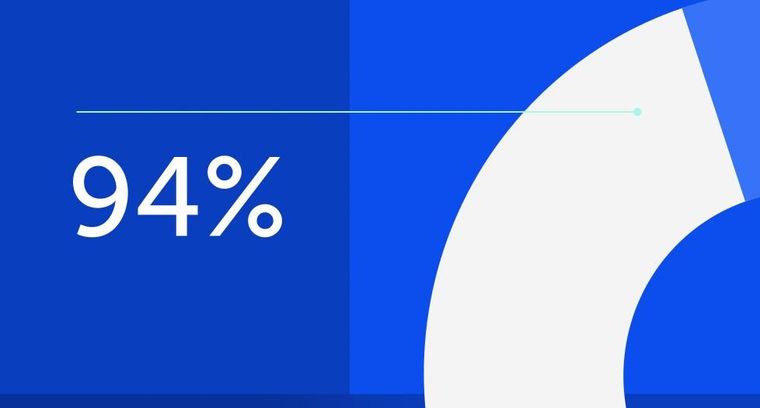
94% of researchers rate our articles as excellent or good
Learn more about the work of our research integrity team to safeguard the quality of each article we publish.
Find out more
PERSPECTIVE article
Front. Astron. Space Sci., 13 July 2023
Sec. Space Physics
Volume 10 - 2023 | https://doi.org/10.3389/fspas.2023.1203598
This article is part of the Research TopicThe Future of Space Physics 2022View all 66 articles
In the domain of space science, numerous ground-based and space-borne data of various phenomena have been accumulating rapidly, making analysis and scientific interpretation challenging. However, recent trends in the application of artificial intelligence (AI) have been shown to be promising in the extraction of information or knowledge discovery from these extensive data sets. Coincidentally, preparing these data for use as inputs to the AI algorithms, referred to as AI-readiness, is one of the outstanding challenges in leveraging AI in space science. Preparation of AI-ready data includes, among other aspects: 1) collection (accessing and downloading) of appropriate data representing the various physical parameters associated with the phenomena under study from different repositories; 2) addressing data formats such as conversion from one format to another, data gaps, quality flags and labeling; 3) standardizing metadata and keywords in accordance with NASA archive requirements or other defined standards; 4) processing of raw data such as data normalization, detrending, and data modeling; and 5) documentation of technical aspects such as processing steps, operational assumptions, uncertainties, and instrument profiles. Making all existing data AI-ready within a decade is impractical and data from future missions and investigations exacerbates this. This reveals the urgency to set the standards and start implementing them now. This article presents our perspective on the AI-readiness of space science data and mitigation strategies including definition of AI-readiness for AI applications; prioritization of data sets, storage, and accessibility; and identifying the responsible entity (agencies, private sector, or funded individuals) to undertake the task.
Space science is characterized by the abundance of observational data acquired by spacecraft and ground-based instruments. For decades, statistical methods have been indispensable for the analysis and interpretation of these data. With the advancement of technology, these data are ever increasing in volume and diversity, and it is becoming impractical to extract useful scientific information from these vast volumes (terabytes and petabytes) of data with traditional methods. However, the implementation of artificial intelligence (AI) in the space sciences have shown to be a powerful tool for data analysis and data mining with predictive capability. AI methods such as machine learning (ML) and neural networks (NN) are built on advanced statistical methods and data science (DS), and have proven to be greatly successful in augmenting physics-based and empirical modeling, and data analysis (e.g., Lundstedt, 1996; Wintoft and Lundstedt, 1997; Bobra and Couvidat, 2015; Ansdell et al., 2018; McGranaghan et al., 2018; Shallue and Vanderburg, 2018; Camporeale, 2019; Camporeale et al., 2019; Barros et al., 2020; Camporeale and SOC-ML-Helio, 2020; Nikolaou et al., 2020; Osborn et al., 2020; Armstrong et al., 2021; Azari et al., 2021; de Beurs et al., 2021; McGranaghan et al., 2021; Himes et al., 2022; Wing et al., 2022). This includes, but is not limited to, methods such as time series analysis, segmentation, Bayesian methods, probabilistic inference, information theory, and surrogate modeling. These methods are critical for future scientific findings and discoveries. While the interpretability and explainability of the AI models built on various techniques are still being explored and established, AI and DS are revolutionizing the way scientific problems in the space physics are conceptualized and addressed.
A review of these methods as applied to the space sciences has been carried out in the form of a virtual international conference, “Applications of Statistical Methods and Machine Learning in the Space Sciences” organized by the Space Science Institute (SSI) during 17–21 May 2021 (http://spacescience.org/workshops/mlconference2021.php). This multidisciplinary conference brought together experts in various fields to compare and contrast AI and statistical methods and to assess the needs of different space science subfields. The conference proceedings are published as a Frontiers topical collection (Poduval et al., 2023).
The highlight of the conference was the discussion sessions designated to handle different topics. “AI-readiness” (defined and discussed in detail in Section 3) of the various spacecraft data was one of the topics common to all the 45-min discussion sessions each day. Topics such as availability and easy access to various data sets, data preprocessing, and metadata guidelines were a few of the main aspects discussed. Inspired by these preliminary discussions and our understanding of the significance of the issues related to accessing the various data sets and their (pre)processing in the context of AI applications, we explored these aspects in greater detail after the conference which resulted in a multi-authored white paper (Poduval et al., 2022), “AI-ready Data in Solar Physics and Space Science: Concerns, Mitigation and Recommendations”, submitted to the National Academies of Science, Engineering, and Medicine’s Decadal Survey for Solar and Space Physics (Heliophysics) 2024–2033. In the article presented here, we summarize the major recommendations to the community such as known problems with accessing existing data and ways of addressing them efficiently in a cost-effective manner with the aim of providing repositories of AI-ready data in all domains of space science within the next decade.
While AI application is the main driving need behind AI-ready data, processed data sets of this nature and access methods are also useful for many broader applications (including scientific investigations using conventional methods) that benefit from increased data accessibility and unified formats for scientific applications utilizing space science data. As AI/ML techniques are expected to become a common practice in the space sciences in the coming decades, (Figure 1 in Azari et al., 2021), a clearly defined standard would prove valuable to all space science disciplines. Due to the wide range of applicability of ML methods in addressing scientific problems in all the fields of space science—especially space weather and related studies as evidenced by the many works cited in Section 1—we are not discussing specific science goals in this article.
Researchers in the space sciences implementing AI methods have encountered several difficulties with the existing data sets. As discussed at the SSI virtual conference (Poduval et al., 2023) and other meetings in space science, getting the existing data organized, standardized, and easily accessible for implementing AI methods is a major challenge. We argue that while these data are publicly available, using them for AI applications requires considerable effort by individual researchers pursuing a specific science question. In this section, we compiled the common problems encountered while using these data for implementing AI methods. Similar problems and limitations exist in ground-based data and in the data from other domains such as atmospheric sciences, astronomy and cosmology. These and other related problems call for focused studies on the existing barriers to utilizing these data as well as the development of a well documented, consistent set of data easily accessible to the scientific community in the near future.
Methods of AI and DS often require very large data sets to obtain statistically reliable results and are often intolerant of missing data. Angryk et al. (2020) have carried out an extensive study to homogenize data and eliminate data gaps, and created a set of multivariate time series data from the Space Weather HMI Active Region Patch (SHARP) series [here, HMI stands for Helioseismic and Magnetic Imager on board the Solar Dynamics Observatory (SDO)]. Many existing ML packages require input data to be organized in special formats in which case reformatting the vast stretches of data is often very time consuming. Below we provide some specific examples of solar and interplanetary data that demonstrate the immediate need to organize data from various spacecraft so as to have large sets of AI-ready data in the immediate future.
1. Solar wind data measured close to Earth: A survey of Wind data sources available through the Space Physics Data Facility (SPDF) revealed that there exist about 128 distinct data sources in various locations, most of which are not comprehensive in the types of data provided, and the physical parameters measured by them are not consistent; that is, if some provide magnetometer data, others may be providing parameters such as solar wind density or velocity. Moreover, for data of a single type (e.g., magnetic field), the measurement cadence and the coordinate system in which the data are measured will be different for different sources. (see Section 5)
2. OMNI Solar Wind Data: One of the long-term data sets extensively used in space science and solar physics is the in situ solar wind measurements since the 1960s (https://spdf.gsfc.nasa.gov or https://omniweb.gsfc.nasa.gov). These are numerical time series data. While the data are easily accessible and well documented, these are multi-spacecraft compilations that are propagated to a reference distance near the Earth’s bow-shock region and therefore lack critical information for specific calculations relevant to magnetospheric studies.
3. Solar Imagery: Another data set that would benefit (if used for AI applications) from more information on data and metadata is solar imagery such as the ones provided by SDO (https://sdo.gsfc.nasa.gov/data/) and the Solar and Heliospheric Observatory (SOHO: https://soho.nascom.nasa.gov). Though these spacecraft and many similar ones record and store data digitally, the resolution, cadence and other relevant information are so different among them that it is challenging to combine them for a specific project, especially using ML. This is because data pre-processing becomes tedious or even impossible due to lack of sufficient information and expertise in cross-calibration of different spacecraft data.
Typically, the calibrated and processed data from various spacecraft exist in a variety of formats. As discussed above, it is easier for users, especially for those implementing AI techniques, if all the data of a particular type (e.g., solar wind measurements) from various spacecraft have the same format, or have information on, or have access to a software package for, converting from one format to another easily. This is in agreement with the NASA’s Transform to Open Science (TOPS, https://nasa.github.io/Transform-to-Open-Science/) mission and Science Policy Document (SPD-41a, https://science.nasa.gov/sciencered/s3fs-public/atoms/files/SMDinformation-policy-SPD-41a.pdf) that requires transparency and access to data and software for NASA-funded science investigations and missions.
An important aspect to consider when getting the space science data AI-ready, at least in some cases, is the limited or little access to orbital information and the characteristics of the region in which the observations are made, as described in Item 1 in Section 2.1. For example, the spacecraft may be in the solar wind inside or outside the foreshock region. If the spacecraft is at a substantial distance from Earth, the data need to be propagated to some reference point such as the subsolar bow shock. Moreover, since most of these spacecraft are in eccentric orbits, the solar wind is only intermittently available and a continuous record requires the assembly of data from multiple sources. This is a common problem for planetary science and heliophysics (e.g., Ruhunusiri et al., 2018).
It requires considerable domain knowledge and spacecraft details to identify available data that can be used for a specific scientific problem. Understanding of the instruments and their characteristics is necessary for data reduction and cross calibration of the various data sets from different sources so as to produce data sets that have a uniform coordinate system and cadence. An illustration of how complex this can be is provided by the National Science Foundation (NSF) funded SuperMAG project at the Applied Physics Laboratory of the Johns Hopkins University. This project has acquired ground magnetometer data from almost all existing magnetometers starting in 1975. Currently this includes more than 200 data sources. The data are corrected and transformed to a consistent coordinate system and interpolated to a fixed cadence. Quiet backgrounds for every station and component are calculated and subtracted from the data to obtain perturbations caused by magnetic activity.
While there exist NASA-funded repositories for synthetic data (e.g., models and simulations) generated by individual researchers in certain space science domains, there is no central repository publicly available in other fields of space science. Lack of such an archival can be a major limitation in addressing specific science topics where observational data are insufficient or sub-optimal. For example, in the field of exoplanets, where use of ML has grown over the past decade, especially for areas of exoplanet science lacking in measured data, (e.g., Ansdell et al., 2018; Márquez-Neila et al., 2018; Shallue and Vanderburg, 2018; Zingales and Waldmann, 2018; Cobb et al., 2019; Barros et al., 2020; Nikolaou et al., 2020; Osborn et al., 2020; Armstrong et al., 2021; de Beurs et al., 2021; Emsenhuber et al., 2021; Himes et al., 2022), investigators rely on synthetic data to employ ML methods (e.g., for atmospheric retrieval Márquez-Neila et al., 2018; Zingales and Waldmann, 2018; Cobb et al., 2019; Himes et al., 2022). The NASA Exoplanet Archive and Goddard’s Exoplanet Modeling and Analysis Center (EMAC: https://emac.gsfc.nasa.gov) offer hosting of large exoplanet-related data sets with metadata. However, investigators who generate synthetic data may elect to not share their data, and those who share their data may have provided insufficient metadata for applications beyond what was considered in their use case. Adherence to FAIR (Findable, Accessible, Interoperable, Reusable) standards (see Item VI in Section 3) may be useful in this scenario. Looking ahead to the coming decades, open access to these data will become increasingly important in order to discern the optimal ML methods for these types of problems. Synthetic data in other fields such as solar physics and magnetospheric science should also be archived and made accessible to the research community in a similar fashion, wherever appropriate.
It is well-known that some AI-applications demand enormous volumes (terabytes and petabytes) of data. Equally important are the “pre-processing” requirements and normalization of the data sets. All of these critically depend on the accessibility to the data and the various key information of data collection and processing (or metadata) such as cadence, resolution, calibration, format, and standardization (or information for standardization) of data from multiple sources (e.g., Items 1 and 3 in Section 2.1). Therefore, by AI-readiness, we imply that, “the data must be queryable, easily accessible, and include location information and a description of the data (metadata)”. The analogy would be to treat space science data like LEGO® pieces: standardized and modular. For greater clarity, we further elaborate on the definition of AI-readiness as described below.
I. The data must be well documented by addressing technicalities including, but not limited to, hard-coded thresholds, processing steps, possible causal relationships, potential latent variables/known unknowns, anomalies, noise level estimates, saturation levels, any or all operational assumptions made, uncertainty, ideal and updated instrumental profiles, biases, and ambiguities. This is expected to minimize the challenge of data (pre)processing for non-domain experts and, thereby, reduce the risk of misinterpretation of the data.
II. Metadata must include information such as spacecraft location, measure of instrumental degradation (monitor data drift), image resolution, and data shape.
III. It is envisioned that data certification or data validation issued through automation or peer review (similar to benchmarks for algorithms and referee reports for papers) would ensure community-wide standards and best-practices for data integrity and reproducibility. These should appear in a data catalogue and point to approved queryable databases.
IV. Include operations performed on the data (levels of data processing and pre-processing) in the flags because these operations could mask or confound ML pattern discovery.
V. If labels or annotations are part of the data set, include information on how the labels are obtained; that is, whether by expert labelers or volunteers. If volunteers, indicate which guidelines they used and how well defined were those guidelines to obtain a measure of uncertainty or annotation variability.
VI. Data should be flagged on a quality measure and adhere to FAIR data principles.
VII. Queryable flags would facilitate efficient selection of representative training sets.
VIII. Quality should encompass completeness, accuracy, availability, consistency, and latency.
In this section, we summarize the standards for the technical aspects in the preparation of AI-ready data based on the best practices, guidelines and tasks in the preparation for AI-ready data at each stage from data collection to data release.
Data repositories such as SPDF hold spacecraft data extensively used in the space sciences, particularly in space weather studies. However, there exist significant challenges in using them in ML applications due to non-uniform data formats and lack of appropriate metadata as discussed in Section 2. Therefore, the following aspects must be ensured during data collection.
a. Adopt a common format for data representation, such as NetCDF, CDF, HDF, or FITS.
b. Include quality flag(s).
c. Implement metadata tags suitable for the science topic as per the NASA Space Physics Archive Search and Extract (SPASE) standards for metadata.
d. Follow FAIR (Item VI in Section 3) data principles for open access to AI/ML ready data.
e. Develop open-source code for transforming data from one standard representation to another.
These are two important steps to handle missing data, remove outliers and normalize data across multiple sources. However, the precise manner in which the data cleaning operations are performed are often specific to the science topic being solved and the ML technique being used. Data normalization is a necessary data processing step to ensure that data from multiple sensors measuring similar observations adhere to common calibration metrics—e.g., instruments may be recording data at varying cadences which may require that they are resampled at a common cadence. Common questions include:
a. What is the tolerable length of data gaps?
b. How is the data interpolated and how does it impact data quality?
Including annotation tags is an integral part of data preparation for AI-readiness as it facilitates their (re)use among researchers with or without domain expertise. Listed below are a few essential tags:
a. Data quality measure.
b. Annotation of any kind of data pre-processing, required for reproducibility.
c. Annotation of features that are of scientific interest.
There are aspects to data preparation that must be considered to successfully transfer the ML and AI models from research to operations. Due to the data intensive nature of ML and AI models, they can be very sensitive to changes in the underlying data or applications. Changes in data patterns over time occur as sensors age or get replaced as new sensors are added (wherever applicable) or as underlying data correction and normalization identify and correct previously unknown data contamination. Therefore, it is important to annotate each step of the data preparation process in order that data provenance be available to the AI/ML model so that it may be retrained on the new data.
By defining AI-readiness for implementation and outlining the requirements for creating AI-ready data within a few years, we recommend a plausible course of action to achieve this. Getting the existing data AI-ready by accounting for the problems discussed in the foregoing sessions will require active involvement of scientists working on science projects using these data sets. To achieve this within the next few years, we envisage government agencies make available research opportunities to prepare, utilize, and archive data sets useful for ML applications in partnership with funding projects utilizing applications of AI. Investigators must carry out a relevant scientific study using the data they have organized to be AI-ready to demonstrate that the data are adequately documented and simple to use.
To demonstrate this idea, let us take our example of near-Earth observations of the solar wind (Item 1 in Section 2.1). In order to get these data organized, one must fetch the data stored in different formats at the various repositories, re-process the original data (if necessary), apply new calibrations when required, and organize the output in simple flat files. Moreover, the data must be transformed to a single coordinate system such as geocentric solar magnetospheric (GSM) and at a fixed cadence. These data are to be stored as time series with missing data flags wherever necessary. Orbit and attitude information should be combined with the observations and provided as metadata, preferably in the standard SPASE: https://spase-group.org/index.html format. Providing User Guides with descriptions of processing history and limitations of the data would also be useful. The observations and metadata should be placed in a public repository for the easy access of the public and the research community.
A possible project, in the above example, would be the response function of selected magnetospheric variables to solar wind drivers using neural networks. Functions obtained with data propagated from L1 to the bow shock could be compared to the same function determined from near-Earth observations. Another example is getting the SDO and SOHO data AI-ready as mentioned in Item 3 in Section 2.1. The SDO-ML project, an effort of the 2018 NASA Frontier Development Laboratory Program (FDL: e.g., Galvez et al., 2019; Shneider et al., 2021), is an attempt to overcome the difficulties discussed in Item 3 in Section 2.1 but more extensive efforts are urgently needed.
We suggest building investigation teams with strong collaboration between research scientists (domain experts) and data scientists. This would ensure that the data are structured conveniently for research and are organized in a logical manner for computer access by AI algorithms. The projects would require identification of data sources (scope of prioritization of space science data to be AI-ready) and plans for creation (or modification) of metadata and other aspects of AI-readiness in accordance with suggestions in Section 3 and Section 4. An added advantage of such collaboration and projects would be the open-source software interfaces that assist in using the original data sets that can be expected as secondary deliverables.
The process of enabling existing data to be AI-ready will require investment and continual updates of repositories (e.g., updating calibration methods, error corrections, data from new spacecraft missions and ground-based observatories), ensuring the implementation of the requirements outlined in Section 3.
We have identified the major difficulties in accessing and taking full advantage of existing space science data when implementing AI and DS methods. To address these problems and obtain the space science data AI-ready within the coming decade, we recommend that the scientific community and funding agencies support multi-year data engineering efforts led by domain experts who aim at providing AI-ready data that users could easily access from a publicly available repository for specific problems relevant to space science. In recognition of the multidisciplinary nature of this problem, such a program should include both data scientists and AI experts. We suggest that this effort to mitigate the obstacles faced by researchers implementing ML methods to be pursued as a project similar to the NASA/NSSDC efforts to create the OMNI database (https://omniweb.gsfc.nasa.gov). NSSDC uses data from the L1 point, 250 R⊕ (Earth-radius) upstream, to propagate it to the subsolar bow shock and when a spacecraft changes, the new data are cross calibrated to maintain a consistent record. The availability of the OMNI data has enabled a very large number of studies of the solar wind interaction with Earth. To achieve our vision of AI-ready data, we recommend that government agencies such as NASA and NSF create new research program(s) like NSSDC that would facilitate data engineers and scientists to come together to prepare the AI-ready data sets. We emphasize that this is envisaged as a long-term effort focused on getting AI-ready data and extends beyond applications of AI methods.
The original contributions presented in the study are included in the article/Supplementary material, further inquiries can be directed to the corresponding author.
BP led the SSI Virtual conference, with KP, MG, OV, JB, PW, and SW on the scientific organizing committee. BP wrote the first draft of the manuscript and all authors contributed to manuscript revision, read, and approved the submitted version. All authors contributed to the article and approved the submitted version.
The material presented here is based in part upon work supported by the National Science Foundation under Award No. AGS-2114219. Any opinions, findings, and conclusions or recommendations expressed in this publication are those of the authors and do not necessarily reflect the views of the National Science Foundation. MH was supported by the NASA Fellowship Activity under NASA Grant 80NSSC20K0682 and by an appointment to the NASA Postdoctoral Program at the NASA Goddard Space Flight Center, administered by Oak Ridge Associated Universities under contract with NASA. The work of JB was supported at the Space Science Institute by the NSF GEM Program via grant AGS-2027569 and by the NASA Heliophysics LWS program via award NNX16AB75G, by the NASA HERMES Interdisciplinary Science Program via grant 80NSSC21K1406. The work of BP is supported by National Science Foundation grant 2026579.
OV acknowledges that portions of work were performed at the Jet Propulsion Laboratory, California Institute of Technology, under a contract with NASA.
Author SK is employed by Computational Physics Inc., Lowell, MA, United States.
The remaining authors declare that the research was conducted in the absence of any commercial or financial relationships that could be construed as a potential conflict of interest.
All claims expressed in this article are solely those of the authors and do not necessarily represent those of their affiliated organizations, or those of the publisher, the editors and the reviewers. Any product that may be evaluated in this article, or claim that may be made by its manufacturer, is not guaranteed or endorsed by the publisher.
Angryk, R. A., Martens, P. C., Aydin, B., Kempton, D., Mahajan, S. S., Basodi, S., et al. (2020). Multivariate time series dataset for space weather data analytics. Sci. Data7, 227. doi:10.1038/s41597-020-0548-x
Ansdell, M., Ioannou, Y., Osborn, H. P., Sasdelli, M., Caldwell, D., et al. (2018). NASA Frontier Development Lab Exoplanet Team, Smith, J. C., 2018). Scientific domain knowledge improves exoplanet transit classification with deep learning. Astrophysical J. Lett.869, L7. doi:10.3847/2041-8213/aaf23b
Armstrong, D. J., Gamper, J., and Damoulas, T. (2021). Exoplanet validation with machine learning: 50 new validated kepler planets. Mon. Notices R. Astronomical Soc.504, 5327–5344. doi:10.1093/mnras/staa2498
Azari, A., Biersteker, J. B., Dewey, R. M., Doran, G., Forsberg, E. J., Harris, C. D. K., et al. (2021). Integrating machine learning for planetary science: Perspectives for the next decade. Bull. AAS53. doi:10.3847/25c2cfeb.aa328727
Barros, S. C. C., Demangeon, O., Díaz, R. F., Cabrera, J., Santos, N. C., Faria, J. P., et al. (2020). Improving transit characterisation with Gaussian process modelling of stellar variability. Astronomy Astrophysics634, A75. doi:10.1051/0004-6361/201936086
Bobra, M. G., and Couvidat, S. (2015). Solar flare prediction using SDO/HMI vector magnetic field data with a machine-learning algorithm. Astrophys. J.798, 135–146. doi:10.1088/0004-637X/798/2/135
Camporeale, E., Chu, X., Agapitov, O. V., and Bortnik, J. (2019). On the generation of probabilistic forecasts from deterministic models. Space weather.17, 455–475. doi:10.1029/2018sw002026
Camporeale, E., and Soc-Ml-Helio, (2020). Ml-helio: An emerging community at the intersection between heliophysics and machine learning. J. Geophys. Res.125. doi:10.1029/2019JA027502
Camporeale, E. (2019). The challenge of machine learning in space weather: Nowcasting and forecasting. Space weather.17, 1166–1207. doi:10.1029/2018sw002061
Cobb, A. D., Himes, M. D., Soboczenski, F., Zorzan, S., O’Beirne, M. D., Baydin, A. G., et al. (2019). An ensemble of bayesian neural networks for exoplanetary atmospheric retrieval. Astronomical J.158, 33. doi:10.3847/1538-3881/ab2390
de Beurs, Z. L., Vanderburg, A., Shallue, C. J., Collaboration, H.-N., Cameron, A. C., Leet, C., et al. (2021). Identifying exoplanets with deep learning. IV. removing stellar activity signals from radial velocity measurements using neural networks. Astronomical J.164, 49. doi:10.3847/1538-3881/ac738e
Emsenhuber, A., Asphaug, E., Cambioni, S., Gabriel, T. S. J., and Schwartz, S. R. (2021). Collision chains among the terrestrial planets. II. an asymmetry between Earth and Venus. Planet. Sci. J.2, 199. doi:10.3847/psj/ac19b1
Galvez, R., Fouhey, D. F., Jin, M., Szenicer, A., Munoz-Jaramillo, A., Cheung, M. C. M., et al. (2019). A machine learning dataset prepared from the nasa sdo mission. arXiv:1903.04538 [astro-ph.SR].
Himes, M. D., Harrington, J., Cobb, A. D., Baydin, A. G., Soboczenski, F., O’Beirne, M. D., et al. (2022). Accurate machine-learning atmospheric retrieval via a neural-network surrogate model for radiative transfer. Planet. Sci. J.3, 91. doi:10.3847/PSJ/abe3fd
Lundstedt, H. (1996). Solar origin of geomagnetic storms and predictions. J.Atmos. Terr. Phys.58, 821–830. doi:10.1016/0021-9169(95)00105-0
Márquez-Neila, P., Fisher, C., Sznitman, R., and Heng, K. (2018). Supervised machine learning for analysing spectra of exoplanetary atmospheres. Nat. Astron.2, 719–724. doi:10.1038/s41550-018-0504-2
McGranaghan, R. M., Mannucci, A. J., Wilson, B. D., Mattmann, C. A., and Chadwick, R. (2018). New capabilities for prediction of high-latitude ionospheric scintillation: A novel approach with machine learning. Space weather.16, 1817–1846. doi:10.1029/2018SW002018
McGranaghan, R. M., Ziegler, J., Bloch, T., Hatch, S., Camporeale, E., Lynch, K., et al. (2021). Toward a next generation particle precipitation model: Mesoscale prediction through machine learning (a case study and framework for progress). Space weather.19, e2020SW002684. doi:10.1029/2020SW002684
Nikolaou, N., Waldmann, I. P., Tsiaras, A., Morvan, M., Edwards, B., Hou Yip, K., et al. (2020). Lessons learned from the 1st ariel machine learning challenge: Correcting transiting exoplanet light curves for stellar spots. arXiv e-prints ArXiv:2010.15996.
Osborn, H. P., Ansdell, M., Ioannou, Y., Sasdelli, M., Angerhausen, D., Caldwell, D., et al. (2020). Rapid classification of tess planet candidates with convolutional neural networks. Astronomy Astrophysics633, A53. doi:10.1051/0004-6361/201935345
Poduval, B., McPherron, R. L., Walker, R., Himes, M. D., Pitman, K. M., Azari, A. R., et al. (2022). “AI-ready data in solar physics and space science: Concerns, mitigation and recommendations,” in White paper submitted to the decadal survey for solar and space physics (heliophysics) 2024-2033. Available at: http://surveygizmoresponseuploads.s3.amazonaws.com/fileuploads/623127/6920789/107-1870187ec154eee48664bed68513f0cb_PoduvalBala.pdf.
Poduval, B., Pitman, K., Verkhoglyadova, O., and Wintoft, P. (2023). Editorial: Applications of statistical methods and machine learning in the space sciences. Front. Astron. Space Sci.10. doi:10.3389/fspas.2023.1163530
Ruhunusiri, S., Halekas, J. S., Espley, J. R., Eparvier, F., Brain, D., Mazelle, C., et al. (2018). An artificial neural network for inferring solar wind proxies at Mars. Geophys. Res. Lett.45, 10855–10865. doi:10.1029/2018gl079282
Shallue, C. J., and Vanderburg, A. (2018). Identifying exoplanets with deep learning: A five-planet resonant chain around kepler-80 and an eighth planet around kepler-90. Astronomical J.155, 94. doi:10.3847/1538-3881/aa9e09
Shneider, C., Hu, A., Tiwari, A. K., Bobra, M. G., Battams, K., Teunissen, J., et al. (2021). A machine-learning-ready dataset prepared from the solar and heliospheric observatory mission. arXiv:2108.06394 [astro-ph.SR]. doi:10.48550/arXiv.2108.06394
Wing, S., Johnson, J. R., Turner, D. L., Ukhorskiy, A. Y., and Boyd, A. J. (2022). Untangling the solar wind and magnetospheric drivers of the radiation belt electrons. J. Geophys. Res. Space Phys.127, e2021JA030246. doi:10.1029/2021JA030246
Wintoft, P., and Lundstedt, H. (1997). Prediction of daily average solar wind velocity from solar magnetic field observations using hybrid intelligent systems. Phys. Chem. Earth22, 617–622. doi:10.1016/s0079-1946(97)00186-9
Keywords: AI-ready data, machine learning, space science, statistical methods, decadal survey, space weather, exoplanet, planetary science
Citation: Poduval B, McPherron RL, Walker R, Himes MD, Pitman KM, Azari AR, Shneider C, Tiwari AK, Kapali S, Bruno G, Georgoulis MK, Verkhoglyadova O, Borovsky JE, Lapenta G, Liu J, Alberti T, Wintoft P and Wing S (2023) AI-ready data in space science and solar physics: problems, mitigation and action plan. Front. Astron. Space Sci. 10:1203598. doi: 10.3389/fspas.2023.1203598
Received: 11 April 2023; Accepted: 19 June 2023;
Published: 13 July 2023.
Edited by:
Daniel Okoh, The National Space Research and Development Agency (NASRDA), NigeriaReviewed by:
Reinaldo Roberto Rosa, National Institute of Space Research (INPE), BrazilCopyright © 2023 Poduval, McPherron, Walker, Himes, Pitman, Azari, Shneider, Tiwari, Kapali, Bruno, Georgoulis, Verkhoglyadova, Borovsky, Lapenta, Liu, Alberti, Wintoft and Wing. This is an open-access article distributed under the terms of the Creative Commons Attribution License (CC BY). The use, distribution or reproduction in other forums is permitted, provided the original author(s) and the copyright owner(s) are credited and that the original publication in this journal is cited, in accordance with accepted academic practice. No use, distribution or reproduction is permitted which does not comply with these terms.
*Correspondence: Bala Poduval, YmFsYS5wb2R1dmFsQHVuaC5lZHU=
Disclaimer: All claims expressed in this article are solely those of the authors and do not necessarily represent those of their affiliated organizations, or those of the publisher, the editors and the reviewers. Any product that may be evaluated in this article or claim that may be made by its manufacturer is not guaranteed or endorsed by the publisher.
Research integrity at Frontiers
Learn more about the work of our research integrity team to safeguard the quality of each article we publish.