- 1Space Sciences Laboratory, University of California, Berkeley, CA, United States
- 2Air Force Research Laboratory, Kirtland AFB, Albuquerque, NM, United States
In the first part of this work, we highlighted a drift-diffusion equation capable of resolving the magnetic local time dimension when describing the effects of trapped particle transport on radiation belt intensity. Here, we implement these general considerations in a special case. Specifically, we determine the various transport and diffusion coefficients required to solve the drift-diffusion equation for equatorial electrons drifting in a dipole magnetic field in the presence of a specific model of time-varying electric fields. Random electric potential fluctuations, described as white noise, drive fluctuations of trapped particle drift motion. We also run a numerical experiment that consists of tracking trapped particles’ drift motion. We use the results to illustrate the validity of the drift-diffusion equation by showing agreement in the solutions. Our findings depict how a structure initially localized in magnetic local time generates drift-periodic signatures that progressively dampen with time due to the combined effects of radial and azimuthal diffusions. In other words, we model the transition from a drift-dominated regime, to a diffusion-dominated regime. We also demonstrate that the drift-diffusion equation is equivalent to a standard radial diffusion equation once the distribution function is phase-mixed. The drift-diffusion equation will allow for radiation belt modeling with a better spatiotemporal resolution than radial diffusion models once realistic inputs, including localized transport and diffusion coefficients, are determined.
1 Introduction
The effects of trapped particle spatial transport on radiation belt intensity are usually described by the radial diffusion paradigm. According to this model, in the absence of any other process besides spatial transport, the time evolution of radiation belt intensity is described by a one-dimensional diffusion equation:
where
where
In the following, we specify the field and particle characteristics assumed to compute the transport and diffusion coefficients introduced in Eq. 2 in a special case. For the sake of simplicity, we focus on the magnetic equator and assume dipolar magnetic field lines thereafter. In this context,
2 Theoretical setup
The objective of this section is to show how to determine the localized transport (
2.1 Fields
We assume a magnetic dipole field,
where
where
Characteristics of the electric fluctuation: The electric fluctuation,
The standard deviation of the white noise,
2.2 Trapped particles
2.2.1 Computation of the localized transport and diffusion coefficients
The objective of this Section is to determine
where
In our case, the time interval,
The equations for the drift motion of equatorial particles trapped in the fields described in Section 2.1 are:
where
where
Using Eq. 8, the general expressions for the total variations in radial and azimuthal locations after a time interval
We consider a time interval,
With the definition provided Eq. 6, the MLT-localized diffusion coefficients are:
We note that the diffusion coefficients provided in Eq. 12 are functions of magnetic local time,
The diffusion coefficients provided in Eq. 12 are also proportional to
2.2.2 Contextualization using Hamiltonian equations
We expect a relationship between the first and second moments characterizing transport. Indeed, assuming small variations over the course of a couple of bounce periods, we have shown in the first part of this work (Lejosne and Albert, 2023) that:
where
A second-order Taylor expansion of Eq. 10 yields
Leveraging Eqs 9, 11 and 15, it is straightforward to verify Eq. 14. A notable consequence of this result is that:
when the drift phase is resolved. In other words, the commonly assumed relationship between the first and second moments of radial transport,
3 On the drift-diffusion equation
3.1 Equivalence with a radial diffusion equation in the case of an azimuthally symmetric distribution function
We leverage the coefficients computed in Section 2.2 to demonstrate that Eq. 2 is like a radial diffusion equation (Eq. 1) when the distribution function is independent of MLT, i.e., when
when
Leveraging Equations 9, 12 yields:
Averaging over all MLT-phases, we have that:
Introducing the drift-averaged diffusion coefficient,
Equation 20 also becomes:
We emphasize that
Thus, we have shown how the drift-diffusion Eq. 2 relates to the standard radial diffusion Eq. 1 when the distribution function is phase-mixed (i.e., independent of MLT). We have also shown that the corresponding radial diffusion coefficient,
3.2 Change of variables to remove the cross terms
The drift-diffusion equation (Eq. 2) contains cross-terms
This means that 0 is an eigenvalue of the diffusion matrix, and there exists a system of coordinates in which the diffusive part of the drift-diffusion equation is one-dimensional. We introduce a new set of variables:
which corresponds to a conversion from polar to Cartesian coordinates. In this coordinate system, with the values of the diffusion coefficients provided Eq. 12, Eq. 2 becomes:
with
Looking back at the drift motion equations (Eq. 8), we notice that the perturbation of the drift velocity is indeed along the
In the following, we assume
This latest equation is the one used for numerical implementation, as discussed in Section 4.
4 Numerical simulations
4.1 Numerical setups and methods
Parameters: Since this work assumes electric potential fluctuations in a time-stationary dipole field, we focus on a region where this is most likely to happen, namely, the inner belt and slot region (below L = 4). We consider populations that have been associated with drift period structures in this region, i.e., electrons in the tens to hundreds of keV energy range (e.g., Ukhorskiy et al., 2014). Specifically, we focus on equatorial electrons with kinetic energy of 200 keV at
Method for particle tracking: We solve Eq. 8 to determine trapped particle drift motion. We launch particles in many different sequences of outcomes for
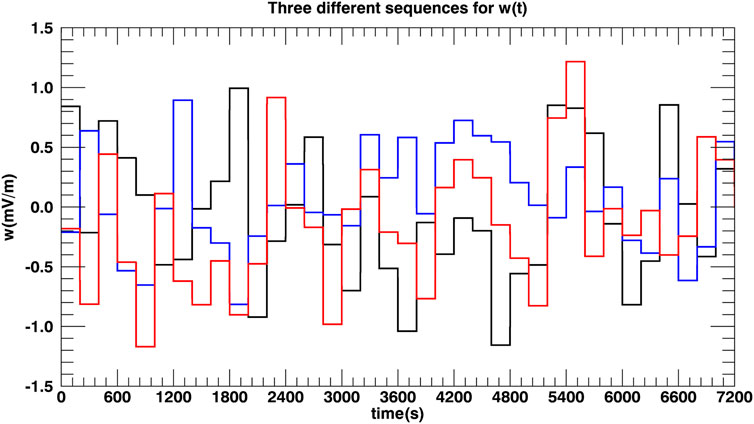
FIGURE 1. Three different sequences of randomly generated outcomes for the white noise signal,
Initial condition: To solve numerically Eq. 28, we consider a simple initial condition, assuming that:
- Particles are present homogeneously at all MLTs at
- Particles are also present homogeneously in an area mimicking a localized injection, extending from
The distribution function,
Method for numerical simulation: To solve numerically Eq. 28, we use an operator splitting method. At each time step, we first solve the transport part of the equation, using the method of characteristics. We then use the updated function to solve the diffusive part of the equation, using an explicit scheme for the sake of simplicity. We record the value of the distribution function,
4.2 Results
4.2.1 Comparison between the results of the test particle experiments and the solution of the drift-diffusion equation
We compare: a) the outputs of the particle tracking experiment with b) the solution of Eq. 28. First, we focus on one location (L = 3.5 ± 0.05 and 22:00 MLT ± 00:15): We record the time evolution of the distribution functions derived from the drift-diffusion equation and from the particle tracking experiment. The results, presented in Figure 2, highlight the consistency of the two approaches. The location is initially out of the artificial injection region thus
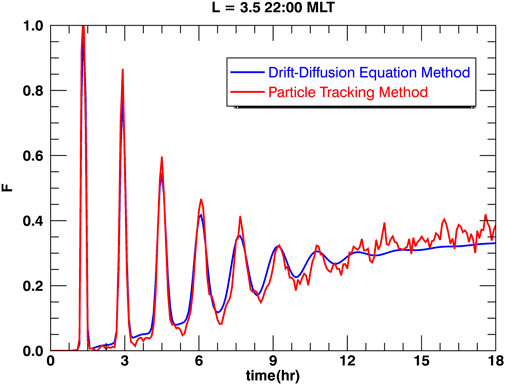
FIGURE 2. Time evolution of the distribution function centered at L = 3.5 and 22:00 MLT. The magnitude of the flux oscillation characteristic of trapped particle injections decreases with time until it vanishes after a time characteristic of phase mixing. This figure compares numerical results from (in red) a test particle simulation and (in blue) the solution of the drift-diffusion equation. It illustrates the transition from a drift-dominated regime (with the presence of drift periodic oscillations in the distribution function) to a diffusion-dominated regime (characterized by a slow and steady variation of the distribution function).
4.2.2 Visualization of the solution of the drift-diffusion equation
A 2D video of the simulation run for the solution of the drift-diffusion equation over a 24 h interval is provided in Supplementary Material. Two screenshots (at t = 3:00 h and t = 10:00 h) are provided in Figure 3.
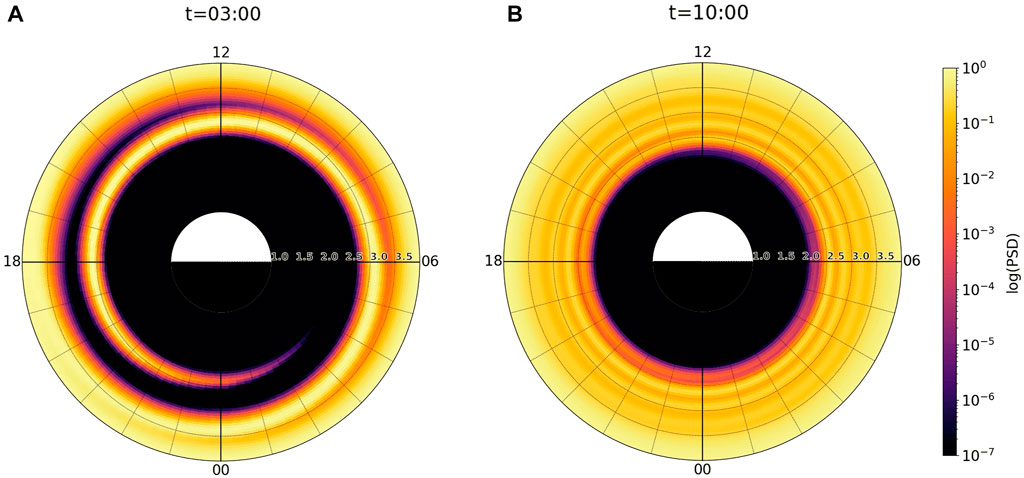
FIGURE 3. Time evolution of a distribution function (initially centered around 00:00 MLT for
From the video, it is clear that the effect of the azimuthal drift on radiation belt intensity is at first more striking than the effects of radial and azimuthal diffusion. That said, drift alone would only lead to trajectories wrapping around, meaning that the distribution function would only become more structured. Radial and azimuthal diffusions act to smooth out the MLT-dependent structure, dampening it until it disappears and the distribution becomes independent of MLT. This phase-mixing process is consistent with observations. It allows for the transition from a drift-dominated regime to a diffusion-dominated regime.
5 Conclusion
We have shown how the drift-diffusion equation is capable of modeling phase mixing, allowing for a transition from drift-resolved structures (e.g., drift-periodic fluctuations associated with MLT-localized sources or losses) to the standard radial diffusion framework. We illustrated our case using simple assumptions, focusing on the magnetic equator of a dipole field and modeling electric potential fluctuations by a white noise. A next step of physical importance is to model the effect of the thermospheric wind driven electric field fluctuations on radiation belt dynamics. Indeed, electric potential fluctuations are present in the inner belt. They are viewed as the primary driver of radial diffusion in this region (e.g., O’Brien et al., 2016). In particular, electric fluctuations associated with quiet time wind dynamo have significant day-to-day variability, even during geomagnetically quiet periods (e.g., Fejer, 1993). Thermospheric wind driven electric fields are also known to shape the inner belt drift shells (Lejosne et al., 2021). Future work should consist of determining a more realistic form for the electric perturbation using information on thermospheric wind driven electric field fluctuations. Going back to the general expression of the drift-diffusion equation, future work should also consist of determining the various transport and diffusion coefficients in the presence of a time varying magnetic field. Once realistic inputs, including localized transport and diffusion coefficients, are determined, the drift-diffusion equation will enable operational radiation belt modeling with a better spatiotemporal resolution than current radial diffusion models.
Data availability statement
The raw data supporting the conclusion of this article will be made available by the authors, without undue reservation.
Author contributions
We describe contributions to the paper using the CRediT (Contributor Roles Taxonomy) categories (Brand et al., 2015). Conceptualization, Writing—Original Draft: SL and JA Writing—Review and Editing: All authors. Visualization: SL and SW. All authors contributed to the article and approved the submitted version.
Funding
SL work was performed under NASA Grant Awards 80NSSC18K1223 and 80NSSC20K1351. SW work was performed under NASA Grant Award 80NSSC20K1351. JA was supported by NASA grant 80NSSC20K1270, AFOSR grant 22RVCOR002, and the Space Vehicles Directorate of the Air Force Research Laboratory.
Conflict of interest
The authors declare that the research was conducted in the absence of any commercial or financial relationships that could be construed as a potential conflict of interest.
Publisher’s note
All claims expressed in this article are solely those of the authors and do not necessarily represent those of their affiliated organizations, or those of the publisher, the editors and the reviewers. Any product that may be evaluated in this article, or claim that may be made by its manufacturer, is not guaranteed or endorsed by the publisher.
Author disclaimer
The views expressed are those of the authors and do not reflect the official guidance or position of the United States Government, the Department of Defense or of the United States Air Force. The appearance of external hyperlinks does not constitute endorsement by the United States Department of Defense (DoD) of the linked websites, or the information, products, or services contained therein. The DoD does not exercise any editorial, security, or other control over the information you may find at these locations.
Supplementary material
The Supplementary Material for this article can be found online at: https://www.frontiersin.org/articles/10.3389/fspas.2023.1232512/abstract#supplementary-material
References
Albert, J. M. (2018). Diagonalization of diffusion equations in two and three dimensions. J. Atmos. Solar-Terrestrial Phys. 177, 202–207. doi:10.1016/j.jastp.2017.08.008
Albert, J. M., and Young, S. L. (2005). Multidimensional quasi-linear diffusion of radiation belt electrons. Geophys. Res. Lett. 32, L14110. doi:10.1029/2005GL023191
Birmingham, T. J., Northrop, T. G., and Fälthammar, (1967). Charged particle diffusion by violation of the third adiabatic invariant. Phys. Fluids 10, 2389. Number 11. doi:10.1063/1.1762048
Brand, A., Allen, L., Altman, M., Hlava, M., and Scott, J. (2015). Beyond authorship: Attribution, contribution, collaboration, and credit. Learn. Pub. 28, 151–155. doi:10.1087/20150211
Fälthammar, C.-G. (1968), Radial diffusion by violation of the third adiabatic invariant, in Earth’s particles and fields. B. M. McCormac (New York: Reinhold), 157
Fejer, B. G. (1993). F region plasma drifts over Arecibo: Solar cycle, seasonal, and magnetic activity effects. J. Geophys. Res. 98 (8), 13645–13652. doi:10.1029/93JA-00953
Lejosne, S., Fedrizzi, M., Maruyama, N., and Selesnick, R. S. (2021). Thermospheric neutral winds as the cause of drift shell distortion in Earth’s inner radiation belt. Front. Astron. Space Sci. 8, 725800. doi:10.3389/fspas.2021.725800
Lejosne, S., and Albert, J. M. (2023). Drift phase resolved diffusive radiation belt model: 1. Theoretical framework. Front. Astron. Space Sci. 10, 1200485. doi:10.3389/fspas.2023.1200485
Lejosne, S., and Kollmann, P. (2020). Radiation belt radial diffusion at Earth and beyond. Space Sci. Rev. 216, 19. doi:10.1007/s11214-020-0642-6
O'Brien, T. P., Claudepierre, S. G., Guild, T. B., Fennell, J. F., Turner, D. L., Blake, J. B., et al. (2016). Inner zone and slot electron radial diffusion revisited. Geophys. Res. Lett. 43, 7301–7310. doi:10.1002/2016GL069749
Roederer, J. G. (1970). Dynamics of geomagnetically trapped radiation. Heidelberg: Springer Berlin. doi:10.1007/978-3-642-49300-3
Schulz, M., and Lanzerotti, L. J. (1974). Particle diffusion in the radiation belts. Berlin: Springer. doi:10.1007/978-3-642-65675-0
Selesnick, R. S. (2012). Atmospheric scattering and decay of inner radiation belt electrons. J. Geophys. Res. 117, A08218. doi:10.1029/2012JA017793
Tao, X., Albert, J. M., and Chan, A. A. (2009). Numerical modeling of multidimensional diffusion in the radiation belts using layer methods. J. Geophys. Res. 114, A02215. doi:10.1029/2008JA013826
Tao, X., Chan, A. A., Albert, J. M., and Miller, J. A. (2008). Stochastic modeling of multidimensional diffusion in the radiation belts. J. Geophys. Res. 113, A07212. doi:10.1029/2007JA012985
Tao, X., Zhang, L., Wang, C., Li, X., Albert, J. M., and Chan, A. A. (2016). An efficient and positivity-preserving layer method for modeling radiation belt diffusion processes. J. Geophys. Res. Space Phys. 121, 305–320. doi:10.1002/2015JA022064
Ukhorskiy, A., Sitnov, M., Mitchell, D., Takahashi, K., Lanzerotti, L. J., and Mauk, B. H. (2014). Rotationally driven ‘zebra stripes’ in Earth’s inner radiation belt. Nature 507, 338–340. doi:10.1038/nature13046
Whipple, E. C. (1978). U, B, K) coordinates: A natural system for studying magnetospheric convection. J. Geophys. Res. 83 (A9), 4318–4326. doi:10.1029/JA083iA09p04318
Appendix
We explain how we derived the expressions for
A first-order Taylor expansion for the expressions of the total variations in radial and azimuthal locations (Eq. 10) yields:
where
The expression for the ensemble average of the square of the total variation of the radial displacement is:
Since
Since the signal w is a piecewise constant function, we have that:
And by definition of the white noise sequence,
As a result:
A similar approach allows for a computation of
where
The formula provided Eq. A6 is not dependent on the energy of the particles considered, provided that the drift period is very long in comparison with the updating time,
Glossary
Keywords: radiation belts, fokker-planck equation, adiabatic invariants, radial transport, radial diffusion, azimuthal diffusion, cross-terms, electric fields
Citation: Lejosne S, Albert JM and Walton SD (2023) Drift phase resolved diffusive radiation belt model: 2. implementation in a case of random electric potential fluctuations. Front. Astron. Space Sci. 10:1232512. doi: 10.3389/fspas.2023.1232512
Received: 31 May 2023; Accepted: 13 July 2023;
Published: 08 August 2023.
Edited by:
Qianli Ma, Boston University, United StatesReviewed by:
Greg Cunningham, Los Alamos National Laboratory (DOE), United StatesXin Tao, University of Science and Technology of China, China
Copyright © 2023 Lejosne, Albert and Walton. This is an open-access article distributed under the terms of the Creative Commons Attribution License (CC BY). The use, distribution or reproduction in other forums is permitted, provided the original author(s) and the copyright owner(s) are credited and that the original publication in this journal is cited, in accordance with accepted academic practice. No use, distribution or reproduction is permitted which does not comply with these terms.
*Correspondence: Solène Lejosne, solene@berkeley.edu