- 1NASA Goddard Space Flight Center, Greenbelt, MD, United States
- 2ADNET Systems, Inc., Greenbelt, MD, United States
- 3Department of Physics, Catholic University of America, Washington, DC, United States
- 4Department of Physics, Aberystwyth University, Ceredigion, United Kingdom
- 5Southwest Research Institute, Boulder, CO, United States
- 6Center for Astrophysics, Cambridge, MA, United States
- 7Physics and Astronomy, College of Science, George Mason University, Fairfax, VA, United States
- 8Johns Hopkins U. Applied Physics Laboratory, Laurel, MD, United States
Establishing the Sun-Earth connection requires overcoming the challenges of exploring the data from past and current missions and leveraging tools and models (data mining) to create an efficient system treatment of the Sun and heliosphere. However, solar and heliospheric environment data constitute a vast source of information whose potential is far from being optimally exploited. In the next decade, the solar and heliospheric community will have to manage the increasing amount of information coming from new missions, improve re-analysis of data from past and current missions, and create new data products from the application of new methodologies. This complex task is further complicated by practical challenges such as different datasets and catalogs in different formats that may require different pre-processing and analysis tools, and the need for numerous analysis approaches that are not all fully optimized for large volumes of data. While several ongoing efforts aim at addressing these problems, the available datasets and tools are not always used to their full potential often due to lack of awareness of available resources. In this paper, we summarize the issues raised and goals discussed by members of the community during recent conference sessions focused on data mining for science.
1 Introduction
Increasingly, diverse data sets are being used to understand the Sun-Earth connection. Data analysis tools applied to remote-sensing solar measurements, e.g., GOES/X-ray flux (Hanser and Sellers, 1996); RHESSI (Lin et al., 2002); SDO (Pesnell et al., 2012); STEREO (Kaiser et al., 2008), and in situ heliospheric measurements, e.g., ACE (McComas et al., 1998); Wind (Harten and Clark, 1995); SOHO (Domingo et al., 1995), contribute crucial information to our understanding of the Sun/Corona/Heliosphere as a single physical system. This includes understanding the response of the Earth’s magnetosphere-ionosphere-thermosphere system further supported by satellite constellations (e.g., Cluster, Escoubet et al., 1997; THEMIS, Angelopoulos, 2008; MMS, Burch et al., 2016), geostationary and low-Earth-orbit satellites (e.g., GOES, Sullivan, 2020; Swarm, Friis-Christensen et al., 2006), and ground based assets [e.g., magnetometers (Gjerloev, 2012) and radars (Greenwald et al., 1995)]. The examples of solar-terrestrial missions are hardly exhaustive or representative of past, current, and future missions and ground-based assets across many international agencies. Figure 1 shows NASA’s Heliophysics fleet of spacecraft from the Sun to the Earth, ESA’s fleet in the Solar System and NOAA’s fleet of satellite missions. The availability of multiple datasets from these missions (and concluded missions not shown) is matched by a plethora of analysis and processing techniques, all of which extract useful information from the observations (Paschmann and Schwartz, 2000; Ireland and Young, 2009; Dunlop and Lühr, 2020). These include advanced image processing techniques to reveal structural detail, inversion methods for physical values (e.g., density, temperature), robust statistical approaches, feature recognition and tracking, and processing of data for use as boundary conditions for models. Moreover, increases in processor speed and massively parallel computational techniques have enabled improvements in the possible spatio-temporal resolution of analysis methods and models (Camporeale, 2019). The consequent improvements in physics-based numerical simulations have also furthered our understanding of the underlying heliosphere physical processes. On the other hand, robust statistical approaches to data analysis can give new insight into new and historical datasets (e.g., Di Matteo and Sivadas, 2022; Lockwood et al., 2022; Sivadas and Sibeck, 2022). These arguments point to the increasing need for improved standardization for analysis tools particularly those that combine multiple-source data (Burrell et al., 2018). Overcoming the challenges of past/current missions and exloiting past (historic) and current data, tools and models will set the basis for a system treatment of the heliosphere and thus create the synergy between different Heliophysics fields enabling solar-terrestrial science. However, current datasets and tools are not always used to their full potential often due to lack of awareness of available resources. This paper describes some of the challenges raised and future goals discussed in recent conference sessions focused on data mining for solar-terrestrial science. The primary question we aim to highlight is: How can our community of scientists, engineers, computer scientists work together to improve the various datasets/tools/models available, in order to maximize the information extracted from observations/measurements/simulations/etc., and to study the Sun-Earth connection as a single system?
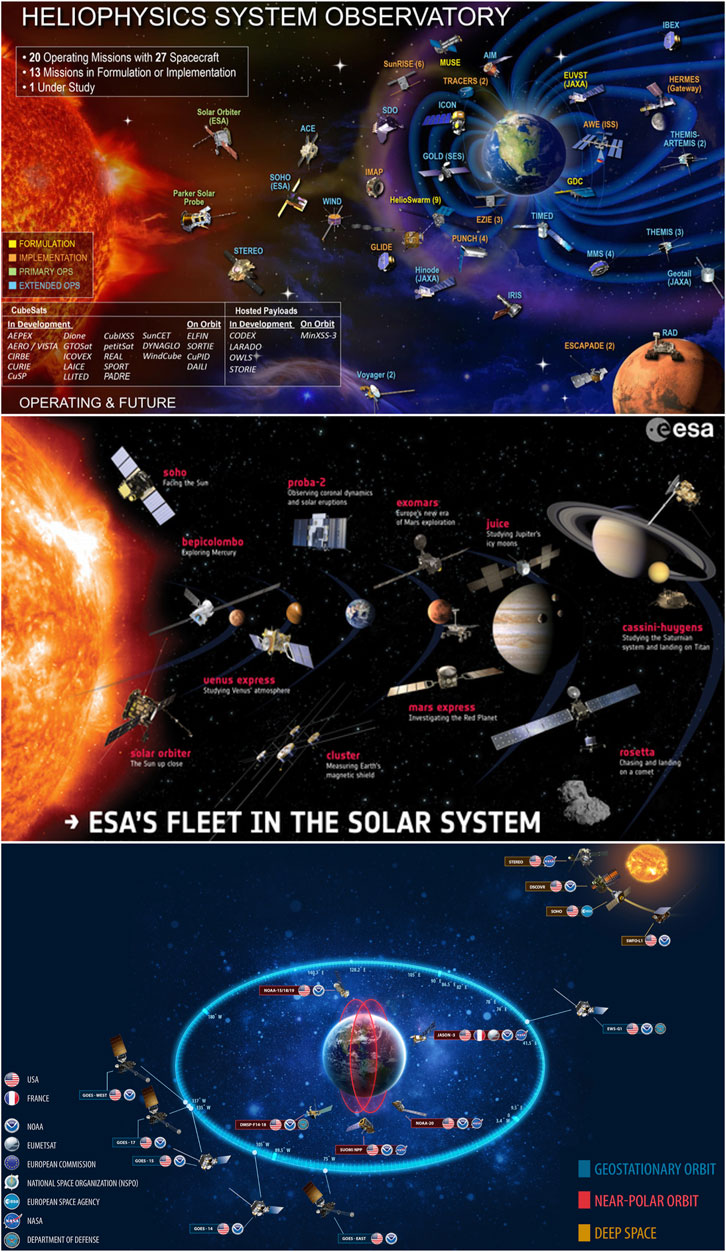
FIGURE 1. Example showing space fleet of three space agencies. Top) NASA’s Heliophysics fleet of solar, heliospheric, geospace and planetary spacecraft. Only currently operating missions are shown here. Credit: NASA’s Goddard Space Flight Center: https://svs.gsfc.nasa.gov/30822. Middle) ESA’s fleet in the Solar System. Credit: ESA: https://www.esa.int/About_Us/ESAC/Extended_life_for_ESA_s_science_missions. Bottom) NOAA’s fleet of satellite missions. Credit: NOAA: https://nesdis-prod.s3.amazonaws.com/2023-01/NOAA_Satellite_System.2023.01.04_0.png. Other examples include: JAXA’s fleet and ISRO’s fleet.
2 Current challenges and needs
2.1 Data and computational challenges and needs
Data mining (turning raw data into useful information) is fundamental for integration of key parameters in models, comparison of model output with data and fostering the development of new tools, as needed. Among the various data and computational challenges in Heliophysics, here we provide examples specific to solar remote sensing observations but relatable across solar-terrestrial disciplines.
Extraction of useful information from remote sensing observations is essential for exploring the physical properties of the plasma to then be compared with models and, when possible, in situ measurements. For example, emission from spectral lines from different charge states of the same element serve as a diagnostic for electron temperature (e.g., Habbal et al., 2010). Other examples include the use of polarimetric observations in select spectral lines for coronal magnetometry (see Judge, 1998; Lin et al., 2000).
Fully extracting useful information from observational data requires advanced image/signal processing techniques. For example, both visible-light and extreme ultraviolet (EUV) observations benefit from recent advances in image processing algorithms, to separate the faint scientific signal from much brighter backgrounds from stray light and other non-solar signals; this separation is made more difficult by the observed steep decreases in plasma density/emission with heliocentric distance from the Sun. For example, previous studies (Stenborg et al., 2008; Morgan et al., 2013; Alzate and Morgan, 2016, 2017; Alzate et al., 2023a, 2021) have focused on the application of advanced image processing techniques to EUV and coronagraph data to reveal fine-scale signatures in the low corona that have an impact on the structure of the extended corona, which would otherwise go unnoticed.
Unraveling the physics of the Sun-Earth connection requires multi-point and multi-spacecraft observations supported by numerical simulations and models. For example, currently no instrument independently spans an uninterrupted FOV from the low corona out to several solar radii. One way is to combine remote sensing data from different instrument suites to create “maps” to reveal the connection among activity at various heights in the corona and the heliosphere (e.g., DeForest et al., 2013; Alzate et al., 2023a, 2021). Other commonly used tools for extracting information from dynamic events include, for example, J-maps (Sheeley et al., 1999), Huygens plotting (Wills-Davey and Thompson, 1999) and persistent mapping (Thompson and Young, 2016). Developing techniques for application on current datasets will prove valuable in interpreting future datasets. For example, both Solar Orbiter (SO; Müller et al., 2013) and the Daniel K. Inouye Solar Telescope (DKIST; Tritschler et al., 2016) would benefit from contextual imagery and modeling to fully utilize their capabilities and expand their science outcomes. Current missions in development, such as PUNCH Polarimeter to UNify the Corona and Heliosphere (PUNCH), 2023 extend remote sensing data to wide distances from the Sun out to Earth through wide-field observations of the tenuous solar wind plasma.
Indeed, new missions present new challenges to the data analysis capabilities of the solar and heliospheric community. The spatial/temporal resolution of observations continues to improve with new instrumentation. This leads to high, petabyte-sized, volumes of data, which test the limits of current computational capabilities, and require compromises between the amount of information that can be gathered in time and space versus the amount of information actually used for science. For example, SO is an “encounter” mission that strictly limits the duration of data collection forcing the intrument teams into protracted and complex planning exercises to optimize the science return. The DKIST, on the other hand, will produce up to 15 Tb per day (https://dkist.nso.edu/node/1819), with respect to a very small region of the Sun.
The emerging data science and parallel computing capabilities, combined with the large amount of data that will be available from near future and future missions, will provide unprecedented opportunities and challenges (Camporeale, 2019). Coordination between missions is necessary to ensure that mission-specific tools are interoperable and can be used to merge output from complementary efforts; this enables breakthrough cross-mission science, that is, necessarily outside the narrowly defined scientific scope of each mission, as well as improving the costs for software development within each mission. However, future missions in isolation will not fully solve science and problems but developing tools to seamlessly merge output from complementary efforts will greatly enhance the performance of future missions.
2.2 Community challenges and needs
The challenges outlined in Section 2.1 have been the focus of discussion sessions in recent scientific meetings [e. g., the Solar Heliospheric and INterplanetary Environment (SHINE) Workshop 2019/2022 and the Triennial Earth-Sun Summit (TESS) Meeting 2022]. During the SHINE 2022 meeting, we ran a session on Data Mining for Science performed in the format of open discussion. The purpose of the session was to foster discussions among researchers in the solar and heliospheric community on accessibility and interoperability of various observations, analysis tools and models for extracting information about the Sun-Earth connection as a single system. Additionally, discussions were held on advanced analysis and processing procedures, including machine learning methods, and their integration into next-generation instruments. Current limitations and challenges faced by the community, considered to be the result of the vast number of methods/tools/datasets that are not fully/equally distributed/disseminated, were identified as follows.
• Different datasets and catalogs are in different formats and might require different pre-processing and analysis tools based on the science questions asked (e.g., Candey et al., 2018; Antunes et al., 2022; Hurlburt et al., 2012).
• Different datasets often cover different physical regions (see Figure 1); standardization of pre-processing method and analysis tools might enable routine extraction and/or inference of physical quantities of interest, from different datasets even though covering different physical regions, to be assimilated for modeling purposes (e.g., Geospace Data Assimilation Working Group GeoDAWG, 2023).
• Configurations of spacecraft and ground-based instruments are constrained by science operations, which limits cross-mission investigations (e.g., in situ and remote sensing observations).
• Numerous analysis approaches (e.g., image processing, time series analysis) are not all fully optimized for petabyte-sized data volumes.
• Even though the publicly available, open source tools for heliophysics are increasing in number [e.g., Python in Heliophysics Community, pyHC, Burrell et al. (2018); Barnum et al. (2022)], there is a lack of awareness of the available resources.
During our series of community forums, one of the main points raised was the cataloging of observations and simulation outputs in standardized formats of both data and metadata on an international level. The exchange of information included in the metadata is fundamental for data integration in models, comparison of model output with data, and, most importantly, for the development of new tools, as needed. During the discussions, we collected a series of key points. Regarding data management, the main suggestions were.
• The need for standardized data formats since a unique repository collecting large amounts of data is difficult and expensive to maintain.
• Data should be accompanied by clear documentation, with capabilities similar to Jupyter notebooks, with data handling examples.
• Updates on instrument conditions, new data products, caveats, etc., stemming from increased insight on missions over time, should be collected in a centralized repository for easy access to the international community and not remain behind published paywalls.
Another identified challenge was that groups/individuals develop and publish results using their own research software, with rather unsophisticated approach to open-source sharing. Most of these codes have dependencies on other software libraries, but without careful package curation and maintenance, these dependencies can become tuned to individual researchers’ local libraries, and thus impede collaborations among users.
3 Current efforts
One of the first steps in addressing some of the challenges mentioned, is the cataloging of observations and simulation outputs in standardized formats. For example, data and metadata already have a strong degree of uniformity for NASA missions following NASA ISTP metadata model and NASA CDF data format or the large effort made in recent decades to standardize Flexible Image Transport System (FITS; Wells et al., 1981) (Metadata standardization). However, the interoperability between data/metadata missions is more challenging across international agencies using different formats (e.g., ESPAS—Near-earth space data infrastructure for e-science, Häggström, 2014). The Space Physics Archive Search and Extract metadata model is one current community effort toward addressing this challenge (Roberts et al., 2018). Standardization efforts can also be focused on specific sets of datasets like the JPL Spice kernels for spacecraft and planetary ephemeris and the World Coordinate System (WCS; Greisen and Calabretta, 2002) part of Solarsoft (SSW; Freeland and Handy, 1998) for data coordinates and transforms [there is also a Python implementation available at pyHC, Burrell et al. (2018); Barnum et al. (2022)].
Standardization of software and tools include efforts on open-source libraries like Solarsoft Freeland and Handy (1998) and Sunpy Barnes et al. (2023) as well as SPEDAS (Angelopoulos et al., 2019) and pySPEDAS (Grimes et al., 2022). These efforts have been invaluable in research, with excellent value-for-money compared to the overall cost of missions. Frameworks where people’s research analysis software can be more widely accessed, with ease-of-use and some standardized approaches, are already available, for example, pyHC (Burrell et al., 2018; Barnum et al., 2022) or online repositories such as Github is used as the industry standard for code libraries and source control.
During the TESS 2022 discussion session, members of the community presented various tools. Each of these tools have contributed significantly in improving how data are shared and handled, providing valuable perspective on the future practices that our community should pursue. Among the showcased tools were.
• The custom model runs available through the (Hesse et al., 2002; Community Coordinated Modeling CenterCCMC, 2016).
• The HelioCloud platform (Thomas et al., 2022), an open cloud-based research platform for the research community to better democratize access to very large datasets with associated compute to enable big data research. HelioCloud can handle multi-petabytes of science volumes and can facilitate open science publishing and collaboration among different teams.
• Kamodo (Pembroke et al., 2022), which is an open-source Python toolkit for access, analysis and visualization of data.
• Executable papers (Gabriel and Capone, 2011; Lasser, 2020), which are described as Jupyter notebooks with all the software and data necessary to reproduce the work described in the paper.
4 Future challenges and efforts needed
The challenges and current efforts described in Section 2 comprise only a small portion of the discussions among scientists from different fields in the solar and heliospheric community; the main limitation was the short amount of time available during the meetings. In fact, the final point of the sessions was the need for creating a venue for regular discussions and exchange of ideas on these topics to optimize investment (e.g., NASA, NSF, ISSI, etc., grants opportunity), computational resources, and coordinate the solar/heliophysics community effort. For example, previous efforts such as the SIPWork (Solar Information Processing Workshop), should be revived and adapted to the new needs of our community. Similar workshops/working-groups are ongoing like the inaugural workshop Data, Analysis, and Software in Heliophysics (DASH) and the Geospace Data Assimilation Working Group (GeoDAWG).
The Solar Information Processing Workshop (SIPWork) series was initiated in 2003 to tackle the challenges of optimizing the science return of solar and heliospheric missions. It ran until 2014 and consisted of a series of workshops focusing on the evolving data challenges as the Heliophyiscs System Observatory (HSO) grew. SIPWork brought together different communities (solar and space scientists, statisticians, and data and image processing experts) to address the data analysis challenges of these missions. These highly popular meetings stimulated collaboration resulting in many proposals, and research papers. Major solar community initiatives like SunPy (sunpy.org—python open source The SunPy Community, 2015) arose from the SIPWork collaborations together with Special Issues in journal (Young and Ireland, 2008) and a book collecting developed tools (Ireland and Young, 2009). Website updates are underway at: http://www.sipwork.org/. We are currently working to organize the next workshop and expand it to include all of Heliophysics. Thus, the name will be SHIPWork (Solar and Heliospheric Information Workshop). This venue could potentially provide new opportunities including training/tutorials for scientists, for example, in designing user-friendly software tools using online repositories such as Github, and showcasing specific application of old and new tools.
Community needs highlighted in Section 2.2 should be further accompanied by community best practice to.
• Contact the instrument’s Principal Investigator for information.
• Teach new generation of young scientists the best-practices for proper documentation of data and software while also providing workshops to update mid-level scientists.
• Assure as much as possible the longevity of software (also paying attention to compatibility over time with new software versions).
• Advocate for proper referencing of software, data analysis tools and data products used in research activities.
• Deprecate proprietary tools and encourage open source software and toolchains to ensure reproducibility of science results and longevity of tools (e.g., NASA Open-Source Science Initiative). For example, the statistical language R provides a citing package which is important both to properly credit the developer and to allow for science reproducibility.
5 Conclusion
In the next decade, we should pursue the following.
• The continued standardization of tools and techniques for the analysis of heliospheric data.
• The establishment of web services for collecting and cataloging the tools themselves and their outputs; current examples are the Community Coordinated Modeling Center (CCMC), 2016; Hesse et al. (2002), Virtual Solar Observatory (VSO), 2022; Hill et al. (2004) and Heliophysics Events Knowledgebase (HEK), 2016; Hurlburt et al. (2012).
• Targeting investment in the full use of computational resource capabilities.
• Improving the synergy among observations, tools and models aimed at the study of the Sun-Earth system.
• Coordinating working groups (public and private) focused on these subjects, including computer scientists dedicated to optimizing software tools, under the guidance of an international dedicated core team.
• This coordination effort should also follow the Findable, Accessible, Interoperable and Reusable (FAIR) Barker et al. (2022) and Inclusion, Diversity, Equity and Accessibility (IDEA) principles.
We envision the community fully engaged in using and developing a library of open-source software tools and techniques that would be the basis for the extensive and optimized use of archived datasets, which set the standard for the way new observations are utilized. The documentation and examples from this library would be freely hosted through a comprehensive web interface (comparable to the current SAO/NASA Astrophysics Data System (ADS) for publications).
Data availability statement
The original contributions presented in the study are included in the article/Supplementary Material, further inquiries can be directed to the corresponding author.
Author contributions
NA was responsible for the organization of this article and contributed to all sections. All authors were involved in the preparation of the final manuscript and revisions before submission.
Funding
NA acknowledges support from NASA ROSES through HGI Grant No. 80NSSC20K1070 and PSP-GI grant No. 80NSSC21K1945. HM acknowledges Leverhulme Grant RPG-2019-361 to Aberystwyth University. The work of SD was supported under the NASA Grant No. 80NSSC21K0459 and PSP-GI Grant No. 80NSSC21K1945. AV was supported by NASA Grant No. 80NSSC22K0970. LB was supported under the NASA-LWS Grant No. 80NSSC19K0069. MM is supported by NASA Grants 80NSSC20K1445 and 80NSSC21K0725 to the Smithsonian Astrophysical Observatory.
Acknowledgments
Finally, the authors would like to thank the supporters of this work listed in the white paper for the Decadal Survey for Solar and Space Physics (Heliophysics) 2024–2033 (Alzate et al., 2023b).
Conflict of interest
Author NA was employed by the company ADNET Systems, Inc.
The remaining authors declare that the research was conducted in the absence of any commercial or financial relationships that could be construed as a potential conflict of interest.
Publisher’s note
All claims expressed in this article are solely those of the authors and do not necessarily represent those of their affiliated organizations, or those of the publisher, the editors and the reviewers. Any product that may be evaluated in this article, or claim that may be made by its manufacturer, is not guaranteed or endorsed by the publisher.
References
Alzate, N., Morgan, H., and Di Matteo, S. (2023a). Tracking nonradial outflows in extreme ultraviolet and white light solar images. Astrophysical J. 945, 116. doi:10.3847/1538-4357/acba08
Alzate, N., and Morgan, H. (2017). Identification of low coronal sources of “stealth” coronal mass ejections using new image processing techniques. Astrophysical J. 840, 103. doi:10.3847/1538-4357/aa6caa
Alzate, N., and Morgan, H. (2016). Jets, coronal “puffs,” and a slow coronal mass ejection caused by an opposite-polarity region within an active region footpoint. Astrophysical J. 823, 129. doi:10.3847/0004-637X/823/2/129
Alzate, N., Morgan, H., Viall, N., and Vourlidas, A. (2021). Connecting the low to the high corona: A method to isolate transients in STEREO/COR1 images. Astrophysical J. 919, 98. doi:10.3847/1538-4357/ac10ca
Alzate, N., Seaton, D. B., Kirk, M., Morgan, H., Di Matteo, S., West, M., et al. (2023b). “Data mining for science of the sun-earth connection as a single system,” in To appear in the bulletin of the American astronomical society.
Angelopoulos, V., Cruce, P., Drozdov, A., Grimes, E. W., Hatzigeorgiu, N., King, D. A., et al. (2019). The space physics environment data analysis system (SPEDAS). Space Sci. Rev. 215, 9. doi:10.1007/s11214-018-0576-4
Angelopoulos, V. (2008). The THEMIS mission. Space Sci. Rev. 141, 5–34. doi:10.1007/s11214-008-9336-1
Antunes, A. K., Winter, E., Vandegriff, J. D., Thomas, B. A., and Bradford, J. W. (2022). Profiling heliophysics data in the pythonic cloud. Front. Astronomy Space Sci. 9. doi:10.3389/fspas.2022.1006839
Barker, M., Chue Hong, N. P., Katz, D. S., Lamprecht, A.-L., Martinez-Ortiz, C., Psomopoulos, F., et al. (2022). Introducing the FAIR Principles for research software. Sci. Data 9, 622. doi:10.1038/s41597-022-01710-x
Barnes, W. T., Christe, S., Freij, N., Hayes, L. A., Stansby, D., Ireland, J., et al. (2023). The SunPy Project: An interoperable ecosystem for solar data analysis. Front. Astronomy Space Sci. 10, 1076726. doi:10.3389/fspas.2023.1076726
Barnum, J., Masson, A., Friedel, R. H., Roberts, A., and Thomas, B. A. (2022). Python in heliophysics community (pyhc): Current status and future outlook. Adv. Space Res. doi:10.1016/j.asr.2022.10.006
Burch, J. L., Moore, T. E., Torbert, R. B., and Giles, B. L. (2016). Magnetospheric multiscale overview and science objectives. Space Sci. Rev. 199, 5–21. doi:10.1007/s11214-015-0164-9
Burrell, A. G., Halford, A., Klenzing, J., Stoneback, R. A., Morley, S. K., Annex, A. M., et al. (2018). Snakes on a spaceship—an overview of Python in heliophysics. J. Geophys. Res. (Space Phys. 123, 10,384–10,402. doi:10.1029/2018JA025877
Camporeale, E. (2019). The challenge of machine learning in space weather: Nowcasting and forecasting. Space weather. 17, 1166–1207. doi:10.1029/2018SW002061
Candey, R. M., Harris, B. T., Kessel, R., Kovalick, T. J., Liu, M. H., McGuire, R. E., et al. (2018). “Importance of Heliophysics standards and metadata guidelines for effective data analysis,” in AGU fall meeting abstracts, 2018. IN11D–0651.
Community Coordinated Modeling Center (CCMC) (2016). Community coordinated modeling center (CCMC). Available at: https://ccmc.gsfc.nasa.gov.
DeForest, C. E., Howard, T. A., and McComas, D. J. (2013). Tracking coronal features from the low corona to earth: A quantitative analysis of the 2008 december 12 coronal mass ejection. Astrophysical J. 769, 43. doi:10.1088/0004-637X/769/1/43
Di Matteo, S., and Sivadas, N. (2022). Solar-wind/magnetosphere coupling: Understand uncertainties in upstream conditions. Front. Astronomy Space Sci. 9, 1060072. doi:10.3389/fspas.2022.1060072
Domingo, V., Fleck, B., and Poland, A. I. (1995). The SOHO mission: An overview. Sol. Phys. 162, 1–37. doi:10.1007/BF00733425
Escoubet, C. P., Schmidt, R., and Goldstein, M. L. (1997). Cluster - science and mission overview. Space Sci. Rev. 79, 11–32. doi:10.1023/A:1004923124586
Freeland, S. L., and Handy, B. N. (1998). Data analysis with the SolarSoft system. Sol. Phys. 182, 497–500. doi:10.1023/A:1005038224881
Friis-Christensen, E., Lühr, H., and Hulot, G. (2006). Swarm: A constellation to study the Earth’s magnetic field. Earth, Planets Space 58, 351–358. doi:10.1186/BF03351933
Gabriel, A., and Capone, R. (2011). Executable paper grand challenge workshop. Procedia Comput. Sci. 4, 577–578. Proceedings of the International Conference on Computational Science, ICCS 2011. doi:10.1016/j.procs.2011.04.060
Geospace Data Assimilation Working Group (GeoDAWG) (2023). Geospace data assimilation working group (GeoDAWG). Available at: https://sites.google.com/view/geodawg/home.
Gjerloev, J. W. (2012). The SuperMAG data processing technique. J. Geophys. Res. (Space Phys. 117, A09213. doi:10.1029/2012JA017683
Greenwald, R. A., Baker, K. B., Dudeney, J. R., Pinnock, M., Jones, T. B., Thomas, E. C., et al. (1995). Darn/superdarn: A global view of the dynamics of high-lattitude convection. Space Sci. Rev. 71, 761–796. doi:10.1007/BF00751350
Greisen, E. W., and Calabretta, M. R. (2002). Representations of world coordinates in FITS. Astronomy Astrophysics 395, 1061–1075. doi:10.1051/0004-6361:20021326
Grimes, E. W., Harter, B., Hatzigeorgiu, N., Drozdov, A., Lewis, J. W., Angelopoulos, V., et al. (2022). The space physics environment data analysis system in Python. Front. Astronomy Space Sci. 9, 1020815. doi:10.3389/fspas.2022.1020815
Habbal, S. R., Druckmüller, M., Morgan, H., Scholl, I., Rušin, V., Daw, A., et al. (2010). Total solar eclipse observations of hot prominence shrouds. Astrophysical J. 719, 1362–1369. doi:10.1088/0004-637X/719/2/1362
Häggström, I. (2014). “ESPAS — Near-earth space data infrastructure for e-science,” in 2014 XXXIth URSI General Assembly and Scientific Symposium (URSI GASS) 1–1. doi:10.1109/URSIGASS.2014.6929740
Hanser, F. A., and Sellers, F. B. (1996). “Design and calibration of the GOES-8 solar x-ray sensor: The XRS,” in GOES-8 and beyond of society of photo-optical instrumentation engineers (SPIE) conference series. Editor E. R. Washwell, 2812, 344–352. doi:10.1117/12.254082
Harten, R., and Clark, K. (1995). The design features of the GGS wind and polar spacecraft. Space Sci. Rev. 71, 23–40. doi:10.1007/BF00751324
Heliophysics Events Knowledgebase (HEK) (2016). Heliophysics events Knowledgebase (HEK). Available at: https://www.lmsal.com/hek/.
Hesse, M., Kuznetsova, M., Rastaetter, L., Keller, K., Falasca, A., Ritter, S., et al. (2002). “The community coordinated modeling center: A strategic approach to the transition of research models to operations,” in AGU spring meeting abstracts, 2002, SH51B–07.
Hill, F., Bogart, R. S., Davey, A., Dimitoglou, G., Gurman, J. B., Hourcle, J. A., et al. (2004). “The virtual solar observatory: Status and initial operational experience,” in Optimizing scientific return for Astronomy through information technologies of society of photo-optical instrumentation engineers (SPIE) conference series. Editor P. J. Quinn, and A. Bridger, 5493, 163–169. doi:10.1117/12.552842
Hurlburt, N., Cheung, M., Schrijver, C., Chang, L., Freeland, S., Green, S., et al. (2012). Heliophysics event Knowledgebase for the solar dynamics observatory (SDO) and beyond. Sol. Phys. 275, 67–78. doi:10.1007/s11207-010-9624-2
Ireland, J., and Young, C. A. (2009). Solar image analysis and visualization. doi:10.1007/978-0-387-98154-3
Judge, P. G. (1998). Spectral lines for polarization measurements of the coronal magnetic field. I. Theoretical intensities. Astrophysical J. 500, 1009–1022. doi:10.1086/305775
Kaiser, M. L., Kucera, T. A., Davila, J. M., Cyr, O. C., Guhathakurta, M., and Christian, E. (2008). The STEREO mission: An introduction. Space Sci. Rev. 136, 5–16. doi:10.1007/s11214-007-9277-0
Lasser, J. (2020). Creating an executable paper is a journey through Open Science. Commun. Phys. 3, 143. doi:10.1038/s42005-020-00403-4
Lin, H., Penn, M. J., and Tomczyk, S. (2000). A new precise measurement of the coronal magnetic field strength. Astrophysical J. Lett. 541, L83–L86. doi:10.1086/312900
Lin, R. P., Dennis, B. R., Hurford, G. J., Smith, D. M., Zehnder, A., Harvey, P. R., et al. (2002). The reuven ramaty high-energy solar spectroscopic imager (RHESSI). Sol. Phys. 210, 3–32. doi:10.1023/A:1022428818870
Lockwood, M., Owens, M. J., Barnard, L. A., Scott, C. J., Frost, A. M., Yu, B., et al. (2022). Application of historic datasets to understanding open solar flux and the 20th-century grand solar maximum. 1. Geomagnetic, ionospheric, and sunspot observations. Front. Astronomy Space Sci. 9, 960775. doi:10.3389/fspas.2022.960775
McComas, D. J., Bame, S. J., Barker, P., Feldman, W. C., Phillips, J. L., Riley, P., et al. (1998). Solar wind electron proton alpha monitor (SWEPAM) for the advanced composition explorer. Space Sci. Rev. 86, 563–612. doi:10.1023/A:1005040232597
Morgan, H., Jeska, L., and Leonard, D. (2013). The expansion of active regions into the extended solar corona. Astrophysical J. Suppl. 206, 19. doi:10.1088/0067-0049/206/2/19
Müller, D., Marsden, R. G., Cyr, St.O. C., and Gilbert, H. R. (2013). Solar orbiter: Exploring the sun–heliosphere connection. Sol. Phys. 285, 25–70. doi:10.1007/s11207-012-0085-7
The SunPy Community Mumford, S. J., Christe, S., Pérez-Suárez, D., Ireland, J., Shih, A. Y., Inglis, A. R., et al. (2015). SunPy-Python for solar physics. Comput. Sci. Discov. 8, 014009. doi:10.1088/1749-4699/8/1/014009
Paschmann, G., and Schwartz, S. J. (2000). “ISSI book on analysis methods for multi-spacecraft data,” in Cluster-II workshop multiscale/multipoint plasma measurements. Editor R. A. Harris (ESA Special Publication), 449, 99.
Pembroke, A., DeZeeuw, D., Rastaetter, L., Ringuette, R., Gerland, O., Patel, D., et al. (2022). Kamodo: A functional api for space weather models and data. J. Open Source Softw. 7, 4053. doi:10.21105/joss.04053
Pesnell, W. D., Thompson, B. J., and Chamberlin, P. C. (2012). The solar dynamics observatory (SDO). Sol. Phys. 275, 3–15. doi:10.1007/s11207-011-9841-3
Polarimeter to UNify the Corona and Heliosphere (PUNCH) (2023). Polarimeter to UNify the corona and heliosphere (PUNCH). Available at: https://punch.space.swri.edu/.
Roberts, D. A., Thieman, J., Génot, V., King, T., Gangloff, M., Perry, C., et al. (2018). The spase data model: A metadata standard for registering, finding, accessing, and using heliophysics data obtained from observations and modeling. Space weather. 16, 1899–1911. doi:10.1029/2018SW002038
Sheeley, N. R., Walters, J. H., Wang, Y. M., and Howard, R. A. (1999). Continuous tracking of coronal outflows: Two kinds of coronal mass ejections. J. Geophys. Res. 104, 24739–24767. doi:10.1029/1999JA900308
Sivadas, N., and Sibeck, D. G. (2022). Regression bias in using solar wind measurements. Front. Astronomy Space Sci. 9, 924976. doi:10.3389/fspas.2022.924976
Stenborg, G., Vourlidas, A., and Howard, R. A. (2008). A fresh view of the extreme-ultraviolet corona from the application of a new image-processing technique. Astrophysical J. 674, 1201–1206. doi:10.1086/525556
Sullivan, P. C. (2020). “Chapter 3 - goes-r series spacecraft and instruments,” in The GOES-R series. Editors S. J. Goodman, T. J. Schmit, J. Daniels, and R. J. Redmon (Elsevier), 13–21. doi:10.1016/B978-0-12-814327-8.00003-2
Thomas, B., Antunes, A., Yeakel, K., Bradford, J., Winter, E., Mo, W., et al. (2022). Heliocloud: An open cloud-based platform for heliophysics research. Bull. AAS 54.
Thompson, B. J., and Young, C. A. (2016). Persistence mapping using EUV solar imager data. Astrophysical J. 825, 27. doi:10.3847/0004-637X/825/1/27
Tritschler, A., Rimmele, T. R., Berukoff, S., Casini, R., Kuhn, J. R., Lin, H., et al. (2016). Daniel K. Inouye solar telescope: High-resolution observing of the dynamic Sun. Astron. Nachrichten 337, 1064–1069. doi:10.1002/asna.201612434
Virtual Solar Observatory (VSO) (2022). Virtual solar observatory (VSO). Available at: https://sdac.virtualsolar.org.
Wells, D. C., Greisen, E. W., and Harten, R. H. (1981). Fits - a flexible image Transport system. Astronomy Astrophysics Suppl. 44, 363.
Wills-Davey, M. J., and Thompson, B. J. (1999). Observations of a propagating disturbance in TRACE. Sol. Phys. 190, 467–483. doi:10.1023/A:1005201500675
Keywords: Sun-Earth connection, sun, data mining for science, data management, data interoperability, open-source, heliophysics decadal survey
Citation: Alzate N, Di Matteo S, Morgan H, Seaton DB, Miralles MP, Balmaceda L, Kirk MS, West M, DeForest C and Vourlidas A (2023) Data mining for science of the sun-earth connection as a single system. Front. Astron. Space Sci. 10:1151785. doi: 10.3389/fspas.2023.1151785
Received: 26 January 2023; Accepted: 06 April 2023;
Published: 14 April 2023.
Edited by:
Gian Luca Delzanno, Los Alamos National Laboratory (DOE), United StatesReviewed by:
Giovanni Lapenta, KU Leuven, BelgiumSteven Morley, Los Alamos National Laboratory (DOE), United States
Copyright © 2023 Alzate, Di Matteo, Morgan, Seaton, Miralles, Balmaceda, Kirk, West, DeForest and Vourlidas. This is an open-access article distributed under the terms of the Creative Commons Attribution License (CC BY). The use, distribution or reproduction in other forums is permitted, provided the original author(s) and the copyright owner(s) are credited and that the original publication in this journal is cited, in accordance with accepted academic practice. No use, distribution or reproduction is permitted which does not comply with these terms.
*Correspondence: Nathalia Alzate , nathalia.alzate@nasa.gov