- 1Max-Planck-Institut für Sonnensystemforschung, Göttingen, Germany
- 2Georg-August-Universität Göttingen, Göttingen, Germany
The study of planetary atmospheres is crucial for understanding the origin, evolution, and processes that shape celestial bodies like planets, moons and comets. The interpretation of planetary spectra requires a detailed understanding of radiative transfer (RT) and its application through computational codes. With the advancement of observations, atmospheric modelling, and inference techniques, diverse RT and retrieval codes in planetary science have been proliferated. However, the selection of the most suitable code for a given problem can be challenging. To address this issue, we present a comprehensive mini-overview of the different RT and retrieval codes currently developed or available in the field of planetary atmospheres. This study serves as a valuable resource for the planetary science community by providing a clear and accessible list of codes, and offers a useful reference for researchers and practitioners in their selection and application of RT and retrieval codes for planetary atmospheric studies.
1 Introduction
Planetary science, including the study of exoplanets, is currently a very active and fascinating multi-disciplinary field covering astronomy, astrophysics, geophysics, astrobiology, among other fields. By studying planets, we can answer fundamental questions about their origin, formation, evolution, potential for life, and physico-chemical processes. The spectrum of a planet contains valuable information about its atmospheric chemical composition and physical processes. Deriving atmospheric properties like the vertical thermal structure, chemical composition and dynamics is essential to understand the origin, evolution, and how the atmospheres are influenced by physical and chemical mechanisms.
Numerous physico-chemical problems in planetary atmospheres require a detailed understanding of radiative transfer (RT) of photons in different environments. Detailed solutions of the RT equation demand high computational capabilities. The technical implementation of these solutions in codes is a rapidly developing area, and numerous RT approaches have been attempted in recent years. Several groups then attacked the problem from a variety of perspectives by using completely different, independent and dedicated numerical algorithms. Some atmospheric RT codes have been developed with a particular aim or for the interpretation of a particular planet. Later on, in some cases, their functionalities have been extended beyond their original purposes but still limited to the study of a specific type of planets. In recent years, breakthroughs in atmospheric retrieval have been made possible through a combination of advancements in instrumentation, computational resources, sophisticated atmospheric modelling, and powerful statistical inference techniques.
There are varying levels of complexity in atmospheric models and the physico-chemical processes considered in RT and inversion codes, motivated by atmospheric observations. As a result, a significant number of RT and inversion codes have been developed and widely used in different atmospheric contexts, depending on whether the planet being studied is Earth, another planet or body in the Solar System, or an exoplanet. Spectra of Solar System planets can be spatially distributed and have a high signal-to-noise ratio, and can be supplemented with in situ measurements or a priori knowledge. However, exoplanetary spectroscopy currently lacks this observational capability, and retrievals must navigate a much larger parameter space for exoplanetary atmospheres than that for Solar System planets.
The selection of a particular code may depend on various factors, such as the physical problem to face, the estimation technique, computation time, flexibility, user-friendliness, among others. The selection of the most appropriate code suited for a specific problem may result in more accurate and reliable results, highlighting the importance of having a comprehensive list of available codes. This mini-review provides a comprehensive overview of the current RT and inversion codes used in the planetary and exoplanetary communities, as seen from the perspective of a user. These codes are crucial in predicting and interpreting spectra of planetary atmospheres, both in hydrostatic equilibrium and in expanding comas. The quality and extent of these codes are critical for the effective use of space and ground-based telescopes facilities. Madhusudhan (2018) has already provided a review of exoplanetary atmospheric retrieval, some existing retrieval codes, and a description of their differences, Barstow and Heng (2020) provides a discussion of open problems in retrieval analysis, and more recently, MacDonald and Batalha (2023) provides a catalogue of the atmospheric retrieval codes for exoplanets published to date. In this mini-review, we expand and update the codes list, including a large new generation of RT and atmospheric retrieval codes for Solar System and exoplanets.
2 RT and inversion codes
One commonly used way to interpret the measured spectra is calculating a synthetic spectrum for comparison with that measured by solving the radiative transfer (forward model) -i.e., computation of the outgoing radiation from the planetary surface for a given set of free parameters-, and inferring parameters like temperature and chemical abundance profiles. This last step is called inversion or retrieval and consists in comparing the measured and best modelled/synthetic spectra adjusting the atmospheric parameters in such a way as to minimise any discrepancy. A number of radiative transfer codes or forward models and inversion algorithms are already constructed, and generally available and used by the planetary and exoplanetary characterisation communities. A comprehensive list of RT and retrieval codes in the literature is shown in Table 1, indicating where to find them (if available) and the estimation technique employed (Section 3).
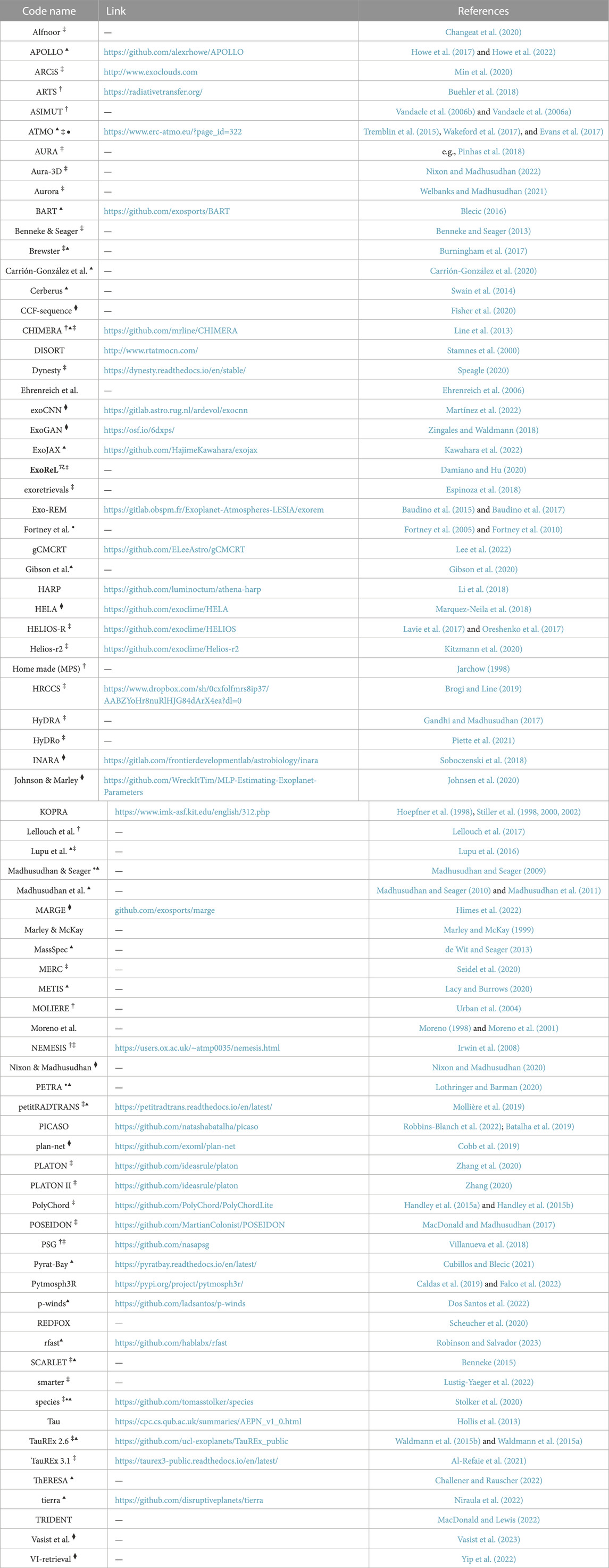
TABLE 1. Some radiative transfer and inversion codes used in the (exo) planetary community. Symbols: OE algorithm (†), nested sampling (‡), MCMC method (▴), Grid search (•), and ML approaches (⧫).
Retrieval codes can use either parametric models or self-consistent equilibrium models to estimate the composition and pressure-temperature (P-T) profiles from spectral data. Parametric models do not make any assumptions about thermochemical and radiative-convective equilibrium, using instead parametric forms for the P-T profile and composition. Self-consistent models compute profiles based on assumptions of the atmosphere’s physical and chemical properties and processes, with varying levels of complexity from 1-D atmospheres to full 3-D general circulation models. We do not cover 3-D general circulation codes specifically in this paper, they are complex and couple many processes together in a three-dimensional time-marching calculation. For a discussion of the principles of atmospheric retrievals of exoplanets see Gandhi and Madhusudhan (2017). When calculating a synthetic atmospheric spectrum, lines are read from atomic and molecular databases -databases differ in completeness and accuracies-, and opacities are calculated via diverse approaches (Rengel, 2022).
Some RT models used for Solar System planets and exoplanets have their roots in Earth codes. There are several RT codes used in the Earth atmospheric community but they are just listed here: 4A/OP [Scott (1974); Scott and Chedin (1981)], 6S [Lee et al. (2020)], AccuRT1[Stamnes et al. (2018)]; ARMS Weng et al. (2020), BIRRA2 [Gimeno García et al. (2011)], BTRAM3 [Chapman et al. (2004)], DISORT [Stamnes et al. (1988)], Eradiate4 [Govaerts et al. (2022)], FASCODE [Smith et al. (1978); Clough et al. (1981)], FUTBOLIN [Martin-Torres and Mlynczak (2005)], GARLIC [Schreier et al. (2014)], GENLN2 [Edwards (1992)], IMAP-DOAS [Frankenberg et al. (2005)], IMLM Gloudemans et al. (2005), KCARTA56 [DeSouza-Machado et al. (2020)], LBLRTM78 [Clough et al. (2005)], LEEDR [Fiorino et al. (2014)], LinePak9 [Gordley et al. (1994)], libRadtran10 [Mayer and Kylling (2005)], MODTRAN11 [Berk et al. (1998)], MPI12 [Gropp et al. (1998)], PUMAS13 [Villanueva et al. (2015); Villanueva et al. (2022)], RFM14 [Dudhia (2017)], RTMOM [Govaerts (2006)], RTTOV15 [Saunders et al. (1999); Matricardi (2007)], SARTA1617 [Strow et al. (2003)], SARTre [Mendrok (2006)], SASKTRAN18 [Bourassa et al. (2008); Zawada et al. (2015)], SBDART19 [Ricchiazzi et al. (2002)], SCIATRAN20 [Rozanov et al. (2005); Rozanov et al. (2014)], SICOR21 [Borsdorff et al. (2017); Borsdorff et al. (2018)], SKIRT22 [Baes et al. (2003)], SMART-G23 [Ramon et al. (2019)], TOMRAD [Dave (1964)], VDISORT [Lin et al. (2022)], VLIDORT/LIDORT24 [Spurr and Christi (2019)], WFM-DOAS [Buchwitz et al. (2004); Buchwitz et al. (2005)] as well as models by Hartogh (1989), Tinetti et al. (2006a), Tinetti et al. (2006b) and Robinson et al. (2011).
3 Retrieval or parameter fitting or estimation techniques
The objective of an optimization algorithm is to extensively and efficiently sample a high-dimensional parameter space to find the best solution space given the data. The retrieval or parameter fitting or estimation techniques commonly used are Optimal Estimation (OE) algorithm (†), nested sampling (‡), Markov chain Monte Carlo (MCMC) method (▴), Grid search (•), and more recently, data-driven Machine Learning (ML) approaches (⧫). OE is popular in the Solar System community and assumes Gaussian statistics. It is fast and efficient and applicable to exoplanets under some specific conditions (e.g., Rengel et al., 2008; Hartogh et al., 2010; Lee et al., 2011; Line et al., 2012; Shulyak et al., 2019; Rengel et al., 2022; Villanueva et al., 2022). Grid search is simple and computationally cheap, but it can be inefficient (e.g., Madhusudhan & Seager, 2009). MCMC provides a better parameter exploration of the parameter space but with limitations in calculating the model evidence for model comparison and can be computationally expensive (e.g., Benneke and Seager, 2012; Madhusudhan et al., 2014; Waldmann et al., 2015b; Blecic, 2016; Cubillos, 2016; Evans et al., 2017; Wakeford et al., 2017; Lacy and Burrows, 2020). Nested sampling algorithm facilitates efficient parameter space exploration and calculation of model evidence (e.g., Benneke and Seager, 2013; Waldmann et al., 2015a; Gandhi and Madhusudhan, 2017; Pinhas et al., 2018; Brogi and Line, 2019; Fisher and Heng, 2019; Mollière et al., 2019; Min et al., 2020; Seidel et al., 2020; Shulyak et al., 2020). ML algorithms can be computationally efficient, but they require large amounts of training data and can be sensitive to biases in the training set (e.g., Waldmann, 2016; Marquez-Neila et al., 2018; Soboczenski et al., 2018; Zingales and Waldmann, 2018; Cobb et al., 2019; Fisher et al., 2020; Hayes et al., 2020; Nixon and Madhusudhan, 2020). An example of an application of the forward model and retrieval technique is provided in Figure 1.
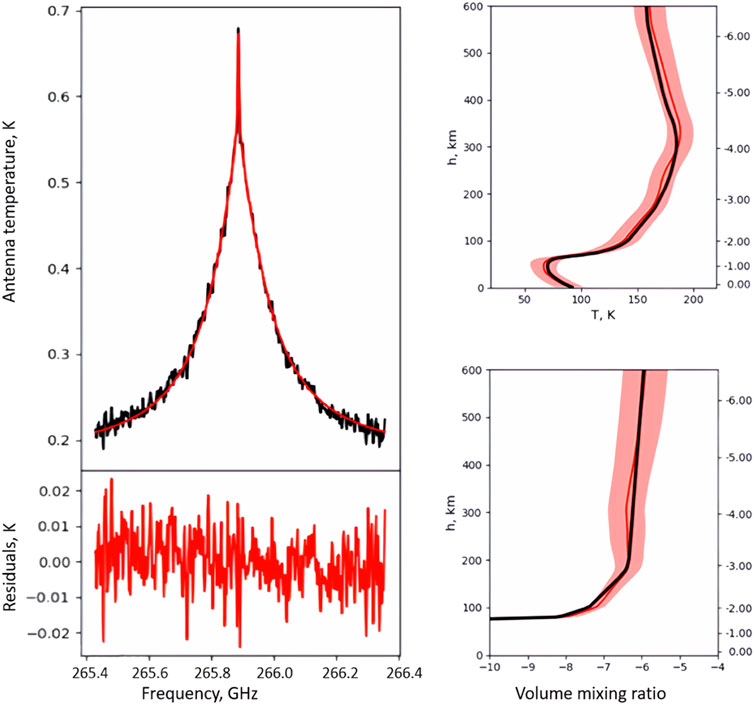
FIGURE 1. Example of forward model and retrieval applied to the case of HCN in Titan: Left: comparison between observed and best-fit simulated HCN (3-2) lines (black and red, respectively, upper panel), and the difference between the observed and fitted spectra (lower panel). Right: retrieved temperature and HCN distribution derived from the spectrum. The black and red lines, and the pink shadow show the initial and retrieved profiles, and the error bars, respectively. Figure based on Figure 1 in Rengel et al. (2022).
4 Verification and validation of RT and retrieval codes
The retrieval problem is a challenging one due to its ill-posed and ill-conditioned nature. This means that even a small change in measurements can result in a significant deviation in the estimated model, making the inverse solution computation extremely unstable (e.g., Ih and Kempton, 2021). Currently, each retrieval code has its unique method for computing opacities and input models, lacking community standards. Furthermore, models continue to become more complex and data quality improves, retrieval codes face increasingly complex and degenerate problems (e.g., Welbanks and Madhusudhan, 2019).
Verification and validation of planetary retrieval codes is an important aspect. Verification can be readily accomplished using synthetic measurements and code inter-comparison. Inter-model comparisons of forward and retrieval suites have already been underway, with notable studies by Clarmann et al., 2003; Baudino et al., 2017; Schreier et al., 2018; Barstow et al., 2020; Barstow et al., 2022). Validation is challenging due to the lack of reference “truth” data (e.g., in situ measurements). Thus, for an assessment of exoplanet atmospheric remote sensing, data dedicated to Solar System planets like Earth, Mars and Venus is an ideal test case. To our knowledge, this kind of data have been rarely used to demonstrate the capabilities of exoplanet atmospheric studies. Observing planetary transits in our own Solar System, for example, can serve as an invaluable benchmark and can provide crucial information for future exoplanet characterizations (e.g., Ehrenreich et al., 2011; Ehrenreich et al., 2012; Montañés-Rodríguez et al., 2015; López-Puertas et al., 2018; Schreier et al., 2018).
Results from inter-comparison show that small differences in the forward model setup can lead to noticeable differences in the retrieval outcome (Barstow et al., 2020). These efforts are crucial for improving the field and providing a clearer understanding of the sources of variability and their impact on the results. Such comparisons provide a roadmap for future advancements and refinements in modelling, enabling us to compare theoretical predictions with observations. They also offer an opportunity to identify any remaining problems, leading to the improvement of existing codes and the development of more reliable and consistent codes. The continued pursuit of inter-model comparisons will drive the field forward towards a better understanding of the retrieval problem. Furthermore, the inherent similarities in methods was investigated (Line et al., 2013), and there is now considerable work to understand and quantify the role of different modelling choices affecting accuracy and precision on constraints (Barstow et al., 2020).
5 Final remarks
Our current understanding of planetary atmospheres is limited by model completeness and robustness. Forward modelling and spectral retrieval is the leading technique for interpretation of spectra and is employed by various teams using a variety of forward models and parameter estimation algorithms. Numerous codes are available and continue been developed. The code employed which contain the complexity of models should be chosen with care depending on the specific questions whose answer is sought and on the nature of the data at hand (considering spectral coverage and region, resolution, precision, signal-to-noise ratio, etc.). The efficiency of these codes is a critical issue, but beyond the scope of this paper.
The study of RT in planetary atmospheres is a rapidly developing field, with numerous advances being made in both the understanding of the RT process and in the development of RT codes. With continued research and development including the improving of observational capabilities, it is likely that even more sophisticated RT codes and inversion algorithms will be developed in the future, enabling us to better understand the diversity of planetary systems beyond our own and the mechanisms shaping their atmospheres.
Author contributions
MR wrote the first draft of the manuscript. All authors contributed to the article and approved the submitted version.
Funding
Authors acknowledge the support by the DFG priority program SPP 1992 “Exploring the Diversity of Extrasolar Planets” (DFG PR 36 24602/41).
Acknowledgments
This research has made use of NASA’s Astrophysics Data System Bibliographic Services.
Conflict of interest
The authors declare that the research was conducted in the absence of any commercial or financial relationships that could be construed as a potential conflict of interest.
Publisher’s note
All claims expressed in this article are solely those of the authors and do not necessarily represent those of their affiliated organizations, or those of the publisher, the editors and the reviewers. Any product that may be evaluated in this article, or claim that may be made by its manufacturer, is not guaranteed or endorsed by the publisher.
Footnotes
1http://www.geminor.com/accurt.html.
2https://www.dlr.de/eoc/en/desktopdefault.aspx/tabid-5447/9970_read-20673/.
3https://blueskyspectroscopy.com/?page_id=21.
4https://www.eradiate.eu/site/.
5http://asl.umbc.edu/pub/packages/kcarta.html.
6https://github.com/sergio66/kcarta_gen.
7http://rtweb.aer.com/lblrtm.html.
8https://github.com/AER-RC/LBLRTM.
9https://spectralcalc.com/info/about.
10http://www.libradtran.org/doku.php.
11http://modtran.spectral.com.
12https://mitpress.mit.edu/9780262571234/mpi-the-complete-reference/.
14http://eodg.atm.ox.ac.uk/RFM/.
15https://nwp-saf.eumetsat.int/site/software/rttov/.
16https://github.com/strow/sarta.
17https://github.com/clhepp/sarta.
18https://arg.usask.ca/docs/sasktran/.
19https://github.com/paulricchiazzi/SBDART.
20https://www.iup.uni-bremen.de/sciatran/.
21https://pypi.org/project/sicor/.
22https://skirt.ugent.be/root/_home.html.
23https://www.hygeos.com/smartg.
24http://www.rtslidort.com/about_overview.html.
References
Al-Refaie, A. F., Changeat, Q., Waldmann, I. P., and Tinetti, G. (2021). TauREx 3: A fast, dynamic, and extendable framework for retrievals. Astrophys. J.917, 37. doi:10.3847/1538-4357/ac0252
Baes, M., Davies, J. I., Dejonghe, H., Sabatini, S., Roberts, S., Evans, R., et al. (2003). Radiative transfer in disc galaxies – III. the observed kinematics of dusty disc galaxies. Mon. Notices R. Astronomical Soc.343, 1081–1094. doi:10.1046/j.1365-8711.2003.06770.x
Barstow, J. K., Changeat, Q., Chubb, K. L., Cubillos, P. E., Edwards, B., MacDonald, R. J., et al. (2022). A retrieval challenge exercise for the ariel mission. Exp. Astron.53, 447–471. doi:10.1007/s10686-021-09821-w
Barstow, J. K., Changeat, Q., Garland, R., Line, M. R., Rocchetto, M., and Waldmann, I. P. (2020). A comparison of exoplanet spectroscopic retrieval tools. Mon. Not. R. Astron. Soc.493, 4884–4909. doi:10.1093/mnras/staa548
Barstow, J. K., and Heng, K. (2020). Outstanding challenges of exoplanet atmospheric retrievals. Outst. Challenges Exopl. Atmos. Retrievals216, 82. doi:10.1007/s11214-020-00666-x
Batalha, N. E., Marley, M. S., Lewis, N. K., and Fortney, J. J. (2019). Exoplanet reflected-light spectroscopy with picaso. Astrophysical J.878, 70. doi:10.3847/1538-4357/ab1b51
Baudino, J.-L., Mollière, P., Venot, O., Tremblin, P., Bézard, B., and Lagage, P.-O. (2017). Toward the analysis ofJWSTExoplanet spectra: Identifying troublesome model parameters. Identifying Troubl. Model. Param.850, 150. doi:10.3847/1538-4357/aa95be
Baudino, J. L., Bézard, B., Boccaletti, A., Bonnefoy, M., Lagrange, A. M., and Galicher, R. (2015). Interpreting the photometry and spectroscopy of directly imaged planets: a new atmospheric model applied to β Pictoris b and SPHERE observations, 582, A83. doi:10.1051/0004-6361/201526332
Benneke, B., and Seager, S. (2012). Atmospheric retrieval for super-earths: Uniquely constraining the atmospheric composition with transmission spectroscopy. Astrophys. J.753, 100. doi:10.1088/0004-637X/753/2/100
Benneke, B., and Seager, S. (2013). How to distinguish between cloudy mini-neptunes and water/volatile-dominated super-earths. Astrophys. J.778, 153. doi:10.1088/0004-637X/778/2/153
Benneke, B. (2015). Strict upper limits on the carbon-to-oxygen ratios of eight hot jupiters from self-consistent atmospheric retrieval. arXiv e-prints , arXiv:1504.07655.
Berk, A., Bernstein, L., Anderson, G., Acharya, P., Robertson, D., Chetwynd, J., et al. (1998). Modtran cloud and multiple scattering upgrades with application to aviris. Remote Sens. Environ.65, 367–375. doi:10.1016/S0034-4257(98)00045-5
Blecic, J. (2016). Observations, thermochemical calculations, and modeling of exoplanetary atmospheres. arXiv e-prints , arXiv:1604.02692.
Borsdorff, T., Aan de Brugh, J., Hu, H., Aben, I., Hasekamp, O., and Landgraf, J. (2018). Measuring carbon monoxide with tropomi: First results and a comparison with ecmwf-ifs analysis data. Geophys. Res. Lett.45, 2826–2832. doi:10.1002/2018GL077045
Borsdorff, T., aan de Brugh, J., Hu, H., Nédélec, P., Aben, I., and Landgraf, J. (2017). Carbon monoxide column retrieval for clear-sky and cloudy atmospheres: A full-mission data set from sciamachy 2.3µm reflectance measurements. Atmos. Meas. Tech.10, 1769–1782. doi:10.5194/amt-10-1769-2017
Bourassa, A., Degenstein, D., and Llewellyn, E. (2008). Sasktran: A spherical geometry radiative transfer code for efficient estimation of limb scattered sunlight. J. Quantitative Spectrosc. Radiat. Transf.109, 52–73. doi:10.1016/j.jqsrt.2007.07.007
Brogi, M., and Line, M. R. (2019). Retrieving temperatures and abundances of exoplanet atmospheres with high-resolution cross-correlation spectroscopy. Astronomical J.157, 114. doi:10.3847/1538-3881/aaffd3
Buchwitz, M., de Beek, R., Bramstedt, K., Noël, S., Bovensmann, H., and Burrows, J. P. (2004). Global carbon monoxide as retrieved from SCIAMACHY by WFM-DOAS. Atmos. Chem. Phys.4, 1945–1960. doi:10.5194/acp-4-1945-2004
Buchwitz, M., de Beek, R., Noël, S., Burrows, J. P., Bovensmann, H., Bremer, H., et al. (2005). Carbon monoxide, methane and carbon dioxide columns retrieved from sciamachy by wfm-doas: Year 2003 initial data set. Atmos. Chem. Phys.5, 3313–3329. doi:10.5194/acp-5-3313-2005
Buehler, S. A., Mendrok, J., Eriksson, P., Perrin, A., Larsson, R., and Lemke, O. (2018). ARTS, the Atmospheric Radiative Transfer Simulator - version 2.2, the planetary toolbox edition. Geosci. Model. Dev.11, 1537–1556. doi:10.5194/gmd-11-1537-2018
Burningham, B., Marley, M. S., Line, M. R., Lupu, R., Visscher, C., Morley, C. V., et al. (2017). Retrieval of atmospheric properties of cloudy L dwarfs. Mon. Notices R. Astronomical Soc.470, 1177–1197. doi:10.1093/mnras/stx1246
Caldas, A., Leconte, J., Selsis, F., Waldmann, I. P., Bordé, P., Rocchetto, M., et al. (2019). Effects of a fully 3d atmospheric structure on exoplanet transmission spectra: Retrieval biases due to day–night temperature gradients. Astronomy Astrophysics623, A161. doi:10.1051/0004-6361/201834384
Carrión-González, O., García Muñoz, A., Cabrera, J., Csizmadia, Sz., Santos, N. C., and Rauer, H. (2020). Directly imaged exoplanets in reflected starlight: The importance of knowing the planet radius. A&A640, A136. doi:10.1051/0004-6361/202038101
Challener, R. C., and Rauscher, E. (2022). Theresa: Three-dimensional eclipse mapping with application to synthetic jwst data. Astronomical J.163, 117. doi:10.3847/1538-3881/ac4885
Changeat, Q., Al-Refaie, A., Mugnai, L. V., Edwards, B., Waldmann, I. P., Pascale, E., et al. (2020). Alfnoor: A retrieval simulation of the ariel target list. Astronomical J.160, 80. doi:10.3847/1538-3881/ab9a53
Chapman, I. M., Naylor, D. A., and Phillips, R. R. (2004). Correlation of atmospheric opacity measurements by SCUBA and an infrared radiometer. Mon. Notices R. Astronomical Soc.354, 621–628. doi:10.1111/j.1365-2966.2004.08223.x
Clarmann, T., Höpfner, M., Funke, B., López-Puertas, M., Dudhia, A., Jay, V., et al. (2003). Modelling of atmospheric mid-infrared radiative transfer: The amil2da algorithm intercomparison experiment. J. Quantitative Spectrosc. Radiat. Transf.78, 381–407. doi:10.1016/S0022-4073(02)00262-5
Clough, S. A., Kneizys, F. X., Rothman, L. S., and Gallery, W. O. (1981). “Atmospheric spectral transmittance and radiance: FASCOD1 B,”. Atmospheric transmission. Editor R. W. Fan (Washington, DC: International Society for Optics and Photonics), 0277, 152–167. doi:10.1117/12.931914
Clough, S., Shephard, M., Mlawer, E., Delamere, J., Iacono, M., Cady-Pereira, K., et al. (2005). Atmospheric radiative transfer modeling: A summary of the aer codes. J. Quantitative Spectrosc. Radiat. Transf.91, 233–244. doi:10.1016/j.jqsrt.2004.05.058
Cobb, A. D., Himes, M. D., Soboczenski, F., Zorzan, S., O’Beirne, M. D., Baydin, A. G., et al. (2019). An ensemble of bayesian neural networks for exoplanetary atmospheric retrieval. Astronomical J.158, 33. doi:10.3847/1538-3881/ab2390
Cubillos, P. E., and Blecic, J. (2021). The PYRAT BAY framework for exoplanet atmospheric modelling: A population study of hubble/WFC3 transmission spectra. Mon. Not. R. Astron. Soc.505, 2675–2702. doi:10.1093/mnras/stab1405
Cubillos, P. E. (2016). Characterizing exoplanet atmospheres: From light-curve observations to radiative-transfer modeling. arXiv e-prints, arXiv:1604.01320. doi:10.48550/arXiv.1604.01320
Damiano, M., and Hu, R. (2020). ExoReL: A bayesian inverse retrieval framework for exoplanetary reflected light spectra. Astronomical J.159, 175. doi:10.3847/1538-3881/ab79a5
Dave, J. V. (1964). Meaning of successive iteration of the auxiliary equation in the theory of radiative transfer. Astrophysical J.140, 1292. doi:10.1086/148024
de Wit, J., and Seager, S. (2013). Constraining exoplanet mass from transmission spectroscopy. Science342, 1473–1477. doi:10.1126/science.1245450
DeSouza-Machado, S., Strow, L. L., Motteler, H., and Hannon, S. (2020). kcarta: a fast pseudo line-by-line radiative transfer algorithm with analytic jacobians, fluxes, nonlocal thermodynamic equilibrium, and scattering for the infrared. Atmos. Meas. Tech.13, 323–339. doi:10.5194/amt-13-323-2020
Dos Santos, L. A., Vidotto, A. A., Vissapragada, S., Alam, M. K., Allart, R., Bourrier, V., et al. (2022). p-winds: An open-source python code to model planetary outflows and upper atmospheres. A&A659, A62. doi:10.1051/0004-6361/202142038
Dudhia, A. (2017). The reference forward model (rfm). J. Quantitative Spectrosc. Radiat. Transf.186, 243–253. doi:10.1016/j.jqsrt.2016.06.018
Edwards, D. P. (1992). GENLN2: A general line-by-line atmospheric transmittance and radiance model. Version 3.0: Description and users guide.
Ehrenreich, D., Tinetti, G., des Etangs, A. L., Vidal-Madjar, A., and Selsis, F. (2006). The transmission spectrum of earth-size transiting planets. Astronomy Astrophysics448, 379–393. doi:10.1051/0004-6361:20053861
Ehrenreich, D., Vidal-Madjar, A., Widemann, T., Grono, G., Tanga, P., Barthelemy, M., et al. (2011). VizieR online data catalog: Transmission spectrum of Venus (Ehrenreich+, 2012). VizieR Online Data Catalog , J/A+A/537/L2.
Ehrenreich, D., Vidal-Madjar, A., Widemann, T., Gronoff, G., Tanga, P., Barthélemy, M., et al. (2012). Transmission spectrum of Venus as a transiting exoplanet, 537, L2. doi:10.1051/0004-6361/201118400
Espinoza, N., Rackham, B. V., Jordán, A., Apai, D., López-Morales, M., Osip, D. J., et al. (2018). Access: A featureless optical transmission spectrum for WASP-19b from magellan/IMACS. Mon. Notices R. Astronomical Soc.482, 2065–2087. doi:10.1093/mnras/sty2691
Evans, T. M., Sing, D. K., Kataria, T., Goyal, J., Nikolov, N., Wakeford, H. R., et al. (2017). An ultrahot gas-giant exoplanet with a stratosphere548, 58–61. doi:10.1038/nature23266
Falco, A., Zingales, T., Pluriel, W., and Leconte, J. (2022). Toward a multidimensional analysis of transmission spectroscopy. Astronomy Astrophysics658, A41. doi:10.1051/0004-6361/202141940
Fiorino, S. T., Randall, R. M., Via, M. F., and Burley, J. L. (2014). Validation of a uv-to-rf high-spectral-resolution atmospheric boundary layer characterization tool. J. Appl. Meteorology Climatol.53, 136–156. doi:10.1175/JAMC-D-13-036.1
Fisher, C., and Heng, K. (2019). How much information does the sodium doublet encode? Retrieval analysis of non-lte sodium lines at low and high spectral resolutions. Astrophysical J.881, 25. doi:10.3847/1538-4357/ab29e8
Fisher, C., Hoeijmakers, H. J., Kitzmann, D., Márquez-Neila, P., Grimm, S. L., Sznitman, R., et al. (2020). Interpreting high-resolution spectroscopy of exoplanets using cross-correlations and supervised machine learning. Astronomical J.159, 192. doi:10.3847/1538-3881/ab7a92
Fortney, J. J., Marley, M. S., Lodders, K., Saumon, D., and Freedman, R. (2005). Comparative planetary atmospheres: Models of TrES-1 and HD 209458b. Astrophysical J.627, L69–L72. doi:10.1086/431952
Fortney, J. J., Shabram, M., Showman, A. P., Lian, Y., Freedman, R. S., Marley, M. S., et al. (2010). Transmission spectra of three-dimensional hot jupiter model atmospheres. Astrophysical J.709, 1396–1406. doi:10.1088/0004-637x/709/2/1396
Frankenberg, C., Platt, U., and Wagner, T. (2005). Retrieval of co from sciamachy onboard envisat: Detection of strongly polluted areas and seasonal patterns in global co abundances. Atmos. Chem. Phys.5, 1639–1644. doi:10.5194/acp-5-1639-2005
Gandhi, S., and Madhusudhan, N. (2017). Retrieval of exoplanet emission spectra with HyDRA. Mon. Notices R. Astronomical Soc.474, 271–288. doi:10.1093/mnras/stx2748
Gibson, N. P., Merritt, S., Nugroho, S. K., Cubillos, P. E., de Mooij, E. J. W., Mikal-Evans, T., et al. (2020). Detection of Fei in the atmosphere of the ultra-hot Jupiter WASP-121b, and a new likelihood-based approach for Doppler-resolved spectroscopy. Mon. Notices R. Astronomical Soc.493, 2215–2228. doi:10.1093/mnras/staa228
Gimeno García, S., Schreier, F., Lichtenberg, G., and Slijkhuis, S. (2011). Near infrared nadir retrieval of vertical column densities: Methodology and application to sciamachy. Atmos. Meas. Tech.4, 2633–2657. doi:10.5194/amt-4-2633-2011
Gloudemans, A. M. S., Schrijver, H., Kleipool, Q., van den Broek, M. M. P., Straume, A. G., Lichtenberg, G., et al. (2005). The impact of sciamachy near-infrared instrument calibration on ch4 and co total columns. Atmos. Chem. Phys.5, 2369–2383. doi:10.5194/acp-5-2369-2005
Gordley, L. L., Marshall, B. T., and Allen Chu, D. (1994). Linepak: Algorithms for modeling spectral transmittance and radiance. J. Quantitative Spectrosc. Radiat. Transf.52, 563–580. doi:10.1016/0022-4073(94)90025-6
Govaerts, Y., Nollet, Y., and Leroy, V. (2022). Radiative transfer model comparison with satellite observations over ceos calibration site Libya-4. Atmosphere13, 1759. doi:10.3390/atmos13111759
Gropp, W., Huss-Lederman, S., Lumsdaine, A., Lusk, E., Nitzberg, B., Saphir, W., et al. (1998). MPI - The Complete Reference, 2. The MIT Press.
Handley, W. J., Hobson, M. P., and Lasenby, A. N. (2015a). polychord: nested sampling for cosmology. Mon. Notices R. Astronomical Soc. Lett.450, L61–L65. doi:10.1093/mnrasl/slv047
Handley, W. J., Hobson, M. P., and Lasenby, A. N. (2015b). polychord: next-generation nested sampling. Mon. Notices R. Astronomical Soc.453, 4385–4399. doi:10.1093/mnras/stv1911
Hartogh, P., Błecka, M. I., Jarchow, C., Sagawa, H., Lellouch, E., de Val-Borro, M., et al. (2010). First results on Martian carbon monoxide fromHerschel/HIFI observations. Herschel/HIFI Obs.521, L48. doi:10.1051/0004-6361/201015159
Hartogh, P. (1989). Chirptransformations-Spektrometer Fur die passive Millimeterwellenradiometrie: Messung der 142 GHz Emissionslinie des atmospharischen Ozons. Ph.D. thesis. Göttingen, Germany: Georg-August-Universität Göttingen.
Hayes, J. J. C., Kerins, E., Awiphan, S., McDonald, I., Morgan, J. S., Chuanraksasat, P., et al. (2020). Optimizing exoplanet atmosphere retrieval using unsupervised machine-learning classification. Mon. Not. R. Astron. Soc.494, 4492–4508. doi:10.1093/mnras/staa978
Himes, M. D., Harrington, J., Cobb, A. D., Baydin, A. G., Soboczenski, F., O’Beirne, M. D., et al. (2022). Accurate machine-learning atmospheric retrieval via a neural-network surrogate model for radiative transfer. Planet. Sci. J.3, 91. doi:10.3847/PSJ/abe3fd
Hoepfner, M., Stiller, G. P., Kuntz, M., von Clarmann, T., Echle, G., Funke, B., et al. (1998). Karlsruhe optimized and precise radiative transfer algorithm: II. Interface to retrieval applications. Interface Retr. Appl.3501, 186–195. doi:10.1117/12.317753
Hollis, M., Tessenyi, M., and Tinetti, G. (2013). tau: A 1d radiative transfer code for transmission spectroscopy of extrasolar planet atmospheres. Comput. Phys. Commun.184, 2351–2361. doi:10.1016/j.cpc.2013.05.011
Howe, A. R., Burrows, A., and Deming, D. (2017). An information-theoretic approach to optimize jwst observations and retrievals of transiting exoplanet atmospheres. Astrophysical J.835, 96. doi:10.3847/1538-4357/835/1/96
Howe, A. R., McElwain, M. W., and Mandell, A. M. (2022). Gj 229b: Solving the puzzle of the first known t dwarf with the apollo retrieval code. Astrophysical J.935, 107. doi:10.3847/1538-4357/ac5590
Ih, J., and Kempton, E. M.-R. (2021). Understanding the effects of systematics in exoplanetary atmospheric retrievals. Astronomical J.162, 237. doi:10.3847/1538-3881/ac173b
Irwin, P. G. J., Teanby, N. A., de Kok, R., Fletcher, L. N., Howett, C. J. A., Tsang, C. C. C., et al. (2008). The NEMESIS planetary atmosphere radiative transfer and retrieval tool. J. Quant. Spectrosc. Radiat. Transf.109, 1136–1150. doi:10.1016/j.jqsrt.2007.11.006
Jarchow, C. (1998). Bestimmung atmosphärischer wasserdampf-und ozone profile mittels bodengebundener millimeterwellen-fernerkundung.
Johnsen, T. K., Marley, M. S., and Gulick, V. C. (2020). A multilayer perceptron for obtaining quick parameter estimations of cool exoplanets from geometric albedo spectra. Publ. Astronomical Soc. Pac.132, 044502. doi:10.1088/1538-3873/ab740d
Kawahara, H., Kawashima, Y., Masuda, K., Crossfield, I. J. M., Pannier, E., and van den Bekerom, D. (2022). Autodifferentiable spectrum model for high-dispersion characterization of exoplanets and Brown dwarfs. Astrophysical J. Suppl. Ser.258, 31. doi:10.3847/1538-4365/ac3b4d
Kitzmann, D., Heng, K., Oreshenko, M., Grimm, S. L., Apai, D., Bowler, B. P., et al. (2020). Helios-r2: A new bayesian, open-source retrieval model for Brown dwarfs and exoplanet atmospheres. Astrophysical J.890, 174. doi:10.3847/1538-4357/ab6d71
Lacy, B. I., and Burrows, A. (2020). JWST transit spectra. i. exploring potential biases and opportunities in retrievals of tidally locked hot jupiters with clouds and hazes. Astrophysical J.905, 131. doi:10.3847/1538-4357/abc01c
Lavie, B., Mendonça, J. M., Mordasini, C., Malik, M., Bonnefoy, M., Demory, B.-O., et al. (2017). HELIOS–RETRIEVAL:An open-source, nested sampling atmospheric retrieval code; application to the HR 8799 exoplanets and inferred constraints for planet formation. Astronomical J.154, 91. doi:10.3847/1538-3881/aa7ed8
Lee, E. K. H., Wardenier, J. P., Prinoth, B., Parmentier, V., Grimm, S. L., Baeyens, R., et al. (2022). 3D radiative transfer for exoplanet atmospheres. gCMCRT: A GPU-accelerated mcrt code. gCMCRT A GPU-accelerated MCRT Code929, 180. doi:10.3847/1538-4357/ac61d6
Lee, J.-M., Fletcher, L. N., and Irwin, P. G. J. (2011). Optimal estimation retrievals of the atmospheric structure and composition of HD 189733b from secondary eclipse spectroscopy. Mon. Notices R. Astronomical Soc.420, 170–182. doi:10.1111/j.1365-2966.2011.20013.x
Lee, K.-S., Lee, C. S., Seo, M., Choi, S., Seong, N.-H., Jin, D., et al. (2020). Improvements of 6s look-up-table based surface reflectance employing minimum curvature surface method. Asia-Pacific J. Atmos. Sci.56, 235–248. doi:10.1007/s13143-019-00164-3
Lellouch, E., Gurwell, M., Butler, B., Fouchet, T., Lavvas, P., Strobel, D., et al. (2017). Detection of CO and HCN in pluto’s atmosphere with ALMA. Icarus286, 289–307. doi:10.1016/j.icarus.2016.10.013
Li, C., Le, T., Zhang, X., and Yung, Y. L. (2018). A high-performance atmospheric radiation package: With applications to the radiative energy budgets of giant planets. J. Quantitative Spectrosc. Radiat. Transf.217, 353–362. doi:10.1016/j.jqsrt.2018.06.002
Lin, Z., Stamnes, S., Li, W., Hu, Y., Laszlo, I., Tsay, S.-C., et al. (2022). Polarized radiative transfer simulations: A tutorial review and upgrades of the vector discrete ordinate radiative transfer computational tool. Front. Remote Sens.3. doi:10.3389/frsen.2022.880768
Line, M. R., Wolf, A. S., Zhang, X., Knutson, H., Kammer, J. A., Ellison, E., et al. (2013). A systematic retrieval analysis of secondary eclipse spectra. I. A Comp. Atmos. Retr. Tech.775, 137. doi:10.1088/0004-637X/775/2/137
Line, M. R., Zhang, X., Vasisht, G., Natraj, V., Chen, P., and Yung, Y. L. (2012). Information content of exoplanetary transit spectra: AN initial look. Astrophysical J.749, 93. doi:10.1088/0004-637x/749/1/93
López-Puertas, M., Montañés-Rodríguez, P., Pallé, E., Höpfner, M., Sánchez-López, A., García-Comas, M., et al. (2018). Aerosols and water ice in jupiter’s stratosphere from UV-nir ground-based observations. Astron. J.156, 169. doi:10.3847/1538-3881/aadcef
Lothringer, J. D., and Barman, T. S. (2020). The PHOENIX exoplanet retrieval algorithm and using H− opacity as a probe in ultrahot jupiters. Astronomical J.159, 289. doi:10.3847/1538-3881/ab8d33
Lupu, R. E., Marley, M. S., Lewis, N., Line, M., Traub, W. A., and Zahnle, K. (2016). Developing atmospheric retrieval methods for direct imaging spectroscopy of gas giants in reflected light. i. methane abundances and basic cloud properties. Astronomical J.152, 217. doi:10.3847/0004-6256/152/6/217
Lustig-Yaeger, J., Sotzen, K. S., Stevenson, K. B., Luger, R., May, E. M., Mayorga, L. C., et al. (2022). Hierarchical bayesian atmospheric retrieval modeling for population studies of exoplanet atmospheres: A case study on the habitable zone. Astronomical J.163, 140. doi:10.3847/1538-3881/ac5034
MacDonald, R. J., and Batalha, N. E. (2023). A catalog of exoplanet atmospheric retrieval codes. Res. Notes AAS7, 54. doi:10.3847/2515-5172/acc46a
MacDonald, R. J., and Lewis, N. K. (2022). Trident: A rapid 3d radiative-transfer model for exoplanet transmission spectra. Astrophysical J.929, 20. doi:10.3847/1538-4357/ac47fe
MacDonald, R. J., and Madhusudhan, N. (2017). HD 209458b in new light: Evidence of nitrogen chemistry, patchy clouds and sub-solar water. Mon. Not. R. Astron. Soc.469, 1979–1996. doi:10.1093/mnras/stx804
Madhusudhan, N. (2018). Atmospheric retrieval of exoplanets. Cham: Springer International Publishing, 1–30. doi:10.1007/978-3-319-30648-3_104-1
Madhusudhan, N., Crouzet, N., McCullough, P. R., Deming, D., and Hedges, C. (2014). H2O abundances in the atmospheres of three hot jupiters, 791–L9. doi:10.1088/2041-8205/791/1/L9
Madhusudhan, N., Harrington, J., Stevenson, K. B., Nymeyer, S., Campo, C. J., Wheatley, P. J., et al. (2011). A high C/O ratio and weak thermal inversion in the atmosphere of exoplanet WASP-12b. 469, 64–67. doi:10.1038/nature09602
Madhusudhan, N., and Seager, S. (2009). A temperature and abundance retrieval method for exoplanet atmospheres, 707, 24–39. doi:10.1088/0004-637X/707/1/24
Madhusudhan, N., and Seager, S. (2010). On the inference of thermal inversions in hot jupiter atmospheres. Astrophys. J.725, 261–274. doi:10.1088/0004-637X/725/1/261
Marley, M. S., and McKay, C. P. (1999). Thermal structure of uranus’ atmosphere. Icarus138, 268–286. doi:10.1006/icar.1998.6071
Marquez-Neila, P., Fisher, C., Sznitman, R., and Heng, K. (2018). Supervised machine learning for analysing spectra of exoplanetary atmospheres. doi:10.48550/ARXIV.1806.03944
Martin-Torres, F. J., and Mlynczak, M. G. (2005). FUTBOLIN (full transfer by ordinary LINe-by-line methods): A new radiative transfer code for atmospheric calculations in the visible and infrared 2005, A21A–A05.
Martínez, F. A., Min, M., Kamp, I., and Palmer, P. I. (2022). Convolutional neural networks as an alternative to bayesian retrievals for interpreting exoplanet transmission spectra. Astron. & Astrophys.662, A108. doi:10.1051/0004-6361/202142976
Matricardi, M. (2007). An inter-comparison of line-by-line radiative transfer models. doi:10.21957/b3amji4k
Mayer, B., and Kylling, A. (2005). Technical note: The libradtran software package for radiative transfer calculations - description and examples of use. Atmos. Chem. Phys.5, 1855–1877. doi:10.5194/acp-5-1855-2005
Mendrok, J. (2006). The SARTre model for radiative transfer in spherical atmospheres and its application to the derivation of cirrus cloud properties. Berlin: Ph.D. thesis, Freie Univ.
Min, M., Ormel, C. W., Chubb, K., Helling, C., and Kawashima, Y. (2020). The ARCiS framework for exoplanet atmospheres. Model. philosophy Retr.642, A28. doi:10.1051/0004-6361/201937377
Mollière, P., Wardenier, J. P., van Boekel, R., Henning, Th., Molaverdikhani, K., and Snellen, I. A. G. (2019). Petitradtrans - a python radiative transfer package for exoplanet characterization and retrieval. A&A627, A67. doi:10.1051/0004-6361/201935470
Montañés-Rodríguez, P., González-Merino, B., Pallé, E., López-Puertas, M., and García-Melendo, E. (2015). Jupiter as an Exoplanet: UV to NIR Transmission Spectrum Reveals Hazes, a Na Layer, and Possibly Stratospheric H2O-ice Clouds, 801, L8. doi:10.1088/2041-8205/801/1/L8
Moreno, R., Marten, A., Biraud, Y., Bézard, B., Lellouch, E., Paubert, G., et al. (2001). Jovian stratospheric temperature during the two months following the impacts of comet Shoemaker–Levy 9Shoemaker-Levy 949, 473–486. doi:10.1016/S0032-0633(00)00139-2
Moreno, R. (1998). Observations millimetriques et submillimetriques des planetes geantes. Etude de jupiter apres la chute de la comete sl9. Ph.D. thesis. Thèse de doctorat dirigée par Marten, André Terre, océan, espace Paris 6 1998.
Niraula, P., de Wit, J., Gordon, I. E., Hargreaves, R. J., Sousa-Silva, C., and Kochanov, R. V. (2022). The impending opacity challenge in exoplanet atmospheric characterization. Nat. Astron.6, 1287–1295. doi:10.1038/s41550-022-01773-1
Nixon, M. C., and Madhusudhan, N. (2020). Assessment of supervised machine learning for atmospheric retrieval of exoplanets. Mon. Not. R. Astron. Soc.496, 269–281. doi:10.1093/mnras/staa1150
Nixon, M. C., and Madhusudhan, N. (2022). Aura-3D: A three-dimensional atmospheric retrieval framework for exoplanet transmission spectra. Astrophys. J.935, 73. doi:10.3847/1538-4357/ac7c09
Oreshenko, M., Lavie, B., Grimm, S. L., Tsai, S.-M., Malik, M., Demory, B.-O., et al. (2017). Retrieval Analysis of the Emission Spectrum of WASP-12b: Sensitivity of Outcomes to Prior Assumptions and Implications for Formation History, 847, L3. doi:10.3847/2041-8213/aa8acf
Piette, A. A. A., Madhusudhan, N., and Mandell, A. M. (2021). HyDRo: Atmospheric retrieval of rocky exoplanets in thermal emission. Mon. Notices R. Astronomical Soc.511, 2565–2584. doi:10.1093/mnras/stab3612
Pinhas, A., Rackham, B. V., Madhusudhan, N., and Apai, D. (2018). Retrieval of planetary and stellar properties in transmission spectroscopy with Aura. Mon. Notices R. Astronomical Soc.480, 5314–5331. doi:10.1093/mnras/sty2209
Ramon, D., Steinmetz, F., Jolivet, D., Compiègne, M., and Frouin, R. (2019). Modeling polarized radiative transfer in the ocean-atmosphere system with the gpu-accelerated smart-g Monte Carlo code. J. Quantitative Spectrosc. Radiat. Transf.222-223, 89–107. doi:10.1016/j.jqsrt.2018.10.017
Rengel, M. (2022). About the atomic and molecular databases in the planetary community. doi:10.5281/zenodo.7040446
Rengel, M., Hartogh, P., and Jarchow, C. (2008). Mesospheric vertical thermal structure and winds on Venus from HHSMT CO spectral-line observations. HHSMT Co. spectral-line Obs.56, 1368–1384. doi:10.1016/j.pss.2008.07.004
Rengel, M., Shulyak, D., Hartogh, P., Sagawa, H., Moreno, R., Jarchow, C., et al. (2022). Ground-based HCN submillimetre measurements in titan’s atmosphere: An intercomparison with herschel observations, 658, A88. doi:10.1051/0004-6361/202141422
Ricchiazzi, P., Yang, S., and Gautier, C. (2002). Sbdart: A practical tool for plane-parallel radiative transfer in the earth’s atmosphere.
Robbins-Blanch, N., Kataria, T., Batalha, N. E., and Adams, D. J. (2022). Cloudy and cloud-free thermal phase curves with picaso: Applications to wasp-43b. Astrophysical J.930, 93. doi:10.3847/1538-4357/ac658c
Robinson, T. D., Meadows, V. S., Crisp, D., Deming, D., A’Hearn, M. F., Charbonneau, D., et al. (2011). Earth as an extrasolar planet: Earth model validation using epoxi Earth observations. Astrobiology11, 393–408. doi:10.1089/ast.2011.0642
Robinson, T. D., and Salvador, A. (2023). Exploring and validating exoplanet atmospheric retrievals with solar system analog observations. Planet. Sci. J.4, 10. doi:10.3847/PSJ/acac9a
Rozanov, A., Rozanov, V., Buchwitz, M., Kokhanovsky, A., and Burrows, J. (2005). Sciatran 2.0 – A new radiative transfer model for geophysical applications in the 175–2400nm spectral region. Adv. Space Res.36, 1015–1019. doi:10.1016/j.asr.2005.03.012
Rozanov, V., Rozanov, A., Kokhanovsky, A., and Burrows, J. (2014). Radiative transfer through terrestrial atmosphere and ocean: Software package sciatran. J. Quantitative Spectrosc. Radiat. Transf.133, 13–71. doi:10.1016/j.jqsrt.2013.07.004
Saunders, R., Matricardi, M., and Brunel, P. (1999). An improved fast radiative transfer model for assimilation of satellite radiance observations. Q. J. R. Meteorological Soc.125, 1407–1425. doi:10.1002/qj.1999.49712555615
Scheucher, M., Wunderlich, F., Grenfell, J. L., Godolt, M., Schreier, F., Kappel, D., et al. (2020). Consistently simulating a wide range of atmospheric scenarios for K2-18b with a flexible radiative transfer module. Astrophys. J.898, 44. doi:10.3847/1538-4357/ab9084
Schreier, F., Gimeno García, S., Hedelt, P., Hess, M., Mendrok, J., Vasquez, M., et al. (2014). Garlic — A general purpose atmospheric radiative transfer line-by-line infrared-microwave code: Implementation and evaluation. J. Quantitative Spectrosc. Radiat. Transf.137, 29–50. doi:10.1016/j.jqsrt.2013.11.018
Schreier, F., Milz, M., Buehler, S. A., and von Clarmann, T. (2018). Intercomparison of three microwave/infrared high resolution line-by-line radiative transfer codes. J. Quantitative Spectrosc. Radiat. Transf.211, 64–77. doi:10.1016/j.jqsrt.2018.02.032
Scott, N. A. (1974). A direct method of computation of the transmission function of an inhomogeneous gaseous medium - I: Description of the method. J. Quant. Spectrosc. Radiat. Transf.14, 691–704. doi:10.1016/0022-4073(74)90116-2
Scott, N. A., and Chedin, A. (1981). A fast line-by line method for atmospheric absorption computations: The automatized atmospheric absorption atlas. J. Appl. Meteorology20, 802–812. doi:10.1175/1520-0450(1981)020⟨0802:AFLBLM⟩2.0.CO;2
Seidel, J. V., Ehrenreich, D., Pino, L., Bourrier, V., Lavie, B., Allart, R., et al. (2020). Wind of change: Retrieving exoplanet atmospheric winds from high-resolution spectroscopy. A&A633, A86. doi:10.1051/0004-6361/201936892
Shulyak, D., Lara, L. M., Rengel, M., and Nèmec, N. E. (2020). Stellar impact on disequilibrium chemistry and observed spectra of hot Jupiter atmospheres, 639, A48. doi:10.1051/0004-6361/201937210
Shulyak, D., Rengel, M., Reiners, A., Seemann, U., and Yan, F. (2019). Remote sensing of exoplanetary atmospheres with ground-based high-resolution near-infrared spectroscopy, 629, A109. doi:10.1051/0004-6361/201935691
Smith, H. J. P., Dube, D. J., Gardner, M. E., Clough, S. A., and Kneizys, F. X. (1978). Fascode: Fast Atmospheric Signature CODE (spectral transmittance and radiance).
Soboczenski, F., Himes, M. D., O’Beirne, M. D., Zorzan, S., Gunes Baydin, A., Cobb, A. D., et al. (2018). Bayesian deep learning for exoplanet atmospheric retrieval. arXiv e-prints , arXiv:1811.03390.
Speagle, J. S. (2020). dynesty: a dynamic nested sampling package for estimating Bayesian posteriors and evidences. Mon. Notices R. Astronomical Soc.493, 3132–3158. doi:10.1093/mnras/staa278
Spurr, R., and Christi, M. (2019). The LIDORT and VLIDORT linearized scalar and vector discrete ordinate radiative transfer models: Updates in the last 10 years. Cham: Springer International Publishing, 1–62. doi:10.1007/978-3-030-03445-0_1
Stamnes, K., Hamre, B., Stamnes, S., Chen, N., Fan, Y., Li, W., et al. (2018). Progress in forward-inverse modeling based on radiative transfer tools for coupled atmosphere-snow/ice-ocean systems: A review and description of the accurt model. Appl. Sci.8, 2682. doi:10.3390/app8122682
Stamnes, K., Tsay, S. C., Wiscombe, W. J., and Laszlo, I. (2000). Disort: A general-purpose fortran program for discrete ordinate-method radiative transfer in scattering and emitting layered media: Documentation of methodology. Greenbelt, United States: Nasa report, NASA.
Stamnes, K., Tsay, S., Wiscombe, W., and Jayaweera, K. (1988). Numerically stable algorithm for discrete-ordinate-method radiative transfer in multiple scattering and emitting layered media. Appl. Opt.27, 2502–2509. doi:10.1364/ao.27.002502
Stiller, G. P., Hoepfner, M., Kuntz, M., von Clarmann, T., Echle, G., Fischer, H., et al. (1998). Karlsruhe optimized and precise radiative transfer algorithm. Part I: Requirements, justification, and model error estimation. Justif. Model. error Estim.3501, 257–268. doi:10.1117/12.317754
Stiller, G. P. (2000). The Karlsruhe optimized and precise radiative transfer algorithm. Karlsruhe, Germany: Tech. rep. LK 01; Wissenschaftliche Berichte, FZKA-6487 (Dezember 2000). doi:10.5445/IR/270048971.22.02.11
Stiller, G. P., von Clarmann, T., Funke, B., Glatthor, N., Hase, F., Höpfner, M., et al. (2002). Sensitivity of trace gas abundances retrievals from infrared limb emission spectra to simplifying approximations in radiative transfer modelling. J. Quantitative Spectrosc. Radiat. Transf.72, 249–280. doi:10.1016/S0022-4073(01)00123-6
Stolker, T., Quanz, S. P., Todorov, K. O., Kühn, J., Mollière, P., Meyer, M. R., et al. (2020). Miracles: Atmospheric characterization of directly imaged planets and substellar companions at 4–5 μm: I. Photometric analysis of β pic b, HIP 65426 b, PZ tel B, and HD 206893 B. A&A635, A182. doi:10.1051/0004-6361/201937159
Strow, L., Hannon, S., De Souza-Machado, S., Motteler, H., and Tobin, D. (2003). An overview of the airs radiative transfer model. IEEE Trans. Geoscience Remote Sens.41, 303–313. doi:10.1109/TGRS.2002.808244
Swain, M. R., Line, M. R., and Deroo, P. (2014). On the detection of molecules in the atmosphere of hd 189733b using hst nicmos transmission spectroscopy. Astrophysical J.784, 133. doi:10.1088/0004-637X/784/2/133
Tinetti, G., Meadows, V. S., Crisp, D., Fong, W., Fishbein, E., Turnbull, M., et al. (2006a). Detectability of planetary characteristics in disk-averaged spectra. I: The earth model. Astrobiology6, 34–47. doi:10.1089/ast.2006.6.34
Tinetti, G., Meadows, V. S., Crisp, D., Kiang, N., Kahn, B. H., Velusamy, T., et al. (2006b). Detectability of planetary characteristics in disk-averaged spectra II: Synthetic spectra and light-curves of earth. Astrobiology6, 881–900. doi:10.1089/ast.2006.6.881
Tremblin, P., Amundsen, D. S., Mourier, P., Baraffe, I., Chabrier, G., Drummond, B., et al. (2015). Fingering convection and cloudless models for cool Brown dwarf atmospheres, 804, L17. doi:10.1088/2041-8205/804/1/L17
Urban, J., Baron, P., Lautié, N., Schneider, N., Dassas, K., Ricaud, P., et al. (2004). Moliere (v5): A versatile forward- and inversion model for the millimeter and sub-millimeter wavelength range. J. Quantitative Spectrosc. Radiat. Transf.83, 529–554. doi:10.1016/S0022-4073(03)00104-3
Vandaele, A., Kruglanski, M., and Maziere, D. (2006b). Modeling and retrieval of atmospheric spectra using asimut. Atmos. Sci. Conf.628, 71.
Vandaele, A., Kruglanski, M., and De Mazière, M. (2006a). Modeling and retrieval of atmospheric spectra using asimut. European Space Agency. (Special Publication) ESA SP.
Vasist, M., Rozet, F., Absil, O., Mollière, P., Nasedkin, E., and Louppe, G. (2023). Neural posterior estimation for exoplanetary atmospheric retrieval.
Villanueva, G. L., Liuzzi, G., Faggi, S., Protopapa, S., Kofman, V., Fauchez, T., et al. (2022). Fundamentals of the planetary spectrum generator.
Villanueva, G. L., Mumma, M. J., Novak, R. E., Käufl, H. U., Hartogh, P., Encrenaz, T., et al. (2015). Strong water isotopic anomalies in the martian atmosphere: Probing current and ancient reservoirs. Science348, 218–221. doi:10.1126/science.aaa3630
Villanueva, G. L., Smith, M. D., Protopapa, S., Faggi, S., and Mandell, A. M. (2018). Planetary Spectrum Generator: An accurate online radiative transfer suite for atmospheres, comets, small bodies and exoplanets. small bodies Exopl.217, 86–104. doi:10.1016/j.jqsrt.2018.05.023
Wakeford, H. R., Sing, D. K., Kataria, T., Deming, D., Nikolov, N., Lopez, E. D., et al. (2017). HAT-P-26b: A neptune-mass exoplanet with a well-constrained heavy element abundance. Science356, 628–631. doi:10.1126/science.aah4668
Waldmann, I. P., Rocchetto, M., Tinetti, G., Barton, E. J., Yurchenko, S. N., and Tennyson, J. (2015a). Tau-REx II: Retrieval of emission spectra. Astrophys. J.813, 13. doi:10.1088/0004-637X/813/1/13
Waldmann, I. P., Tinetti, G., Rocchetto, M., Barton, E. J., Yurchenko, S. N., and Tennyson, J. (2015b). Tau-REx I: A next generation retrieval code for exoplanetary atmospheres. Astrophys. J.802, 107. doi:10.1088/0004-637X/802/2/107
Welbanks, L., and Madhusudhan, N. (2021). Aurora: A generalized retrieval framework for exoplanetary transmission spectra. Astrophysical J.913, 114. doi:10.3847/1538-4357/abee94
Welbanks, L., and Madhusudhan, N. (2019). On degeneracies in retrievals of exoplanetary transmission spectra. Astronomical J.157, 206. doi:10.3847/1538-3881/ab14de
Weng, F., Yu, X., Duan, Y., Yang, J., and Wang, J. (2020). Advanced radiative transfer modeling system (ARMS): A new-generation satellite observation operator developed for numerical weather prediction and remote sensing applications. Adv. Atmos. Sci.37, 131–136. doi:10.1007/s00376-019-9170-2
Yip, K. H., Changeat, Q., Al-Refaie, A., and Waldmann, I. (2022). To sample or not to sample: Retrieving exoplanetary spectra with variational inference and normalising flows.
Zawada, D. J., Dueck, S. R., Rieger, L. A., Bourassa, A. E., Lloyd, N. D., and Degenstein, D. A. (2015). High-resolution and Monte Carlo additions to the sasktran radiative transfer model. Atmos. Meas. Tech.8, 2609–2623. doi:10.5194/amt-8-2609-2015
Zhang, M., Chachan, Y., Kempton, E. M. R., Knutson, H. A., and Chang, W. H. (2020). Platon II: New capabilities and a comprehensive retrieval on HD 189733b transit and eclipse data. Transit Eclipse Data899, 27. doi:10.3847/1538-4357/aba1e6
Zhang, X. (2020). Atmospheric regimes and trends on exoplanets and Brown dwarfs. Res. Astronomy Astrophysics20, 099. doi:10.1088/1674-4527/20/7/99
Keywords: atmospheres, radiative transfer, planets and satellites, retrieval, exoplanets
Citation: Rengel M and Adamczewski J (2023) Radiative transfer and inversion codes for characterizing planetary atmospheres: an overview. Front. Astron. Space Sci. 10:1176740. doi: 10.3389/fspas.2023.1176740
Received: 28 February 2023; Accepted: 15 May 2023;
Published: 30 May 2023.
Edited by:
Josep M. Trigo-Rodríguez, Spanish National Research Council (CSIC), SpainReviewed by:
Knut Stamnes, Stevens Institute of Technology, United StatesCopyright © 2023 Rengel and Adamczewski. This is an open-access article distributed under the terms of the Creative Commons Attribution License (CC BY). The use, distribution or reproduction in other forums is permitted, provided the original author(s) and the copyright owner(s) are credited and that the original publication in this journal is cited, in accordance with accepted academic practice. No use, distribution or reproduction is permitted which does not comply with these terms.
*Correspondence: M. Rengel, cmVuZ2VsQG1wcy5tcGcuZGU=