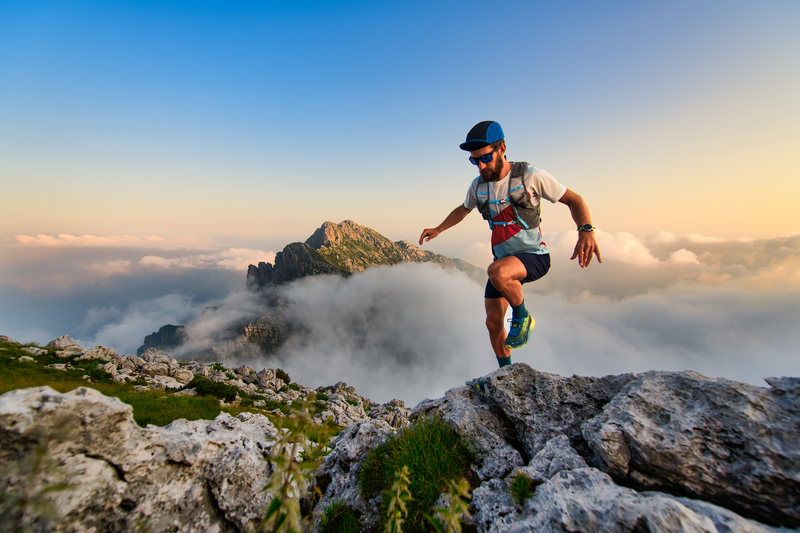
94% of researchers rate our articles as excellent or good
Learn more about the work of our research integrity team to safeguard the quality of each article we publish.
Find out more
ORIGINAL RESEARCH article
Front. Sports Act. Living , 24 May 2023
Sec. Elite Sports and Performance Enhancement
Volume 5 - 2023 | https://doi.org/10.3389/fspor.2023.1183881
Introduction: Due to the development in team handball, there is a need to optimize the physical capacities of team handball players for which knowledge of the physical match demands is essential. The aim of this study was to investigate the physical match demands of four LIQUI-MOLY Handball-Bundesliga (HBL) teams across three seasons with respect to the effects of season, team, match outcome, playing position, and halftime.
Methods: A fixed installed local positioning system (Kinexon) was used, collecting 2D positional and 3D inertial measurement unit data at 20 and 100 Hz, respectively. The physical match demands were operationalized by basic (e.g., distance, speed, and acceleration) and more advanced variables (e.g., jumps, throws, impacts, acceleration load, and metabolic power). A total of 347 matches (213 with an additional ball tracking) were analyzed from four teams (one top, two middle, and one lower ranked) during three consecutive seasons (2019–2022). One-way ANOVAs were calculated to estimate differences between more than two groups (e.g., season, team, match outcome, playing position). Mean differences between halftimes were estimated using Yuen’s test for paired samples.
Results: Large effects were detected for the season (), team (), and playing position (). Medium effects were found for match outcome () and halftime ().
Conclusion: For the first time, we provide a comprehensive analysis of physical match demands in handball players competing in the LIQUI-MOLY Handball-Bundesliga. We found that physical match demands differ on that top-level with up to large effect sizes concerning the season, team, match outcome, playing position, and halftime. Our outcomes can help practitioners and researchers to develop team and player profiles as well as to optimize talent identification, training, regeneration, prevention, and rehabilitation procedures.
Team handball is a contact team-sport with the objective to score more goals than the opponent (1). The match performance is a multidimensional construct, including physical, technical-tactical, cognitive, and further factors. It is determined by the individual performance of the players and their interaction (2). In team handball, the physical match demands involve activities as running, jumping, throwing, and blocking, whereby playing positional differences exist (3). During the last decades, it is assumed that the physical match demands have increased in team handball (4). Potential reasons for that development may be related to more competition per seasons (2), decreased performance differences between teams (5), and rule changes (e.g., passive game, goal keeper substitution, and fast throw off following a goal) (4). Due to that development, there is a need to optimize the physical capacities of team handball players for which knowledge of the physical match demands is essential (1).
To quantify physical match demands in team handball, player tracking technologies, particularly local positioning systems (LPS) and inertial measurement units (IMU), have become standard procedures (6). In fact, since the season 2019/2020, all matches of the German LIQUI-MOLY Handball Bundesliga are systematically tracked by these technologies (Kinexon Web). Based on the collected data, it is possible to compute basic (e.g., distance, speed, and acceleration) and more advanced variables (e.g., jumps, throws, impacts, acceleration load, and metabolic power), as indicators of the physical match demands (7–9). Specifically, such data may be helpful to develop team and player profiles as well as to optimize talent identification, training, regeneration, prevention, and rehabilitation procedures (10, 11).
Previous studies show that running related match demands such as total distance, speed, and acceleration do not differ with respect to the match outcome in top-level team handball (12, 13). However, other studies found differences between winning and losing teams in high-intensity actions (14, 15). Also, differences between playing positions were comprehensively investigated in previous studies (9–12, 16), indicating specific profiles for wingers, centers, and pivots. Additionally, fatigue during certain match periods was studied (2, 3, 17, 18) and the findings reveal that high-intensity movements as the number of stops, change of directions, and also one-to-one situations (3) as well as distances covered at fast running and sprinting (17) decrease from first to second half and also towards the end of both halves. However, there is no study that has investigated the aforementioned aspects of the physical match demands across multiple seasons and for several top-level handball teams. Since most of the previous studies have investigated one tournament or few players, there is a need for a comprehensive study, systematically taking the physical match demands of top-level handball teams by LPS and IMU technologies into account.
Thus, the aim of this study was to investigate the physical match demands of four LIQUI-MOLY Handball-Bundesliga teams across three seasons with respect to the effects of season, team, match outcome, playing position, and halftime.
Within a retrospective observational study, a range of common physical variables (7) (Table 1) of four men’s handball teams (A, B, C, and D) competing in the LIQUI-MOLY Handball-Bundesliga (HBL) were analyzed across the seasons 2019/20, 2020/21, and 2021/22. Since the season 2019/2020, all matches of the Handball-Bundesliga are tracked by the same LPS technology (Kinexon GmbH, Munich, Germany), which allowed valid comparisons. To investigate the effect of season, team, match outcome, playing position, and halftime, differences in means were analyzed. The match outcomes were obtained from the official HBL-LIQUI-MOLY website. The collected data is free available for each team competing in the HBL, and an approval was granted by the four clubs to analyze and publish the data. Therefore, Ethics Committee clearance was not required. All procedures were conducted in accordance with the Declaration of Helsinki.
Table 2 shows number of players, played matches, and observations for each playing position of the four LIQUID-MOLY Handball Bundesliga teams across the three seasons. The teams finished the seasons accordingly: Team A: 1st to 3rd place; Team B: 7th to 8th place; Team C: 6th to 9th place; and Team D: 11th to 13th place. Thus, the teams can be considered as top (Team A), middle (Team B and Team C), and lower ranked (Team D).
Table 2. Number of players, played matches and observations for each playing position of four LIQUID-MOLY Handball Bundesliga teams across three seasons.
A fixed installed LPS technology (Kinexon Perform LPS) was used, as described in detail elsewhere (21). The technology collected 2D positional and 3D IMU (accelerometer, gyroscope, and magnetometer) data at 20 and 100 Hz, respectively. Briefly, in all sports halls, 12 antennae were fix installed around the playing field connected to one base station. During the matches, the players wore a harness packed with a sensor at the center of the upper back, which transmitted time signals via radio technology to the antenna, and via a wide local area network to the base station. Additionally, a sensor was included in the balls to allow their tracking. The used technology has been considered as valid and reliable for movement patterns of indoor team sports including team handball (22–27). However, the metrics derived from a combination of LPS and IMU data (e.g., impacts and jumps) are less studied yet. Furthermore, the anchor and field placement could influence the LPS output (28).
Match summary statistic files (n = 394) were exported using the Kinexon system software (Kinexon Web Application, HBL-Cloud). The players were grouped into the following positions: wing (right and left winger), center (right and left back, center back), and pivot (16). The match outcomes were grouped into win, lost, and draw. After visiting the data, 11, 1, and 4 files were removed due to different column names, missing entries, and multiple entries of the same players, respectively. Thus, 378 files (347 matches) remained and were further checked for plausibility. In 134 matches, no ball was tracked, meaning that no passes and shots were reported. Thus, these matches were excluded from the throwing analysis. Additionally, two observations with 127 jumps and 65 impacts per match were removed due to implausible high counts. At least, all players with no playing time were excluded. Also, goalkeepers were excluded, because their clearly different technical-tactical demands compared to the other playing positions (12). To quantify the team performance, the sum of players for each physical variable was calculated, with exception of variables representing a maximum, where the mean of the players was used. Players that return after an injury were included in the data set. In total, 347 matches (213 with an additional ball tracking) with 109 different players were used for statistical analysis. A detailed description of all variables used in this study is shown in Table 1.
Data distribution were explored visually. To estimate differences between more than two groups (i.e., season, team, match outcome, and playing position), one-way ANOVAs on trimmed means (tr = 0.2) were calculated. The mean differences between halves were estimated using Yuens trimmed means test for paired samples (29). Effect sizes were computed, whereby , 0.30 to 0.50, and >0.50 correspond to small, medium, and large effects, respectively (29, 30). The results were reported with trimmed means and corresponding standard deviations as well as effect sizes from one-way ANOVA analysis. When large effects were detected by the ANOVA, pairwise comparisons were conducted on trimmed means. All mean differences were reported with 95% confidence intervals. A multiple testing Bonferroni correction was applied. An alpha level of 0.05 was used for statistical significance. All calculations and visualizations were conducted in R version 4.2.0 (31–33).
Table 3 shows the effects of the season on the physical match demands. There were medium and small effects ranging between and , respectively. Large effects were found for the accumulated acceleration load () and the maximum metabolic power (). The post-hoc tests show that the load was lower in 2019/2020 compared to 2020/2021 () and 2021/2022 (). Also, the maximum metabolic power was lower in 2019/2020 () and in 2020/2021 () compared to 2021/2022.
Table 3. Physical match demands of four LIQUI-MOLY Handball Bundesliga teams across three seasons (nall matches: 2019/2020 = 90, 2020/2021 = 136, 2021/2022 = 121; nball tracking: 2019/2020 = 33, 2020/2021 = 61, 2021/2022 = 119). Values are expressed as trimmed means (trim = 0.2) and corresponding standard deviation of summarized team performance with exception of variables representing a maximum, where the mean was used.
Table 4 shows the physical match demands of the four teams and the results from the ANOVA. There were large, medium, and small effects ranging between , , and , respectively. Additionally, Figure 1 shows the distributions and results from the post-hoc tests of the variables with large effect sizes.
Figure 1. Selected distributions of physical match demands from the four LIQUI-MOLY Handball Bundesliga teams. Pairwise comparisons were done on trimmed means. Variables were selected by large effects resulting from ANOVA on trimmed means. Variables without unit are presented as count.
Table 4. Physical match demands of the four LIQUI-MOLY Handball Bundesliga teams. Values are expressed as trimmed means (trim = 0.2) and corresponding standard deviation of summarized team performance with exception of variables representing a maximum, where the mean was used.
The mean differences of post-hoc test associated with Table 4 show that compared to Team B, Team A showed higher values for time at high () and very-low speed (), and numbers of low acceleration (), time at high () and low metabolic power (), and jumps (). Lower values were found for them for time at low speed (), time at medium metabolic power (), and passes ().
Compared to Team C, Team A had higher values for time at very-low speed (), time at high () and low metabolic power (). Lower values were found for them for time at low speed (), numbers of low acceleration (), time at medium metabolic power (), jumps (), and passes ( ).
Compared to Team D, Team A had higher values for time at high speed () and time at high metabolic power (). Lower values were found for them for time at low speed (), numbers of low acceleration (), time at medium metabolic power (), jumps (), and passes ( ).
Compared to Team C, Team B had higher values for time at very-low speed () and time at low metabolic power (). Lower values were found for them for time at high speed ( ), numbers of low acceleration ( ), time at high metabolic power zone (), and jumps ().
Compared to Team D, Team B had higher values for time at low speed () and time at medium metabolic power (). Lower values were found for them for time at high () and very-low speed (. Also, lower values were found in number of low acceleration ( ), time at high () and low metabolic power (), jumps (), and passes ( ).
Compared to Team D, Team C had higher values for time at high () and low speed ( ) and time at medium metabolic power ( ). Lower values were found for them for time at very-low speed (), time at low metabolic power (), jumps ( ), and passes ( ).
Table 5 summarizes the effects of the match outcome on the physical match demands. There were medium and small effects ranging between and , respectively.
Table 5. Physical match demands of four LIQUI-MOLY Handball Bundesliga teams dependent on the match outcome (nall matches: win = 196, draw = 28, lost = 147; nball tracking: win = 125, draw = 17, lost = 86). Values are expressed as trimmed means (trim = 0.2) and corresponding standard deviation of summarized team performance with exception of variables representing a maximum, where the mean was used.
Table 6 shows the effects of the playing position on the physical match demands and the corresponding results from the ANOVA. There were large, medium, and small effects ranging between , , and , respectively. Figure 2 displays differences in the distribution of the variables and summarizes the results from post-hoc tests for all large effect sizes.
Figure 2. Selected distributions of physical match demands from handball player of four LIQUI-MOLY Handball Bundesliga teams depending on the playing position. Pairwise comparisons were done on trimmed means. Variables were selected by large effects resulting from ANOVA on trimmed means. Variables without unit are presented as count.
Table 6. Physical match demands of four LIQUI-MOLY Handball Bundesliga teams dependent on the playing position. Values are expressed as trimmed means (trim 0.2) and corresponding standard deviation.
The mean differences of post-hoc test associated with Figure 2 show that compared to centers, wingers showed higher values for maximum running speed (), time at very-high () and high speed (), maximum acceleration (), numbers of very-high () and high acceleration (]), maximum deceleration (), and numbers of very-high () and high deceleration (). Additionally, wingers had higher values for maximum () and time at very-high metabolic power (]. Also, lower values were found for them for numbers of impacts ( ) and passes ).
Compared to pivots, wingers had higher values for maximum running speed (), time at very-high ( ) and maximum acceleration (), numbers of very-high () and high acceleration (), maximum deceleration ( ), and numbers of very-high () and high deceleration (). Also, wingers had higher values for maximum metabolic power ( ) and time at very-high metabolic power (). Furthermore, lower values were detected for them regarding numbers of impacts ( ).
Related to pivots, centers had higher values for passes ) and lower for time at high speed ().
Table 7 shows the effects of the halftime on the physical match demands. Medium and small effects were observed ranging between and , respectively.
Table 7. Physical match demands of four LIQUI-MOLY Handball Bundesliga teams dependent on the halftime. Values are expressed as trimmed means (trim 0.2) and corresponding standard deviation of summarized team performance with exception of variables representing a maximum, where the mean was used.
The aim of this study was to investigate the physical match demands of four LIQUI-MOLY Handball-Bundesliga teams across three seasons with respect to the effects of season, team, match outcome, playing position, and halftime. Our main findings show that there were up to large effects concerning the (i) season, (ii) team, and (iii) playing position. Up to medium effects were evident concerning the (iv) match outcome and (v) halftime.
The first main finding was that there were up to large effects concerning the season (Table 3). In fact, there was a large increase in the accumulated acceleration load from the season 2019/2020 to both consecutive seasons and an increase in maximum metabolic power in season 2021/2022. Our findings support the assumption that the match demands have increased in top-level team handball (2, 4, 5) and provide, for the first time, quantitative evidence for such a progress. Due to the calculation of the accumulated acceleration load as an overall acceleration-based metric (Table 1), its large effect may be caused by the medium differences observed in the playing time and time spent at very-high metabolic power as well as number of impacts and jumps (Table 3). In that context, one explanation for the lower accumulated acceleration load in 2019/2020 is that, during Covid-19 pandemic, players have dropped out and player rotation strategies may have changed, which could both influence the team performance. A further interesting observation was that the reduced number of matches played in the season 2019/2020 (Table 3) caused by the pandemic had no negative physical impact on the following seasons. Comparable results are reported concerning instructed home-based strength and endurance program in team handball players (34, 35). Taken overall, the results suggest that top-level team handball has changed into a more dynamic team sport. However, more longitudinal studies are needed to clarify our observations.
In team handball, the success, expressed by the match outcome, is determined by multiple factors (1). In this context, we discuss the effects of team and match outcome together. We found up to large and medium effects concerning the team (Figure 1 and Table 1) and match outcome (Table 5), respectively. While previous studies reported no differences in physical match demands between top- and lower-ranked or winning and losing teams (12, 13), another study showed that on the European Championship 2020 and World Championships Qatar 2015 the winning teams conducted more metabolic power associated running activities compared to losing teams (15). With respect to high-intensity activities, our outcomes support those of (15), namely that the higher-ranked team (Team A) spent more time at high-speed and high metabolic power, whereas they executed a smaller number of jumps and passes (Figure 1 and Table 4) compared to the lower ranked teams (Team B, C, and D). In this context, winning teams perform less passes than drawing teams, but do not differ in terms of high-intensity running actions (Table 5). These findings may provide a new perspective of the influence of physical match demands on success in top-level team handball. It can be hypothesized that all the players are physically well developed (12) and technical-tactical actions are more important to win a match at this top-level. In conclusion, our data suggest that the running related physical match demands alone are not suitable to explain the match outcomes in top-level team handball. Thus, more studies that consider the technical-tactical context to explain the success are needed.
The third main finding was that small to large effects of playing position on physical match demands were present (Figure 2 and Table 6). Concretely, wingers showed the highest amount of high-intensity runs, pivots received the most impacts, and center throwed the most passes (Figure 2 and Table 6). These findings are consistent with those from previous studies (9, 10, 16, 36), showing that pivots cover the shortest distance at sprinting, but still operate at high-intensity due to high number of impacts (10, 36). In contrast, wingers show the lowest number of impacts (10) and perform together with center backs the greatest amount of high-intensity runs (12, 16, 35, 36). Additionally, backs are characterized by a substantially higher number of passes and shots (10). One well-accepted explanation for the differences is related to the different technical-tactical roles of the playing positions (10). Wingers cover longer distances back in the defense compared to center and pivots, and are the favored pass recipient in fast break situations in offensive situations. Our results underline the high-speed running and acceleration capacities of wingers and the high passing demands of center players (Table 6). Future studies should examine how these results could be successfully transferred into position-specific training. In summary, existing position differences in physical match demands indicate that there are highly specific position roles in top-level team handball.
The last main finding was that there are up to medium effects of the halftime on physical match demands (Table 7). In detail, time spent at very-high speed and very-high metabolic power decreased from first to second half. These findings are in line with those from previous studies, which investigate differences between halftimes during matches in men’s Portuguese and Danish top-level handball leagues (3, 17, 18). These previous studies show that physical match demands, which are related to high-intensity movements such as fast-running (17) or one-on-one situations (3), are decreasing from the first to the second half. Also, studies show that fatigue may occurs in certain phases of a match, mainly towards the end of the halftimes (2, 18). Furthermore, recent studies show position-specific fatigue effects (16, 37). Explanations for lower high intensity running activities in the second half in our study may be related to the scoreline and subsequent tactical changes (e.g., formation and player rotation) or individual fatigue. However, it is noteworthy that fatigue can be caused by numerous central and peripheral factors (38), which remained unknown in observational studies as conducted here. For the clarification, if differences in high-intensity actions between halftimes are caused by fatigue, additional standardized maximal muscular tests combined with electrophysiological measures are needed (38, 39). In summary, high-intensity running activities are lower in the second compared to the first half. The underlining technical-tactical or physiological causes remained unknown and need further studies.
There are a few limitations, which should be mentioned. Normalizing the data to playing time concerning position differences might lead to different results (12). However, we decided to stay close to the raw values, because we could not consider offense and defense match segments. Also, we decided to use an ordinal scale for the match outcome. Reducing success to an ordinal scale may have led to a loss of information. Our decision based on the fact that a basic descriptive analysis, as conducted here, is the first step before building more complex models. Furthermore, studies indicate that the metabolic power model by Osgnach et al. (19) could underestimate the true energy expenditure (40, 41), which could lead to misinterpretations. At least deep insights into the calculation methods of certain variables (e.g., jumps, shots, passes, and impacts) could not be reported due to property rights. Therefore, more studies are needed that investigate the validity of the metabolic power approach (42) and the detected throws, impacts, jumps (43), and change of directions grounding on tracking based measures. All points can be considered by more detailed studies in the future.
For the first time, we provide a comprehensive analysis of physical match demands in handball players competing in the LIQUI-MOLY Handball-Bundesliga. We found that physical match demands differ on that top-level with up to large effect sizes concerning the season, team, match outcome, playing position, and halftime. Our outcomes can help practitioners and researchers to develop team and player profiles as well as to optimize talent identification, training, regeneration, prevention, and rehabilitation procedures, and gives numerous indications for future research directions.
The data analyzed in this study is subject to the following licenses/restrictions: The collected data is freely available for each team competing in the LIQUI-MOLY Handball Bundesliga. Requests to access these datasets should be directed toaW5mb0BsaXF1aW1vbHktaGJsLmRl.
CS, CB, and MWH substantially contributed to conception and design of the study. CS organized the database. CS and CB performed the statistical analysis. CS and MWH wrote the first draft of the manuscript. All authors contributed to the article and approved the submitted version.
The author(s) acknowledge support from the German Research Foundation (DFG) and Universität Leipzig within the program of Open Access Publishing.
We would like to thank J. Zemke for the data download.
The authors declare that the research was conducted in the absence of any commercial or financial relationships that could be construed as a potential conflict of interest.
All claims expressed in this article are solely those of the authors and do not necessarily represent those of their affiliated organizations, or those of the publisher, the editors and the reviewers. Any product that may be evaluated in this article, or claim that may be made by its manufacturer, is not guaranteed or endorsed by the publisher.
1. Wagner H, Finkenzeller T, Würth S, von Duvillard SP. Individual, team performance in team-handball: a review. J Sports Sci Med. (2014) 13:808–16. PMID: 25435773; PMCID: PMC4234950
2. Michalsik LB, Madsen K, Aagaard P. Physiological capacity, physical testing in male elite team handball. J Sports Med Phys Fitness. (2015) 55:415–29. PMID: 24402441
3. Póvoas SC, Seabra AF, Ascensão AA, Magalhães J, Soares JM, Rebelo AN. Physical, physiological demands of elite team handball. J Strength Cond Res. (2012) 26(12):3365–75. doi: 10.1519/JSC.0b013e318248aeee
4. Bilge M. Game analysis of olympic, world and european championships in men’s handball. J Hum Kinet. (2012) 35:109–18. doi: 10.2478/v10078-012-0084-7
5. Meletakos GP, Noutsos SK, Bayios AI. Stable and changing characteristics of high-level handball as evidenced from world men’s championships. J Phys Educ Sport. (2020) 20:1354–61. doi: 10.7752/jpes.2020.03187
6. Pino-Ortega J, Oliva-Lozano J, Gantois P, Nakamura FY, Rico-González M. Comparison of the validity and reliability of local positioning systems against other tracking technologies in team sport: A systematic review. Proc Inst Mech Eng P J Sport Eng Technol. (2021) 236:73–82. doi: 10.1177/175433712098823
7. Torres-Ronda L, Beanland E, Whitehead S, Sweeting A, Clubb J. Tracking systems in team sports: a narrative review of applications of the data and sport specific analysis. Sports Med Open. (2022) 8:15. doi: 10.1186/s40798-022-00408-z
8. van den Tillaar R, Bhandurge S, Stewart T. Can machine learning with imus be used to detect different throws and estimate ball velocity in team handball? Sensors. (2021) 21:7. doi: 10.3390/s21072288
9. Font R, Karcher C, Reche X, Carmona G, Tremps V, Irurtia A. Monitoring external load in elite male handball players depending on playing positions. Biol Sport. (2021) 38:475–81. doi: 10.5114/biolsport.2021.101123
10. Karcher C, Buchheit M. On-court demands of elite handball, with special reference to playing positions. Sports Med. (2014) 44:797–814. doi: 10.1007/s40279-014-0164-z
11. García-Sánchez C, Navarro RM, Karcher C. Physical demands during official competitions in elite handball: a systematic review. Int J Environ Res Public Health. (2023) 20:30. doi: 10.3390/ijerph20043353
12. Manchado C, Pueo B, Chirosa-Rios LJ, Tortosa-Martínez J. Time-motion analysis by playing positions of male handball players during the european championship 2020. Int J Environ Res Public Health. (2021) 18:15. doi: 10.3390/ijerph18062787
13. Cardinale M, Whiteley R, Hosny AA, Popovic N. Activity profiles, positional differences of handball players during the world championships in qatar 2015. Int J Sports Physiol Perform. (2017) 12:908–15. doi: 10.1123/ijspp.2016-0314
14. González-Haro P, Gómez-Carmona C, Bastida-Castillo A, Rojas-Valverde D, Gómez-López M, Pino Ortega J. Analysis of playing position, match status-related differences in external load demands on amateur handball: a case study. Rev Bras de Cineantropometria e Desempenho Hum. (2020) 22:13. doi: 10.1590/1980-0037.2020v22e71427
15. Venzke J, Schäfer R, Niederer D, Manchado C, Platen P. Metabolic power and success in men’s handball in the European championship. In In: Dela F, Piacentini M, Helge J, Calvo Lluch Á, Sáez E, Pareja Blanco F, Tsolakidis E, editors. 27th Annual Congress of the European College of Sport Science 30 August – 2 September 2022 Book of Abstracts, Sevilla, Spanien: European College of Sport Science (2022). 217 p.
16. Fleureau A, Rabita G, Leduc C, Buchheit M, Lacome M. Peak locomotor intensity in elite handball players: a first insight into player position differences and training practices. J Strength Cond Res. (2023) 37(2):432–8. doi: 10.1519/JSC.0000000000004247
17. Michalsik LB, Aagaard P, Madsen K. Locomotion characteristics, match-induced impairments in physical performance in male elite team handball players. Int J Sports Med. (2013) 34:590–9. doi: 10.1055/s-0032-1329989
18. Póvoas SCA, Ascensão AAMR, Magalhães J, Seabra AT, Krustrup P, Soares JC, et al. Analysis of fatigue development during elite male handball matches. J Strength Cond Res. (2014) 28:2640–8. doi: 10.1519/JSC.0000000000000424
19. Osgnach C, Poser S, Bernardini R, Rinaldo R, di Prampero PE. Energy cost, metabolic power in elite soccer: a new match analysis approach. Med Sci Sports Exerc. (2010) 42:170–8. doi: 10.1249/MSS.0b013e3181ae5cfd
20. Boyd LJ, Ball K, Aughey RJ. The reliability of minimaxx accelerometers for measuring physical activity in australian football. Int J Sports Physiol Perform. (2011) 6:311–321. doi: 10.1123/ijspp.6.3.311
21. Hoppe MW, Baumgart C, Polglaze T, Freiwald J. Validity and reliability of GPS and LPS for measuring distances covered and sprint mechanical properties in team sports. PLoS ONE. (2018) 13:21. doi: 10.1371/journal.pone.0192708
22. Alt PS, Baumgart C, Ueberschär O, Freiwald J, Hoppe MW. Validity of a local positioning system during outdoor and indoor conditions for team sports. Sensors. (2020) 20:10. doi: 10.3390/s20205733
23. Gamble ASD, Bigg JL, Pignanelli C, Nyman DLE, Burr JF, Spriet LL. Reliability and validity of an indoor local positioning system for measuring external load in ice hockey players. Eur J Sport Sci. (2023) 23(3):311–8. doi: 10.1080/17461391.2022.2032371
24. Blauberger P, Marzilger R, Lames M. Validation of player and ball tracking with a local positioning system. Sensors (Basel). (2021) 21:13.
25. Fleureau A, Lacome M, Buchheit M, Couturier A, Rabita G. Validity of an ultra-wideband local positioning system to assess specific movements in handball. Biol Sport. (2020) 37:351–7. doi: 10.5114/biolsport.2020.96850
26. Luteberget LS, Holme BR, Spencer M. Reliability of wearable inertial measurement units to measure physical activity in team handball. Int J Sports Physiol Perform. (2018) 13:467–73. doi: 10.1123/ijspp.2017-0036
27. Pillitteri G, Thomas E, Battaglia G, Navarra GA, Scardina A, Gammino V, et al. Validity and reliability of an inertial sensor device for specific running patterns in soccer. Sensors. (2021) 21:10. doi: 10.3390/s21217255
28. Luteberget LS, Spencer M, Gilgien M. Validity of the catapult clearsky t6 local positioning system for team sports specific drills, in indoor conditions. Front Physiol. (2018) 9:10. doi: 10.3389/fphys.2018.00115
29. Mair P, Wilcox R. Robust statistical methods in R using the WRS2 package. Behav Res Methods. (2020) 52:464–88. doi: 10.3758/s13428-019-01246-w
30. Wilcox RR, Tian TS. Measuring effect size: a robust heteroscedastic approach for two or more groups. J Appl Stat. (2011) 38:1359–68. doi: 10.1080/02664763.2010.498507
31. Fox J, Weisberg S. An R companion to applied regression. 3rd ed. Thousand Oaks, CA: Sage (2019).
32. R Core Team. R: A Language and Environment for Statistical Computing. Vienna, Austria: R Foundation for Statistical Computing (2021).
33. Dowle M, Srinivasan A. data.table: extension of ‘data.frame‘ (2022). Available from: https://r-datatable.com, https://Rdatatable.gitlab.io/data.table, https://github.com/Rdatatable/data.table.
34. Fikenzer S, Fikenzer K, Laufs U, Falz R, Pietrek H, Hepp P. Impact of COVID-19 lockdown on endurance capacity of elite handball players. J Sports Med Phys Fitness. (2021) 61:977–82. doi: 10.23736/S0022-4707.20.11501-9
35. Font R, Irurtia A, Gutierrez J, Salas S, Vila E, Carmona G. The effects of COVID-19 lockdown on jumping performance and aerobic capacity in elite handball players. Biol Sport. (2021) 38:753–9. doi: 10.5114/biolsport.2021.109952
36. Manchado C, Tortosa Martínez J, Pueo B, Cortell Tormo JM, Vila H, Ferragut C, et al. High-performance handball player’s time-motion analysis by playing positions. Int J Environ Res Public Health. (2020) 17:15. doi: 10.3390/ijerph17186768
37. Venzke J, Schäfer R, Niederer D, Manchado C, Platen P. Metabolic power in the men’s European Handball Championship 2020. SportRxiv.
38. Thorpe RT, Atkinson G, Drust B, Gregson W. Monitoring fatigue status in elite team-sport athletes: implications for practice. Int J Sports Physiol Perform. (2017) 12:S2-27–S2-34. doi: 10.1123/ijspp.2016-0434
39. Brochhagen J, Ackermann N, Heinrich L, Hoppe M. GPS-Daten zur Detektion von muskulärer Ermüdung in den Sportspielen. LSB. (2022) 63:57–74.
40. Fuchs PX, Fuchs P, von Duvillard SP, Wagner H, Shiang T-Y. Critical assessment of a wide-spread method for estimating energy expenditure during accelerated running based on positioning tracking systems. J Sport Health Sci. (2022) 11:641–3. doi: 10.1016/j.jshs.2022.03.001
41. Fuchs P, Luteberget LS, Fuchs PX, Wagner H. Comparative analysis of the indirect calorimetry and the metabolic power method to calculate energy expenditure in team handball. Appl Sci. (2022) 12:10. doi: 10.3390/app12041952
Keywords: LPS, IMU, team performance, handball, activity, tracking, metabolic power
Citation: Saal C, Baumgart C, Wegener F, Ackermann N, Sölter F and Hoppe MW (2023) Physical match demands of four LIQUI-MOLY Handball-Bundesliga teams from 2019–2022: effects of season, team, match outcome, playing position, and halftime. Front. Sports Act. Living 5:1183881. doi: 10.3389/fspor.2023.1183881
Received: 10 March 2023; Accepted: 3 May 2023;
Published: 24 May 2023.
Edited by:
João Brito, Portuguese Football Federation, PortugalReviewed by:
Herbert Wagner, University of Salzburg, Austria© 2023 Saal, Baumgart, Wegener, Ackermann, Sölter and Hoppe. This is an open-access article distributed under the terms of the Creative Commons Attribution License (CC BY). The use, distribution or reproduction in other forums is permitted, provided the original author(s) and the copyright owner(s) are credited and that the original publication in this journal is cited, in accordance with accepted academic practice. No use, distribution or reproduction is permitted which does not comply with these terms.
*Correspondence: Christian Saal Y2hyaXN0aWFuLnNhYWxAdW5pLWxlaXB6aWcuZGU=
Disclaimer: All claims expressed in this article are solely those of the authors and do not necessarily represent those of their affiliated organizations, or those of the publisher, the editors and the reviewers. Any product that may be evaluated in this article or claim that may be made by its manufacturer is not guaranteed or endorsed by the publisher.
Research integrity at Frontiers
Learn more about the work of our research integrity team to safeguard the quality of each article we publish.