- 1Department of Geophysics, College of the Geology Engineering and Geomatics, Chang’an University, Xi’an, China
- 2Center for Environmental Research and Earth Sciences (CERES), Salem, MA, United States
- 3Institute of Earth Physics and Space Science (ELKH EPSS), Sopron, Hungary
In this research, the Potential Field Source Surface–Wang–Sheeley–Arge (PFSS–WSA) solar wind model is used. This model consists of the Potential Field Source Surface (PFSS) coronal magnetic field extrapolation module and the Wang–Sheeley–Arge (WSA) solar wind velocity module. PFSS is implemented by the POT3D package deployed on Tianhe 1A supercomputer system. In order to obtain the three–dimensional (3D) distribution of the coronal magnetic field at different source surface radii (Rss), the model utilizes the Global Oscillation Network Group (GONG) photospheric magnetic field profiles for two Carrington rotations (CRs), CR2069 (in 2008) and CR2217 (in 2019), as the input data, with the source surface at Rss = 2Rs, Rss = 2.5Rs and Rss = 3Rs, respectively. Then the solar wind velocity, the coronal magnetic field expansion factor, and the minimum angular distance of the open magnetic field lines from the coronal hole boundary are estimated within the WSA module. The simulated solar wind speed is compared with the value for the corona extrapolated from the data observed near 1 AU, through the calculations of the mean square error (MSE), root mean square error (RMSE) and correlation coefficient (CC). Here we extrapolate the solar wind velocity at 1 AU back to the source surface via the Parker spiral. By comparing the evaluation metrics of the three source surface heights, we concluded that the solar source surface should be properly decreased with respect to Rss = 2.5Rs during the low solar activity phase of solar cycle 23.
1 Introduction
Among all the cosmic objects, the Sun has the most immediate and greatest impact on the Earth’s space environment. The solar phenomena that propagate from the surface of the Sun to the Earth can cause catastrophic space weather events, impacting the near-Earth space environment. Consequently, they can be hazardous for technology and human life, e.g., by threatening the safety of astronauts, affecting radio communications, disrupting theglobal positioning systems (GPS), and damaging satellites in orbit(Baker, 2002; Cao, 2012; Eastwood et al., 2017; Riley et al., 2018; Schwenn, 2006). Nowadays, human beings are highly dependent on the aerospace environment, radio communications, and GPS positioning, so it is increasingly necessary to understand and prepare for any potentially disastrous space weather events.
Therefore, the first requirement is to be able to monitor the space weather and forecast before the disaster occurs, which is indeed the goal for worldwide research (Robinson and Behnke, 2001). In recent years, the inversion studies of the 3D numerical model of coronal and interplanetary processes, based on mathematical physical methods, have been rapidly developed (Caplan et al., 2016; Feng et al., 2013; Feng et al., 2017; Feng et al., 2019; Mikić et al., 2018). The improvement of coronal and interplanetary 3D numerical models is and will continue to be an important topic in space weather for a long time (Feng et al., 2011; Gressl et al., 2014; Sahade et al., 2020).
We focus here on the solar magnetic field structure and the solar wind. The solar wind is a continuous stream of plasma emerging from the Sun carries an interplanetary magnetic field that shapes the large-scale fundamental structure of coronal interplanetary space and is the background for the propagation of other eruptive perturbation events. Forecasting the background solar wind is the basis for forecasting other coronal interplanetary outburst phenomena.
The commonly used solar wind models are the Wang–Sheeley (WS) model (Wang and Sheeley, 1990), the distance from the coronal hole boundary (DCHB) model (Riley et al., 2015), and the Wang–Sheeley–Arge (WSA) model (Arge et al., 2003). The WS model describes the quantitative relationship between velocity
The PFSS model is a coronal magnetic field model. It extrapolates the magnetic field of the photosphere onto a sphere with a “source surface.” Field lines that loop back down to the photosphere within the source surface form closed loops and are considered closed field lines. On the contrary, field lines that thread the source surface and extend above it, away from the Sun are considered open field lines. The source surface radius in a PFSS model is a free parameter (Arden et al., 2014). The radius of the source surface is important for the magnetic field simulation, which determines the size of the coronal hole area in the low coronal region. Increasing the source surface radius leads to less open flux and fewer and/or smaller coronal hole areas, while decreasing the source surface radius leads to more open flux and more and/or larger coronal hole areas (Arden et al., 2014; Riley et al., 2006). Usually, the radius of the source surface is assumed to obtain values within the interval of
The ordinary methods for solving potential field models include the spherical harmonics expansion method (Altschuler and Newkirk, 1969; Altschuler et al., 1977; Mackay and Yeates, 2012; Nikolj and Trichtchenko, 2012; Schulz et al., 1978; Schulz, 1997) and the finite difference method (Tóth, et al., 2011) and the least-squares method (Levine et al., 1982). In this paper, we solve the Laplace equation in a finite-difference numerical format to obtain the structure of the magnetic field at the source surface.
By using the PFSS model, the 3D distribution of the coronal magnetic field can be derived (Schatten et al., 1969; Schatten, 1971). By adding this magnetic field value into the formulae from the WSA model, physical parameters such as velocity (
In this paper, the WSA solar wind model is investigated. This model takes the photospheric magnetic field approximation from the Global Oscillation Network Group (GONG) as the input lower boundary condition. In addition, the magnetograms we used are synchronic. The coronal magnetic field extrapolation is performed for CR2069 and CR2217 through the PFSS. We start with the field line tracing method to determine
2 Numerical model
2.1 The PFSS model
In this paper, the approximate solution of the solar corona magnetic field is calculated by using the PFSS model with GONG (NSO/GONG: Data Access) measurements of the photospheric magnetic field as the boundary condition. GONG has six stations around the world, and they are ground-based stations that use the helioseismological principle to study the solar interior and can satisfy the near-continuous observation of solar oscillations. The GONG obtains the magnetic field of the entire photospheric surface based on the full-disk magnetogram of the six stations on the photosphere. The magnetogram files used in our study were retrieved from https://gong2.nso.edu/archive/patch.pl?menutype=zeroPoint.
The PFSS model is usually used to solve for the coronal magnetic field. The PFSS model assumes that there is no current in the corona and only uses the radial component of the magnetic field (Altschuler and Newkirk, 1969). We start from the momentum equation of the ideal MHD theory and assume that the corona is in a quasi-static equilibrium and has low-plasma beta. Low-plasma beta means that the magnetic pressure dominates over plasma pressure. In the corona region, we also assume that the magnetic pressure dominates non-magnetic forces (such as gravity for example). By eliminating now in the momentum equation all the terms that become 0 based on these three assumptions, one ends up with only one term:
where
The simulations of the PFSS approximation are performed employing the POT3D code, which solves the Laplace equation using the finite-difference numerical scheme. The finite-difference approach can match better with the data resolution than with the harmonic approach and can achieve better high resolution for localization (Caplan et al., 2021; Tóth, et al., 2011). Therefore, we use the finite-difference approach to solve the PFSS model. The reader is referred to Caplan et al. (2021) for a detailed understanding of the mathematical representation of PFSS and the solution in the finite-difference format.
We set the grid resolution of
The POT3D code adopts the FORTRAN programming language with MPI parallel programming on high-performance clusters for efficiency (Caplan et al., 2021). We run the code in the Chinese supercomputing Tianjin Tianhe No.1A cluster environment Linux system (Wang and Yuan, 2021) (more details about the computer center are available at https://www.nscc–tj.cn/). The POT3D code was installed and configured following the accompanying README instructions.
The input of this code is an approximate map of the photospheric magnetic field in HDF5 and free parameter variables in the DAT format. The output includes three components of the magnetic field with
2.2 The WSA model
By obtaining the magnetic field at the source surface, we can calculate
substituting
where
2.3 The solar wind speed at L1 extrapolated to the solar corona
With the PFSS model and the WSA empirical model, we can calculate the distribution of the solar wind velocity on the source surface of the corona. Before we compare the simulated
where
2.4 Statistical evaluation of continuous solar wind parameters
Statistical parameters such as the mean square error (MSE), root mean square error (RMSE), and correlation coefficient (CC) are selected for quantitative evaluation of the deduced solar wind parameters from the model.
The MSE reveals the error between the simulated data and the observed data (Allen, 1971), and the formula is
where N is the number of simulated values,
The RMSE reflects the error between simulated and observed values (Chai and Draxler, 2014). Its formula is expressed as
where N is the number of simulated values,
The CC represents the correlation between observed and simulated data (Asuero et al., 2006). The value of CC ranges from 1 to
where
3 Numerical result
Based on the aforementioned models, the 3D coronal magnetic field structures where the source surface was placed at heights/radii
3.1 The inversion of the coronal magnetic field on the source surface at different radii
By using the POT3D code, we can obtain the extrapolated coronal magnetic field components
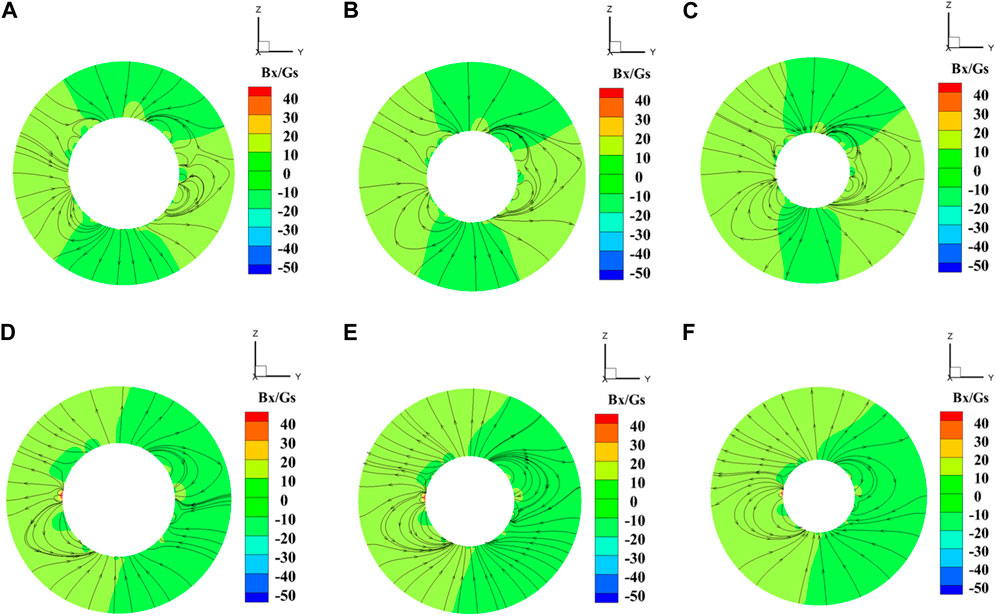
FIGURE 1. The calculated Bx components of the magnetic field for CR2069 at Rss = 2Rs (A), Rss = 2.5Rs (B), and Rss = 3Rs (C), and for CR2217 at Rss = 2Rs (D), Rss = 2.5Rs (E) and Rss = 3Rs (F). The black arrow line represents the magnetic line.
3.2 Calculations of the parameters at different
There are eight free parameters in the WSA model such as
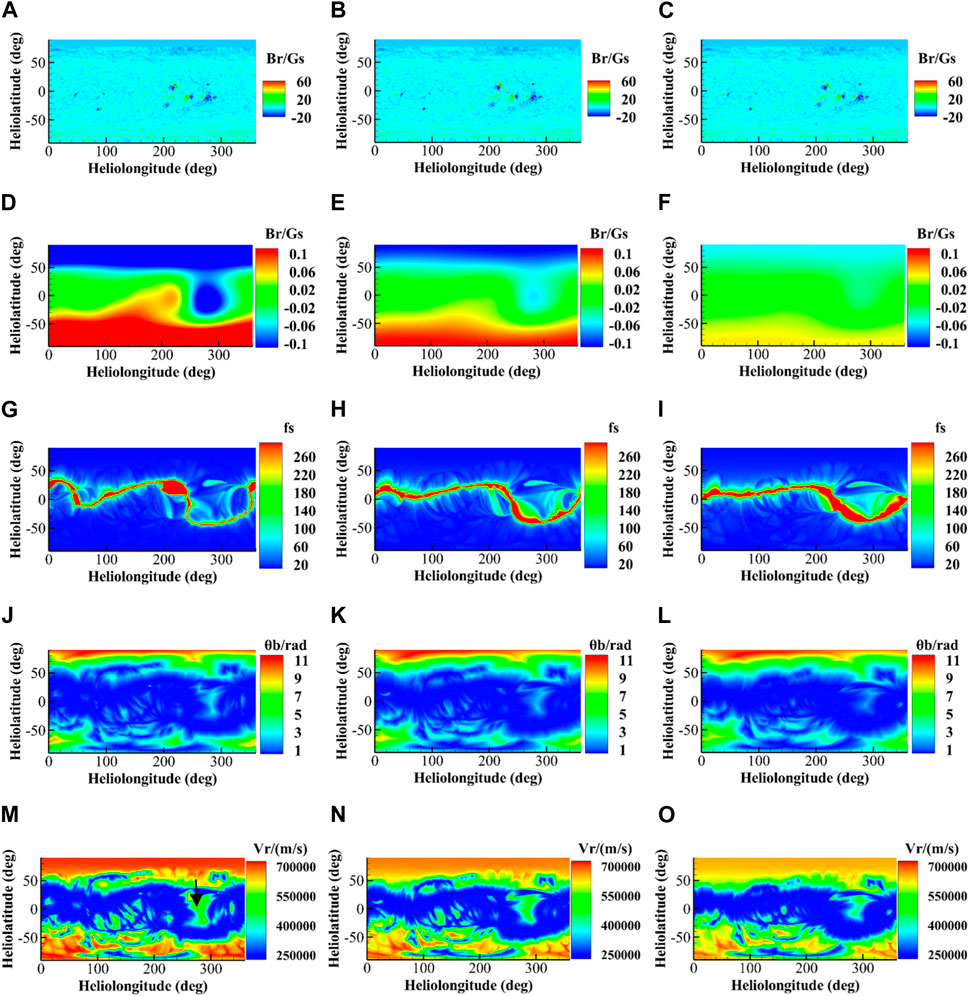
FIGURE 2. The solar wind parameters for CR2069. The abscissa is the longitude of the heliopause and the vertical coordinate is the latitude. The parameters from the first to fifth rows are the magnetic field Br at 1Rs (A–C), the magnetic field Br at 1Rss (D–F), the coronal magnetic field expansion factor fs (G–I), the minimum angular distance θb from the open magnetic line to the coronal hole (J–L), and the solar wind velocity Vr (M–O), respectively. The first column shows the parameters at Rss = 2Rs (A,D,G,J,M). The second column is the parameters when Rss = 2.5Rs (B,E,H,K,N). And the third column shows the parameters at Rss = 3Rs (C,F,I,L,O).
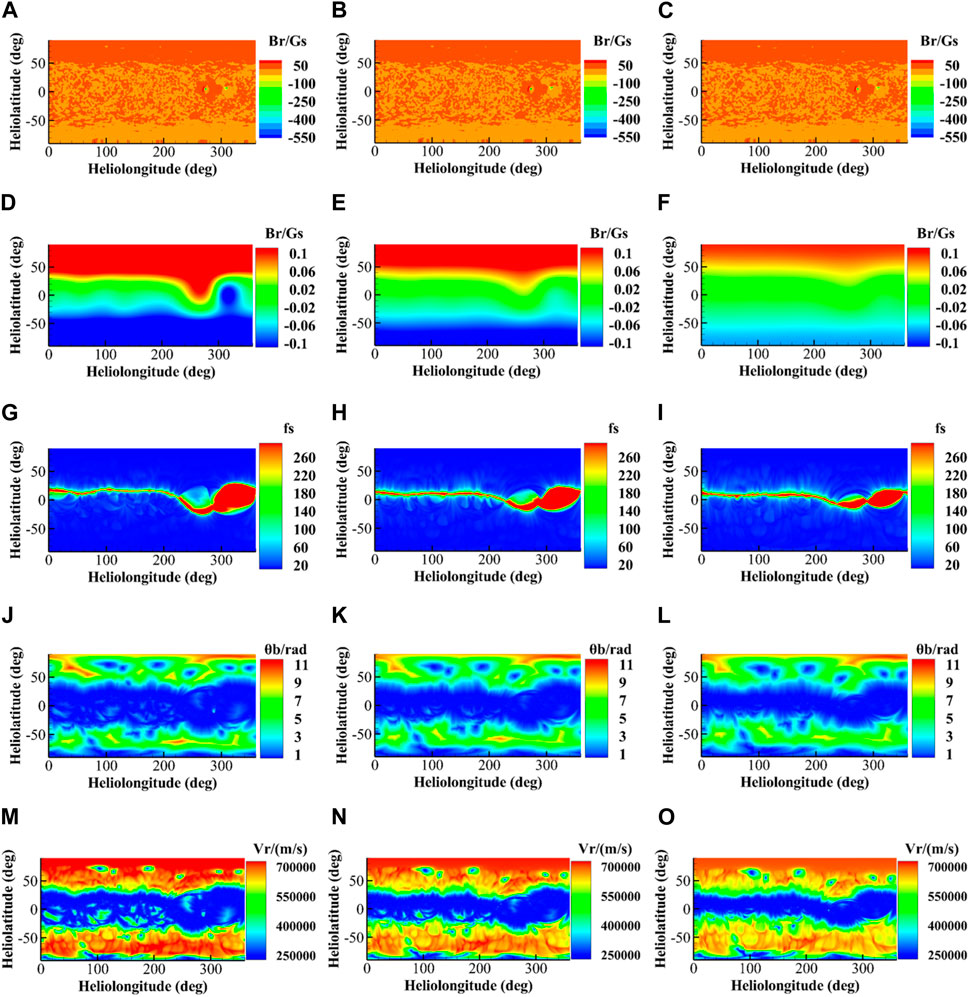
FIGURE 3. The solar wind parameters for CR2217. The abscissa is the longitude of the heliopause and the vertical coordinate is the latitude. The parameters from the first to fifth rows are the magnetic field Br at 1Rs (A–C), the magnetic field at (D–F), the coronal magnetic field expansion factor (G,–I), the minimum angular distance from the open magnetic line to the coronal hole (J–L), and the solar wind velocity Rss = 2Rs (M–O), respectively. The first column shows the parameters at (A,D,G,J,M). The second column is the parameters when Rss = 2.5Rs (B,E,H,K,N). And the third column shows the parameters at Rss = 3Rs (C,F,I,L,O).
In the fourth row of Figure 2, we can see that the magnetic field in the South Pole region extends north at longitude
Figures 2, 3 yield the following results:
(1) The magnetic field at the source surface will decrease with increasing
(2) The parameters
(3) The solar wind velocity,
3.3 Adjusting of WSA model parameters and their effects
According to a previous empirical model (Arge et al., 2003), the solar wind velocity
When the source surface radius
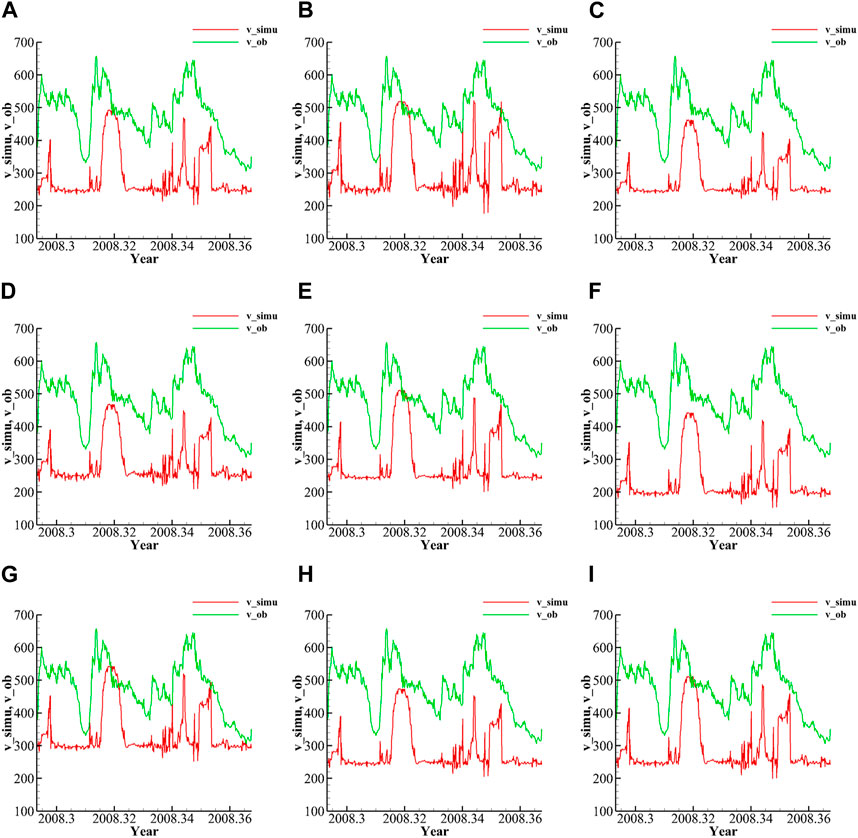
FIGURE 4. Comparison of the observed data and simulated results for different
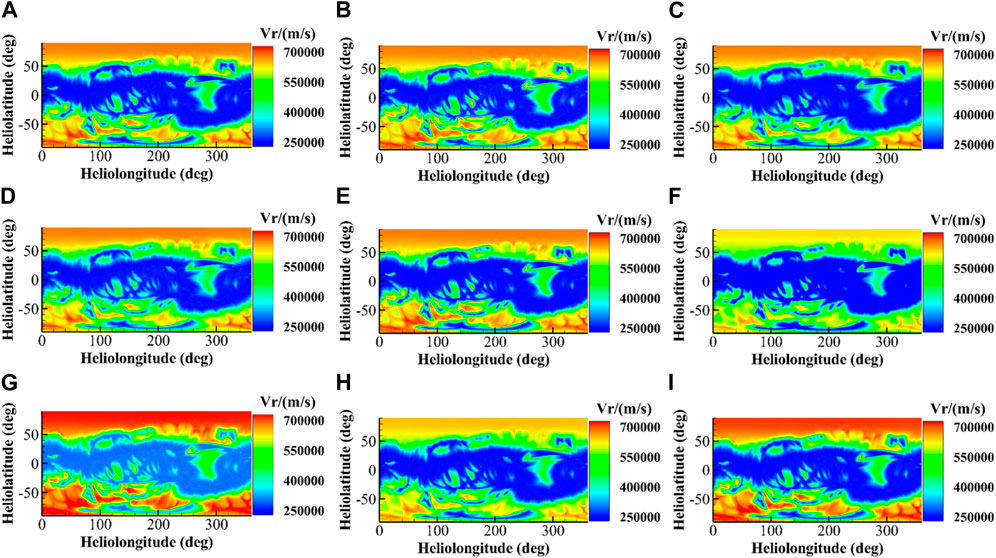
FIGURE 5. Simulated solar wind speed for different
Figures 4, 5 reveal the following results: (1) a decrease in
On the other hand, the variations in MSE, RMSE, and CC are monitored through adjusting different parameters (shown in Table 1). The simulation reveals the results as follows: (1). the decrease in
3.4 Parameter tuning and quantitative evaluation of the WSA model
In this section, for CR2069 the parameters for the source surface radius
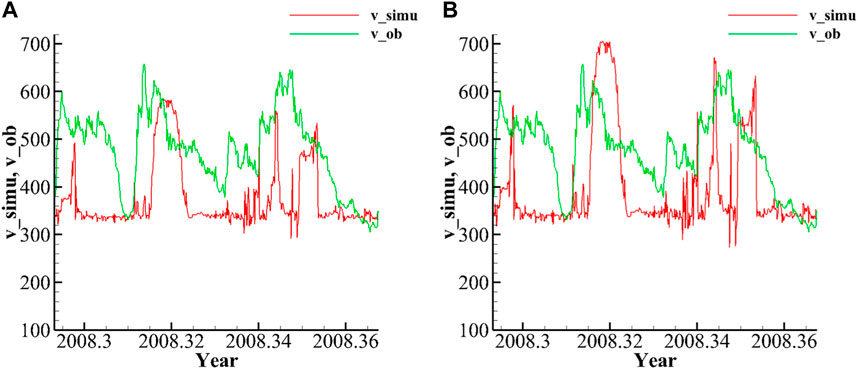
FIGURE 6. (A) Comparison of the observed solar wind speed with the simulated results with the adjustment of
To simplify the calculation, only parameters
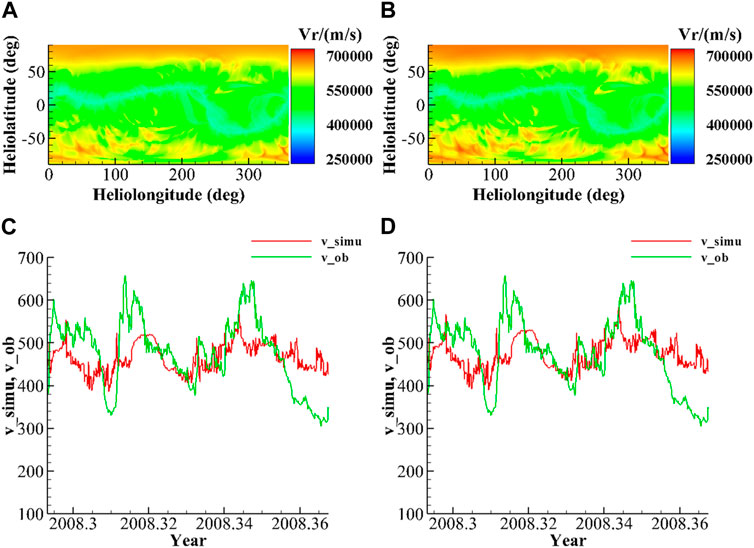
FIGURE 8. Simulated results through parameter tuning with
3.5 Effect of on the WSA simulation results
From the previous studies, it is clear that the height of the source surface in the coronal magnetic field model affects the values of
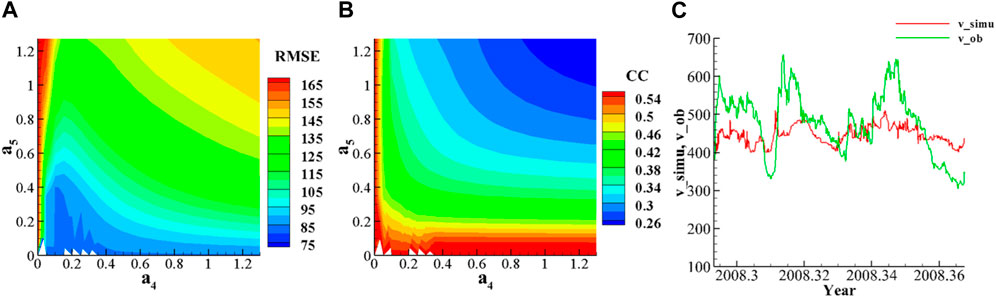
FIGURE 9. (A) When
When
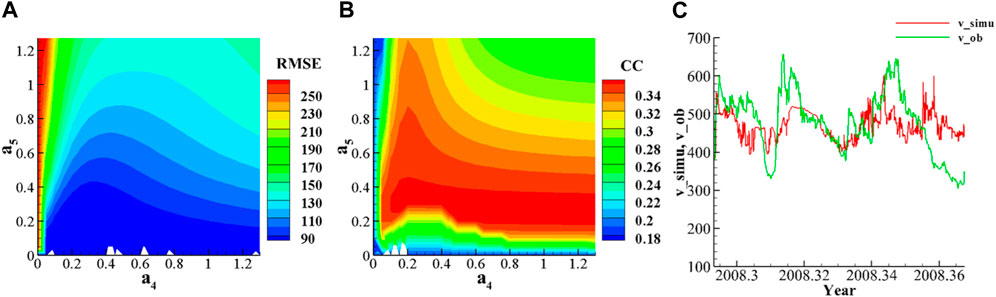
FIGURE 10. (A). When, Rss= 3Rs, the 2D distribution of RMSE for and a4 and a5 (B). The 2D distribution of CC for and a4 and a5 (C) When a4 = 0.25 and a5 = 0.15, the comparison of simulated solar wind speed and observation.
It can be seen that the surface heights of the three sources all reproduce the two high-speed flows well, but the peak values and the duration of high-speed flows are not the same. When
Then, the comparison for the MSE, RMSE, and CC at
Arge and Pizzo. (2000) used the WSA-2000 model to forecast the results for 3 years before and after 1996. The CC between the predicted and observed values is 0.4, and the average relative error is 15%. Owens et al. (2005) used the observed magnetic map of NWO and the PFSS + SCS model to forecast the results from 1995 to 2002. The results show that the RMSE of different years ranges from
4 Conclusion
In this paper, the PFSS–WSA solar wind model is investigated. This model consists of the PFSS coronal magnetic field extrapolation module and the WSA solar wind velocity module. The PFSS is implemented by the POT3D software package deployed on the Tianhe 1A supercomputer system. In our study, we use the GONG of CR2069 and CR2217 as an inner boundary condition to the PFSS model. It selects the source surface radii
First, we analyzed the effects of the four free parameters (
However, our current result is highly preliminary. The simple method used to extrapolate the observed solar wind to the corona still needs to account for more complex interaction processes that occur during the propagation of solar wind and the disturbances from CMEs/solar flares through the interplanetary space. Likewise, we need to consider the stream interaction region (SIR), which has some work to take into account (Jian et al., 2015; Li et al., 2019). In addition, we also find that the traditional diagnostic indices, such as RMSE, MSE, and CC, may not reflect the error quality of the forecast comprehensively. Therefore, it is necessary to introduce other error indicators to jointly constrain the tuning process in our simulated results. These are the issues that need further in-depth investigation and improvement in future works.
5 Data access
The photospheric magnetic field data of CR2069 and CR2217 are available from the GONG (https://gong.nso.edu). The observed speed of the solar wind at L1 point comes from the OMNI database run by NASA (http://omniweb.gsfc.nasa.gov). The POT3D software package comes from GitHub (https://github.com/predsci/POT3D).
Data availability statement
The original contributions presented in the study are included in the article/Supplementary Material; further inquiries can be directed to the corresponding author.
Author contributions
XZ performed data analysis and wrote the manuscript. SQ conceived this study and wrote the manuscript. WS was in charge of the organization and English editing of the whole manuscript. HY made some contributions on the discussions of the manuscript. All authors contributed to the article and approved the submitted version.
Funding
This work is supported by the National Natural Science Foundation of China (No. 41974178).
Acknowledgments
The authors acknowledge the use of a supercomputer system provided by the Tianhe 1A Center, Tianjin, China. The authors thank Dr. Huichao Li of Harbin Institute of Technology, Shenzhen, for his help on discussions.
Conflict of interest
The authors declare that the research was conducted in the absence of any commercial or financial relationships that could be construed as a potential conflict of interest.
Publisher’s note
All claims expressed in this article are solely those of the authors and do not necessarily represent those of their affiliated organizations, or those of the publisher, the editors, and the reviewers. Any product that may be evaluated in this article, or claim that may be made by its manufacturer, is not guaranteed or endorsed by the publisher.
References
Allen, D. M. (1971). Mean square error of prediction as a criterion for selecting variables. Technometrics 13, 469–475. doi:10.1080/00401706.1971.10488811
Altschuler, M. D., Levine, R. H., Stix, M., and Harvey, J. (1977). High resolution mapping of the magnetic field of the solar corona. Sol. Phys. 51 (2), 345–375. doi:10.1007/bf00216372
Altschuler, M. D., and Newkirk, G. (1969). Magnetic fields and the structure of the solar corona. Sol. Phys. 9 (1), 131–149. doi:10.1007/bf00145734
Arden, W. M., Norton, A. A., and Sun, X. (2014). A “breathing” source surface for cycles 23 and 24. J. Geophys. Res. Space Phys. 119 (3), 1476–1485. doi:10.1002/2013ja019464
Arge, C. N., Odstrcil, D., Pizzo, V. J., and Mayer, L. R. (2003). Improved method for specifying solar wind speed near the Sun. AIP Conf. Proc. 679 (1), 190–193. doi:10.1063/1.1618574
Arge, C. N., and Pizzo, V. J. (2000). Improvement in the prediction of solar wind conditions using near-real time solar magnetic field updates. J. Geophys. Res. Space Phys. 105 (5), 10465–10479. doi:10.1029/1999ja000262
Asuero, A. G., Sayago, A., and González, A. (2006). The correlation coefficient: an overview. Crit. Rev. Anal. Chem. 36 (1), 41–59. doi:10.1080/1040834050052-6766
Asvestari, E., Heinemann, S. G., Temmer, M., Pomoell, J., Kilpua, E., Magdalenic, J., et al. (2019). Reconstructing coronal hole areas with EUHFORIA and adapted WSA model: optimizing the model parameters. J. Geophys. Res. Space Phys. 124 (11), 8280–8297. doi:10.1029/2019ja027173
Asvestari, E., Heinemann, S. G., Temmer, M., Pomoell, J., Kilpua, E., Magdalenic, J., et al. (2020). The impact of coronal hole characteristics and solar cycle activity in reconstructing coronal holes with EUHFORIA. J. Phys. Conf. Ser. 1548 (1), 012004. doi:10.1088/1742-6596/1548/1/012004
Badman, S. T., Bale, S. D., Martínez Oliveros, J. C., Panasenco, O., Velli, M., Stansby, D., et al. (2020). Magnetic Connectivity of the Ecliptic Plane within 0.5 au: potential Field Source Surface Modeling of the First Parker Solar Probe Encounter. Astrophysical J. Suppl. Ser. 246 (2), 23. doi:10.3847/1538-4365/ab4da7
Baker, D. N. (2002). How to cope with space weather. Science 297 (5586), 1486–1487. doi:10.1126/science.1074956
Cao, E. (2012). Solar storms and their impact on human social activities. Spacecr. Environ. Eng. 29 (3), 237. doi:10.3969/j.issn.1673-1379.2012.03.001
Caplan, R., Downs, C., Linker, J., and Mikic, Z. (2021). Variations in finite–difference potential fields. Astrophysical J. 915, 44. doi:10.3847/1538-4357/abfd2f
Caplan, R. M., Downs, C., and Linker, J. A. (2016). Synchronic coronal hole mapping using multi-instrument EUV images: data preparation and detection method. Astrophysical J. 823, 53. doi:10.3847/0004-637x/823/1/53
Chai, T., and Draxler, R. R. (2014). Root mean square error (RMSE) or mean absolute error (MAE). Geosci. Model. Dev. Discuss. 7 (1), 1525–1534. doi:10.5194/gmd-7-1247-2014
Eastwood, J. P., Biffis, E., Hapgood, M. A., Green, L., Bisi, M. M., Bentley, R. D., et al. (2017). The economic impact of space weather: where do we stand? Risk Anal. 37 (2), 206–218. doi:10.1111/risa.12765
Feng, X., Li, C., Xiang, C., Zhang, M., Li, H., and Wei, F. (2017). Data-driven modeling of the solar corona by a new three-dimensional path-conservative osher–solomon MHD model. Astrophysical J. Suppl. Ser. 233 (1), 10. doi:10.3847/1538-4365/aa957a
Feng, X., Liu, X., Xiang, C., Li, H., and Wei, F. (2019). A new MHD model with a rotated-hybrid scheme and solenoidality-preserving approach. Astrophysical J. 871 (2), 226. doi:10.3847/1538-4357/aafacf
Feng, X. S., Xiang, C. Q., and Zhong, D. K. (2013). Numerical study of interplanetary solar storms (in Chinese). Sci. Sin. Terrae 43, 912–933. doi:10.1360/zd-2013-43-6-912
Feng, X. S., Xiang, C. Q., and Zhong, D. K. (2011). The state–of–art of three–dimensional numerical study for corona–interplanetary process of solar storms (in Chinese). Sci. Sin–Terrae 41, 1–28. doi:10.3724/SP.J.1011.2011.00181
Gressl, C., Veronig, A. M., Temmer, M., Odstrčil, D., Linker, J. A., Mikić, Z., et al. (2014). Comparative study of MHD modeling of the background solar wind. Sol. Phys. 289 (5), 1783–1801. doi:10.1007/s11207-013-0421-6
Hoeksema, J. T., and Scherrer, P. H. (1986). An atlas of photospheric magnetic field observations and computed coronal magnetic fields: 1976–1985. Sol. Phys. 105 (1), 205–211. doi:10.1007/bf00156388
Hoeksema, J. T., Wilcox, J. M., and Scherrer, P. H. (1982). Structure of the heliospheric current sheet in the early portion of Sunspot Cycle 21. J. Geophys. Res. Space Phys. 87 (12), 10331–10338. doi:10.1029/ja087ia12p10331
Hoeksema, J. T., Wilcox, J. M., and Scherrer, P. H. (1983). The structure of the heliospheric current sheet: 1978–1982. J. Geophys. Res. Space Phys. 88 (A12), 9910–9918. doi:10.1029/ja088ia12p09910
Jian, L. K., MacNeice, P. J., Taktakishvili, A., Odstrcil, D., Jackson, B., Yu, H. S., et al. (2015). Validation for solar wind prediction at Earth: comparison of coronal and heliospheric models installed at the CCMC. Space weather. 13 (5), 316–338. doi:10.1002/2015sw001174
Kruse, M., Heidrich-Meisner, V., and Wimmer-Schweingruber, R. F. (2021). Evaluation of a potential field source surface model with elliptical source surfaces via ballistic back mapping of in situ spacecraft data. Astronomy Astrophysics 645 (1), A83. doi:10.1051/0004-6361/202039120
Kruse, M., Heidrich-Meisner, V., Wimmer-Schweingruber, R., and Hauptmann, M. (2020), An elliptic expansion of the potential field source surface model.
Lee, C. O., Luhmann, J. G., Hoeksema, J. T., Sun, X., Arge, C. N., and de Pater, I. (2011). Coronal field opens at lower height during the solar cycles 22 and 23 minimum periods; IMF comparison suggests the source surface should Be lowered. Sol. Phys. 269 (2), 367–388. doi:10.1007/s11207-010-9699-9
Levine, R. H., Schulz, M., and Frazier, E. N. (1982). Simulation of the magnetic structure of the inner heliosphere by means of a non-spherical source surface. Sol. Phys. 77 (1), 363–392. doi:10.1007/bf00156118
Li, H. C., Feng, X. S., and Xiang, C. Q. (2019). Time–dependments simulation and result validation of interplanetary solar wind. Chin. J. Geophys. 62 (1), 1–18. doi:10.6038/cjg2019L0625
Mackay, D. H., and Yeates, A. R. (2012). The sun’s global photospheric and coronal magnetic fields: observations and models. Living Rev. Sol. Phys. 9 (1), 6. doi:10.12942/lrsp-2012-6
Mikić, Z., Downs, C., Linker, J. A., Caplan, R. M., Mackay, D. H., Upton, L. A., et al. (2018). Predicting the corona for the 21 August 2017 total solar eclipse. Nat. Astron. 2 (11), 913–921. doi:10.1038/s41550-018-0562-5
Nikolj, L., and Trichtchenko, L. (2012). Development of coronal field and solar wind components for MHD interplanetary simulations, world Academy of science, engineering and technology. Int. J. Environ. Chem. Ecol. Geol. Geophys. Eng. 6, 698–702. doi:10.5281/zenodo.1062642
Owens, M. J., Arge, C. N., Spence, H. E., and Pembroke, A. (2005). An event-based approach to validating solar wind speed predictions: high-speed enhancements in the Wang-Sheeley-Arge model. J. Geophys. Res. Space Phys. 110 (12), A12105. doi:10.1029/2005ja011343
Panasenco, O., Velli, M., D’Amicis, R., Shi, C., Réville, V., Bale, S. D., et al. (2020). Exploring Solar Wind Origins and Connecting Plasma Flows from the Parker Solar Probe to 1 au: nonspherical Source Surface and Alfvénic Fluctuations. Astrophysical J. Suppl. Ser. 246 (2), 54. doi:10.3847/1538-4365/ab61f4
Parker, E. N. (1958). Dynamics of the interplanetary gas and magnetic fields. Astrophysical J. 128, 664. doi:10.1086/146579
Riley, P., Baker, D., Liu, Y. D., Verronen, P., Singer, H., and Güdel, M. (2018). Extreme space weather events: from cradle to grave. Space Sci. Rev. 214 (1), 21–24. doi:10.1007/s11214-017-0456-3
Riley, P., Linker, J. A., and Arge, C. N. (2015). On the role played by magnetic expansion factor in the prediction of solar wind speed. Space weather. 13, 154–169. doi:10.1002/2014sw001144
Riley, P., Linker, J. A., Mikić, Z., Lionello, R., Ledvina, S. A., and Luhmann, J. G. (2006). A comparison between global solar magnetohydrodynamic and potential field source surface model results. Astrophysical J. 653 (2), 1510–1516. doi:10.1086/508565
Robinson, R. M., and Behnke, R. A. (2001). The U.S. National space weather program: a retrospective. Geophys. Monogr. Ser. 2001, 1–10. doi:10.1029/GM125p0001
Sahade, A., Cécere, M., and Krause, G. (2020). Influence of coronal holes on CME deflections: numerical study. Astrophysical J. 896 (1), 53. doi:10.3847/1538-4357/ab8f25
Schatten, K. H., Wilcox, J. M., and Ness, N. F. (1969). A model of interplanetary and coronal magnetic fields. Sol. Phys. 6 (3), 442–455. doi:10.1007/bf00146478
Schulz, M., Frazier, E. N., and Boucher, D. J. (1978). Coronal magnetic-field model with non-spherical source surface. Sol. Phys. 60 (1), 83–104. doi:10.1007/bf00152334
Schulz, M. (1997). Non-spherical source-surface model of the heliosphere: a scalar formulation. Ann. Geophys. 15 (11), 1379–1387. doi:10.1007/s00585-997-1379-1
Schwenn, R. (2006). Space weather: the solar perspective. Living Rev. Sol. Phys. 3 (1), 2. doi:10.12942/lrsp-2006-2
Sun, X., and Hoeksema, J. T. (2009). A new source surface radius in potential field modeling during the current weak solar minimum? arXiv.
Thompson, W. (2006). Coordinate systems for solar image data. Astronomy Astrophysics 449, 791–803. doi:10.1051/0004-6361:20054262
Tóth, G., van der Holst, B., and Huang, Z. (2011). Obtaining potential field solutions with spherical harmonics and finite differences. Astrophysical J. 732 (2), 102. doi:10.1088/0004-637x/732/2/102
Wang, Y. L., and Yuan, L. (2021). Tianhe bright interviewed meng xiangfei, assistant director of national supercomputing Tianjin center. Talents 01, 32–35.
Wang, Y. M., Robbrecht, E., and Sheeley, N. R. (2009). ON the weakening of the polar magnetic fields during solar cycle 23. Astrophysical J. 707 (2), 1372–1386. doi:10.1088/0004-637x/707/2/1372
Wang, Y. M., and Sheeley, N. R. (1990). Solar wind speed and coronal flux–tube expansion. Astrophysical J. 355, 726. doi:10.1086/168805
Keywords: solar wind, coronal magnetic field, numerical simulation, WSA solar wind model, PFSS model
Citation: Zhang X, Qiu S, Soon W and Yousof H (2023) Three-dimensional inversion of corona structure and simulation of solar wind parameters based on the photospheric magnetic field deduced from the Global Oscillation Network Group. Front. Astron. Space Sci. 10:1234391. doi: 10.3389/fspas.2023.1234391
Received: 04 June 2023; Accepted: 27 July 2023;
Published: 21 August 2023.
Edited by:
Vaibhav Pant, Aryabhatta Research Institute of Observational Sciences, IndiaReviewed by:
Zhongwei Yang, Chinese Academy of Sciences (CAS), ChinaXinhua Zhao, Chinese Academy of Sciences (CAS), China
Copyright © 2023 Zhang, Qiu, Soon and Yousof. This is an open-access article distributed under the terms of the Creative Commons Attribution License (CC BY). The use, distribution or reproduction in other forums is permitted, provided the original author(s) and the copyright owner(s) are credited and that the original publication in this journal is cited, in accordance with accepted academic practice. No use, distribution or reproduction is permitted which does not comply with these terms.
*Correspondence: Shican Qiu, c2NxQHVzdGMuZWR1LmNu