- 1State Key Laboratory of Resources and Environmental Information System, Institute of Geographic Sciences and Natural Resources Research, Chinese Academy of Sciences, Beijing, China
- 2University of Chinese Academy of Sciences, Beijing, China
- 3Jiangsu Center for Collaborative Innovation in Geographical Information Resource Development and Application, Nanjing, China
- 4Chinese Academy of Sciences, Center for Excellence in Comparative Planetology, Hefei, China
The study of Martian surface topography is important for understanding the geological evolution of Mars and revealing the spatial differentiation of the Martian landscape. Identifying typical landform units is a fundamental task when studying the origin and evolution of Mars and provides important information for landing on and exploring Mars, as well as estimating the age of the Martian surface and inferring the evolution of the Earth’s environment. In this paper, we first investigate Mars exploration, data acquisition and mapping, and the classification methods of Martian landforms. Then, the identification of several typical Martian landform types, such as aeolian landforms, fluvial landforms, and impact landforms, is shown in detail. Finally, the prospects of Mars data acquisition, landform mapping, and the construction and identification of the Martian landform classification system are presented. The construction of the Martian landform classification system and the identification of typical Martian landforms using deep learning are important development directions in planetary science.
1 Introduction
Mars is the most Earth-like planet in the Solar System (Ouyang and Xiao, 2011). Following studies of the Moon, Mars is a frontier area for space agency competition. As the planet that is closest to the Earth in the Solar System, Mars has attracted the attention of the United States, the European Union, India, and the former Soviet Union. Mars exploration began in October 1960, and vehicles that have successfully reached Mars to carry out missions include Mariner 9, the Viking series, the Mars Global Surveyor, Mars Odyssey, Mars Express, and the Mars Reconnaissance Orbiter. With the successful return of data from China’s “Tianwen-1” probe, new research on Mars is well underway. Mars exploration is the first and crucial step from lunar exploration to planetary exploration. The study of the evolution of Mars is helpful for further exploration of possible life on Mars. Moreover, it has an important role in promoting evolutionary simulations and future climate predictions of the Earth.
The surface environment of Mars resembles those of both the Earth and the Moon. Mars has a lunar-like impact landform and volcanic landforms. However, the current state of the Martian landscape is more complex than that of the moon. Mars has sand dunes, gullies, alluvial fans and canyons, which have a similar landscape to that of Earth. Mars has a thin atmosphere and four seasons. Thus, Mars can be considered a composite version of the Moon and the Earth, which is a combination of many types of landforms. Explorations of the geological evolution of Mars and the conditions needed for the existence of life have attracted the attention of many researchers. Scientists have begun to search for areas on Earth that are similar to the Martian landscape to simulate and study the Martian environment. Mars-like regions are called “Mars analogs”, such as the Antarctic Dry Valleys, the Atacama Desert in Chile, the Mars Desert Experiment Station in Utah, and the Tarim and Qaidam Basins in China (Xiao et al., 2017; Gou et al., 2018). Mars has many similarities to Earth; therefore, the study of the morphological characteristics of the Martian landscape has implications for the study of future evolutionary processes on Earth.
Martian landforms result from a combination of internal and external forces, revealing important geological events on Mars. Martian landforms reflect the highs and lows on the Martian surface and record the global distribution of water, heat and atmospheric motion on Mars. Currently, although many countries and institutions have launched probes to Mars, it remains impossible to bring back Martian samples to be studied, such as through dating and analysis (Yue et al., 2022). Therefore, it is important for Martian geomorphology research to focus on the surface topographic features of Mars based on remote sensing. With the continuous acquisition of higher-resolution and higher-accuracy Mars data, the quantification of Martian surface topography is becoming increasingly urgent. The quantitative analysis of Mars topographic features can provide a research basis for the exploration of Mars, landing on Mars, and the exploitation of Martian resources. Martian geomorphology research deepens the understanding of geomorphology research on Earth and is valuable as an important reference for estimating the age of the Martian surface, selecting of rover landing areas, and the assessing the evolution of the Martian geological landscape. However, there are few review studies of Martian surface topography. In this paper, we reviewed Mars exploration, data acquisition and mapping, as well as Mars geomorphological classification and identification methods, to reveal the characteristics of Martian topography. Additionally, the current research status of typical geomorphic unit identification was explored, and a reference and basis for both Mars science and related fields was provided.
2 Progress in Mars data acquisition and mapping
Humans have been exploring Mars since the 1960s, and the launched probes are shown in Table 1. Between 1960 and 2022, more than 40 probe missions were conducted, and a large amount of scientific data was obtained (Liu et al., 2006). Prior to the 1990s, Mars exploration projects mainly ended in failure. However, following the 1990s, the success rate of Mars exploration projects increased significantly and the requirements for exploration missions became increasingly high, evolving from flybys to orbits to landing and rover missions (Di et al., 2018). “Tianwen-1” was the first Mars probe launched in China, and “orbiting, landing and patrolling” were completed simultaneously (Li et al., 2015), which was historically significant.
Exploring the geomorphology, material composition, and atmospheric activity of Mars was among the main reasons to launch the probe. The successful launch of the rover has enabled the human understanding of Mars to evolve from “long-range exploration” (astronomical telescopes) to “close-range exploration” (orbiters, Mars rovers, etc.). The image data for Mars include both visible and thermal infrared images as well as topographic data. Currently, the above data are available for download on the internet at various spatial resolutions, as shown in Table 2.
NASA’s Mars Survey/Mapping Working Group (MGCWG) defines the Mars coordinate system in polar and right-handed systems with specific parameters (Seidelmann, 2002). The stellar solid coordinate system (Di et al., 2021) used for Mars positioning and mapping includes the stellar solid Cartesian coordinate system (Vaucouleurs et al., 1973). The stellar solid geodetic coordinate system (Duxbury et al., 2002; Archinal et al., 2011), with an origin and a base plane, is similar to that of the Earth. Kim and Muller, 2008 developed a processing workflow by combining digital terrain models (DTMs) of 0.5–4 m networks extracted from the High-Resolution Imaging Science Experiment (HiRISE) and DTMs of 12–18 m grids extracted from stereo image pairs. Yan et al. (2022) produced a topographic dataset of the “Tianwen-1” landing area based on the HiRIC stereo photogrammetry processing scheme, with a ground sampling distance of 0.7 m, a digital orthophoto map (DOM) resolution of 3.5 m, and a digital elevation model (DEM) resolution of 3.5 m. Lakdawalla (2005) used Mars Orbiter Laser Altimeter (MOLA) data to produce a global topographic map of Mars.
Mars topographic mapping is the most direct means of studying Martian landforms, which provides a solid foundation for the selection of the landing area of Mars exploration projects and the study of the geological evolution of Mars. Currently, the accepted method of Mars mapping is to divide Mars into 30 panels (MC1-30), whose latitude and longitude are shown in Table 3. The USGS published a geologic map of Mars by Tanaka et al. (2014), which is geologically zoned to map Mars in a geologic age combining geomorphic unit code, which contains 44 geologic units at a scale of 1:20,000,000. In terms of a single type of landform mapping, Piqueux et al. (2019) mapped the location of near-surface ice across Mars based on the location of ice found under thin sandy soils. Liu et al. (2020) mapped the global distribution of centroids of Martian yardangs based on multisource Mars remote sensing data and provided a presumed time of yardang landform formation. Zhao (2017) mapped different types of paleolakes in the southern highlands of Mars, including open-system paleolakes. Dong and his team (Dong, 2020a) created the world’s first aeolian geomorphologic map of Mars, classifying wind erosion and wind accretion landforms and filling the gap in the thematic map of Mars wind and sand. Carr (2006), Alemanno et al. (2018), Hynek et al. (2010) and Luo and Stepinski (2009) mapped the distribution of the global valley networks on Mars and formed a vector database, among which Luo and Stepinski (2009) also calculated the volume, length and other attributes of valley networks.
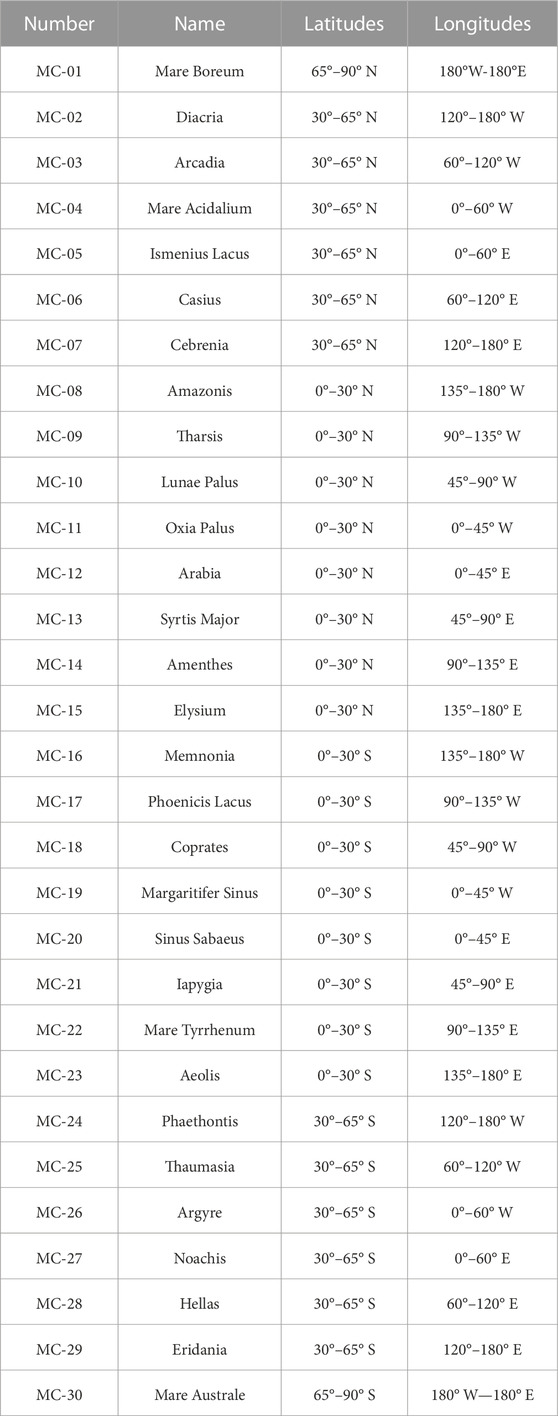
TABLE 3. Mars Quadrangles (modified from https://marspedia.org/Mars_Quadrangles).
3 Research progress on the classification of Martian landform types
3.1 Macroscopic analysis of the surface topographic features of Mars
The north‒south dichotomy is the most obvious geomorphic feature of Mars. The Southern Hemisphere is highly topographically variable with complex landform types, and includes impact craters, highlands, canyons, dry rivers, sand dunes, yardangs, volcanoes, and other landform types of various sizes. There are three clearly visible impact basins on Mars: the Argyre and Hellas basins in the south and the Isidis basin near the equator. Figure 1 shows the global topography of Mars. On the other hand, the Northern Hemisphere is dominated by low-relief plains. The main northern plain consists of Vastitas Borealis. The Tharsis bulge is near the equator, and its northern edge includes three volcanic regions: Olympus Mons, Alba Mons, and Tempe Terra. The presence of liquid water on Mars has been a mystery pursued by scientists. Orosei et al. (2018), through the Mars Express orbiter mission, found a 20-km-wide body of liquid water at a depth of 1.5 km below the ice cap.
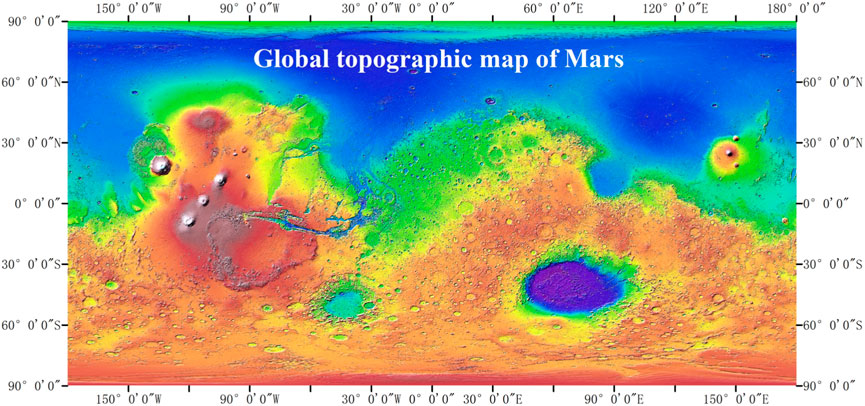
FIGURE 1. Global topographic map of Mars (MOLA DEM; Resolution: 463 m per pixel.) (https://astrogeology.usgs.gov/search/map/Mars/GlobalSurveyor/MOLA/Mars_MGS_MOLA_ClrShade_merge_global_463m).
3.2 The basis of Martian landform classification
The landform types mentioned above have been influenced by endogenic and exogenic forces. The geological lifetime of Mars ended when the magma activity in the interior of Mars ceased. Subsequently, the external magnetic field of Mars disappeared with the disappearance of nuclear convection. The disappearance of the magnetic field left Mars completely exposed to solar wind and cosmic high-energy rays. The combination of endogenic forces, such as early volcanic eruptions and tectonic movements, and exogenic forces, including meteorite impacts, wind accumulation and erosion, and water scouring, have resulted in the formation of a variety of landforms.
The common landforms included impact craters, volcanic landforms, glacial landforms, valley networks, and sand dunes on the surface of Mars and Earth (Wang, 2018). Typical flowing water features, such as alluvial fans and canyons, were preserved on the Martian surface, indicating the possibility of liquid water on Mars. Atmospheric movement and the high diurnal temperature differences have contributed to the formation of typical aeolian landforms, such as sand dunes and yardangs. The University of Western Ontario (Canada) initiated the Interactive Mapping of Mars (https://imars.uwo.ca/tutorial/) project, which classified Martian landforms into aeolian, water, glacial/periglacial, impact cratering, mass movement and volcanic landforms. Di et al. (2021) classified the landforms according to the causes of formation, including aeolian landforms, fluvial landforms, and tectonic landforms (referring to impact geomorphology and volcanic geomorphology). The above macroscopic landform classifications were further subdivided based on morphology and formation. OuYang and Zou, 2015 proposed classifying Martian landforms into aeolian, fluvial, canyon, impact, volcanic, and glacial landforms. This classification, combined with the traditional method of Martian landform classification, was used in this paper according to the relevant formation mechanisms, as shown in Figure 2.
In addition, the geomorphological classification systems of the Earth and Moon can aid in the geomorphological classification of Mars. The three-level and nine-class classification system of Earth (Institute of Geography Chinese Academy of Sciences, 1987; Geomorphic Map Editorial Committee of the People’s Republicof China, 2009; Zhou et al., 2009) includes the geomorphological category, geomorphological class, geomorphological shape (three levels), and macromorphological type subclass, land elevation and seafloor bathymetry subclass, maincrop force type subclass, maincrop force mode of action subclass, material composition and lithology subclass, geomorphic age subclass, combined morphological subclass, micromorphological subclass, and slope morphological subclass (nine classes). The three-level and eight-class classification system of the Moon (Cheng et al., 2018; Liu et al., 2022) does not include the maincrop force mode of action compared with the three-level and nine-class classification systems of Earth.
3.3 Method of classifying Martian landforms
In terms of overall classification, Martian landform classification methods can be divided into two categories.
One category is mainly based on surface elevation, relief, slope and other features, which can be used to parameterize the surface morphology or extract surface features to classify landforms with clustering or machine learning methods. Bue and Stepinski, 2007 classified Martian landforms into highlands, impact craters, lowlands, high-relief landforms, and channels based on six topographic parameters (elevation, flood, slope, flooded slope, contributing area, and flooded contributing area), using a self-organizing mapping method and Ward clustering. However, a limitation was misclassification for certain features, such as craters. Wang et al. (2017) classified lunar landforms into high-relief, highlands, lowlands, impact craters and other landform types. Comparably, Wang et al. (2017) added relief to topographic parameters and replaced Ward clustering with ISO clustering to achieve an overall accuracy of 83.34% and a kappa coefficient of up to 0.77, despite certain limitations influenced by aggradation, degradation, and complex landform types.
Additionally, with the rapid development of machine learning, deep learning and other methods to segment the content of images at the pixel level, image segmentation has been used in classification tasks. The related steps include creating training sets, designing network model structures, and adjusting parameters, among others, for the binary/multiclass segmentation of landform elements. Shang and Barnes, 2013 used the fuzzy rough feature selection method combined with a support vector machine (SVM) to classify landform types in a single Mars image, and the comparative experimental results showed that the method outperformed decision trees and K-nearest neighbor classification. Jiang et al. (2021) developed a new end-to-end deep learning framework, rotated SSD, to localize and identify different Martian landforms at the same time by using a rotatable-based anchor box mechanism and introducing unsupervised training based on autoencoders. Barrett et al. (2022) used HiRISE images of Oxia Planum and Mawrth Vallis and developed a deep learning terrain classification system (NOAH-H) based on semantic segmentation with deep neural networks. Wright et al. (2022) used NOAH-H to classify four HiRISE images of the terrain near the 2020 Perseverance Rover landing site at Jezero based on impact crater images, and the classification results agreed with the manually created geological maps. Rothrock et al. (2016) developed an algorithm (SPOC) based on deep convolutional neural networks (CNNs) that can identify terrain elements, such as sand, bedrock, wrinkled ridges, and steep slopes, and the algorithm was successfully applied for terrain identification in the Mars 2020 rover landing zone and MSL mission sliding prediction. Wang et al. (2021) designed a new framework for Mars rover image classification with semisupervised contrast learning; it ignores intraclass pairs in labeled data and counterexample pairs in unlabeled data, thus substantially improving the classification model.
With the rapid development of computer vision, image segmentation, deep learning and other fields, Martian landform classification research has also been rapidly developed, and an increasing number of types of landform elements can now be recognized, thus providing the basis for research on landform recognition and the evolution pattern of Mars landings.
4 Progress in the identification of typical landform types on Mars
4.1 Aeolian landforms
Aeolian landforms are the most prevalent and active geomorphic features on Mars, and the ancient Chinese name for Mars “Yinghuo,” is in part due to the sand phenomenon that causes Mars to be bright and pale (Dong et al., 2020b). Li et al. (2022) developed a similar visual degradation process based on the remote sensing images of “Tianwen-1” to synthesize real dust images and used these real dust images to train a deep learning model to identify dust-free images, inspired by the fog formation process on Earth. Yao, 2021 identified 882 dust storms with a diameter of 4,000 km in the southern part of Utopia based on Tianwen-1 landing area image data and analyzed their causes and distribution characteristics.
Sand dunes are the most typical manifestation of aeolian landforms. The methods for identifying sand dunes on Mars can be classified into four types.
1) Visual observation: Hayward et al. (2007) produced a database of medium and large sand dunes with areas larger than 1 km2, covering 550 dunes between 65°N and 65°S; however, many dunes are still not included in the database. “Visual observation” could obtain sand dunes intuitively and qualitative information, while it is inefficient for analyzing large-scale datasets.
2) Extracting gradient and grayscale statistical features: Carrera et al. (2019) used gradient and statistical features to structure a probabilistic classifier based on the R-vine distribution to identify Martian dunes and compared it with a centralized advanced classification algorithm, and superior results were obtained. “Extracting gradient and grayscale statistical features” can efficiently provide quantitative data related to texture and shape efficiently. Moreover, it could be used as input features for machine learning models. However, it is sensitive to variations in lighting and image quality and may miss complex patterns.
3) Machine learning and deep learning: Rubanenko et al. (2021) used Mask R-CNN based on the MRO’s background camera (CTX) for the automatic extraction of Martian dunes and then mapped the distribution of the full Martian dune field, discovering that dunes were more abundant in the Northern Hemisphere than in the Southern Hemisphere, which might be attributed to latitudinally dependent wind regimes, sediment supply, or sediment availability. “Machine learning and deep learning” can be used to process large-scale image analysis and reduce human subjectivity. Nevertheless, it requires substantial training data and depends on the data quality.
4) Combining machine learning with feature extraction: Bandeira et al. (2010) extracted gradient and grayscale histogram features from images aggregated into larger areas, which constituted detection units, and combined boosting and SVM classifiers to extract Martian surface dunes with a 98.7% recognition rate. This method combines the strengths of both approaches, while it requires expert knowledge in both feature extraction and machine/deep learning. This high complexity makes it difficult to interpret subsequent results.
Machine/deep learning requires a complete training dataset. The diverse shapes (Li, 2018) and sizes (Mandt and LeoneYardang, 2015) of yardang landforms make it difficult to establish a complete training dataset or a unified identification standard. Therefore, the identification of yardang landforms is primarily performed manually. Researchers have focused on the color, morphology, and formation conditions of yardang landforms to characterize the Martian atmospheric environment and prevailing wind direction. Ward (1979), Bridges et al. (2007), and Zimbelman and Griffin, 2010 conducted analyses of the aspect ratio, scale distribution, and material properties of large-scale yardang landforms in the Martian Amazonian plain and Medusa trough layer, respectively. Additionally, some scholars have focused on dating yardang landforms (Kerber et al., 2011; Zimbelman and Scheidt, 2012; Liu et al., 2021a).
The identification of transverse aeolian ridges (TARs) is largely based on their sediment composition (Fenton et al., 2003; Balme and Bourke, 2005; Balme et al., 2008; Bourke et al., 2003) and the difference in albedo (Bouke et al., 2008; Gou et al., 2022) using visual interpretation. Lu et al. (2022) identified four crescent-shaped lateral sand ridges based on Zhurong rover exploration data and analyzed the erosion process of these beds. The orientation of these beds is related to the angle between the bed crest and the wind direction. Several typical aeolian landforms are shown in Figure 3.
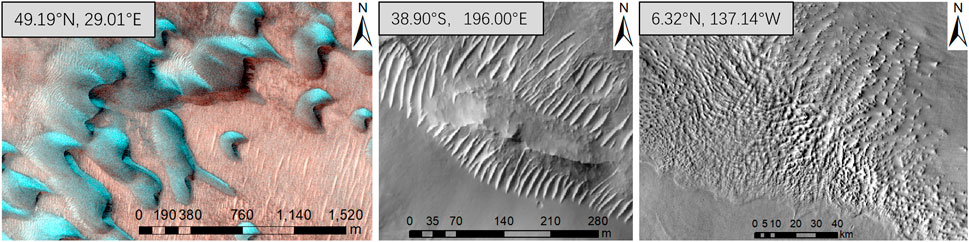
FIGURE 3. Several typical aeolian landforms (left, dunes, HiRISE; middle, TARs, HiRISE; right, yardangs, Themis day IR; modified after Balme et al., 2008).
4.2 Fluvial landforms
Fluvial landforms have long been regarded as the best evidence for the existence of liquid water on the Martian surface, which is essential for the existence of life and an indication of the warm and humid climate that once existed on Mars. Fluvial landforms can be categorized into narrowly defined fluvial landforms and broadly defined fluvial landforms. Narrowly, Ouyang and Zou (2015) further classified the fluvial landforms of Mars into outflow channels, valley networks, and gullies according to their size scale, as shown in Figure 4. Broadly, Zhao et al. (2021) classified the fluvial landforms of Mars into valley networks, outflow channels, paleolake basins, and alluvial fans and deltas. In this article, fluvial landforms were discussed from a narrow perspective, namely, outflow channels, valley networks, and gullies. These three landforms were distinguished by their sizes, patterns, and formation mechanisms.
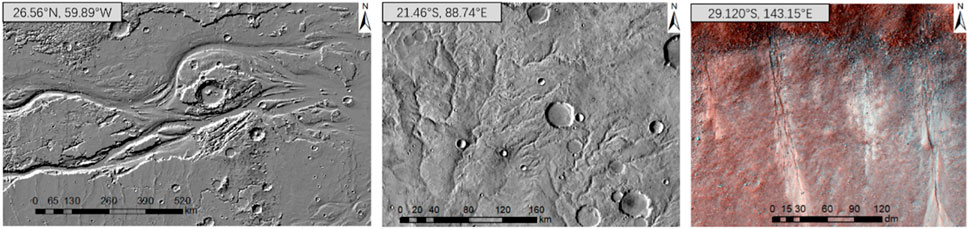
FIGURE 4. Several typical fluvial landform types (left, outflow channels, MOLA hillshade; middle, valley networks, Themis day IR; right, gullies, HiRISE).
The outflow channels on Mars formed during catastrophic floods (Cutts and Blasius, 1981), with a width of 1 km to several hundred km. Consequently, the age of their formation was deduced to be Hesperian (Liu et al., 2021b). Outflow channels are mostly found in fracture zones or near canyons. There are distinct tear-drop islands in the channels. In addition to their size, another obvious difference between valley networks and outflow channels is dendritic branching. Valley networks are mostly found in the old southern hemisphere and are very rare in the younger northern hemisphere, with a width of several kilometers. This suggested that valley network formation occurred on early Mars (Tanaka et al., 2014; Ouyang and Zou, 2015; Liu et al., 2021b). The traditional view holds that valleys resulted from surface runoff on early Mars, which climate was warm and wet (Carr, 2006; Hynek et al., 2010). Another view holds that valleys resulted from groundwater loss or glaciation. Gullies are distributed along slopes, with a width of several meters. Their formation is controversial, and may have included melting snow or CO2 ice. Generally, the identification of gullies is based on high-resolution data due to their small-scale features.
The methods used to identify valley networks can be divided into 1) Visual interpretation: Carr (2006) mapped the distribution of Martian valley networks and outflow channels. Hynek et al. (2010) mapped a Mars-wide network of canyons based on visible, infrared, and topographic data and analyzed the reasons for the presence of these canyons. Alemanno et al. (2018) modified the approach of Carr (2006), and the results showed that the valley network of Mars is predominantly located in the Southern Hemisphere, with small distributions in the Northern Hemisphere on the rim of the plateau and near Elysium volcano. “Visual interpretation” relies on the level of experts and costs a lot of manpower and time, which is similar with the pros and cons of visual interpretation for aeolian landforms. 2) Hydrological analysis: Stepinski and Collier (2004) proposed a method for extracting drainage networks from lower basins using a contributing area threshold, which was validated for 28 Noachian-age regions on Mars, with high efficiency and accuracy. Gou et al. (2018) extracted valley networks based on the DEM data from the Evros Vallis basin using traditional hydrological analysis methods, such as filling, flow direction analysis, catchment accumulation, and catchment area calculation. “Hydrological analysis” relies on quantitative algorithms and can provide more objective results compared to visual interpretation, reducing the potential for human bias. However, the accuracy of hydrological analysis methods depends on the validity of certain assumptions, which may not hold true in all situations. Furthermore, it is limited by the resolution of DEM. 3) Extraction of elevation change characteristics: Molly and Stepinski (2007) proposed a DEM-based method for the identification of landforms characterized by curvature and separated valleys from other landforms with convex shapes and then reconnected the segments along the drainage networks. The research about identification of gullies is rare due to the data resolution limitations (Li et al., 2015). Li et al. (2015) extracted Martian gullies in six regions using HiRISE images based on mathematical morphology, the bottom-hat transform and path opening and closing, with detection rates reaching 76%–94%. “Extraction of elevation change characteristics” is suitable for regional and global studies, while choosing appropriate parameters for elevation change analysis can be challenging.
4.3 Impact landforms
Impact landforms include impact craters and impact basins, such as the geomorphic units shown in Figure 5. As tracers of surface processes, impact craters are informative for studying subsurface minerals and useful for dating the planetary surface (Neukum et al., 1975; Wilhelms et al., 1987; Hartmann and Neukum, 2001). Thus, related studies are important for assessing planetary geomorphology. Furthermore, impact basins are often regarded as impact craters when they are identified. Mars and the Moon are largely similar in their morphological characteristics regarding impact crater landforms, and therefore, this paper combines the progression of impact crater studies on Mars and the Moon.
The methods for identifying impact craters can be divided into manual and automatic identification methods. Manual identification aims to identify accurate boundaries based on the visual interpretation of impact craters in images, topographic data and the subsequent derived data. However, manual identification is time-consuming and laborious, relying heavily on expert knowledge of individual identifiers, and the identification standards are inconsistent. Currently, the published Mars impact crater databases include the Barlow (1988) database, MA132843GT (Salamuniccar et al., 2012; Robbins and Hynek, 2010; Robbins and Hynek, 2012) database. The Barlow database manually extracted 25,826 impact craters with diameters greater than or equal to 8 km and relatively young ages based on Viking images, of which 60% were formed by heavy bombardment. The MA132843GT catalog is based on the MA130301GT catalog (Salamunićcar et al., 2011b), which combines manually mapped impact craters and 72,668 impact craters extracted by automatic identification algorithms. The Robbins database contains 384,343 impact craters with diameters greater than or equal to 1 km, which were manually identified from images.
The automatic recognition algorithm saves time and effort compared with manual recognition, and the recognition standard is uniform. Automatic recognition algorithms can be roughly divided into four categories: 1) Traditional edge detection and circle fitting algorithms. Edge extraction is achieved by using grayscale mutation on both sides of the impact crater rim (Kim et al., 2005), such as via the Sobel operator (Lu et al., 2013), the Robert operator (Yuan et al., 2013), the Prewitt operator, the Canny algorithm (Salamunićcar et al., 2010; Jiang et al., 2013), the region growing method (Luo et al., 2014), and the Hough transform. In addition, the bright and dark areas formed by impact craters in optical images (Urbach and Stepinski, 2009) can be paired to identify impact craters. This method can be easily understood, while the results were fitting circles, instead of accurate boundaries. 2) Digital terrain analysis method. Based on the changes in terrain factors, such as elevation and slope at the rim of a crater, watershed analysis, contour lines, and the extraction of terrain features were used to identify impact craters from a three-dimensional perspective (Bue and Stepinski, 2007; Luo et al., 2013; Xie et al., 2013; Liu et al., 2017; Chen et al., 2018). This method obtained changes from elevation and variations in crater boundaries. However, it may provide low efficiency and be influenced easily by minor terrain mutations. 3) Traditional machine learning algorithms. These methods tend to first express the features of impact craters using feature descriptors, such as Haar and PHOG, and then combine them with traditional machine learning models, such as SVM and AdaBoost (Ding et al., 2013; Bandeira et al., 2014), to perform impact crater detection. The quality of the training results depends on the quality of feature selection (Liu et al., 2023). 4) Deep learning algorithms. Deep learning is data-driven and is used to delegate feature selection to a machine learning algorithm. Deep learning can be used to extract features automatically from images, which is comparable to the idea of a “black box”. Nevertheless, it is highly dependent on the quality of the data and uneasily interpretative (Hsu et al., 2021; Silburt et al., 2019; Yang et al., 2020; Zheng et al., 2020; Gao et al., 2022). Combining digital terrain analysis and deep learning may be a further development direction, which could consider geographical information. The results could provide accurate boundaries over large-scale regions.
4.4 Glacial landforms
Martian glacial landforms are closely related to the presence of water, the stable presence of which is necessary to support life. The topography of Mars has a distinct north‒south dichotomy, with high south and low north topography. The large topographic height difference redistributes water between the poles, resulting in glacial landforms in the mid-latitudes (Hepburn et al., 2020), as shown in Figure 6.
Souness and Hubbard, 2012 identified and cataloged Martian glacier-like landforms from 8058 CTX images, 1,309 of which were concentrated at mid-latitudes of 39.3°N and 40.7°S, and inferred the response mechanisms of Martian glacial landforms based on latitude and altitude. There are various types of Martian glacial landforms, including concentric crater fills (CCFs), lobed valley fills (LVFs), lobate debris aprons (LDAs), and viscous flow features (VFFs) (Levy et al., 2010; Pedersen and Head, 2010; Liu et al., 2021c). Milliken et al. (2003) used 13,000 Mars orbiting camera images distributed globally to identify 146 images containing VFFs and marked the locations of these VFFs as points. Galofre et al. (2022) combined data from CaSSIS, SHARAD, CTX, and HiRISE to explore the deformation history of tongue-shaped rocky debris slopes, their internal structure, and their influence on regional climate change from surface morphology, which can be used to infer past glaciation on Mars. Petersen et al. (2018) used SHARAD radar data to estimate the water ice content (greater than 80%) in LDAs. Furthermore, only a small fraction of cases that matched typical surface water erosion indicated extensive subglacial erosion on the Martian surface after analyzing more than 10,000 Martian valleys.
In addition to Martian glacial landforms (beyond CCFs, LVFs, LDAs, and VFFs), periglacial landforms, such as pingos (Dundas et al., 2008; Richard et al., 2021), scallop-shaped depressions (Lefort et al., 2010), polygonal terrains (Soare et al., 2021), and thermokarst depressions (Bernhardt et al., 2016), are also present. Especially, some glacial-relevant landforms were found in the mid-latitudes of the Utopia Planitia. Wang et al. (2021) applied 2D-PCA and identified potential water ice in Utopia Planitia using FP-SPR data from the Zhurong rover. Bina and Osinski, 2021 found a new landform “decameter-scale rimmed depressions” in Utopia Plantita using SHARAD, which represents a further marker for the presence of ground ice in the northern plains of Mars.
Although glacial landforms, such as VFFs, CCFs, LVFs, and LDAs, have been discussed, studies have focused more on the formation, evolution, material composition, and structure of the above landforms and less on their identification. Additionally, the relationship of periglacial and glacial landforms between Mars climate evolution has been a focus of glacial landform research.
5 Prospects for studying Martian surface topography
Since Martian samples cannot be returned to Earth at present, the study of the surface topographic features of Mars based on remote sensing is still a very important direction of research, which has promoted a better understanding of the geological development and evolution of Mars, the dating of typical geomorphic units and the inference of its genesis. The Mars exploration project, the production of Mars optical image data and derivative products, and the study of Martian aeolian landforms and impact landforms have received more attention than other topics. However, more focus and deeper research are still needed in the directions of Martian landform classification and mapping, fluvial and glacier landform identification, and comparative planetary studies.
5.1 Mars data acquisition and mapping
Topographic data are characterized without the limitation of optical light and darkness and reflect the real terrain. Global-scale Martian data are usually characterized by low resolution, and localized data have a high resolution but a limited range. In low-resolution data, some fine-textured geomorphic units, such as gullies, miniature yardangs, and secondary impact craters, cannot be identified. Data resolution is an important factor affecting the recognition accuracy. However, the current Mars global DEM data resolution is 463 m/pixel (the blended DEM from MOLA and HRSC data resolution has a resolution of 200 m/pixel), which is not sufficient for the recognition and analysis of fine features. The production of higher-resolution global-scale Mars topographic data would be beneficial for the identification of finer geomorphic units.
In Mars geomorphology mapping research, some specific geomorphological units and Mars geological maps have been produced, but there is a lack of global geomorphological regionalization maps for Mars. Therefore, the digital geomorphological regionalization methods of the Earth and Moon could be used as references to draw the Mars geomorphological regionalization classification map based on the topographic indexes of Mars, such as relief, slope and elevation. This approach could aid in exploring the spatial differentiation characteristics of the Mars surface evolution process.
5.2 Construction of a classification system for Martian landforms
Mars has landforms that are typical of both the Earth and the Moon. The “three classes and nine levels” classification system for Earth’s landforms and the “three classes and eight levels” classification system for lunar landforms are useful for establishing a complete classification system of Martian landforms. However, the applicability of these classification systems to Martian landforms needs to be further explored. At present, studies on impact craters (Lei, 2017), sand dunes (Li et al., 2020), and yardangs (Liu, 2021) have established a detailed morphological index system, but index systems for describing other geomorphic types has been less studied. Most of the existing geomorphological classification studies (Bue and Stepinski, 2006; Wang et al., 2017; Deng et al., 2022; Liu et al., 2022) have divided geomorphological units into subtypes based on morphology. However, there have been few studies of descriptive indexes reflecting the formation or material composition. Combining the cause of formation and morphology is the key to geomorphological classification (Shen et al., 1982; Cheng et al., 2011). Combined with multisource remote sensing data, including visible images, thermal infrared images and topographic data, descriptive indexes considering the formation, material composition and geological age was introduced to build a complete index system and a database of corresponding geomorphic units. The construction of an index system for Martian geomorphic classification needs to be further explored.
5.3 Identification of typical geomorphic units of Mars
In terms of recognizable objects, automatic recognition has been rapidly developed in the field of impact crater recognition; however, to recognize more complex geomorphic units, such as sand dunes, yardangs, canyons, valley networks, gullies and degraded impact craters, manual recognition is still the main identification method. Manual identification could represent the real morphology accurately, but it is time-consuming and laborious and depends on the professional knowledge level of the experts. That results in heterogeneous mapping standards and heterogeneous mapping quality. Therefore, in research on the recognition of geomorphological units with complex morphology, automatic recognition methods need to be studied further.
In terms of the applicable scope of the recognition methods, most existing terrain classification methods are applicable to small-scale or local-scale terrain classification, while there are fewer automatic recognition methods applicable to large-scale or even global-scale classification. Although large-scale catalogs of impact craters (Robbins and Hynek, 2012), sand dunes (Hayward et al., 2007), yardangs (Liu, 2021), valley networks (Alemanno et al., 2018), and glacial-like landforms (Souness and Hubbard, 2012) were proposed, these features were identified manually rather than automatically. Because the accuracy, efficiency and robustness of these automatic methods for the global-scale regions were unsatisfactory, the automatic methods were typically applied in local regions. The methods that are applicable only for local small-scale target recognition lose the essential advantages of automatic recognition methods. Therefore, automatic recognition methods that are applicable at large and global scales require further research.
In terms of the requirements of recognition methods based on the users’ level of knowledge, the current Mars morphology recognition are mostly based on traditional machine learning, with a lack of end-to-end deep learning methods. On the one hand, the effect of machine learning depends on feature selection (Guyon and Elisseeff, 2003; Domingos, 2012), which requires a high level of expertise for the user. Deep learning is data-driven with the ability of automatic representation learning (Qian et al., 2022; Chen et al., 2023). On the other hand, traditional machine learning provides interpretable models, while deep learning is often seen as a “black box”. It is difficult to interpret the modeling process. Furthermore, deep learning requires more computational resources and larger training datasets than machine learning. Currently, end-to-end deep learning models are widely used in various professional fields, and it is hoped that they can be applied to intelligent terrain type recognition in the case of Mars. Broadly applicable training datasets and well-performing models are expected to be developed to identify multiple Martian terrain units.
Author contributions
DL: Investigation, Writing–original draft, Writing–review and editing, Conceptualization. WC: Conceptualization, Funding acquisition, Project administration, Supervision, Writing–review and editing.
Funding
The author(s) declare financial support was received for the research, authorship, and/or publication of this article. This article was funded by the B-type Strategic Priority Program of the Chinese Academy of Sciences, Grant No. XDB41000000 and National Natural Science Foundation of China, No. 42130110.
Acknowledgments
We appreciate the detailed suggestions and constructive comments from the editor and the reviewers.
Conflict of interest
The authors declare that the research was conducted in the absence of any commercial or financial relationships that could be construed as a potential conflict of interest.
Publisher’s note
All claims expressed in this article are solely those of the authors and do not necessarily represent those of their affiliated organizations, or those of the publisher, the editors and the reviewers. Any product that may be evaluated in this article, or claim that may be made by its manufacturer, is not guaranteed or endorsed by the publisher.
References
Alemanno, G., Orofino, V., and Mancarella, F. (2018). Global map of Martian fluvial systems, age and total eroded volume estimations. Earth Space Sci. 5 (10), 560–577. doi:10.1029/2018ea000362
Archinal, B. A., A’Hearn, M. F., Bowell, E., Conrad, A., Consolmagno, G. J., Courtin, R., et al. (2011). Report of the IAU working group on cartographic coordinates and rotational elements: 2009. Celest. Mech. Dyn. Astron. 109 (2), 101–135. doi:10.1007/s10569-010-9320-4
Balme, M., Berman, D. C., Bourke, M. C., and Zimbelman, J. R. (2008). Transverse aeolian ridges (TARs) on Mars. Geomorphology 101 (4), 703–720. doi:10.1016/j.geomorph.2008.03.011
Balme, M. R., and Bourke, M. C. (2005). Preliminary results from a new study of transverse aeolian ridges (TARS) on mars//36th lunar and planetary science conference. Houston, Texas: The Open University 1892.
Bandeira, L., Machado, M., and Pina, P. (2014). Automatic detection of sub-km craters on the Moon. Lunar Planet. Sci. Conf.
Bandeira, L., Marques, J. S., and Saraiva, J. (2010). Automated detection of sand dunes on Mars//7th international conference on image analysis and recognition. Image Analysis Recognit. 6112 (1), 306–315. doi:10.1007/978-3-642-13775-4_31
Barlow, N. G. (1988). Crater size-frequency distributions and a revised Martian relative chronology. Icarus 75 (2), 285–305. doi:10.1016/0019-1035(88)90006-1
Barrett, M. A., Balme, R. M., Woods, M., Karachalios, S., Petrocelli, D., Joudrier, L., et al. (2022). NOAH-H, a deep-learning, terrain classification system for Mars: results for the ExoMars Rover candidate landing sites. Icarus 371 (1), 114701. doi:10.1016/j.icarus.2021.114701
Bernhardt, H., Reiss, D., Hiesinger, H., and Ivanov, M. A. (2016). The honeycomb terrain on the Hellas basin floor, Mars: a case for salt or ice diapirism. J. Geophys. Res. Planets 121 (4), 714–738. doi:10.1002/2016je005007
Bina, A., and Osinski, R. G. (2021). Decameter-scale rimmed depressions in Utopia Planitia: insight into the glacial and periglacial history of Mars. Planet. Space Sci. 204, 105253. doi:10.1016/j.pss.2021.105253
Bourke, M. C., Wilson, S. A., and Zimbelman, J. R. (2003). The variability of transverse aeolian ridges in troughs on Mars//34th Lunar and Planetary Science Conference.Texas: League, 2090.
Bridges, N. T., Geissler, P. E., Mcewen, A. S., Thomson, B. J., Chuang, F. C., Herkenhoff, K. E., et al. (2007). Windy Mars: a dynamic planet as seen by the HiRISE camera. Geophys. Res. Lett. 34 (23), 497–507. doi:10.1029/2007gl031445
Bue, B. D., and Stepinski, T. F. (2006). Automated classification of landforms on Mars. Comput. Geosci. 32 (5), 604–614. doi:10.1016/j.cageo.2005.09.004
Bue, B. D., and Stepinski, T. F. (2007). Machine detection of Martian impact craters from digital topography data. IEEE Trans. Geosci. Remote Sens. 45 (1), 265–274. doi:10.1109/tgrs.2006.885402
Carrera, D., Bandeira, L., Santana, R., and Lozano, J. A. (2019). Detection of sand dunes on Mars using a regular vine-based classification approach. Knowl. Based Syst. 163 (1), 858–874. doi:10.1016/j.knosys.2018.10.011
Chen, M., Liu, D. Y., Qian, K. J., Li, J., Lei, M., and Zhou, Y. (2018). Lunar crater detection based on terrain analysis and mathematical morphology methods using digital elevation models. IEEE Trans. Geosci. Remote Sens. 56 (7), 3681–3692. doi:10.1109/tgrs.2018.2806371
Chen, M., Qian, Z., Boers, N., Jakeman, A. J., Kettner, A. J., Brandt, M., et al. (2023). Iterative integration of deep learning in hybrid Earth surface system modelling. Nat. Rev. Earth Environ. 4, 568–581. doi:10.1038/s43017-023-00452-7
Cheng, W. M., Liu, Q. Y., and Wang, J. (2018). A preliminary study of classification method on lunar topography and landforms. Adv. Earth Sci. 33 (9), 885–897. doi:10.11867/j.issn.1001-8166.2018.09.0885
Cheng, W. M., Zhou, C. H., Li, B. Y., Shen, Y., and Zhang, B. (2011). Structure and contents of layered classification system of digital geomorphology for China. J. Geogr. Sci. 21 (5), 771–790. doi:10.1007/s11442-011-0879-9
Cutts, J. A., and Blasius, K. R. (1981). Origin of Martian outflow channels: the Eolian hypothesis. J. Geophys. Res. 86 (NB6), 5075–5102. doi:10.1029/jb086ib06p05075
Deng, J. Y., Cheng, W. M., Liu, Q. Y., Jiao, Y. M., and Liu, J. Z. (2022). Morphological differentiation characteristics and classification criteria of lunar surface relief amplitude. J. Geogr. Sci. 32 (11), 2365–2378. doi:10.1007/s11442-022-2052-z
Di, K. C., Liu, B., and Liu, Z. Q. (2018). Review and prospect of Mars mapping technique using remote sensing data. Spacecr. Eng. 27 (1), 10–24. doi:10.3969/j.issn.1673-8748.2018.01.002
Di, K. C., Ye, L. J., Wang, R. Z., and Wang, Y. (2021). Advances in planetary target detection and classification using remote sensing data. Nat. Remote Sens. Bull. 25 (1), 365–380. doi:10.11834/jrs.20210231
Ding, M., Cao, Y. F., and Wu, Q. X. (2013). Novel approach of crater detection by crater candidate region selection and matrix-pattern-oriented least squares support vector machine. Chin. J. Aeronaut. 26 (2), 385–393. doi:10.1016/j.cja.2013.02.016
Domingos, P. (2012). A few useful things to know about machine learning. Commun. ACM 55 (10), 78–87. doi:10.1145/2347736.2347755
Dong, Z. B., Lu, P., and Li, C. (2020b). Research methodology of Martian aeolian geomorphology. Adv. Earth Sci. 35 (8), 771–788.
Dundas, C. M., Mellon, M. T., McEwen, A. S., Lefort, A., Keszthelyi, L. P., and Thomas, N. (2008). HiRISE observations of fractured mounds: possible Martian pingos. Geophys. Res. Lett. 35, L04201. doi:10.1029/2007GL031798
Duxbury, T. C., Krik, R. L., and Archinal, B. A. (2002). Mars geodesy/cartography working group recommendations on mars cartographic constants and coordinate systems//Proceeding of the ISPRS Commission IV Symposium “Geospatial Theory, Processing and applications”. Ottawa: ISPRS Comission IV.
Fenton, L. K., Bandfield, J. L., and Ward, A. W. (2003). Aeolian processes in Proctor Crater on Mars:Sedimentary history as analyzed from multiple data sets. J. Geophys. Res. Planets 108 (E12), 5129. doi:10.1029/2002JE002015
Galofre, G. A., Whipple, K. X., Christensen, P. R., and Conway, S. J. (2022). Valley networks and the record of glaciation on ancient Mars. Geophys. Res. Lett. 49, e2022GL097974. doi:10.1029/2022GL097974
Gao, A., Zhou, Y. J., and Wang, J. W. (2022). Lightweight deep learning method for Lunar surface crater detection. J. Astron. 43 (6), 830–838. doi:10.3873/j.issn.1000-1328.2022.06.014
Geomorphic Map Editorial Committee of the People’s Republic of China (2009). Geomorphic atlas of the People’s Republic of China (1:100000). Science Press.
Gou, S., Yue, Z. Y., Di, K. C., and Xu, Y. (2018). Quantitative comparison of morphometric and hydrological characteristics of valley networks between Evros Vallis on Mars and Kaidu River in Tarim Basin as terrestrial analog. J. Remote Sens. 22 (2), 313–323. doi:10.11834/jrs.20187014
Gou, S., Yue, Z. Y., Di, K. C., Zhao, C., Bugiolacchi, R., Xiao, J., et al. (2022). Transverse aeolian ridges in the landing area of the tianwen-1 Zhurong rover on utopia Planitia, Mars. Earth Planet. Sci. Lett. 595 (10), 117764. doi:10.1016/j.epsl.2022.117764
Guyon, I., and Elisseeff, A. (2003). An introduction to variable and feature selection. J. Mach. Learn. Res. 3, 1157–1182. doi:10.5555/944919.944968
Hartmann, W. K., and Neukum, G. (2001). Cratering chronology and the evolution of Mars. Space Sci. Rev. 96 (1-4), 165–194. doi:10.1023/a:1011945222010
Hayward, R. K., Mullins, K. F., Fenton, L. K., Hare, T. M., Titus, T. N., Bourke, M. C., et al. (2007). Mars global digital dune database and initial science results. J. Geophys. Res. Planets 112 (E11), E11007. doi:10.1029/2007je002943
Hepburn, A. J., Ng, F. S. L., Holt, T. O., and Hubbard, B. (2020). Late amazonian ice survival in kasei valles, Mars. J. Geophys. Res. Planets 125, e2020JE006531. doi:10.1029/2020JE006531
Hsu, C. Y., Li, W., and Wang, S. (2021). Knowledge-Driven GeoAI: integrating spatial knowledge into multi-scale deep learning for Mars crater detection. Remote Sens. 13 (11), 2116. doi:10.3390/rs13112116
Hynek, M. B., Beach, M., and Hoke, T. M. (2010). Updated global map of Martian valley networks and implications for climate and hydrologic processes. J. Geophys. Res. Planets 115 (E9), E09008. doi:10.1029/2009je003548
Institute of Geography, Chinese Academy of Sciences (1987). China’s 1:100000 topographic map mapping specification[S]. Science Press.
Jiang, H. K., Tian, X. L., and Xu, A. (2013). An automatic algorithm for detecting lunar impact craters in a defined feature space. Sci. Sin-Phys Mech. As 43 (11), 1430–1437. doi:10.1360/132013-321
Jiang, S. C., Wu, F., Yung, K. L., Yang, Y., Ip, W., Gao, M., et al. (2021). A robust end-to-end deep learning framework for detecting Martian landforms with arbitrary orientations. Knowl. Based. Syst. 12, 107562. doi:10.1016/j.knosys.2021.107562
Kerber, L., Head, J. W., Madeleine, J.-B., Forget, F., and Wilson, L. (2011). The dispersal of pyroclasts from apollinaris patera, Mars: implications for the origin of the medusae fossae formation. Icarus 216 (1), 212–220. doi:10.1016/j.icarus.2011.07.035
Kim, J. R., Muller, J. P., Van Gasselt, S., Morley, J. G., and Neukum, G. (2005). Automated Crater detection, A new tool for Mars cartography and chronology. Photogramm. Eng. Remote Sens. 71 (10), 1205–1217. doi:10.14358/pers.71.10.1205
Kim, J. R., and Muller, J. P. (2008). Very high resolution stereo DTM extraction and its application to surface roughness estimation over Martian surface. Int. Arch. Photogramm. Remote Sens. Spat. Inf. Sci. 37 (B4), 993–998. doi:10.14358/PERS.71.10.1205
Lakdawalla, E. (2005). The planetary society. Map of Mars with major regions labeled. Available at: https://www.planetary.org/space-images/map-mars-major-features.
Lefort, A., Russell, P. S., and Thomas, N. (2010). Scalloped terrains in the peneus and amphitrites paterae region of Mars as observed by HiRISE. Icarus 205 (1), 259–268. doi:10.1016/j.icarus.2009.06.005
Lei, M. L. (2017). Indexes of lunar impact craters based on the morphology characteristics. Nanjing: Nanjing Normal University.
Levy, J., Head, J. W., and Marchant, D. R. (2010). Concentric crater fill in the northern mid-latitudes of Mars: formation processes and relationships to similar landforms of glacial origin. Icarus 209 (2), 390–404. doi:10.1016/j.icarus.2010.03.036
Li, C., Dong, Z. B., Lü, P., Zhao, J., Fu, S., Feng, M., et al. (2020). A morphological insight into the Martian dune geomorphology. Chin. Sci. Bull. 65, 80–90. doi:10.1360/TB-2019-0168
Li, H. L., Li, J., and Ren, X. (2022). Deep learning eliminates massive dust storms from images of tianwen-1. Comput. Vis. Pattern Recognit. ArXiv. Preprint. Available at: https://arxiv.org/abs/2206.10145.
Li, W., Di, K. C., Yue, Z. Y., Liu, Y., and Sun, S. (2015). Automated detection of martian gullies from HiRISE imagery. Photogramm. Eng. Remote Sens. 81 (12), 913–920. doi:10.14358/pers.81.12.913
Liu, D. Y., Chen, M., Qian, K. J., Lei, M., and Zhou, Y. (2017). Boundary detection of dispersal impact craters based on morphological characteristics using lunar digital elevation model. IEEE J-STARS 10 (12), 5632–5646. doi:10.1109/jstars.2017.2749403
Liu, D. Y., Cheng, W. M., Qian, Z., Deng, J. Y., Liu, J. Z., and Wang, X. M. (2023). Boundary delineator for martian crater instances with geographic information and deep learning. Remote Sens. 15, 4036. doi:10.3390/rs15164036
Liu, J., Di, K. C., and Gou, S. (2020). Mapping and spatial statistical analysis of Mars Yardangs. Planet. Space Sci. 192, 105035. doi:10.1016/j.pss.2020.105035
Liu, J. (2021). Global mapping and formation mechanism study of Mars yardangs. Beijing: University of Chinese Academy of Sciences.
Liu, J., Yue, Z. Y., Di, K. C., Gou, S., and Niu, S. (2021a). A study about the temporal constraints on the martian yardangs’ development in medusae fossae formation. Remote Sens. 13, 1316. doi:10.3390/rs13071316
Liu, J. Z., Ouyang, Z. Y., and Li, C. L. (2006). A preliminary study on the scientific objectives and optimization principles of mars exploration//improve the scientific quality of the whole people and build an innovative country – proceedings of the 2006 annual conference of the Chinese association for science and Technology, Volume II.
Liu, Q. Y., Cheng, W. M., and Yan, G. J. (2022). Distribution characteristics and classification schemes of lunar surface elevation. Acta Geogr. Sin. 76 (1), 106–119. doi:10.1016/j.epsl.2022.117785
Liu, Y., Wu, X., Liu, Z. H., Zhou, Q., and Chen, X. (2021b). Geological evolution and habitable environment of Mars: progress and prospects. Rev. Geophys. Planet. Phys. 52 (4), 416–436. doi:10.1038/d41573-021-00080-0
Liu, Y., Liu, Z. H., Wu, X., Qin, L., Wu, Y. H., Zhang, C. L., et al. (2021c). Evolution of water environment on Mars. Acta Geologica Sinica 95 (9), 2725–2741. doi:10.19762/j.nki.Izhixuebao.2021270
Lu, Y. H., Miao, F., and Du, J. (2013). An automatic detection algorithm of lunar craters based on feature matching. Sci. Surv. Map. 38 (5), 5.
Lu, Y., KennethEdgettWu, S. B., Wang, Y., Li, Z., Michael, G. G., et al. (2022). Aeolian disruption and reworking of TARs at the Zhurong rover field site, southern Utopia Planitia, Mars. Earth Planet Sci. Lett. 595 (10), 117785. doi:10.1016/j.epsl.2022.117785
Luo, L., Mu, L. L., Wang, X. Y., Li, C., Ji, W., Zhao, J., et al. (2013). Global detection of large lunar craters based on the CE-1 digital elevation model. Front. Earth Sci. 7 (4), 456–464. doi:10.1007/s11707-013-0361-3
Luo, W., and Stepinski, T. F. (2009). Computer-generated global map of valley networks on Mars. J. Geophys. Res. 114, E11010. doi:10.1029/2009JE003357
Luo, Z. F., Kang, Z. Z., and Liu, X. Y. (2014). The automatic extraction and recognition of lunar impact craters fusing CCD images and DEM data of Chang’e-1. Acta Geod. Cartogr. Sin. 43 (9), 924–930. doi:10.13485/j.cnki.11-2089.2014.0137
Mandt, K., and LeoneYardang, G. (2015). Encyclopedia of planetary landforms. Editors H. Hargitai, and A. Kereszturi (New York: Springer), 2340–2347.
Milliken, R. E., Mustard, J. F., and Goldsby, D. L. (2003). Viscous flow features on the surface of Mars: observations from high-resolution Mars Orbiter Camera (MOC) images. J.Geophys. Res. 108 (E6), 5057. doi:10.1029/2002je002005
Molly, I., and Stepinski, T. F. (2007). Automatic mapping of valley networks on Mars. Comput. Geosci. 33, 728–738. doi:10.1016/j.cageo.2006.09.009
Neukum, G., König, B., and Arkani-Hamed, J. (1975). A study of lunar impact crater size-distributions. moon 12 (2), 201–229. doi:10.1007/bf00577878
Orosei, R., Lauro, S. E., Pettinelli, E., Cicchetti, A., Coradini, M., Cosciotti, B., et al. (2018). Radar evidence of subglacial liquid water on Mars. Science 361 (6401), 490–493. doi:10.1126/science.aar7268
Ouyang, Z. Y., and Xiao, F. G. (2011). Major scientific issues involved in Mars exploration. Spacecr. Environ. Eng. 28 (3), 205–217. doi:10.3969/j.issn.1673-1379.2011.03.001
OuYang, Z. Y., and Zou, Y. L. (2015). Introduction to martian science. Shanghai Science and Technology Education Press.
Pedersen, G. B. M., and Head, J. W. (2010). Evidence of widespread degraded Amazonian-aged ice-rich deposits in the transition between Elysium Rise and Utopia Planitia, Mars: guidelines for the recognition of degraded ice-rich materials. Planet. Space Sci. 58 (14-15), 1953–1970. doi:10.1016/j.pss.2010.09.019
Petersen, L. E., Holt, W. J., and Levy, S. J. (2018). High ice purity of Martian lobate debris aprons at the regional scale: evidence from an orbital radar sounding survey in Deuteronilus and Protonilus Mensae. Geophys. Res. Lett. 45, 11595–11604. doi:10.1029/2018gl079759
Piqueux, S., Buz, J., Edwards, C. S., Bandfield, J. L., Kleinböhl, A., Kass, D. M., et al. (2019). Widespread shallow water ice on Mars at high latitudesand midlatitudes. Geophys. Res. Lett. 46 (24), 14290–14298. doi:10.1029/2019GL083947
Qian, Z., Min, C., Teng, Z., Fan, Z., Rui, Z., Zhang, Z. X., et al. (2022). Deep Roof Refiner: a detail-oriented deep learning network for refined delineation of roof structure lines using satellite imagery. Int. J. Appl. Earth Obs. 107, 102680. doi:10.1016/j.jag.2022.102680
Robbins, S. J., and Hynek, B. M. (2012). A new global database of Mars impact craters≥ 1 km: 1. Database creation, properties, and parameters. J. Geophys. Res. Planets 117 (E5). 1991 –2012. E05004. doi:10.1029/2011je003966
Robbins, S. J., and Hynek, B. M. (2010). Progress towards a new global catalog of Martian craters and layered ejecta properties, complete to 1.5 km//Proceedings of the 41st Lunar and Planetary Science Conference.
Rothrock, B., Kennedy, R., and Cunningham, C. (2016). SPOC: deep learning-based terrain classification for mars rover missions, 9. AIAA Space.
Rubanenko, L., Perez-Lopez, S., Schull, J., and Lapotre, M. G. A. (2021). Automatic detection and segmentation of barchan dunes on Mars and Earth using a convolutional neural network. IEEE J-STARS. 14 (11), 9364–9371. doi:10.1109/jstars.2021.3109900
Salamunićcar, G., Loncaric, S., and Lončarić, S. (2010). Method for crater detection from Martian digital topography data using gradient value/orientation, morphometry, vote analysis, slip tuning, and calibration. IEEE Trans. Geosci. Remote Sens. 48 (5), 2317–2329. doi:10.1109/tgrs.2009.2037750
Salamuniccar, G., Loncaric, S., and Mazarico, E. M. (2012). LU60645GT and MA132843GT catalogues of Lunar and Martian impact craters developed using a Crater Shape-based interpolation crater detection algorithm for topography data. Planet. Space Sci. 60, 236–247. doi:10.1016/j.pss.2011.09.003
Salamunićcar, G., Loncaric, S., Pina, P., Bandeira, L., and Saraiva, J. (2011b). MA130301GT catalogue of Martian impact craters and advanced evaluation of crater detection algorithms using diverse topography and image datasets. Planet. Space Sci. 59 (1), 111–131. doi:10.1016/j.pss.2010.11.003
Seidelmann, P. K. (2002). Report of the IAU/IAG working group on cartographic coordinates and rotational elements of the planets and satellites: 2000. Celest. Mech. Dyn. Astron. 82, 83–110. doi:10.1007/s10569-007-9072-y
Shang, C., and Barnes, D. (2013). Fuzzy-rough feature selection aided support vector machines for Mars image classification. Comput. Vis. Image Underst. 117 (3), 202–213. doi:10.1016/j.cviu.2012.12.002
Shen, Y. C., Su, S. Y., and Yin, Z. S. (1982). Retrospect and prospect of the research work on the classification, regionalization and mapping of the geomorphology of China. Sci. Geol. Sin. 2 (2), 97–105. doi:10.13249/j.cnki.sgs.1982.02.97
Silburt, A., Ali-Dib, M., Zhu, C. C., Jackson, A., Valencia, D., Kissin, Y., et al. (2019). Lunar crater identification via deep learning. Icarus 317 (1), 27–38. doi:10.1016/j.icarus.2018.06.022
Soare, J. R., Williams, J. P., Conway, J. S., and El-Maarry, M. R. (2021). Pingo-like mounds and possible polyphase periglaciation/glaciation at/adjacent to the Moreux impact crater. Mars Geol. Enigmas, 407–435. doi:10.1016/B978-0-12-820245-6.00014-8
Souness, C., and Hubbard, B. (2012). Mid-latitude glaciation on Mars. Prog. Phys. Geogr. Earth Env. 36 (2), 238–261. doi:10.1177/0309133312436570
Stepinski, T. F., and Collier, M. L. (2004). Extraction of Martian valley networks from digital topography. J. Geophys. Res. Planets. 109 (E11): E11005, doi:10.1029/2004je002269
Tanaka, L. K., Skinner, A. J., and Dohm, M. J. (2014). Geologic map of Mars: U.S. Geol. Surv. Sci. Investig. Map 43. 3292, scale 1:20,000,000, pamphlet. doi:10.3133/sim3292
Urbach, E. R., and Stepinski, T. F. (2009). Automatic detection of sub-km craters in high resolution planetary images. Planet. Space Sci. 57 (7), 880–887. doi:10.1016/j.pss.2009.03.009
Vaucouleurs, G. D., Davies, M. E., and Sturms, F. M. (1973). Mariner 9 areographic coordinate system. J. Geophys. Res. 78 (20), 4395–4404. doi:10.1029/jb078i020p04395
Wang, J. (2018). Geologic characteristics of yardangs on Mars and their implications for paleo-environments: constraints from analog study between the Qaidam Basin and Aeolis-Zephyria region. Wuhan: China University of Geosciences.
Wang, J., Cheng, W. M., Zhou, C. H., and Zheng, X. (2017). Automatic mapping of lunar landforms using DEM-derived geomorphometric parameters. J. Geogr. Sci. 27 (11), 1413–1427. doi:10.1007/s11442-017-1443-z
Wang, W. J., Lin, L. L., Fan, Z. J., and Liu, J. (2021). Semi-supervised learning for Mars imagery classification. IEEE international conference on image processing (ICIP). doi:10.1109/ICIP42928.2021.9506533
Wang, Y., Feng, X., Zhou, H., Dong, Z., Liang, W., Xue, C., et al. (2021). Water ice detection research in utopia Planitia based on simulation of Mars rover full-polarimetric subsurface penetrating radar. Remote Sens. 13, 2685. doi:10.3390/rs13142685
Ward, A. W. (1979). Yardangs on Mars: evidence of recent wind erosion. J. Geophys. Res. 84 (B14), 8147–8166. doi:10.1029/jb084ib14p08147
Wilhelms, D. E., Mccauley, J. F., and Trask, N. J. (1987). The geologic history of the Moon. Washington DC: US Government Printing Office.
Wright, J., Barrett, M. A., Fawdon, P., Favaro, E. A., Balme, M. R., Woods, M. J., et al. (2022). Jezero crater, Mars: application of the deep learning NOAH-H terrain classification system. J. Maps. 18 (2), 484–496. doi:10.1080/17445647.2022.2095935
Xiao, L., Wang, J., Dang, Y. N., Cheng, Z. Y., Huang, T., Zhao, J. N., et al. (2017). A new terrestrial analogue site for Mars research: the Qaidam Basin, Tibetan Plateau (NW China). Earth-Science Rev. 164, 84–101. doi:10.1016/j.earscirev.2016.11.003
Xie, Y. Q., Tang, G. A., Yan, S. J., and Hui, L. (2013). Crater detection using the morphological characteristics of Chang’E-1 digital elevation models. IEEE Geosci. Remote Sens. Lett. 10 (4), 885–889. doi:10.1109/lgrs.2012.2226432
Yan, W., Ren, X., Liu, J. J., Zhang, L., Chen, W., Wang, D., et al. (2022). Topographic reconstruction of the “tianwen-1” landing area on the Mars using high resolution imaging camera images. IEEE Trans. Geosci. Remote Sens. 60 (9), 1–14. doi:10.1109/tgrs.2022.3206961
Yang, C., Zhao, H., Bruzzone, L., Benediktsson, J. A., Liang, Y., Liu, B., et al. (2020). Lunar impact crater identification and age estimation with Chang'E data by deep and transfer learning. Nat. Commun. 11 (12), 6358. doi:10.1038/s41467-020-20215-y
Yao, P. W. (2021). Spatiotemporal distribution of dust storm activity in Tianwen-1 landing area and Mars non-polar region based on Mars remote sensing images. Jinan: Shandong University.
Yuan, Y. F., Zhu, P. M., and Zhao, N. (2013). Automated identification of circular mare craters based on mathematical morphology. Sci. Sin-Phys Mech. As 43 (3), 324–332. doi:10.1360/132012-425
Yue, Z. Y., Di, K. C., Gregory, M., Gou, S., Lin, Y., and Liu, J. (2022). Martian surface dating model refinement based on Chang'E-5 updated lunar chronology function. Earth Planet. Sci. Lett. 595, 117765. doi:10.1016/j.epsl.2022.117765
Zhao, J. N. (2017). Geologic characteristics of the paleolakes in Martian southern highland: implications for Martian paleo-climate and paleo-environment. China University of Geosciences.
Zhao, J. N., Shi, Y. T., and Zhang, M. J. (2021). Advances in Martian water-related landforms. Acta Geol. Sin. 95 (9), 2755–2768. doi:10.19762/j.cnki.dizhixuebao.2021267
Zheng, L., Hu, W. D., and Liu, C. (2020). Large crater identification method based on deep learning. J. B. Univ. Aeron. Astron. 46 (5), 994–1004. doi:10.13700/j.bh.1001-5965.2019.0342
Zhou, C. H., Cheng, W. M., and Qian, J. K. (2009). Digital geomorphical interpretation and mapping from remote sensing/. Science Press.
Zimbelman, J. R., and Griffin, L. J. (2010). HiRISE images of yardangs and sinuous ridges in the lower member of the Medusae Fossae Formation, Mars. Icarus 205 (1), 198–210. doi:10.1016/j.icarus.2009.04.003
Keywords: Martian topography, landform classification, target recognition, Mars science, Martian mapping
Citation: Liu D and Cheng W (2023) Progress and prospects for research on Martian topographic features and typical landform identification. Front. Astron. Space Sci. 10:1275516. doi: 10.3389/fspas.2023.1275516
Received: 10 August 2023; Accepted: 13 October 2023;
Published: 02 November 2023.
Edited by:
Guangfei Wei, Deep Space Exploration Laborarory, ChinaReviewed by:
Yuyan Zhao, Chengdu University of Technology, ChinaJiannan Zhao, China University of Geosciences Wuhan, China
Copyright © 2023 Liu and Cheng. This is an open-access article distributed under the terms of the Creative Commons Attribution License (CC BY). The use, distribution or reproduction in other forums is permitted, provided the original author(s) and the copyright owner(s) are credited and that the original publication in this journal is cited, in accordance with accepted academic practice. No use, distribution or reproduction is permitted which does not comply with these terms.
*Correspondence: Weiming Cheng, Y2hlbmd3bUBscmVpcy5hYy5jbg==