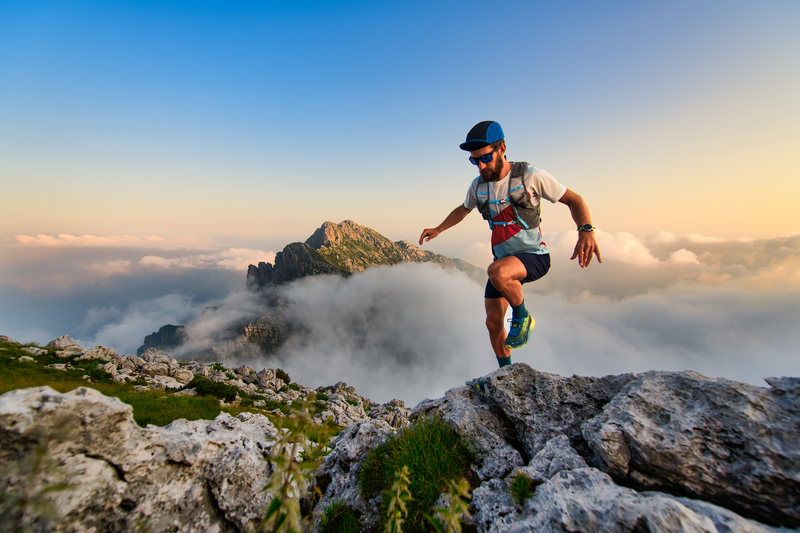
94% of researchers rate our articles as excellent or good
Learn more about the work of our research integrity team to safeguard the quality of each article we publish.
Find out more
ORIGINAL RESEARCH article
Front. Astron. Space Sci. , 27 November 2024
Sec. Planetary Science
Volume 11 - 2024 | https://doi.org/10.3389/fspas.2024.1485483
Some of the most common life detection techniques for planetary exploration focus on organic molecule characterization, but life on other planets may not chemically resemble that found on Earth. Therefore, an agnostic detection system of signs of life (biosignatures) is essential. Assembly Theory (AT) is a conceptual tool for understanding evolution and object formation that has been useful in developing an approach to quantify molecular complexity via the Molecular Assembly index, which when combined with abundance, allows the total assembly number of a sample to be calculated. Because AT makes no assumptions about the chemistry of life, it is an agnostic tool that identifies molecular structures that are probabilistically more likely to have arisen via selection and therefore biological processes. AT uses graph theory to quantify molecular complexity by finding the shortest sequence of joining operations (e.g., chemical bonds) required to build a compound from a set of starting materials allowing recursive reuse of units or fragments. For molecules, this number of steps is the MA value. We explore the use of Fourier transform (i.e., Orbitrap) mass spectrometry for approximating MA by quantifying how a molecule breaks apart into fragments. We analyze amino acid and nucleoside standards individually and as mixtures, as well as amino acids from naturally occurring biological and meteoritic sources. Aside from sample type, we evaluate the effect of analyte concentration and fragmentation energies on the generated MA value. Additionally, an older Orbitrap model similar to flight prototype instrumentation, was tested. The raw mass spectrometry data was compared with two different MA processing algorithms - one that uses the parent molecule spectrum and molecular weight (recursive) and one that does not (non-recursive). Concentration, fragmentation energy, and sample type all influence the raw mass spectra. However, the recursive algorithm reports MA estimates that are more consistent across sample types, concentrations, and fragmentation energies. We discuss instrument requirements for approximating MA that can be applied to future flight and sample return missions.
The origin and proliferation of life on Earth remains a mystery and the search for life beyond our planet is a difficult feat. Of importance for this exploration is an understanding of detectable signals that constitute life, especially given that life in other parts of the solar system is likely very different from life on Earth. Biosignatures, signs of life, past or present, are defined on a variety of levels from planetary to molecular (Schweiterman et al., 2018; Chan et al., 2019). An agnostic approach to biosignature detection requires the ability to recognize functions, structures, patterns, and/or remains of unknown life. Many analytical instruments exploring our solar system, like mass spectrometers, focus on measuring chemical signals (e.g., Mahaffy et al., 2012; Chou et al., 2021) as a means of detecting molecular structures, patterns, and potential signatures of life.
Mass spectrometers have long been used for compound identification and have a history in solar system exploration (e.g., see Chou et al., 2021 for a review). Several flight missions have mass spectrometers planned or in development such as The Venus Mass Spectrometer aboard the Deep Atmosphere Venus Investigation of Noble Gases, Chemistry, and Imagining - DAVINCI - probe or the Dragonfly mass spectrometer aboard the Dragonfly lander which will explore Titan. Amongst those in development for future space flight missions are Orbitrap mass spectrometers, like the LAbCosmOrbitrap prototype instrument (Arevalo et al., 2018; Briois et al., 2016; Selliez et al., 2023). Orbitrap™ mass spectrometry is a type of Fourier transform mass spectrometry where ions are injected into the mass analyzer in packets and the frequency of their axial oscillation around a central electrode is analyzed (Zubarev and Makarov, 2013; Hecht et al., 2019). The frequency of these oscillations is then converted into mass-to-charge ratios (m/z) which allows for compound identification and differentiation. This type of mass spectrometry is equipped with a nitrogen-filled collision cell to fragment ions produced by the ionization source at different energies for further identification aid. Fourier transform mass spectrometers (FT-MS) have high mass resolving power (105−6 M/ΔM) and low sample size requirements (pmol range) making them attractive instruments for low abundance samples across Earth and space.
FT-MS can be interfaced with a variety of ionization sources which makes it a versatile tool for analytical chemistry. Electrospray ionization is capable of ionizing intact molecules (i.e., as a molecular ion), which circumvents issues of recombination or the destruction of chemical information that is common for other types of ionization (e.g., electron impact ionization that is used in gas chromatography mass spectrometry, GC-MS). However, electrospray ionization is biased towards more polar molecules and requires liquid sample introduction and ionization (i.e., high voltage is applied to a liquid spray). An intact molecular ion (in positive ionization mode this is a protonated form, M + 1, and in negative ionization mode this is a deprotonated form, M-1) can be isolated by the quadrupole (a mass filtering component, Figure 1) with a narrow mass window (e.g., ± 0.5 m/z) and fragmented in the collision cell (Figure 1), allowing for increased probability that generated fragments come from a specific parent molecule. Additionally, analysis by electrospray ionization does not require derivatization (chemical modification of functional groups to make analytes more volatile and improve chromatographic separation, often required for GC-MS), and solvent choice combined with negative or positive ion polarity mode selection can be used to optimize ionization efficiency for individual molecules or mixtures.
Figure 1. Schematic of the Q Exactive Orbitrap mass spectrometer with components identified. Abbreviations are as follows: H-ESI II: Heated-electrospray ionization II. C-Trap: Curved Linear Trap. HCD: High-energy Collision-induced Dissociation. Ionization and ion transmission occurs between components 1–3. The quadrupole mass filter (4) filters ions by m/z. The transfer multipole (5) transfers ions from the quadrupole into the C-Trap (6). Ions are collected in “packets” (ion amounts in each packet are defined by the Automatic Gain Control Target setting in the instrument software) before being sent into the Orbitrap mass analyzer (8) for analysis. Each packet analysis is called a “scan.” Additional fragmentation is performed in the HCD cell (7) using nitrogen as a collision gas.
Mass spectrometry has a history in planetary science (see Chou et al., 2021 for a review) and is a key tool for disentangling the origins of compounds in natural mixtures. Understanding the formation of molecules with increasing structural complexity and size is important for the study of the origins of life and the search for life on other worlds (Chan et al., 2019). Defining and quantifying complexity is something of debate, and several molecular complexity metrics have been proposed (e.g., Hazen et al., 2007; Rouvray and Bonchev, 2003); however, none of these measurements are directly measurable experimentally. Assembly Theory (AT) is one such theory that investigates the smallest number of steps required to form a given product from a defined set of starting materials (Marshall et al., 2021; Marshall et al., 2017; Sharma et al., 2023), and is measurable spectroscopically (Jirasek et al., 2024). This theory is not specific and can be applied to a variety of object formation questions, among them the construction of molecules. Because AT does not consider chemical or thermodynamic constraints, it is useful for recognizing chemistries of complex systems that may differ from what is known on Earth, making it agnostic.
The Molecular Assembly (MA) Index is based on AT but specifically describes the number of unique joining operations (bond formations) to build a molecule (Marshall et al., 2017) using recursive operations from existing pieces or fragments. This empirical index has only been measured in two studies: one by Marshall et al. (2021) and one by Jirasek et al. (2024). Marshall et al. (2021) argued that only living systems can make molecules above a certain level of complexity in abundance and untargeted MA approximation using analytical instruments should be able to detect this resulting “anomaly”. The theoretical MA value for a given molecule is calculated as the number of joining operations, adding single atoms or subunits of atoms, required to form a defined molecule (bottom up) (Marshall et al., 2017; Marshall et al., 2021). Empirical estimation of MA for single molecules was demonstrated using nuclear magnetic resonance spectroscopy and infrared spectroscopy by monitoring the observed chemical shift or vibrational energies of bonds within a molecule (Jirasek et al., 2024). MA estimation was also determined by Fourier transform tandem mass spectrometry (FT-MS, i.e., Orbitrap™; Marshall et al., 2021; Jirasek et al., 2024). Experimental MA estimation, specifically by FT-MS, is the reverse of the theoretical method since it focuses on how molecules break apart (i.e., fragment) during instrument analysis. Estimation of MA from generated fragments was demonstrated for single molecules as well as chemical mixtures and environmental samples (Marshall et al., 2021). The number of fragments detected correlates strongly with AT numbers and molecular weight.
Only two previous studies have focused on describing the relationship between different analytical techniques and theory (Marshall et al., 2021; Jirasek et al., 2024). Method optimization or testing how analytical choices (like different instrument settings and sample workup procedures) influence the correlation between fragments and MA estimations has not yet been done. Such choices can dramatically influence the number of fragments observed and consequently the estimated MA value, potentially leading to over/under-estimation (determining a molecule to be more complex than it is) or negative results.
In this paper, we address this problem by analyzing amino acids and nucleosides using a commercially available Q Exactive Orbitrap™ mass spectrometer (Thermo Fisher Scientific, 2024) to investigate the effects of concentration, fragmentation energy, and different sample types. The Q Exactive Orbitrap is a widely used mass spectrometer for proteomics and metabolomics research and Orbitrap mass spectrometers in general are a part of the instrumentation suites of many laboratories around the world, thus MA estimation using this instrumentation should be widely accessible. Amino acids and nucleosides were selected as target analytes because they are small molecules (molecular weight <250 Da) that include well-known fragmentation patterns and a range of functional groups, and because they are relevant for biosignature detection as they can be formed by both biological and abiotic processes. We also test a selection of molecules on an LTQ-XL Orbitrap mass spectrometer (Thermo Fisher Scientific, 2024), an older, also widely used, model which employs a different method of mass filtration (ion trap), has slower scan rates (3–5 Hz versus 10–12 Hz for the Q Exactive), and is a better analog for the LAb CosmOrbitrap (Arevalo et al., 2018; Briois et al., 2016), a space flight prototype instrument. We discuss how these different parameters influence MA estimates and review data processing steps for users interested in making MA measurements on Earth-based samples as well as researchers and engineers involved in designing instrumentation for future space exploration missions.
Standard 5 mM solutions of each compound (Figure 2) were prepared in water and diluted to 50 μM in milliQ:methanol:formic acid (3:7:0.1% v/v). LC-MS grade methanol (Fisher Chemical) and reagent grade formic acid (Thermo Scientific) were used. Standard mixtures were prepared from the 5 mM stock solutions used for pure compound analyses and contained leucine, phenylalanine, valine, methionine, proline, and tryptophan. The nucleoside standard mixture was composed of adenosine, cytidine, and uridine. Four compounds (cytidine, leucine, methionine, tryptophan) were analyzed in pure form to determine how concentration influenced the number of MS2 fragments produced. The samples for the concentration series were diluted from a 1 mg/mL stock dissolved in milliQ:methanol:formic acid (3:7:0.1% v/v). The concentration ranged from 0.03 to 125 ng/μL (305.18 pg–1.25 μg of analyte consumed for a 1 min acquisition at a 10 μL/min flow rate). To ensure there was no carry-over between samples of different concentrations, the syringe was rinsed with 1.5 mL methanol, the PEEK tubing (connecting the syringe and source) was rinsed with 500 μL methanol, and methanol blanks were analyzed between samples. To quantify fragments and calculate MA, MS2 analyses were performed on the molecular ion with a ± 0.5 m/z isolation window in positive ion mode (M+1). The scan range was 50–300 m/z, resolution was 35,000. Source parameters were as follows: Sheath gas flow = 10, Auxiliary gas flow = 1, Spray voltage = 4 kV, Capillary temperature = 320°C, and S-Lens RF = 50. Each compound was analyzed at six different HCD cell energies: 10, 35, 45, 50, 100, and 150 Normalized Collision Energy™ (NCE) by direct infusion for 1 min at a flow rate of 10 μL/min. The amount of energy required to fragment a molecule is linearly correlated with m/z (Révész et al., 2023). For example, a molecule with lower molecular weight (i.e., m/z) will require less energy than a higher molecular weight molecule. Normalized Collision Energy™ accounts for the mass-dependent effects by scaling the amount of energy with the m/z being isolated (Thermo Fisher Product Support Bulletin 104).
Figure 2. Details of the individual molecules analyzed for fragmentation patterns and molecular assembly estimates by mass spectrometry including chemical structures, molecular weight, theoretical molecular assembly number (MA), and expected number of fragments from Zhang et al. (2019) for amino acids and Liu et al. (2008) for nucleosides.
Amino acid hydrolysates from lyophilized Spirulina (100 mg each for two different pretreatment methods) and the Murchison meteorite (201 mg) were analyzed. The first Spirulina treatment (Spirulina-hot water) and Murchison were hot water extracted with milliQ water heated in a flame-sealed vial for 24 h at 100°C (Elsila et al., 2021). Samples were then centrifuged, supernatant was removed, followed by dry-down in a Centrivap. Once dry, samples were hydrolyzed by vapor phase hydrolysis in 6 N hydrochloric acid (ACS reagent grade, Sigma-Aldrich) for 3 h at 150°C. The second Spirulina treatment (Spirulina-hydrolysis only) was not subjected to hot water extraction, but amino acids were liberated from proteins using vapor phase hydrolysis as described above. Following hydrolysis, samples were desalted using Dowex 50W-X8, 100–200 mesh, hydrogen form resin in prepacked columns purchased from Bio-Rad. The Dowex resin was cleaned using a water rinse and the active groups were removed using 2 M sodium hydroxide (95%–100.5%, Spectrum Chemical MFG Corporation). The resin was then rinsed with milliQ water and reactivated using 1.5 N hydrochloric acid (ACS reagent grade, Sigma-Aldrich), and rinsed with milliQ water. Samples were loaded on the Dowex resin and then rinsed with milliQ water. The samples were then eluted from the resin with 2 M ammonium hydroxide (ACS reagent grade, Supelco). The eluent was dried down using a Centrivap and samples were resuspended in 3:7:0.1% water:methanol:formic acid prior to analysis. Amino acid abundances were much lower for Murchison, so the flow rate was set to 5 μL/min instead of the 10 μL/min used for other samples and only leucine, phenylalanine, and valine were analyzed.
The pure 50 μM samples were also analyzed on an LTQ-XL Orbitrap mass spectrometer (LTQ) that resembles flight prototype instruments like the LAbCosmOrbitrap (Arevalo et al., 2018; Briois et al., 2016). The LTQ is an older model with less sensitivity and slower scan rates than the Q Exactive (Eliuk and Makarov, 2015). The LTQ performs mass filtration using an ion trap whereas the Q Exactive is equipped with a quadrupole mass filter (Figure 1). There are trade-offs between these two types of mass separation, but overall quadrupole mass filters push all oscillating ions through to the detector whereas ion traps discharge any ions with unstable oscillations. Both instruments contain a high-energy collision-induced dissociation (HCD) cell for fragmentation using nitrogen as the collision gas. The geometry of the Orbitrap-HCD cell-Curved linear-Trap (C-Trap) configuration is more compact on the Q Exactive, resulting in improved sensitivity over the LTQ (Eliuk and Makarov, 2015). Both instruments were equipped with a syringe pump for direct infusion of analytes without online separation. Both instruments use electrospray ionization, but The LTQ has a non-heated electrospray ionization (ESI) source whereas the Q Exactive is equipped with a heated electrospray ionization source (HESI II). The impact of fragmentation energy on pure compounds was tested on the LTQ using the same six HCD cell energies used for the Q Exactive. Source parameters were as follows: Sheath gas flow = 5, Auxiliary gas flow = 0.1, Spray voltage = 3.8 kV, Capillary temperature = 275°C, and Tube lens = 110.
To assess the impacts of different instrument settings, the top twenty most abundant ions were exported from instrument. RAW files using the standard instrument results processing program Qual Browser (Thermo). Fragmentation spectra were compared with data from Zhang et al. (2019); Liu et al. (2008). Fragment ions were included in the comparisons if they were ≥0.5% relative abundance of the most abundant ion for a given acquisition and any fragment ions above the m/z of the intact molecule were not counted. We tracked the number of fragments and their intensities across concentrations, collision cell energies, and sample types.
To estimate MA numbers, the instrument. RAW files were converted to. mzML format using ProteoWizard (Chambers et al., 2012) and processed in two ways: a fragment counting method from Marshall et al. (2021) hereafter referred to as “non-recursive” and the “recursive MA” algorithm from Jirasek et al. (2024) both discussed in further detail below.
Data processing methods published by Marshall et al. (2021), the “non-recursive processing,” count all fragments present below the isolated m/z being fragmented in the HCD cell. For example, leucine’s molecular ion is at 132 m/z, and when isolated using a ± 0.5 m/z window, any peak detected below 132 and above 50 m/z (instrument mass window cutoff) would be counted. Fragments are included or excluded based on noise floor and threshold cutoffs that can be defined in the code. This code does not consider the MS1 spectrum (i.e., full scan) or molecular weight as a factor when determining the MA number.
The non-recursive processing method creates an output file which contains the following parameters: parent mass, spectra id, possible isotopes, collision energy, number of spectra, threshold, noise floor, and MS2 values. Parent mass identifies the ion that was isolated and sent into the HCD cell and spectra id notes the scans that the parent mass was detected in. Possible isotopes indicate if any isotopic patterns were detected; if this is empty in the output file it means there were no possible isotopes detected. In the non-recursive processing script, an isotope is defined as the addition or loss of a hydrogen atom, commonly referred to as hydrogen adduct or deprotonation. The collision energy is the HCD cell energy used (instrument range is from 10–200 Normalized Collision Energy™, NCE), and the number of spectra is the total number of scans identified in the. mzML file. The threshold is the percentage of scans that a MS2 fragment is detected in, and the noise floor is an intensity cutoff which means peaks below that value are not included in the final peak count. Finally, the “MS2 values” are the number of MS2 fragments detected using the defined threshold and noise floor values (Marshall et al., 2021). used a noise floor value of 50,000 (peaks with intensities below this were not counted) and a 0.25 threshold (peak had to be present in ≥25% of scans) which we also follow here.
The recursive MA algorithm (Jirasek et al., 2024) can take multiple fragmentation levels into consideration when quantifying MA. Separate from the previous non-recursive processing, this algorithm takes MS1 data and molecular weight into consideration. Within the molecular weight considerations, the algorithm also determines if any heavy elements, like iron, for example, may be present. The algorithm first calculates a baseline estimate of MA using the molecular weight of the parent ion which is positively correlated with MA (Marshall et al., 2021). Parent ion molecular weight is determined from the m/z of the associated MS1 spectrum if it exists; otherwise, the algorithm creates a placeholder parent ion by extracting precursor m/z from the provided MS2 spectrum. Following MA estimation by molecular weight, hierarchical trees are constructed using the MS2 fragments (i.e., child peaks) to determine if there is evidence of any formation pathways with lower assembly index than estimated by molecular weight via the first two strategies. In the first strategy, the algorithm searches for masses of heavy elements, like chlorine or iodine, in the fragmentation spectra. If one is found, then a new estimated MA–MAc–is calculated for the remainder of the molecule excluding that heavy element and the overall MA is reported as MAc + 1, where the heavy atom is represented by a single bond formation in order to form the parent ion. For any atoms heavier than 14 Da, this new estimate will be lower and have a narrower range of uncertainty associated with the inherently lighter remaining fragment.
The recursive MA algorithm’s second strategy for optimizing MA estimates relies on fragmentation levels beyond MS2. Specifically, for each parent ion p in MSn−1, the algorithm searches for any fragment f and complement c = p–f in MSn that share a common granddaughter ion g in MSn+1. This allows the MA(p) estimate of MA(f) + MA(c) + 1 — that is f and c combined to form p—to be reduced to MA (f–g) + MA (c–g) + MA(g) + 3. Although the Q Exactive instrument used here only permits MS2 analyses, the algorithm can infer MS3 peaks by finding m/z triplets (m1, m2, m3) in MS2, where m1 = m2 + m3 suggesting that m2 and m3 could be child ions of m1. MA is estimated across all spectra and these values are combined to determine the MA of the original molecule. As with the non-recursive script, some additional filtering parameters can be modified by the user. We used the following parameters: 0.1% relative intensity threshold (peaks are removed if they are lower than 0.1% of the most intense peak), mass tolerance (i.e., how much the mass shifts across scans throughout the acquisition) of 0.001 Da, and minimum absolute intensity of at least 106 for MS1 and 104 for MS2 analyses. The output file contains the number of MS2 peaks (and MS3-MS5 if data is available), the parent m/z, lower, upper, and mean MA estimates, plus a final column with all MA estimates.
Analyte concentration impacts the abundance of ions that are observed in the mass spectra. Intensities are generally reported as relative abundances and at high concentrations, ions from analytes of interest are more abundant than ions from solvents or instrument backgrounds (hereafter referred to as “background ions”), and thus appear to be the main ions in the raw data. When concentrations of target analytes are low, background ion intensities better match those of the analyte and thus appear more abundant overall (even though they are always present). The low relative intensity of analyte ions at low concentrations makes them harder to resolve from instrument noise, solvent ions, or other non-analyte ions present in complex mixtures (i.e., sample matrix) which can lead to MA overestimation.
We tested four compounds (cytidine, leucine, methionine, and tryptophan) at ten concentrations (between ∼30 pg/μL and 1 μg/μL) across six collision energies (10, 35, 45, 50, 100, and 150 NCE) to determine concentration limits for MA estimation. We focus here on all concentrations at the lowest collision energy, 10 NCE, and address effects of collision energy in the following section.
The 20 most intense peaks vary by concentration (≥0.5% relative abundance), with lower concentrations having more non-analyte fragments. Most notably, higher concentration samples have less ions present above 0.5%, but those ions can all be ascribed to the target analyte. For example, leucine is known to produce one major fragment in high abundance which represents the loss of the carboxyl group (−COOH) resulting in an ion at 86 m/z. The molecular ion (132 m/z) is also detected but in low amounts (between 2.5% and 3.5% relative abundance). Leucine is also known to produce an ion at 69 m/z (loss of the carboxyl and amine groups) but abundances are too low at HCD 10 NCE (Zhang et al., 2019). For the highest concentration, the 132 and 86 m/z ions are the only peaks present at ≥ 0.5% relative abundance (Figure 3A). At the three lowest concentrations, non-leucine ions reach relative abundances of >1% (Figure 3B). At these concentrations (<0.25 ng/μL), presence of non-analyte ions could lead to inflation of MA estimates. Non-analyte ions are present in higher relative abundances at the three lowest concentrations for methionine and cytidine as well. Tryptophan ions remain the dominant ions (above 0.5%) at all concentrations, but eight of the 16 known ions for tryptophan (Zhang et al., 2019) are not detected at ≥ 0.5% relative abundance at any concentration. Given these results, we recommend analyzing target analytes at concentrations above 0.25 ng/μL. When concentrations are unknown (i.e., for untargeted analyses of natural mixtures) it is important to ensure that the most abundant ions have intensities of at least 108 prior to MS/MS analysis or when analyzed with low collision energy (i.e., 10 NCE). This will require an additional screening of the sample using full scan mode or a fragmentation analysis at 10 NCE.
Figure 3. The twenty most abundant ions were extracted from instrument. RAW files. Ions with relative intensities of >0.5% are plotted here for (A) the highest concentration (125 ng/μL) and (B) lowest concentration (0.03 ng/μL) of leucine. The dark colored symbols represent ions known to be leucine (Zhang et al., 2019) and the light-colored symbols represent non-leucine ions. At the highest concentration, these two ions (at 86 and 132 m/z) are the only ions detected above 0.5% relative abundance. At the lowest concentration (B), overall intensity is lower and background ions (e.g., non-analyte ions present in solvents) are present above 0.5% relative abundance. This trend holds for the three other compounds (tryptophan, methionine, and cytidine) analyzed at different concentrations.
If samples cannot be concentrated to reach recommended intensities, instrument settings can be changed to optimize data acquisition. Data from a given analyte is an average of all the mass analyzer scans within a given acquisition period. Here, we used a 1-min direct infusion for each acquisition. Within an Orbitrap mass spectrometer, ions are collected in the Curved Linear Trap (C-Trap, Figure 1) prior to being sent into the Orbitrap mass analyzer in packets (“scans”). The Automatic Gain Control (AGC) Target setting allows for a defined number of ions to be collected in the C-Trap before being sent into the Orbitrap mass analyzer. Collecting a constant number of ions helps to decrease space charge effects (repelling of ions with similar m/z that occurs when a large amount of similarly charged ions accumulate in a confined space) and leads to better sensitivity (Eliuk and Makarov, 2015). Properly defining the AGC Target helps to reduce overloading of the mass analyzer.
Separately, the amount of time it takes to accumulate these ions can be limited for a given measurement. At higher concentrations, a larger number of ions are “thrown away” (i.e., not analyzed by the mass analyzer) rather than being sent into the Orbitrap mass analyzer because the AGC Target is reached quickly, so injection times from the C-Trap into the Orbitrap are faster (<1 ms for the highest concentration sample). At lower concentrations, it takes a longer time to collect the necessary number of ions prior to injection into the mass analyzer (>50 ms for the lower concentration samples). For analyses presented here, maximum ion injection time (IT) was set to 50 ms and lower concentration samples (<0.25 ng/μL) reached this maximum at all HCD cell energies (meaning it took longer to collect the target number of ions in the C-Trap before sending a packet to the Orbitrap mass analyzer). Concentrations above 0.25 ng/μL only reached maximum IT at HCD cell energies of 100 and 150 NCE. The two highest concentration samples had IT <50 ms for all HCD cell energies. To not be limited by IT, higher concentrations or lower AGC Targets should be used, but when concentration cannot be controlled, increasing the maximum IT and lowering the AGC Target are recommended.
We tested increasing the maximum IT and varying the AGC Target for the lowest concentration of leucine (0.03 ng/μL) at HCD of 100 NCE (Table 1). As expected, this generally resulted in lower number of spectra collected than during a 1 min acquisition at higher AGC Targets (29 scans at 1,000,000) and IT ranged from 82.5 to 1,000 ms (i.e., reached the maximum). A lower AGC Target resulted in higher numbers of spectra for a 1 min acquisition (e.g., 431 scans at AGC of 20,000 at HCD cell energy of 100 NCE, the same as a 1 min acquisition of the highest concentration at AGC of 1,000,000). In general, changes to these instrument settings did not influence overall ion intensity but allowed for more scans to be averaged across during a given acquisition which increases confidence in the results. To decrease space charge effects and optimize the sample being analyzed, increasing the maximum IT and lowering AGC Target, especially for lower concentration samples, is recommended. Generally, analytes separated by liquid or gas chromatography have peak widths <1 min and will therefore have less scans overall. Lower AGC Targets and higher maximum IT will be necessary when online separation methods are used for MA analyses.
Table 1. Instrument settings and outputs for lowest concentration Leucine (0.03 ng/μL). As AGC Target decreases, IT time also decreases meaning the mass analyzer is not limited by the number of ions it needs to collect or the time it takes to collect that number. Changing the AGC Target and maximum IT time does not influence intensity (shown here for a fragment of Leucine with m/z of 86) but does increase the number of spectra returned for each acquisition.
Collision energy causes inconsistent changes to fragmentation patterns for the set of molecules analyzed here. There is an increase in the number of fragment peaks for some compounds at higher energies and a decrease in fragment numbers for other compounds (Figure 4). It is well-known that different collision energies change fragmentation for larger molecules (Thermo Fisher Product Support Bulletin 104), like peptides (e.g., Révész et al., 2023), and this is also demonstrated for individual amino acids (e.g., Zhang et al., 2019). Optimal collision energy generally scales with molecule size (i.e., molecules with higher molecular weights require higher energies) and charge state (increasing charge state requires lower energies). Here we assess the influence of collision energy on fragmentation patterns using the high-energy collision-induced dissociation (HCD) cell. There is a clear influence of fragmentation energy on the number and intensity of MS2 peaks, but this varies by compound. For most compounds, the relative abundance of analyte-specific MS2 peaks decreases with increasing collision energy (Figure 4). However, for some compounds (specifically methionine, phenylalanine, tryptophan, and valine) the relative abundance of some MS2 peaks increases with increasing collision energy (Figure 4). The 20 most abundant fragments at higher collision energies are also consistently of lower m/z than those at lower energies, suggesting that the higher energies can produce smaller fragments overall.
Figure 4. Relative abundance of ions at different high-energy collision-induced dissociation (HCD) cell energies for leucine (A) and tryptophan (B) analyzed in pure form. In panel (A), leucine ions are in lower abundance at the highest collision energy of 150 normalized collision energy™ (NCE). In panel (B), some tryptophan ions, 91.05, 115.05, and 117.05 m/z, all increase in abundance at 150 NCE. These differences highlight the importance of analyzing unknown molecules at different collision energies to accurately represent fragmentation patterns of molecules.
For MA analysis the choice of the right fragmentation energy is critical as a high number of small m/z fragments could lead to an inflated MA value and to a potential false positive signal (i.e., noting a molecule as more complex than it really is), however it is also crucial to capture the complexity of a given molecule accurately. Our data suggests that a collision energy of 150 NCE is generally too high as it results in more non-analyte peaks. However, collision energies of 50 and 100 NCE increase the relative abundance of some analyte-specific peaks, making them easier to resolve from background ions. For example, some ions from tryptophan increase in intensity at these energies (Figure 4). Generally, there is a tradeoff between a high fragmentation energy which generates a larger number of fragments and a low energy which may not generate enough fragment ions to accurately represent compound(s) in each sample. Additionally, if a low abundance sample is analyzed, a high collision energy can make some analyte ions detectable in higher abundance. To capture the full variability of an unknown analyte, measuring samples at a low (10 NCE), mid (50 NCE), and high (100 NCE) collision energy will capture the range. We suggest the use of 100 NCE instead of 150 NCE because a larger number of fragments known for individual analytes are not detected in high enough abundance at 150 NCE. We show results for both 100 and 150 NCE in Figures 5, 6 to demonstrate the impacts of these higher collision energies across sample types and the two data processing methods. For untargeted analysis, only peaks that are present for at least two of the three collision energies should be used for MA estimates to ensure they are from analytes of interest.
Figure 5. The spread and intensity of MS2 fragments for Leucine from all five sample types show a clear difference at three HCD cell energies: (A) 10, (B) 50, (C, D) 100, and (E, F) 150 NCE. There is a clear difference in the m/z and intensity of ions across sample types. Fragment intensities are one order of magnitude lower at 50 NCE and two orders of magnitude lower at 100 and 150 NCE relative to 10 NCE. Spirulina-hot water (hot water extracted and acid hydrolyzed) leucine is more abundant than Spirulina-hydrolysis (acid hydrolyzed only), suggesting that pretreatment steps like hot water extraction help increase the abundance of target ions. Except for two ions, the m/z of the 20 most abundant ions (above 0.5% relative abundance) are the same for both Spirulina samples. To represent the full extent of possible fragments, a low, middle, and high collision energies should be used. We suggest that 150 NCE is too high and recommend 10, 50, and 100 NCE. Additionally, sample processing methods like hot water extraction and acid hydrolysis can help remove some background ions and increase abundance of target molecules.
Figure 6. MA estimates for leucine from five sample types at three different HCD cell energies of 10, 50, 100, and 150 NCE using (A) the non-recursive processing script from Marshall et al. (2021) and the (B) recursive algorithm from Jirasek et al. (2024). Using the non-recursive script, the two higher collision energies result in a higher MA estimates relative to the lower two energies for all sample types except for Murchison. The higher estimates at the lower collision energies are likely related to the low concentration of analytes in the Murchison sample. MA estimates are consistent across sample types using the recursive algorithm primarily because this algorithm includes an estimate based on the MA and molecular weight correlation (Marshall et al., 2021).
Sample type (or matrix) also impacts which ions are most abundant in the mass spectra.
We compare amino acids from Spirulina biomass that was hot water extracted and acid hydrolyzed (Spirulina-hot water), Spirulina biomass that was acid hydrolyzed only (Spirulina-hydrolysis), and a Murchison meteorite extract that was hot water extracted and acid hydrolyzed (Murchison) with pure compounds and a standard mixture prepared from the pure compound solutions. All three natural mixtures (Murchison and Spirulina) had a higher number of MS2 peaks than samples in pure form or standard mixture (Figure 5). This amplification highlights increased background and matrix effects (i.e., presence of other compounds) for natural samples. The analysis of complex natural mixtures without separation makes detection of specific analytes difficult. Pretreatment steps, like hot water extraction and acid hydrolysis, can select for specific types of compounds (e.g., amino acids) making their analysis by direct infusion more straightforward. Spirulina and Murchison samples had more ions present overall, confirming that natural mixtures contain a larger number of different compounds. This causes fragmentation patterns to differ from those observed for pure compounds.
The amino acids from the Spirulina-hot water sample had higher intensities than the Spirulina-hydrolysis only sample, confirming that some pretreatment steps are important for increasing the relative abundance of target analytes. Additionally, the abundance of the 69 m/z fragment is much higher than noted for pure leucine. This is likely related to the fact that both leucine and isoleucine are present in natural mixtures and isoleucine is known to have higher relative amounts of the 69 m/z ion than leucine (Zhang et al., 2019). Since our analyses did not include chromatographic separation, both compounds are isolated together. Using chromatographic separation can help prevent this, but analyzing these two isomers together should not impact MA estimates.
The signal intensity for the Murchison meteorite extract was lower than for all other samples (86 m/z ion for leucine was 1.7 × 106 which is around the intensity measured for the lowest concentration leucine sample). A lower AGC Target (100,000) and flow rate (5 μL/min) was used for Murchison to compensate. Nonetheless, all Murchison analyses had more peaks present above 0.5% relative abundance and a larger number of non-analyte peaks than all other sample types which will inflate MA estimates (discussed in Section 3.5). Because the analyses were direct infusion (without chromatographic separation), more material was required overall. With chromatographic separation, samples could be concentrated to help overcome this issue, but the non-analyte peaks would also be concentrated. Thus, additional clean-up (using liquid-liquid and solid phase extractions) or purification steps (fraction collection by high pressure liquid chromatography) may be required for complex, low abundance samples like meteorites. Sample loss can occur at each step of the sample preparation pipeline; thus, it is essential to consider analytical goals when determining sample preparation steps.
The pure compounds (Figure 2) were analyzed on an LTQ-XL Orbitrap mass spectrometer (LTQ) which performs mass filtration using an ion trap like the prototype LAbCosmOrbitrap (Arevalo et al., 2018; Briois et al., 2016). Ion intensities for LTQ analyses were generally in the 105–106 range for the most abundant ions compared to 107–109 for the Q Exactive analyses and a 1 min direct infusion generally resulted in <100 scans (compared to >400 scans on the Q Exactive). At the two highest collision energies, 100 and 150 NCE, ions were between 101 and 103 and difficult to resolve from background signals. In general, not all known fragments were detected above 0.5% relative abundance for all compounds measured on the LTQ regardless of fragmentation energies. For example, the 69 m/z ion from leucine was not detected under any fragmentation energies on the LTQ, whereas it was detected above 0.5% relative abundance for HCD energies of 50, 100, and 150 NCE on the Q Exactive. The lack of detection of analyte-specific fragments would lead to an under-estimation of the MA number for a given molecule.
Accurate mass tandem mass spectrometry was historically a slow process using the first generation of Orbitrap mass spectrometers like the LTQ (Eliuk and Makarov, 2015). Improvements to signal processing (enhanced Fourier transform, Eliuk and Makarov, 2015) and filling the C-Trap with ions while the previous packet of ions is being analyzed in the mass analyzer, led to faster acquisition rates between the LTQ and Q Exactive (Eliuk and Makarov, 2015). These improvements led to increased sensitivity and the ability to detect low abundance ions which help to accurately represent molecules with newer Orbitrap instruments. If possible, including these capabilities on flight instruments will be beneficial for in situ MA estimation.
The MA estimates using the non-recursive processing method capture the variabilities seen in the raw mass spectrometry data. MA estimates are generally higher at higher collision energies (Figure 6A) because of the larger number of fragments observed. The natural mixtures had higher MA estimates than standard mixture and pure compounds (Figure 6A). The Murchison extract had the highest MA estimates and did not follow the trends of increasing MA with increasing collision energy that the other samples follow (Figure 6A). This is likely the result of the larger number and relative abundances of non-analyte ions compared to amino acid specific ions as discussed in Section 3.3. Overall, the non-recursive estimates are more sensitive to noise floor cutoffs, the trend does not appear linear, and estimates can vary between 1 and 7 for leucine depending on where that cutoff is defined (Supplementary Figure S1). For this reason, we caution against using the non-recursive data processing method and suggest the updated Recursive MA algorithm instead, discussed further below.
The Recursive MA algorithm (Jirasek et al., 2024) provides average MA estimates that do not appear to be influenced by instrument parameters in the same manner as the non-recursive script (Figure 6B). For the samples analyzed here the fragmentation data did not provide sufficient evidence for the algorithm to infer an improved MA estimate. Thus, the MA estimates from the Recursive MA algorithm do not capture the variation in concentration, fragmentation energy, and sample type seen for the raw mass spectrometry data or the non-recursive processing script. Using additional levels of fragmentation (for example, on an instrument that fragments molecules using an ion trap like the Thermo Orbitrap Fusion™ Lumos™ Tribrid™ mass spectrometer) may help to refine MA estimates. Parameters like relative intensity threshold and minimum absolute intensities for different MSn levels can be changed to accommodate differences in concentration - particularly relevant for lower concentration samples. However, without modifying the intensity parameters for the concentration series analyzed here, MA estimates remained the same across all concentrations (within ± 0.14, Supplementary Figure S2) because of the molecular weight anchor. Processing mass spectra for MA estimates using the Recursive MA algorithm is preferred because considers molecular weight as a factor when estimating MA.
The methods discussed here are well-suited for analyzing samples on Earth or samples returned to Earth like those from the asteroids Ryugu (Yada et al., 2022) and Bennu (Lauretta et al., 2017). However, Orbitrap mass spectrometers are in development for space flight (Arevalo et al., 2018; Briois et al., 2016; Selliez et al., 2023), and in situ measurements of MA may be possible in the near future.
Flight prototype instruments, like the LAb CosmOrbitrap, do not currently contain collision cells. Including this component would not only improve compound identification capabilities but would enable in situ estimations of MA. Electrospray ionization is also in development for flight (Ulibarri et al., 2024), and combination of electrospray ionization with prototype Orbitrap instruments would enable direct testing of the methods discussed here. Concentration impacts the abundance of target ions and the ability to resolve these from other interfering ions (i.e., those from other analytes in the sample mixture or instrument background ions). The ability to control the target number of ions and the collection time for each packet being analyzed by the mass analyzer, such as AGC Target and IT controls on the Q Exactive, are useful components for flight instruments that may encounter low abundances of target molecules during in situ sampling.
Electrospray ionization commonly employs polar solvents to ionize polar molecules in liquid form Kiontke et al. (2016) and is generally more suited for larger molecular weight molecules. However, individual amino acids ionize well using electrospray ionization (as shown here) and some work suggests that nonpolar solvents and ionization of solid substrates by electrospray ionization is possible (e.g., Hu and Yao, 2018; Huang et al., 2019) which broadens the potential analytes that can be targeted using this technique. Testing of nonpolar solvents and solid substrates for MA estimation would extend the analyzable chemical space to accommodate unknown environments more fully.
Testing MA estimation across a larger range of natural mixtures in a targeted manner will provide information about how different sample types contribute peaks to the mass spectrum. Including peaks that come from the sample matrix may not be prohibitive for MA, but their impact needs to be better understood. This can be achieved by identifying compounds in natural mixtures and monitoring the fragmentation spectra to determine if any changes to the spectra occur across different sample types. Currently, without the capability for sample workup, like vapor phase acid hydrolysis, separation (chemical and/or chromatographic) will be required for in situ measurements to alleviate impacts of non-analyte (i.e., matrix) ions when certain compound classes (like amino acids) are the focus of MA estimates. This is not prohibitive for sample return, but important for in situ analyses. Using chromatographic separation will decrease the influence of matrix ions for analysis of natural samples. Chromatographic separation like gas chromatography (GC) has been used for flight instruments since the Viking mission in 1976 (see Chou et al., 2021 for a review of the history of space flight mass spectrometry) and exploring the correlation between MA and fragmentation using GC-MS and GC-FT-MS is a useful next step in determining feasibility of making MA approximations in space. The electron impact ionization used for GC-MS is a stronger form of ionization than electrospray ionization, can break covalent bonds which can lead to recombination. For this reason, MS1 analysis using electron impact ionization resembles MS2 by electrospray ionization, underlining the need for a new analytical pipeline and calibration. The Mars Organic Molecule Analyzer (MOMA, Goesman et al., 2017) and Dragonfly Mass Spectrometer (DraMS, Trainer er al., 2021) both in development for flight have GC capabilities and could be used to estimate MA in situ. Derivatization is required for many compounds to be analyzed by GC which would inflate MA estimates and thus require correction (i.e., as with isotope ratio analysis, see Silverman et al. (2022) for a description of how this is handled for amino acids).
In this work we have defined a concentration target of above 0.25 ng/μL or signal intensity of 108 for MA analyses by electrospray ionization Fourier transform mass spectrometry. Analyzing samples at three collision energies and counting only peaks that are present for at least two energies will capture variability of a given molecule since some fragments require higher energies and others require lower energies. Natural mixtures have lower abundances of analytes of interest and larger amounts of non-analyte peaks leading to potential false positives for MA estimation. Sample clean up steps and chromatography will help alleviate some of this and more targeted analysis of mixtures is required. Data processing methods impact MA estimates thus considering molecular weight and including a recursive model to connect fragments to parent molecules is essential.
MA is a promising method for agnostic biosignature detection, and this study provides further understanding of the effects of specific instrument settings on values generated. This study shows that future flight hardware using this method would benefit from collision cells or other types of ionization as well as methods that control target ion accumulation time and amounts, plus simultaneous collection of ion packets while preceding packets are analyzed in the mass analyzer. Our analysis shows that the recursive MA algorithm provides more stable estimates which is to be expected since this algorithm most precisely mirrors the theoretical approach to generating assembly spaces and calculating the assembly index. Future testing should qualify the effects of electrospray ionization using non-polar solvents and solid substrates. Chromatographic separation may also be necessary for future flight applications hoping to use MA estimates to explore chemical complexity on other worlds. With carefully adjusted methods and a larger calibration for natural mixtures, MA could help facilitate the search for life for future space flight missions to unknown environments.
The original contributions presented in the study are publicly available through Zenodo. This data can be found here: https://doi.org/10.5281/zenodo.14224720.
GW: Conceptualization, Data curation, Formal Analysis, Investigation, Methodology, Project administration, Visualization, Writing–original draft. SA: Writing–review and editing. HM: Formal Analysis, Writing–review and editing. AC: Formal Analysis, Writing–review and editing. SM: Software, Writing–review and editing. LC: Writing–review and editing. HG: Conceptualization, Funding acquisition, Project administration, Resources, Supervision, Writing–review and editing.
The author(s) declare that financial support was received for the research, authorship, and/or publication of this article. The material is based upon work supported by NASA under award numbers 80GSFC21M0002 and 80NSSC18K1140. SA was supported by the NASA Postdoctoral Program at GSFC. Support for this research was also provided by NASA’s Planetary Science Division Research Program, through the Fundamental Laboratory Research ISFM work package at NASA Goddard Space Flight Center.
We thank Eric Parker and the Astrochemistry Analytical Laboratory at GSFC for time on the Q Exactive Orbitrap mass spectrometer used for these analyses and for the instrument schematic (Figure 1). We also thank Frédéric Seguin for helpful discussions of the dataset.
The authors declare that the research was conducted in the absence of any commercial or financial relationships that could be construed as a potential conflict of interest.
All claims expressed in this article are solely those of the authors and do not necessarily represent those of their affiliated organizations, or those of the publisher, the editors and the reviewers. Any product that may be evaluated in this article, or claim that may be made by its manufacturer, is not guaranteed or endorsed by the publisher.
The Supplementary Material for this article can be found online at: https://www.frontiersin.org/articles/10.3389/fspas.2024.1485483/full#supplementary-material
Arevalo, R., Selliez, L., Briois, C., Carrasco, N., Thirkell, L., Cherville, B., et al. (2018). An Orbitrap-based laser desorption/ablation mass spectrometer designed for spaceflight. Rapid Commun. Mass Spectrom. 32, 1875–1886. doi:10.1002/rcm.8244
Briois, C., Thissen, R., Thirkell, L., Aradj, K., Bouabdellah, A., Boukrara, A., et al. (2016). Orbitrap mass analyser for in situ characterisation of planetary environments: performance evaluation of a laboratory prototype. Planet. Space Sci. 131, 33–45. doi:10.1016/j.pss.2016.06.012
Chambers, M. C., Maclean, B., Burke, R., Amodei, D., Ruderman, D. L., Neumann, S., et al. (2012). A cross-platform toolkit for mass spectrometry and proteomics. Nat. Biotechnol. 30, 918–920. doi:10.1038/nbt.2377
Chan, M. A., Hinman, N. W., Potter-McIntyre, S. L., Schubert, K. E., Gillams, R. J., Awramik, S. M., et al. (2019). Deciphering biosignatures in planetary contexts. Astrobiology 19, 1075–1102. doi:10.1089/ast.2018.1903
Chou, L., Mahaffy, P., Trainer, M., Eigenbrode, J., Arevalo, R., Brinckerhoff, W., et al. (2021). Planetary mass spectrometry for agnostic life detection in the solar system. Front. Astron. Space Sci. 8, 755100. doi:10.3389/fspas.2021.755100
Eliuk, S., and Makarov, A. (2015). Evolution of Orbitrap mass spectrometry instrumentation. Annu. Rev. Anal. Chem. 8, 61–80. doi:10.1146/annurev-anchem-071114-040325
Elsila, J. E., Johnson, N. M., Glavin, D. P., Aponte, J. C., and Dworkin, J. P. (2021). Amino acid abundances and compositions in iron and stony-iron meteorites. Meteorit. Planet. Sci. 56, 586–600. doi:10.1111/maps.13638
Goesmann, F., Brinckerhoff, W. B., Raulin, F., Goetz, W., Danell, R. M., Getty, S. A., et al. (2017). The mars organic molecule analyzer (MOMA) instrument: characterization of organic material in martian sediments. Astrobiology 17, 655–685. doi:10.1089/ast.2016.1551
Hazen, R. M., Griffin, P. L., Carothers, J. M., and Szostak, J. W. (2007). Functional information and the emergence of biocomplexity. Proc. Natl. Acad. Sci. 104, 8574–8581. doi:10.1073/pnas.0701744104
Hecht, E. S., Scigelova, M., Eliuk, S., and Makarov, A. (2019). “Fundamentals and advances of Orbitrap mass spectrometry,” in Encyclopedia of analytical chemistry (John Wiley and Sons, Ltd), 1–40. doi:10.1002/9780470027318.a9309.pub2
Hu, B., and Yao, Z.-P. (2018). Detection of native proteins using solid-substrate electrospray ionization mass spectrometry with nonpolar solvents. Anal. Chim. Acta 1004, 51–57. doi:10.1016/j.aca.2017.11.079
Huang, Z., Yao, Y.-N., Li, W., and Hu, B. (2019). Analytical properties of electrospray ionization mass spectrometry with solid substrates and nonpolar solvents. Anal. Chim. Acta 1050, 105–112. doi:10.1016/j.aca.2018.10.064
Jirasek, M., Sharma, A., Bame, J. R., Mehr, S. H. M., Bell, N., Marshall, S. M., et al. (2024). Investigating and quantifying molecular complexity using assembly theory and spectroscopy. ACS Cent. Sci. 10, 1054–1064. doi:10.1021/acscentsci.4c00120
Kiontke, A., Oliveira-Birkmeier, A., Opitz, A., and Birkemeyer, C. (2016). Electrospray ionization efficiency is dependent on different molecular descriptors with respect to solvent pH and instrumental configuration. PLoS One 11, e0167502. doi:10.1371/journal.pone.0167502
Lauretta, D. S., Balram-Knutson, S. S., Beshore, E., Boynton, W. V., Drouet d’Aubigny, C., DellaGiustina, D. N., et al. (2017). OSIRIS-REx: sample return from asteroid (101955) Bennu. Space Sci. Rev. 212, 925–984. doi:10.1007/s11214-017-0405-1
Liu, R., Ye, Y., Qiang, L., Liao, X., and Zhao, Y. (2008). The fragmentation pathway of the nucleosides under the electrospray ionization multi-stage mass spectrometry. Life Sci. J. 5.
Mahaffy, P. R., Webster, C. R., Cabane, M., Conrad, P. G., Coll, P., Atreya, S. K., et al. (2012). The sample analysis at mars investigation and instrument suite. Space Sci. Rev. 170, 401–478. doi:10.1007/s11214-012-9879-z
Marshall, S. M., Mathis, C., Carrick, E., Keenan, G., Cooper, G. J. T., Graham, H., et al. (2021). Identifying molecules as biosignatures with assembly theory and mass spectrometry. Nat. Commun. 12, 3033. doi:10.1038/s41467-021-23258-x
Marshall, S. M., Murray, A. R. G., and Cronin, L. (2017). A probabilistic framework for identifying biosignatures using Pathway Complexity. Philos. Trans. R. Soc. Math. Phys. Eng. Sci. 375, 20160342. doi:10.1098/rsta.2016.0342
Révész, Á., Hevér, H., Steckel, A., Schlosser, G., Szabó, D., Vékey, K., et al. (2023). Collision energies: optimization strategies for bottom-up proteomics. Mass Spectrom. Rev. 42, 1261–1299. doi:10.1002/mas.21763
Rouvray, D. H., and Bonchev, D. (2003). Complexity in chemistry: introduction and fundamentals, Mathematical chemistry (London; New York: Taylor and Francis).
Schwieterman, E. W., Kiang, N. Y., Parenteau, M. N., Harman, C. E., DasSarma, S., Fisher, T. M., et al. (2018). Exoplanet biosignatures: a review of remotely detectable signs of life. Astrobiology 18, 663–708. doi:10.1089/ast.2017.1729
Selliez, L., Briois, C., Carrasco, N., Thirkell, L., Gaubicher, B., Lebreton, J.-P., et al. (2023). Analytical performances of the LAb-CosmOrbitrap mass spectrometer for astrobiology. Planet. Space Sci. 225, 105607. doi:10.1016/j.pss.2022.105607
Sharma, A., Czégel, D., Lachmann, M., Kempes, C. P., Walker, S. I., and Cronin, L. (2023). Assembly theory explains and quantifies selection and evolution. Nature 622, 321–328. doi:10.1038/s41586-023-06600-9
Silverman, S. N., Phillips, A. A., Weiss, G. M., Wilkes, E. B., Eiler, J. M., and Sessions, A. L. (2022). Practical considerations for amino acid isotope analysis. Org. Geochem. 164, 104345. doi:10.1016/j.orggeochem.2021.104345
Thermo Fisher Scientific (2024). Product Support Bulletin 104 Normalized collision energy technology. Available at: https://tools.thermofisher.com/content/sfs/brochures/PSB104-Normalized-Collision-Energy-Technology-EN.pdf (Access January 8, 2024).
Trainer, M. G., Brinckerhoff, W. B., Grubisic, A., Danell, R. M., Kaplan, D., van Ameron, F. H. W., et al. (2021). “Development of the dragonfly mass spectrometer (DraMS) for titan,” in Presented at the lunar and planetary science conference.
Ulibarri, Z., Petro, E., Bell, S., Hofheins, G., and Hofmann, A. E. (2024). “Initial studies with a vacuum electrospray ionization mass spectrometer,” in Presented at the astrobiology science conference 2024. Washington, DC: AGU.
Yada, T., Abe, M., Okada, T., Nakato, A., Yogata, K., Miyazaki, A., et al. (2022). Preliminary analysis of the Hayabusa2 samples returned from C-type asteroid Ryugu. Nat. Astron. 6, 214–220. doi:10.1038/s41550-021-01550-6
Zhang, P., Chan, W., Ang, I. L., Wei, R., Lam, M. M. T., Lei, K. M. K., et al. (2019). Revisiting fragmentation reactions of protonated α-amino acids by high-resolution electrospray ionization tandem mass spectrometry with collision-induced dissociation. Sci. Rep. 9, 6453. doi:10.1038/s41598-019-42777-8
Keywords: assembly theory, molecular assembly, biosignatures, orbitrap mass spectrometry, electrospray ionization, amino acids, nucleosides
Citation: Weiss GM, Asche S, McLain H, Chung AH, Mehr SHM, Cronin L and Graham HV (2024) Operational considerations for approximating molecular assembly by Fourier transform mass spectrometry. Front. Astron. Space Sci. 11:1485483. doi: 10.3389/fspas.2024.1485483
Received: 23 August 2024; Accepted: 29 October 2024;
Published: 27 November 2024.
Edited by:
Andrea Longobardo, National Institute of Astrophysics (INAF), ItalyReviewed by:
Jan Hendrik Bredehöft, University of Bremen, GermanyCopyright © 2024 Weiss, Asche, McLain, Chung, Mehr, Cronin and Graham. This is an open-access article distributed under the terms of the Creative Commons Attribution License (CC BY). The use, distribution or reproduction in other forums is permitted, provided the original author(s) and the copyright owner(s) are credited and that the original publication in this journal is cited, in accordance with accepted academic practice. No use, distribution or reproduction is permitted which does not comply with these terms.
*Correspondence: Gabriella M. Weiss, Z2FicmllbGxhLndlaXNzQG5hc2EuZ292; Heather V. Graham, aGVhdGhlci52LmdyYWhhbUBuYXNhLmdvdg==
Disclaimer: All claims expressed in this article are solely those of the authors and do not necessarily represent those of their affiliated organizations, or those of the publisher, the editors and the reviewers. Any product that may be evaluated in this article or claim that may be made by its manufacturer is not guaranteed or endorsed by the publisher.
Research integrity at Frontiers
Learn more about the work of our research integrity team to safeguard the quality of each article we publish.