system tracking: variations across common playing formations. Hum Mov Sci. (2016) 49:1–8. doi: 10.1016/j.humov.2016.05.007
47. Fernández D, Moya D, Cadefau JA, Carmona G. Integrating external and internal load for monitoring fitness and fatigue Status in standard microcycles in elite rink hockey. Front Physiol. (2021) 12:1–10. doi: 10.3389/fphys.2021.698463
48. Ritchie D, Hopkins WG, Buchheit M, Cordy J, Bartlett JD. Quantification of training and competition load across a season in an elite Australian football club. Int J Sports Physiol Perform. (2016) 11(4):474–9. doi: 10.1123/ijspp.2015-0294
49. Li AZ, Mao L, Krustrup P, Randers MB. Internal and external load during 8 v 8, 5 v 5 and 3 v 3 in Chinese elite youth male football players. Biol Sport. (2022) 39(4):1065–71. doi: 10.5114/biolsport.2022.113292
50. Rossi A, Perri E, Pappalardo L, Cintia P, Iaia FM. Relationship between external and internal workloads in elite soccer players: comparison between rate of perceived exertion and training load. Appl Sci (Switzerland). (2019) 9:23. doi: 10.3390/app9235174
51. Scott D, Lovell R. Individualisation of speed thresholds does not enhance the dose-response determination in football training. J Sports Sci. (2018) 36(13):1523–32. doi: 10.1080/02640414.2017.1398894
52. Carling CJ, Lacome M, Flanagan E, O’Doherty P, Piscione J. Exposure time, running and skill-related performance in international u20 rugby union players during an intensified tournament. PLoS One. (2017) 12(11):1–15. doi: 10.1371/journal.pone.0186874
53. Dempsey GM, Gibson NV, Sykes D, Pryjmachuk BC, Turner AP. Match demands of senior and junior players during international rugby league. J Strength Cond Res. (2018) 32(6):1678–84. doi: 10.1519/JSC.0000000000002028
54. Delaney JA, Duthie GM, Thornton HR, Pyne DB. Quantifying the relationship between internal and external work in team sports: development of a novel training efficiency index. Sci Med Footb. (2018) 2(2):149–56. doi: 10.1080/24733938.2018.1432885
55. Izzo R, Sopranzetti S, Hosseini Varde’I C, Molitierno G. Video tracking for the detection of external load to establish proper parameters in elite soccer players during high intensity training. J Phy Educ Sport. (2018) 18(2):1199–202. doi: 10.7752/jpes.2018.s2178
56. Neville J, Rowlands D, Wixted A, James D. Application of GPS devices to longitudinal analysis on game and training data. Procedia Eng. (2012) 34:443–8. doi: 10.1016/j.proeng.2012.04.076
57. Fernández D, Fernández D, Novelles A, Tarragó R. Comparing the most demanding passages of official matches and training drills in elite roller hockey. Apunts Educación física y deportes. (2020) 2(140):77–80. doi: 10.5672/apunts.2014-0983.es.(2020/2).140.11
58. Oliva-Lozano JM, Conte D, Fortes V, Muyor JM. Exploring the use of player load in elite soccer players. Sports Health. (2023) 15(1):61–6. doi: 10.1177/19417381211065768
59. Rossi A, Perri E, Trecroci A, Savino M, Alberti G, Iaia FM. GPS Data reflect players’ internal load in soccer. IEEE International Conference on Data Mining Workshops, ICDMW (2017), (September), p. 890–3. doi: 10.1109/ICDMW.2017.122
60. Grünbichler J, Federolf P, Gatterer H. Workload efficiency as a new tool to describe external and internal competitive match load of a professional soccer team: a descriptive study on the relationship between pre-game training loads and relative match load. Eur J Sport Sci. (2020) 20(8):1034–41. doi: 10.1080/17461391.2019.1697374
61. Illa J, Fernandez D, Reche X, Carmona G, Tarragó JR. Quantification of an elite futsal team’s microcycle external load by using the repetition of high and very high demanding scenarios. Front Psychol. (2020) 11(October):1–10. doi: 10.3389/fpsyg.2020.577624
62. Milanez VF, Bueno MJDO, Caetano FG, Chierotti P, De Moraes SMF, Moura FA. Relationship between number of substitutions, running performance and passing during under-17 and adult official futsal matches. Int J Perform Anal Sport. (2020) 20(3):470–82. doi: 10.1080/24748668.2020.1761673
63. Oliva-Lozano JM, Barbier X, Fortes V, Muyor JM. Key load indicators and load variability in professional soccer players: a full season study. Res Sports Med. (2021) 31(3):201–13. doi: 10.1080/15438627.2021.1954517
64. Suarez-arrones L. Match-play activity profile in elite women’s rugby union players. J Strength Cond Res. (2014) 28(2):452–8. doi: 10.1519/JSC.0b013e3182999e2b
65. Reilly B, Akubat I, Lyons M, Collins DK. Match-play demands of elite youth Gaelic football using global positioning system tracking. J Strength Cond Res. (2015) 29(4):989–96. doi: 10.1519/JSC.0000000000000714
66. Malone S, Solan B, Collins KD, Doran DA. Positional match running performance in elite Gaelic football. J Strength Cond Res. (2016) 30(8):2292–8. doi: 10.1519/JSC.0000000000001309
67. Fernandez J, Medina D, Gomez A, Arias M, Gavalda R. From training to match performance: a predictive and explanatory study on novel tracking data. IEEE 16th International Conference on Data Mining Workshops, ICDMW (2016), p. 136–43. doi: 10.1109/ICDMW.2016.0027
68. Cullen BD, Roantree MT, McCarren AL, Kelly DT, O’Connor PL, Hughes SM, et al. Physiological profile and activity pattern of minor Gaelic football players. J Strength Cond Res. (2017) 31(7):1811–20. doi: 10.1519/JSC.0000000000001667
69. Varley MC, Jaspers A, Helsen WF, Malone JJ. Methodological considerations when quantifying high-intensity efforts in team sport using global positioning system technology. Int J Sports Physiol Perform. (2017) 12(8):1059–68. doi: 10.1123/ijspp.2016-0534
70. Spice CA, Gordon DA, Smith L, Johnstone JA. Work rates of international hockey umpires. Int J Perform Anal Sport. (2017) 17(4):484–91. doi: 10.1080/24748668.2017.1353264
71. Bayliff GE, Jacobson BH, Moghaddam M, Estrada C. Global positioning system monitoring of selected physical demands of NCAA division i football players during games. J Strength Cond Res. (2019) 33(5):1185–91. doi: 10.1519/JSC.0000000000003137
72. Douglas AS, Kennedy CR. Tracking in-match movement demands using local positioning system in world-class men’s ice hockey. J Strength Cond Res. (2020) 34(3):639–46. doi: 10.1519/JSC.0000000000003414
73. Principe V, Vale R. (2020). GPS technology to control of external demands of elite Brazilian female football players during competitions (Tecnología GPS para controlar las demandas externas de las jugadoras d… GPS technology to control of external demands of elite Brazilian fema. doi: 10.47197/retos.v1i40.81943
74. Serrano C, Felipe JL, Garcia-Unanue J, Ibañez E, Hernando E, Gallardo L, et al. Local positioning system analysis of physical demands during official matches in the Spanish futsal league. Sensors (Switzerland). (2020) 20(17):1–11. doi: 10.3390/s20174860
75. Ohmuro T, Iso Y, Tobita A, Hirose S, Ishizaki S, Sakaue K, et al. Physical match performance of Japanese top-level futsal players in different categories and playing positions. Biol Sport. (2020) 37(4):359–65. doi: 10.5114/BIOLSPORT.2020.96322
76. Silva N, Travassos B, Gonçalves B, Brito J, Abade E. Pre-match warm-up dynamics and workload in elite futsal. Front Psychol. (2020) 11:584602. doi: 10.3389/fpsyg.2020.584602
77. Cunniffe E, Connor M, Beato M, Grainger A, McConnell W, McCarthy Persson U, et al. The influence of possession status on the physical output of male international hockey players. Int J Sports Sci Coach. (2021) 17(2):412–22. doi: 10.1177/17479541211033958
78. Novak AR, Impellizzeri FM, Trivedi A, Coutts AJ, McCall A. Analysis of the worst-case scenarios in an elite football team: towards a better understanding and application. J Sports Sci. (2021) 39(16):1850–9. doi: 10.1080/02640414.2021.1902138
79. Illa J, Fernandez D, Reche X, Serpiello FR. Positional differences in the most demanding scenarios of external load variables in elite futsal matches. Front Psychol. (2021) 12:625126. doi: 10.3389/fpsyg.2021.625126
80. Altmann S, Forcher L, Ruf L, Beavan A, Groß T, Lussi P, et al. Match-related physical performance in professional soccer: position or player specific? PLoS One. (2021) 16(9):22–4. doi: 10.1371/journal.pone.0256695
81. de Jong JPJ, Caetano FG, de Jong LMS, da Silva V, de Oliveira Bueno MJ, Santiago PRP, et al. The influence of the futsal outfield goalkeeper on Players’ running performance. Human Movement. (2022) 23(3):49–55. doi: 10.5114/hm.2022.107977
82. Nobari H, Alijanpour N, Martins AD, Oliveira R. Acute and chronic workload ratios of perceived exertion, global positioning system, and running-based variables between starters and non-starters: a male professional team study. Front Psychol. (2022) 13:860888. doi: 10.3389/fpsyg.2022.860888
83. Caro E, Campos-vázquez MÁ, Lapuente-sagarra M, Caparrós T. Analysis of professional soccer players in competitive match play based on submaximum intensity periods. PeerJ. (2022) 10:e13309. doi: 10.7717/peerj.13309
84. Nobari H, Arslan E, Martins AD, Oliveira R. Are acute: chronic workload ratios of perceived exertion and running based variables sensible to detect variations between player positions over the season? A soccer team study. BMC Sports Sci Med Rehab. (2022) 14(1):51. doi: 10.1186/s13102-022-00445-x
85. Rabano-Muñoz A, Suarez-Arrones L, Requena B, Asian-Clemente JA. Internal and external loads of young elite soccer players during defensive small-sided games. J Hum Kinet. (2023) 87(April):179–88. doi: 10.5114/jhk/162027
86. Beato M, de Keijzer KL, Costin AJ. External and internal training load comparison between sided-game drills in professional soccer. Front Sports Act Living. (2023) 5:1–10. doi: 10.3389/fspor.2023.1150461
87. Beato M, Vicens-bordas J, Peña J, Costin AJ. Training load comparison between small, medium, and large-sided games in professional football. Front Sports Act Living. (2023) 5:1165242. doi: 10.3389/fspor.2023.1165242
88. Pereira LA, Freitas TT. Variations in internal and external training load measures and neuromuscular performance of professional soccer players during a preseason training period. J Hum Kinet. (2022) 81:149–62. doi: 10.2478/hukin-2022-0012
89. Schuth G, Szigeti G, Dobreff G, Pasic A, Gabbett T, Szilas A, et al. Individual-specific relationship between external training and match load and creatine-kinase response in Youth National Team Soccer players. Sports Health. (2022) 15(5):700–709. doi: 10.1177/19417381221128822
90. Ammann L, Altmann S. Training and match load ratios in professional soccer—should we use player- or position-specific match reference values? Front Sports Act Living. (2023) 5:1–14. doi: 10.3389/fspor.2023.1151828
91. Nobari H, Khalili SM, Zamorano AD, Bowman TG, Granacher U. Workload is associated with the occurrence of non-contact injuries in professional male soccer players: A pilot study. Front Psychol. (2022) 13:925722. doi: 10.3389/fpsyg.2022.925722
92. Silva P, Travassos B, Vilar L, Aguiar P, Davids K, Araújo D, et al. Numerical relations and skill level constrain co-adaptive behaviors of agents in sports teams. PLoS One. (2014) 9(9):1–12. doi: 10.1371/journal.pone.0107112
93. Younesi AS, Rabbani A, Clemente FM, Sarmento H. Ession-to-session variations in external load measures during small-sided games in professional soccer players. Biol Sport. (2021) 38(2):185–93. doi: 10.5114/biolsport.2020.98449
94. Jones L, Marshall P, Denison J. Health and well-being implications surrounding the use of wearable GPS devices in professional rugby league: a Foucauldian disciplinary analysis of the normalised use of a common surveillance aid. Perform Enhanc Health. (2016) 5(2):38–46. doi: 10.1016/j.peh.2016.09.001
95. De Silva V, Caine M, Skinner J, Dogan S, Kondoz A, Peter T, et al. Player tracking data analytics as a tool for physical performance management in football: a case study from Chelsea football club academy. Sports. (2018) 6(4):1–13. doi: 10.3390/sports6040130
96. Hudson JF, Cole M, Morton JP, Stewart CE, Close GL. Daily changes of resting metabolic rate in elite rugby union players. Med Sci Sports Exer. (2020) 52(3):637–44. doi: 10.1249/MSS.0000000000002169
97. Ferraz A, Valente-Dos-santos J, Sarmento H, Duarte-Mendes P, Travassos B. A review of players’ characterization and game performance on male rink-hockey. Int J Environ Res Public Health. (2020) 17(12):1–21. doi: 10.3390/ijerph17124259
98. Ispyrlidis I, Gourgoulis V, Mantzouranis N, Gioftsidou A, Athanailidis I. Match and training loads of professional soccer players in relation to their tactical position. J Phy Educ Sport. (2020) 20(3):2269–76. doi: 10.7752/jpes.2020.s3305
99. Murray NB, Gabbett TJ, Townshend AD. The use of relative speed zones in Australian football: are we really measuring what we think we are? Int J Sports Physiol Perform. (2018) 13(4):442–51. doi: 10.1123/ijspp.2017-0148
100. Fisher P, Faulkner M, Mccann M. The association between pre-season running loads and injury during the subsequent season in elite Gaelic football. Sports. (2022) 10(8):117. doi: 10.3390/sports10080117
101. Gastin PB, Hunkin SL, Fahrner B, Robertson S. Deceleration, acceleration, and impacts are strong contributors to muscle damage in professional Australian football. J Strength Cond Res. (2019) 33(12):3374–83. doi: 10.1519/JSC.0000000000003023
102. Martins F, Marques A, França C, Sarmento H, Henriques R, Ihle A, et al. Weekly external load performance effects on sports injuries of male professional football players. Int J Environ Res Public Health. (2023) 20(2):1–12. doi: 10.3390/ijerph20021121
103. Coutts AJ. Working fast and working slow: the benefits of embedding research in high-performance sport. Int J Sports Physiol Perform. (2016) 11(1):1–2. doi: 10.1123/IJSPP.2015-0781
104. Impellizzeri FM, Rampinini E, Coutts AJ, Sassi A, Marcora SM. Use of RPE-based training load in soccer. Med Sci Sports Exerc. (2004) 36(6):1042–7. doi: 10.1249/01.MSS.0000128199.23901.2F
105. Florian P, Mehdi R, Moktar C, Serge C. Physical activity and high-intensity running during sided games vs. competitive match-play in elite soccer players: a comparative study. J Nov Physiother. (2016) 6(6):1–6. doi: 10.4172/2165-7025.1000320
106. Rojas-Valverde D, Pino-Ortega J, Gómez-Carmona CD, Rico-González M. A systematic review of methods and criteria standard proposal for the use of principal component analysis in team’s sports science. Int J Environ Res Public Health. (2020) 17(23):1–13. doi: 10.3390/ijerph17238712
107. Williams RL. Dietary supplements and regulation. J Am Med Assoc. (2011) 306(15):1657. doi: 10.1001/jama.2011.1494
Keywords: global positioning systems, local positioning systems, performance analysis, training and development, elite athletes
Citation: Ferraz A, Duarte-Mendes P, Sarmento H, Valente-Dos-Santos J and Travassos B (2023) Tracking devices and physical performance analysis in team sports: a comprehensive framework for research—trends and future directions. Front. Sports Act. Living 5:1284086. doi: 10.3389/fspor.2023.1284086
Received: 27 August 2023; Accepted: 6 November 2023;
Published: 23 November 2023.
Edited by:
Sergio José Ibáñez, University of Extremadura, SpainReviewed by:
Carlos David Gómez-Carmona, University of Extremadura, SpainAntonio Tessitore, Foro Italico University of Rome, Italy
© 2023 Ferraz, Duarte-Mendes, Sarmento, Valente-dos-Santos and Travassos. This is an open-access article distributed under the terms of the Creative Commons Attribution License (CC BY). The use, distribution or reproduction in other forums is permitted, provided the original author(s) and the copyright owner(s) are credited and that the original publication in this journal is cited, in accordance with accepted academic practice. No use, distribution or reproduction is permitted which does not comply with these terms.
*Correspondence: António Ferraz YW50ZmVycmF6QGhvdG1haWwuY29t
†ORCID António Ferraz orcid.org/0000-0001-6197-7040 Pedro Duarte-Mendes orcid.org/0000-0001-5055-2696 Hugo Sarmento orcid.org/0000-0001-8681-0642 João Valente-Dos-Santos orcid.org/0000-0003-0980-0269 Bruno Travassos orcid.org/0000-0002-2165-2687