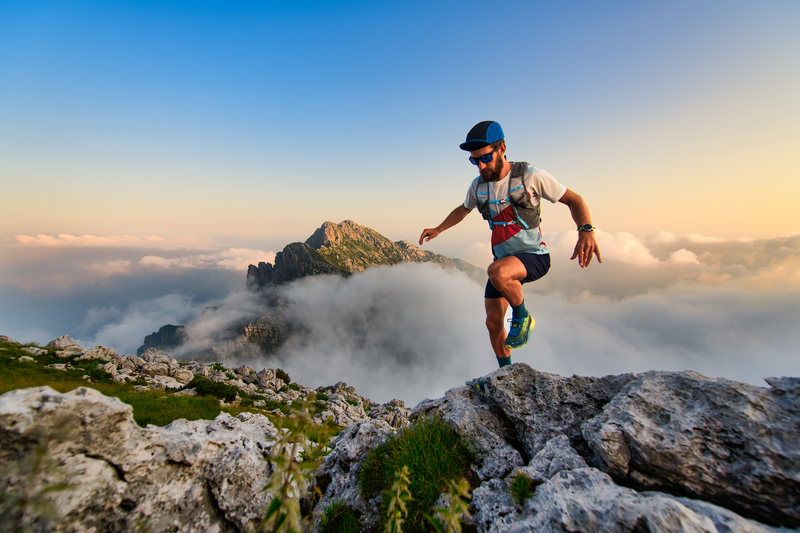
94% of researchers rate our articles as excellent or good
Learn more about the work of our research integrity team to safeguard the quality of each article we publish.
Find out more
SPECIALTY GRAND CHALLENGE article
Front. Astron. Space Sci. , 28 April 2020
Sec. Astrostatistics
Volume 7 - 2020 | https://doi.org/10.3389/fspas.2020.00011
Astrostatistics is not really a new discipline since it has long been recognized as a fundamental requirement for astrophysics (Feigelson and Babu, 1992a). However, it has been only quite recently accepted as a part of the astronomical community with the official creation of a commission of the International Astronomical Union. But, more importantly, sophisticated statistical tools have also pervaded the literature.
We can regret the lengthy time it has taken for awareness of astrostatistics to spread, but success has been achieved in this regard. Cosmology can be seen as the more spectacular demonstration of the usefulness, and even the absolute necessity, of sophisticated statistical analyses; it first started with the determination of the structure of the cosmic web (Barrow et al., 1985), but a more popular outcome emerged in the derivation of the properties of our Universe through Bayesian analyses.
As was already obvious a long time ago (Feigelson and Babu, 1992a), astronomers are not experts in statistics, and interdisciplinary collaborations are therefore necessary. This is routine practice in cosmology but not so much elsewhere, though things are changing rapidly.
The advent of Big Data in astrophysics is a more recent argument in favor of astrostatistics. The development of new telescopes, such as the LSST and SKA among many others, gave birth to astroinformatics for the purpose of managing the foreseen avalanche of data. As a consequence, statistical tools appear in the landscape of astrophysics and naturally foster interest for their use in data analysis.
It is striking to see that many techniques explored in the case of a particular astrophysical problem are never used again in other papers. One of the challenges of astrostatistics is to understand why. Is this because it is so technical, making it useable to only a very few? Is this because the interdisciplinary collaboration collapsed due to career pressures or a lack of funds? Is this because the results did not convince the other astronomers since they could not trust a method that they did not understand? Is this because the results were not easily connectible with the current physical knowledge and understanding of the subject? Or is this simply because statistical methods introduce a different avenue from the traditional way of doing astrophysics?
Doing physics with Machine Learning outcomes is not a specific difficulty of astrophysics, and it appears as a cultural revolution that is sometimes referred to as the transition from object-driven to data-driven science. Physics is deterministic, but cosmology has largely proven that we can use likelihood to predict and assess the most probable parameter values from observations. Indeed, the relative popularity of the Bayesian approach in astrophysics is certainly related to the uncertainties that are unavoidably attached to astronomical data and can seemingly be incorporated easily into such methods. Uncertainties and noise are a specificity of astronomical data that may deter some statisticians or motivate others.
A counter example to the success of Bayesian statistics in astrophysics is that, other than a very pedagogical paper by Feigelson and Babu (1992b), astronomers use nearly exclusively the χ-square minimization regression-type problems without checking the validity of this method for the case under study. The other methods are not part of the basic toolbox of most astronomers, especially for the common truncated and censored data. In the same manner, it is not because the Bayesian approach is popular that it has always been fully understood: in particular, it is sometimes forgotten that the choice of using the prior is arbitrary and can have a significant influence on the result.
It is true that statistics is a huge domain, and every single astrophysical analysis could benefit from sophisticated methods and algorithms. It is thus very difficult for an astronomer to be aware of the state of the art of another discipline. But there is a plethora of robust, available techniques that are not too difficult to understand mathematically. Thanks to many training sessions, workshops, books, and, of course, papers, it is now becoming easier and easier to fill the gap between the two disciplines.
The main difficulty is somehow cultural. Astronomers were used to work with small data sets, describing the diversity by eye and concentrating on the detailed physics of so-called typical objects. The big challenge of the twenty-first century is certainly that eye examination is not possible any more, and, consequently, the scientists have to rely on automatic machines to do the job. This causes serious issues, and only a few are presented below.
Grouping objects obtained from the astronomical observations into distinct categories has always been a necessity imposed by their vast diversity. This is the case for stars, galaxies, asteroids, supernova, active galactic nuclei, gamma-ray bursts, and many others. This clustering (unsupervised classification) is a prerequisite to any physical modeling. For this purpose, astronomers have always used heuristic, simple, and subjective techniques, based on only one or two physical parameters and most often with the help of a visual examination. But the classification algorithms do not always respect the physical properties, they merely distinguish classes on purely mathematical grounds. It often means that the classification is justified only in a multivariate space and that it cannot be summarized by a very few physical characteristics. What, then, can the astronomer make of such a classification (e.g., Fraix-Burnet et al., 2015)?
In addition, with more and more data, the diversity increases, populating the parameter space, which, in astrophysics, is essentially a continuum of object properties without clear boundaries. As a consequence, any reasonable classification is fuzzy, making it more difficult to summarize with simple schemes.
One illustrative instance of this issue is the classification of galaxies. A quantitative and objective classification of the morphologies of galaxies is still an unsolved problem. From dimensionality reduction with Principal Component Analysis, first attempted nearly 40 years ago (Whitmore, 1984; Watanabe et al., 1985), to computationally heavy Deep Learning techniques, no satisfactory solution has been yet found. It is clear that the visual Hubble morphological classification is much too appealing emotionally (as it is simple and beautiful) to be easily replaced by something more thorough but more complex. Nevertheless, “Nature dictates the classification to the scientists,” not the reverse (Adanson, 1763).
The classification of galaxy spectra suffers from the same fate (e.g., Connolly et al., 1995), and this again is due to our inability to see in a high-dimensional space. One can argue that the stellar classification seems to be robust and multivariate, but stars are obviously less complex objects than galaxies.
A big issue of science is robustness and reproducibility. Astronomers are used to checking the validity of a result by examining visually any image or summarizing plot. This is unfortunately not objective, quantitative, or reproducible. Supervised machine learning promised much since the philosophy is to teach the algorithm what we would like it to yield, including human subjectivity. The control is straightforward. But is this really feasible? On the contrary, the exploration of the data requires unsupervised learning, but how can we trust the findings of the algorithms? How can we describe them in a multivariate configuration? How can we summarize the results in simple terms?
Most importantly, even if there are statistical tools to assess the robustness and reproducibility of the outcomes of algorithms, only the physics can validate a result in the sense that it can be useful to our understanding of the Universe. But how can we reconcile these new kinds of data analysis with the physical models? Most theoretical calculations are devised to mimic a single object, and they can be repeated by varying the input parameters. However, the more sophisticated the models are, the heavier the computational cost is; it is probably unrealistic in many cases to produce a statistical analysis of the outcomes of models. Different approaches are used to fit different kinds of objects, such as interpolation, but this might not fully address our area of concern.
Numerical simulations are a recent tool in astrophysics, the cosmological ones in particular, which create a very large diversity of galaxies. It seems to be possible to compare these data with real observations using the same statistical tools. But would it be enough to constrain the very complicated physics that are introduced in the simulations and test various hypotheses or some ad hoc recipes that are often necessary to somewhat lighten the computation?
In a multivariate world, the selection of the attributes is useful, if not crucial. This is often necessary to perform the analysis, and very helpful for the interpretation. This is called dimensionality reduction, and among them the Principal Component Analysis is well-known in astrophysics. But others also exist, such as Discriminant Analysis or Independent Component Analysis. They all have their interests and limitations, but they all share the common drawback of providing unphysical components in the form of combinations of the real observables or physical parameters. Astronomers will likely have to learn to do physics in these artificial spaces as they already do to, for instance, separate stars from galaxies in Principal Component bivariate plots. They may even create many artificial features to improve their regression analyses (e.g., D'Isanto et al., 2018) and envisage clustering of variables.
Time series are ubiquitous in astrophysics, from celestial mechanics to gravitational waves and from exoplanets to quasars, with phenomena that are periodic (orbits, cycles, pulses, rotations, etc.), transient (explosions, bursts, stellar activity, etc.), random (accretion and ejection), or regular (apparent motions). In astrophysics, the detection can be immediate, to alert other telescopes, or very detailed, to identify some exoplanets or probe the interior of stars. The characterization of the light curves is required for the physical modeling and understanding. Classification is, of course, necessary to organize the observations. Time series analysis is widespread in many other disciplines (meteorology, finance, economy, medical sciences, etc.) and is an important branch of research in statistics with significant developments that astronomers often ignore. There is here an important potential for new studies and discoveries.
Graphical methods are relatively under-explored in astrophysics. Yet, the Hubble tuning fork diagram may be seen as a rather old though still extremely popular graphics method depicting the evolution of galaxies. Also, the Minimum Spanning Tree method was used early on to map the cosmic web using two spatial coordinates and the redshift (Barrow et al., 1985). These tools are particularly suited to represent evolutionary paths and are heavily used in bioinformatics. Their use in astrophysics is recent (Fraix-Burnet et al., 2006), and they have already seen many applications to small solar system bodies, stars, stellar clusters, Gamma Ray Bursts, galaxies, and quasars.
Astrostatistics is thus full of challenges and opportunities. We hope that this section of Frontiers in Astronomy and Space Sciences will become a home for experiments, discussions, propositions, and solutions, a place where astronomers and statisticians can come together and where anyone can find research articles, reviews, opinions, codes, and tutorials that can accompany the data science revolution astrophysics is beginning to experience.
The author confirms being the sole contributor of this work and has approved it for publication.
The author declares that the research was conducted in the absence of any commercial or financial relationships that could be construed as a potential conflict of interest.
Adanson, M. (1763). Famille Des Plantes (chez Vincent, impr.-libraire de Mgr le Comte de Provence (Paris)). Num. BNF de l'éd. de. Paris: INALF, 1961- (Frantext; R263Reprod. de l'éd. de, Paris: Vincent).
Barrow, J. D., Bhavsar, S. P., and Sonoda, D. H. (1985). Minimal spanning trees, filaments and galaxy clustering. Mon. Not. R. Astron. Soc. 216, 17–35.
Connolly, A. J., Szalay, A. S., Bershady, M. A., Kinney, A. L., and Calzetti, D. (1995). Spectral classification of galaxies: an orthogonal approach. Astron. J. 110:1071. doi: 10.1086/117587
D'Isanto, A., Cavuoti, S., Gieseke, F., and Polsterer, K. L. (2018). Return of the features. Astron. Astrophys. 616:A97. doi: 10.1051/0004-6361/201833103
Feigelson, E. D., and Babu, G. J. (1992a). “Improving the Statistical Methodology of Astronomical Data Analysis,” Astronomical Society of the Pacific Conference Series, Vol. 25, 237. Available online at: http://aspbooks.org/a/volumes/article_details/?paper_id=7118
Feigelson, E. D., and Babu, G. J. (1992b). Linear regression in astronomy. II. Astrophys. J. 397:55. doi: 10.1086/171766
Fraix-Burnet, D., Choler, P., and Douzery, E. (2006). Towards a phylogenetic analysis of galaxy evolution: a case study with the Dwarf Galaxies of the Local Group. Astron. Astrophys. 455, 845–851. doi: 10.1051/0004-6361:20065098
Fraix-Burnet, D., Thuillard, M., and Chattopadhyay, A. K. (2015). Multivariate approaches to classification in extragalactic astronomy. Front. Astron. Space Sci. 2:3. doi: 10.3389/fspas.2015.00003
Watanabe, M., Kodaira, K., and Okamura, S. (1985). Digital surface photometry of galaxies toward a quantitative classification. iv - principal component analysis of surface-photometric parameters. Astrophys. J. 292, 72–78.
Keywords: statistics, data analysis - methods, interdiscipinarity, future prospects, astrophysics and astronomy
Citation: Fraix-Burnet D (2020) Grand Challenges in Astrostatistics. Front. Astron. Space Sci. 7:11. doi: 10.3389/fspas.2020.00011
Received: 02 March 2020; Accepted: 16 March 2020;
Published: 28 April 2020.
Approved by:
Julio Navarro, University of Victoria, CanadaCopyright © 2020 Fraix-Burnet. This is an open-access article distributed under the terms of the Creative Commons Attribution License (CC BY). The use, distribution or reproduction in other forums is permitted, provided the original author(s) and the copyright owner(s) are credited and that the original publication in this journal is cited, in accordance with accepted academic practice. No use, distribution or reproduction is permitted which does not comply with these terms.
*Correspondence: Didier Fraix-Burnet, ZGlkaWVyLmZyYWl4LWJ1cm5ldEB1bml2LWdyZW5vYmxlLWFscGVzLmZy
Disclaimer: All claims expressed in this article are solely those of the authors and do not necessarily represent those of their affiliated organizations, or those of the publisher, the editors and the reviewers. Any product that may be evaluated in this article or claim that may be made by its manufacturer is not guaranteed or endorsed by the publisher.
Research integrity at Frontiers
Learn more about the work of our research integrity team to safeguard the quality of each article we publish.